Abstract
The contents of individual and total glucosinolate in Chinese kale (Brassica albograbra Bailey) were determined and analyzed using near-infrared spectroscopy. The near infrared calibration mathematics model between total glucosinolate contents and spectral measurement was established based on modified partial least squares. The coefficient of determination of the cross-validation (1-VR), coefficient of determination in prediction and ratio of the standard deviation to the standard error of the cross-validation of the near-infrared spectroscopy calibration equations were 0.92, 0.91, and 3.59 for total glucosinolates; 0.87, 0.82, and 2.78 for gluconapin; 0.93, 0.93, and 3.84 for glucobrassicin; and 0.86, 0.90, and 3.70 for neoglucobrassicin. Near-infrared spectroscopy showed high accuracy in predetermining individual and total glucosinolate contents in Chinese kale.
INTRODUCTION
Glucosinolates (β-thioglucoside-N-hydroxysulphates) are abundant plant secondary metabolites that are found in cruciferous plants[Citation1] and contain a side chain R and a sulfur atom linked D-pyranoid glucose. Glucosinolates have the same basic structure () as shown by X-ray analysis.[Citation2]
The structural diversity of this large group of compounds is due almost entirely to the different substituents possible at the side-chain position R. The R substituent may be an alkyl or alkenyl side-chain, which itself may contain substituent hydroxyl groups or sulfur atoms. Alternatively, the R substituent may be an aromatic or a heteroaromatic group.[Citation3]
The hydrolysis products of glucosinolates are of great interest because they are responsible for many of the beneficial effects of plants containing glucosinolates.[Citation4,Citation5] Glucosinolates have many beneficial effects, such as their antibacterial and antifungal properties,[Citation6,Citation7] and they may act as cancer chemo-prevention agents.[Citation8] Sulforaphane, one of the degradation products of glucosinolates, not only has anti-carcinogenic potential,[Citation9−Citation11] but also produces flavor in cruciferous vegetables.[Citation12]
The glucosinolates in Brassica species have shown some toxic and anti-nutritive effects.[Citation13,Citation14] One of the negative aspects of glucosinolates is the goitrogenic effects in animals of glucosinolate-containing plants.[Citation12,Citation15] One hundred twenty glucosinolates have been found in plants, particularly in 11 dicotyledonous plant groups: Cruciferae, Capparaeae, Tovariaceae, Resedacea, Moringacea, Limnanthaceae, Caricacea, Tropaeolaceae, Gyrostermonaceae, Salvadoraceae, and Euphorbiaceae.[Citation16] Chinese kale (Brassica albograbra Bailey) is a species that is consumed in considerable quantities as a traditional vegetable in China and shows major qualitative and quantitative differences in glucosinolate composition between cultivars.[Citation17]
Reverse phase HPLC quantitative analysis of desulfurized glucosinolates was established by Spinks et al.[Citation18] and has been widely used by many researchers since then. In 1990, the method was confirmed as the official reference method by the European Union.[Citation19] Near-infrared spectroscopy (NIRS) is characterized by low molar absorptivities and scattering, which allows nearly effortless evaluation of pure materials. The near-infrared (NIR) region of the electromagnetic spectrum, once regarded as having little potential for analytical work, has now become one of the most promising areas for molecular spectroscopy. The arrival of inexpensive and powerful computers has contributed to the surge in NIR applications in several fields, including the medical and pharmaceutical fields and in traditional food analysis.[Citation20−Citation24]
The standard analytical techniques for determining glucosinolate composition using conventional methods are costly, require a high labor input, and create delays, all of which affect both the availability of data and swift decision making.[Citation25] In contrast, the use of fast analytical techniques, such as NIRS, have many advantages since analysis can be carried out with a considerable saving of time, at a lower cost and without using hazardous chemicals.
Many authors have used this technique for determining the glucosinolate content in different plant materials from Brassica species.[Citation26−Citation32] The purpose of the current work was to test the potential use of NIRS for predicting the total and individual glucosinolate content in different cultivars of Chinese kale. In addition, some information provided mechanism used by NIRS for the successful determination of glucosinolates in this species.
MATERIALS AND METHODS
Plant Samples
The Chinese kale samples were provided by Beijing Vegetable Research Center (Beijing, China). Freshly harvested individual plants of Chinese kale were collected at different stages of maturity to increase the chemical and physical variability in the samples. The samples were cut into 1.0 cm lengths, freeze-dried, ground into a powder, uniformly mixed, and then stored at –40°C.
HPLC Analysis
Quantitative analysis of desulfurized glucosinolates was carried out by HPLC. Glucosinolate extraction was carried out in a water bath at 80°C to inactivate myrosinase. 0.2 g (±0.0003 g) of the sample powder was mixed with 0.20 ml (67.1 mg glucotropaeolin dissolved in 50 ml double distilled water) with TRO (Glucotropaeolin) as an internal standard, and then rapidly infused with methanol at 80°C. After centrifugation (10 min, 3000 r min−1), the precipitate was extracted twice using 70% aqueous methanol. 2 ml of the combined glucosinolate extracts was filtered using a column containing 2 ml DEAE gel. Desulfurization was carried out by adding 75 μl of sulfatase (EC 3.1.6.1) solution. Desulfurized glucosinolates were eluted with 1.5 ml ultra-pure water three times (0.5 ml × 3) and were filtered through a 0.45 μm nylon membrane-filter. HPLC analyses were performed using a Model LC-20A HPLC instrument (Shimadzu) equipped with a Model SPD-20AD detector (Shimadzu). Glucosinolate separation was performed using a Nova-Pak C18 column (3.9 mm×150 mm, 5 μm) at room temperature, with a detection wavelength of 229 nm and injection volume of 20 μl. Mobile phases consisted of 0.05 % tetramethylammonium chloride aqueous solution (A) and 0.05 % tetramethylammonium chloride with 20% acetonitrile aqueous solution (B) at a flow rate of 1 ml min−1. The gradient condition started at 100% A from 0 to 6 min and was linearly decreased to 0% A at 21 min, and then increased back to 100% A at 26 min. The individual glucosinolate content of each sample was calculated using the internal standard (Formula (1)) and expressed as μmol g−1 of dry weight (dw). The total glucoinolate content was calculated as the sum of all the individual glucosinolates.
W: content of GSL; GPA: peak area of desulfurized GSL; IS: content of internal standard; R: correction index of desulfurized GSL; IPA: peak area of internal standard; M: weight of dried sample.
NIRS Analysis
Near-infrared spectra from all the samples were recorded using a Model 6500 NIRS spectrometer (Foss NIRSystems, Inc., Silver Spring, MD, USA) in reflectance mode. All the Chinese kale samples, which were freeze-dried and ground, were placed in a rectangular sample holder, and their spectra acquired within the range 400 to 2500 nm, at 2 nm intervals. A built-in polystyrene ceramic wafer was used as a reference standard before a spectrum was produced. Each sample spectrum was an average of three recordings and each spectrum record was an average of 32 scans over the sample. In this study, the sample spectra were used to generate the log (1/R; R is reflectance) spectrum using the matching software. A calibration file was formed by adding the reference chemistry values of all glucosinolates obtained by HPLC to the file of spectra.
Using the software WINISI III (Infrasoft International, LLC, Port Matilda, PA, USA), the difference between each sample spectrum and the average bands was computed and expressed as standardized Mahalanobis distances. The sample spectrum data was deleted from the following analysis, if it was exceptional[Citation33] because it may have been caused by improper preparation or operator error. Calibration equations for PRO, SIN, RAA, NAP, 4OH, GBC, 4ME, NEO, and total-GSL were developed using the WINISI III software. The spectra used to develop the equations were processed with transformed combinations of the standard normal variate (SNV), de-detrending (DT) and algorithms (2 [derivative order], 5 [segment of the derivative], 5 [first smooth], 2 [second smooth]). The calibration equations were developed using modified partial least squares (MPLS) as a regression method. Performance of the different calibration equations was determined from cross-validation and the standard deviation (SD), standard error of cross-validation (SECV) and coefficient of determination of the cross-validation (1-VR) were computed. In addition, 30 unknown Chinese kale samples were used to verify the established calibration equations as an independent validation set. These 30 samples were analyzed using HPLC and NIRS. This meant that a more realistic estimate of the applicability of NIRS for GSL analysis could be given.
RESULTS AND DISCUSSION
HPLC analysis of desulfurized glucosinolates is the qualitative and quantitative analysis of glucosinolates that have been processed by sulfatase in order to detach the sulfonic acid oxime. The glucosinolates desulfurization period was long enough (over 12 h on average) to enable the glucosinolates to be totally desulfurized. Using this analysis method, different desulfurized glucosinolates separated well; peaks were distinguished and identified more easily than those of intact glucosinolates and retention times were comparatively constant. In addition, the useful service life of the analytical columns was lengthened to a certain extent.[Citation34] The use of TRO (Glucotropaeolin) as an internal standard and correction factor was successful.[Citation35,Citation36] Analysis of desulfurized glucosinolates was feasible and practical; its superiority being particularly prominent when there was a large number of samples. However, if acyl was present in the samples, desulfurization was difficult making the analysis result less accurate because of the interference of the flavonoid sulfuric acid ester. Furthermore, when the myrosinase content in the samples was high, then the determination of internal standards was affected and this also led to a low accuracy level.
This study found that the glucosinolates found in Chinese kale, were Progoitrin (PRO) (2-hydroxy-3-butenyl-glucosinolate), Sinigrin (SIN) (2-propenyl-glucosinolate), Glucoraphanin (RAA) (4-(methylsulfonyl)butyl-glucosinolate), Gluconapin (NAP) (3-butenyl-glucosinolate), 4-Hydroxyglucobrassicin (4OH) (4-hydroxy-3-indolylmethyl-glucosinolate), Glucobrassicin (GBC) (3-indolylmethyl-glucosinolate), 4-Methoxyglucobrassicin (4ME) (4-methoxy-3-indolylmethyl-glucosinolate) and Neoglucobrassicin (NEO) (1-methoxy-3-indolylmethyl-glucosinolate; and ). Glucosinolates were tentatively identified by comparison of retention times with those of certified standards. The frequency distributions and mean content of total and individual glucosinolates are shown in . The mean content of total glucosinolates (t-GSL; ) was 19.85 μmol g−1 dw and individual content varied between 2.35 and 58.25 μmol g−1 dw. Different cultivars, different planting seasons and different planting places were possible reasons for the variation in t-GSL content found between the samples.[Citation37] The mean contents of PRO (), SIN (), RAA (), NAP () and GBC () were over 1 μmol g−1 dw. NAP was the most common glucosinolate in Chinese kale (10.88 μmol g−1 dw), representing 54.81% of the t-GSL, followed by RAA (2.30 μmol g−1 dw) and GBC (2.30 μmol g−1 dw). Other glucosinolates, such as 4OH () and 4ME (), had mean contents under 0.2 μmol g−1 dw. The t-GSL (), and individual glucosinolates PRO (), RAA (), NAP (), 4ME (), exhibited approximately normal distributions over their intervals.
Figure 2 HPLC chromatogram of glucosinolates. (a) Internal standard TRO. (b) Glucosinolates in a sample.
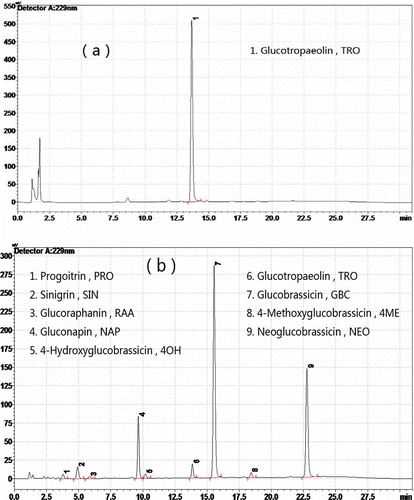
Figure 3 Frequency distribution plots (μmol g dw−1). (a) Total glucosinolates. (b) Progoitrin. (c) Sinigrin. (d) Glucoraphanin. (e) Gluconapin. (f) 4-Hydroxyglucobrassicin. (g) Glucobrassicin. (h) 4-Methoxyglucobrassicin. (i) Neoglucobrassicin.
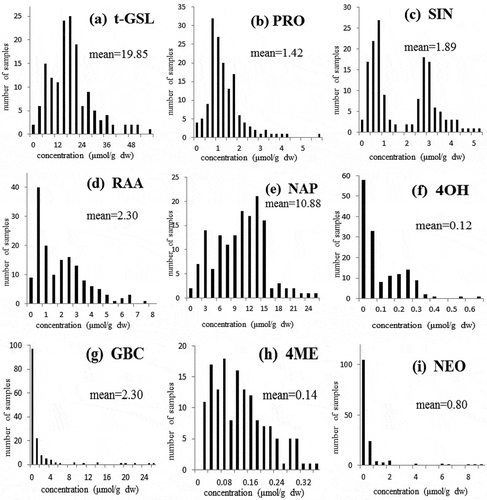
The raw spectra (log 1/R) obtained by NIRS spectrometer are shown in . The raw spectra were processed using the second derivative and SNV+DT algorithms to eliminate the baseline shift caused by differences in particle size and path length. This resulted in the second derivative spectra (2, 5, 5, 2; SNV + DT) of the raw optical data falling in the range 400 to 2500 nm (). The peaks and troughs in corresponded to the points of maximum curvature in the raw spectra () and there were troughs corresponding to each peak in the original spectra. The overlapping peaks in the raw spectra were clearly separated out in the second derivative spectra.
Figure 4 Raw spectra (log 1/R) of the samples of Chinese kale used in this study (n = 150) in the range 400 to 2500 nm.
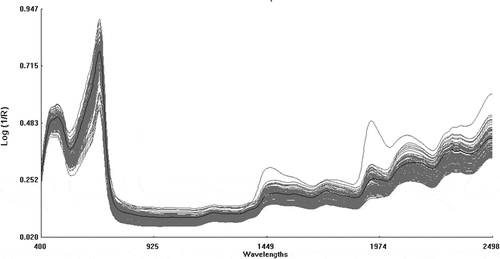
Different NIRS calibration equations for PRO, SIN, RAA, NAP, 4OH, GBC, 4ME, NEO, and t-GSL were developed using the calibration set data processed by SNV+DT and algorithms 2, 5, 5, and 2. NIRS calibration equations were estimated by internal cross-validation with the calibration data set and externally validated using data from the validation set. Internal cross-validation is similar to external validation in that it is based on independent external data. Internal cross-validation is carried out by dividing calibration set into several groups and then each time testing one group of the calibration set. This test method was useful and efficient because all available data from the chemical analyses can be used to determine the calibration equation without the need to maintain separate validation and calibration sets.
In this study, internal cross-validation and external validation were both used to estimate the NIRS calibration model. Estimated performance, which included the number of samples used for developing the NIRS calibration equations, mean content of different glucosinolates, some statistical parameters SD, SECV, 1-VR (coefficient of determination of the cross-validation), SD/SECV (ratio of the standard deviation to the standard error of cross-validation), RSQ (coefficient of determination in prediction), and SEP (standard error of prediction) used in the NIRS calibration model are displayed in . The prediction ability of the NIRS calibration equations developed was estimated using SECV, 1-VR (coefficient of determination of the cross-validation), RSQ, and SEP. When the standard error values are low and the coefficients of determination draw near to one, then the prediction ability of calibration models is stronger. The prediction ability of the calibration models were estimated in more detail using the SD/SECV. If SD/SECV ≥ 3, then the performance of the calibration models was excellent and could be used for actual testing; between 2.5< SD/SECV< 3, then the calibration models were appropriate for quantitative analysis; if SD/SECV< 2.5, then the calibration models were difficult to use for quantitative analysis ().[Citation38]
Table 1 NIRS calibration and validation statistics for the different equations developed for glucosinolates
Figure 5 Second derivative spectra (2, 5, 5, 2; SNV + DT) of the raw optical data in the range 400 to 2500 nm.
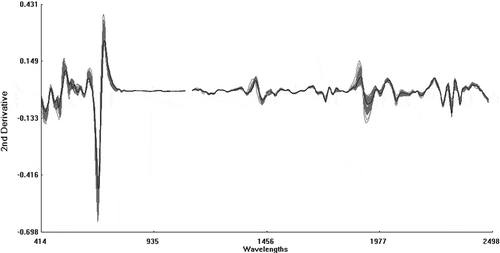
The stability and accuracy of the NIRS calibration equations used in NIRS for assessing the t-GSL content, PRO, SIN, RAA, NAP, 4OH, GBC, 4ME, and NEO in Chinese kale were evaluated. The t-GSL equation showed a high coefficient of determination for cross-validation and prediction (1-VR = 0.92, RSQ = 0.91; ), a low SECV and prediction (SECV= 2.96 μmol g−1 dw, SEP= 2.87 μmol g−1 dw), and a high SD/SECV (3.59) ratio. Similarly, the equations for NAP, GBC and NEO showed a high coefficient of determination in cross-validation (1-VR = 0.87, 0.93, 0.86, respectively ), a high RSQ (RSQ = 0.82, 0.93, 0.90, respectively; , , and ), low SECV (SECV = 1.87, 1.22, 0.44 μmol g−1 dw, respectively ), low SEP (SEP = 2.19, 1.04, 0.39 μmol g−1 dw, respectively), and high ratios of SD/SECV (2.78, 3.84, 3.70, respectively). The ratios of SD/SECV for t-GSL, GBC and NEO were greater than three, and NAP was between 2.5 and 3. So the calibration models for t-GSL, NAP, GBC and NEO can be used for accurate quantitative analysis. In contrast, the equations for PRO and 4ME showed lower coefficients of determination in the cross-validation (1-VR = 0.69, 0.71, respectively) in prediction (RSQ = 0.34, 0.58, respectively; , ), and low ratios of SD/SECV (2.32, 1.96, respectively). The calibration equation for SIN showed higher 1-VR (0.83) and RSQ (0.86; ), but lower SD/SECV (2.32), which was less than 2.5. The separation in frequency distribution of SIN () led to a higher SECV, resulting in a lower SD/SECV. Although the calibration equation for RAA showed high SD/SECV (3.18), the RSQ (0.67; ) was low. Similarly, the calibration equation for 4OH showed a higher SD/SECV (2.70), but the RSQ () was only 0.64. The validity of using cross-validation to evaluate the performance of NIRS equations has been reported and applied successfully by Font[Citation30] and Liu.[Citation39] Cross-validation resulted in SD/ SECV ratios that ranged from 1.96 (4ME) to 3.84 (GBC) (). The higher 1-VR of t-GSL and GBC with respect to other individual glucosinolates led to a lower SECV, and resulted in a high SD/SECV value. The very low contents showed by 4OH (mean value: 0.12 μmol g−1 dw) and 4ME (mean value: 0.14 μmol g−1 dw) in the Chinese kale samples would make them more prone to errors in obtaining the reference values by HPLC. This would lead to a decreasing correlation with the spectral information.
Figure 6 Scatter plots (n = 30). (a) Total glucosinolates. (b) Progoitrin. (c) Sinigrin. (d) Glucoraphanin. (e) Gluconapin. (f) 4-Hydroxyglucobrassicin. (g) Glucobrassicin. (h) 4-Methoxyglucobrassicin. (i) Neoglucobrassicin. Abscissa axis: laboratory values of glucosinolates by HPLC (μmol g dw−1); vertical axis: predicted values of glucosinolates by NIRS (μmol g dw−1).
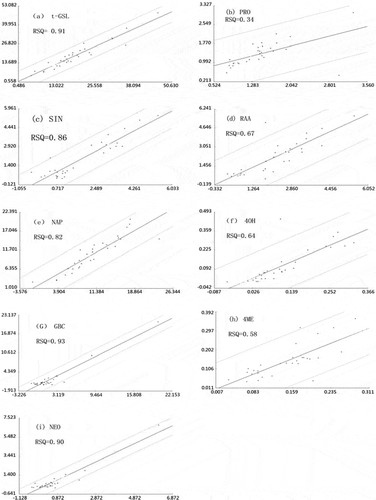
However, the NIRS mathematical model was based on a large body of chemical analysis data. The accuracy and analysis results were related, to a great extent on the chemical analyses. The quantification prediction ability of the NIRS mathematical model will continuously improve with the accumulation of better data and the gradual optimization of the NIRS model.
CONCLUSIONS
The results of the present study indicate that NIRS is feasible and showed a high accuracy in predetermining the t-GSL content and the individual glucosinolate content in Chinese kale. This study concludes that NIRS can be used to predict t-GSL, NAP, GBC, and NEO by quantitative analysis of unknown Chinese kale samples and is an accurate and quick analytical method. It will save labor input, time, and analytical costs. This is particularly true of Chinese kale variety screening, which can now be done without the need for HPLC analysis.
REFERENCES
- Shan, Y.Q.; Zhang, J.L.; He, H.J. The property research of glucosinolate and sulforaphane in Cruciferae Plants. Food Science and Technology 2007, 32, 110–111.
- Marsh, R.E.; Waser, J. Refinement of the crystal structure of Singrin. Acta Crystallographica Section B 1970, 26, 1030–1037.
- Bones, A.M.; Rossiter, J.T. The myrosinase-glucosinolate system, its organisation and biochemistry. Plant Physiology 1996, 97, 194–208.
- Rosa, E.A.S.; Heaney, R.K. The effect of cooking and processing on the glucosinolate content: Studies on four varieties of Portuguese cabbage and hybrid white cabbage. Journal of the Science of Food and Agriculture 1993, 62, 259–265.
- Font, R.; Del Rio-Celestino, M.; Rosa, E.; Aires, A.; De Haro-Bailon, A. Glucosinolate assessment in Brassica olerace leaves by near infrared spectroscopy. Journal of Agricultural Science 2005, 143, 65–73.
- Angus, J.F.; Gardner, P.A.; Kirkegard, J.A.; Desmarchelier, J.M. Biofumigation: Isothio-cyanates released from Brassica roots inhibit growth of the take-all fungus. Plant and Soil 1994, 162, 107–112.
- Fahey, J.W.; Zalcmann, A.T.; Talalay, P. The chemical diversity and distribution of glucosinolates and isothiocyanates among plants. Phytochemistry 2001, 56, 5–51.
- Shapiro, T.A.; Fahey, J.W.; Wade, K.L.; Stephenson, K.K.; Talalay, P. Chemoprotective glucosinolates and isothiocyanates of broccoli sprouts: Metabolism and excretion in humans. Cancer Epidemiology, Biomarkers and Prevention 2001, 10, 501–508.
- Xiu, L.L.; Niu, K.L. Glucosinolates and its degraded products in cruciferous plants. Journal of Zhejiang University of Science and Technology 2004, 16, 187–189.
- Melinda, C.M.; Roderick, H.D. Chemoprotection by sulforaphane: Keep one eye beyond Keap1. Cancer Letters 2006, 233, 208–218.
- Yuan, P.; Chen, B.A.; Liu, D.L. Anticancer mechanisms and researches of isothiocyanates. Chinese Journal of Natural Medicines 2008, 6, 325–332.
- Rosa, E.A.S.; Heaney, R.H.; Fenwick, G.R.; Portas, G.A.M. Glucosinolates in crop plants. Horticultural Reviews 1997, 19, 99–215.
- Wallig, M.A.; Belyea, R.L. Tumbleson, M.E. Effect of pelleting on glucosinolates content of Crambe meal. Animal Feed Science and Technology 2002, 99, 205–214.
- Tanii, H.; Takayasu, T.; Higashi, T.; Leng, S.; Saijoh, K. Allylnitrile: Generation from cruciferous vegetables and behavioral effects on mice of repeated exposure. Food and Chemical Toxicology 2004, 42, 453–458.
- Wilkinson, A.P.; Rhodes, M.J.C.; Fenwick, G.R. Myrosinase activity of cruciferous vegetables. Journal of the Science of Food and Agriculture 1984, 35, 543–552.
- McGregor, D.I.; Mullin, W.J.; Fenwick, G.R. Analytical methodology for determining glucosinolate composition and content. Journal of the Association of Official Analytical Chemists 1983, 66, 825–849.
- He, H.J.; Song, S.H.; Wang, W.Q.; Wu, X.D. HPLC identification of intact glucosinolates in Chinese kale. Modern Instruments 2002, 5, 10−12.
- Spinks, E.A.; Sones, K.; Fenwick, G.R. The quantitative analysis of glucosinolates in cruciferous vegetables, oilseeds, and forage crops using high performance liquid chromatography. Fette, Seifen, Anstrichmittel 1984, 86, 228–231.
- Bertrand, M.; Heinrich, L. Glucosinolates in members of the family brassicaceae: separation and identification by LC/ESI-MS-MS. Journal of Agricultural and Food Chemistry 2000, 48, 2234–2239.
- Blanco, M.; Villaroya, I. NIR spectroscopy: A rapid-response analytical tool. Trends in Analytical Chemistry 2002, 21, 240–250.
- McClure, F.W. 204 years of near infrared technology: 1800–2003. Journal of Near Infrared Spectroscopy 2004, 11, 487–518.
- Roggo, Y.; Chalus, P.; Maurer, L.; Lema-Martinez, C.; Edmond, A.; Jent, N. A review of near infrared spectroscopy and chemometrics in pharmaceutical technologies. Journal of Pharmaceutical and Biomedical Analysis 2007, 44, 683–700.
- Shao, Y.N.; He, Y.; Bao, Y.D.; Mao, J.Y. Near-infrared spectroscopy for classification of oranges and prediction of the sugar content. International Journal of Food Properties 2009, 12, 644–658.
- Liu, F.; Yusuf, B.L.; Zhong, J.L.; Feng, L.; He, Y.; Wang, L. Variety identification of rice vinegars using visible and near infrared spectroscopy and multivariate calibrations. International Journal of Food Properties 2012, 14, 1264–1276.
- Cozzolino, D. Near infrared spectroscopy in natural products analysis. Planta Medica 2009, 75, 746–756.
- Biston, R.; Dardenne, P.; Cwikowski, M.; Marlier, M.; Severin, M.; Wathelet, J.P. Fast analysis of rapeseed glucosinolates by near infrared reflectance spectroscopy. Journal of the American Oil Chemists’ Society 1988, 65, 1599–1600.
- Daun, J.K.; Clear, K.M.; Wiliams, P. Comparison of three whole seed near-infrared analyzers for measuring quality components of canola seed. Journal of the American Oil Chemists’ Society 1994, 71, 1063–1608.
- Velasco, L.; Becker, H.C. Analysis of total glucosinolate content and individual glucosinolates in Brassica spp. by near-infrared reflectance spectroscopy. Plant Breeding 1998, 117, 97–102.
- Font, R.; Del Rio-Celestino, M.; Fernandez-Martinez, J.M.; De Haro-Bailon, A. Use of near infrared spectroscopy for screening the individual and total glucosinolate contents in Indian mustard seed (Brassica juncea L. Czern & Coss). Journal of Agricultural and Food Chemistry 2004, 52, 3563–3569.
- Font, R.; Del Rio-Celestino, M.; Cartea, E.; De Haro-Bailon, A. Quantification of glucosinolates in leaves of leaf rape (Brassica napus ssp. pabularia) by near infrared spectroscopy. Phytochemistry 2005, 66, 175–185.
- Hom, N.; Backer, H.; Mollers, C. Non-destructive analysis of rapeseed quality by NIRS of small seed samples and single seeds. Euphytica 2007, 153, 27–34.
- Montes, J.M.; Paul, C. Melchinger, A.E. Quality assessment of rapeseed accessions by means of near-infrared spectroscopy on combine harvesters. Plant Breeding 2007, 126, 329–330.
- Min, S.D.; Li, N.; Zhang, M.X. Outlier diagnosis and calibration model optimization for near infrared spectroscopy analysis. Spectroscopy and Spectral Analysis 2004, 24, 1205–1209.
- Wathelet, J.P.; Biston, R.; Marlier, M.; Severin, M. Qualitative and quantitative analysis of the glucosinolates contained in rapeseed: Comparison of different analytical methods. In: 7th International Rapeseed Congress; Poland, 1988; 1342–1348.
- Moller, P.; Olsen, O.; Ploger, A.; Rasmussen, K.W.; Sorensen, H. Quantitative analysis of individual glucosinolates in double low oilseed rape by HPLC of intact glucosinolates. World Crops: Production, Utilization and Description 1985, 11, 111–126.
- He, H.J. Studies for growth adaptation and identification of glucosinolates on Chinese B.rassicas. Dissertation, Lehrstuhl fuer Gemuesebau, Technische Universitaet Muenchen, 1999; 56–69.
- Magrath, R.; Herron, C.; Giamoustaris, A.; Mithen, R. The inheritance of aliphatic glucosinolates in Brassica napus. Plant Breeding 1999, 111, 55–72.
- Lu, W.Z.; Yuan, H.F.; Xu, G.T. The Modern Analysis Technique for Near-Infrared Spectra, Chinese Oil and Chemical Press: Beijing, China, 2001.
- Liu, X.; Han, L.J. Feasibility of using near-infrared reflectance spectroscopy for the analysis of compositions of straw silage. Spectroscopy and Spectral Analysis 2006, 20, 2016–2020.