Abstract
The objective of this work was to study the contribution of different peanut varieties with regard to their arachin and conarachin fractions, and their respective subunits to protein gel properties. It was found that the proteins of various cultivars could be classified into two groups according to the lack of the 35.5 kDa acidic subunit of the arachin. Two peanut cultivars, Yueyou7 and Qinglan8, had the highest content of arachin (62.3%) and conarachin (53.5%), respectively. Three indexes, namely, hardness, springiness, and cohesiveness, were used to describe the protein gel properties. An efficient approach was developed to characterize the three gel properties into one index. The correlation coefficients between the newly depicted index and the original ones were 0.87, 0.41, and 0.47, which corresponded to hardness, springiness, and cohesiveness, respectively. Principal component analysis, linear discriminant analysis, and cluster analysis were used as classification techniques. About 40 varieties were classified into three groups by principal component analysis and all group members determined by principal component analysis are in the predicted group that 100.0% of original grouped cases correctly classified by linear discriminant analysis. The result of cluster analysis had some similarities with the principal component analysis.
INTRODUCTION
The peanut (Arachis hypogaea L.) is an important crop grown worldwide. The total yield in China has been the highest in the world since 1993.[Citation1] The yield of peanuts in China was 1.30 million tons in 2007, 1.43 million tons in 2008, and 1.47 million tons in 2009.[Citation2] Peanut seeds have a protein content of 24 to 36% on a dry weight basis. Peanut protein does not contain significant amounts of any intrinsic anti-nutritional factor among the vegetable proteins, which makes it a relatively inexpensive source of protein for human consumption.[Citation3] In China, more than 50% of the peanut yield is used to produce edible oil.[Citation4] After removing the oil, the remaining peanut meal is a protein-rich, inexpensive, and underutilized by-product in the peanut industry that contains 50 to 55% high-quality protein. Hence, a stable supply and high quality of domestic peanut protein for food processing are important.
Peanut proteins play an important role in many food products because of their nutritional value, contribution to food texture, and solubility, among others. For example, proteins with high oil and water binding are desirable for use in meats, sausages, breads, and cakes. Proteins with high emulsifying and foaming capacity are good for salad dressing, sausages, bologna, soups, confectionery, frozen desserts, and cakes.[Citation5] Researchers[Citation6–Citation8] have determined the functional properties of several plant proteins concentrates using alkali solutions with isoelectric precipitation produced from peas and beans. The functional properties of peanut proteins are the subjects of limited studies that focused mainly on peanut flour.[Citation9,Citation10] Little information is available on the functional characteristics of peanut protein isolate.
Peanut proteins are composed of arachin, conarachin I, and conarachin II fractions.[Citation11] Krishna et al.[Citation12] reported that the subunits of conarachin and basic subunits of arachin, did not show any variation. But the arachin acidic subunits, showed variation. There are four varietal classes of arachin polypeptide patterns: Class A comprised three acidic subunits of arachin of molecular weights 47.5, 45.1, and 42.6 kDa and a basic subunit of 21.4 kDa; class B, with three acidic subunits of molecular weights 47.5, 45.1, and 41.2 kDa and a basic subunit of 21.4 kDa; class C of an additive pattern of class A and class B; class D, of two acidic polypeptides of 47.5 kDa, 45.1 kDa and the basic 21.4 kDa subunit. These fractions were also shown to differ in amino acid composition.[Citation13–Citation17] Thus, these protein fractions may differ not only in nutritional value but in functional properties. Several studies have reported that the 7S and 11S proteins of soybeans are important contributors gel properties. Nakamura et al.,[Citation18] Riblett et al.,[Citation19] and Khatib et al.[Citation20] reported a positive correlation between 11S and hardness. Mujoo et al.[Citation21] found a positive correlation between 11S/7S ratio and tofu firmness. Arrese et al.[Citation22] reported that the relationship between the gelling ability of SPI and 11S/7S, the subunits of 11S and 7S was very tight. Shimada and Matsushita[Citation23] found a positive correlation between adhesiveness of the gels and content of 7S. There are also some published results reveal that soybean storage proteins, whose contents vary among different varieties.[Citation21] Hence, the compositions of protein are important to its gel properties.
In this work, the authors were interested in the potential quantitative differences between arachin and conarachin concentration that might be observed in peanut varieties. The focus was on mainly five aspects. First, it was determined the composition of the major peanut proteins (arachin and conarachin) and their contents in 40 peanut varieties. Second, the contents of one subunit were compared with another or one sample with another. Third, the three indexes of gel properties were characterized into one. Fourth, the relationship between peanut protein compositions and gel properties was investigated. Finally, the result of PCA and CA was compared.
MATERIALS AND METHODS
Plant Materials
Forty varieties of peanuts were used in the study. Two varieties were obtained from Hubei province (Zhonghua8 and Zhongnong108), 11 were from Shandong province (Shanhua7, Luhua11, Shanhua9, Fenghua1, Fenghua3, Fenghua4, Fenghua5, Honghua1, Huayu8, Luhua14, and 9616), one was from Jilin province (Silihong), six were from Henan province (Bianhua3, Haihua1, Shuangji2, Baihuasheng, Huaguanwang, and Qinglan8), 11 were from Guangdong province (Yueyou7, Yueyou14, Yueyou20, Yueyou39, Yueyou40, Yueyou45, Yueyou52, Yueyou55, Yueyou86, Zhenzhuhong, and Zhenzhuhei), four were from Fujian province (Minhua9, Zhanhua82, Shanyuo250, and Longhua243), and five were from Guangxi province (Heyou11, Guihua771, Quanhua551, Zhongkaihua9, and Puhua23). The cultivars were obtained from China peanut growers in 2009. These peanuts were stored in sealed bags at 4°C. All chemicals and reagents were acquired from Sigma chemical company and were of analytical grade.
Extraction of Peanut Protein Isolates (PPI)
The peanuts were ground in an altra-mizer (ZhenYuan Powder Engineering Equipment Co., Ltd., Shanghai, China) to coarse powders. Then, the coarse powders were further ground in an Udy cyclone sample mill with a 0.5 mm screen (GS-86 automatic sieving machine, Yarn sieve, Co., Shanghai, China). The powder was extracted with hexane five or six times. The ratio of powder to solvent was 1:1 (w/v) each time, such that the final fat content was less than 1%. The solvent was evaporated at room temperature, and the dried-defatted peanut meal was stored at 4°C.
Defatted peanut flour was dispersed in deionized water (w/v, 1:10). The pH of the dispersion was adjusted to 8.5 with 2 mol/L NaOH. The resultant dispersion was gently stirred at 25°C for 2 h, centrifuged at 3500 g for 20 min in a KDC-160HR high speed centrifuge (ZONKZA Co., China). The supernatant was adjusted to pH 4.5 with 2 mol/L HCl and then centrifuged at 3500 g and 25°C for 20 min. The obtained precipitate was re-dispersed in deionized water. The dispersion was homogenized, adjusted to pH 7.0 with 2 mol/L NaOH and freeze-dried using an LGJ-25 freeze dryer (Sihuan, Science Instrument Factory, Beijing, China). These conditions minimized the denaturation of proteins, hence, the isolates were considered to contain “native” forms of the proteins.[Citation24–Citation26]
Sodium Dodecyl Sulfate-Polyacrylamide Gel Electrophoresis (SDS-PAGE)
Ten milligrams of PPI was dispersed in 2 ml of 0.01 mol/L sodium phosphate buffer (pH 7.2) for 15 min with vortexing every 5 min. The extract was centrifuged for 10 min at 10,000 g. Then, 10 μl of extract was mixed with 10 μl of Tris buffer (pH 6.8), which contained 2% SDS, 10% glycerol, 5% β-mercaptoethanol, and 0.002% bromophenol blue. The mixture was heated at 100°C for 5 min, cooled to room temperature (25°C), and used for SDS-PAGE. SDS-PAGE was performed following the method of Laemmli,[Citation27] with some modifications to accommodate peanut protein. Protein samples (2.5 mg/ml) were prepared using SDS-PAGE[Citation28] in a vertical electrophoresis unit (Hoefer Scientific Instru-ments, San Francisco, CA) at 80 V constant voltage for 1 h, followed by 110 V constant voltage until the tracking dye migrated to the bottom edge of the gel (3 h). The gel slab was removed after the completion of electrophoresis. The gels were stained with Coomassie Brilliant Blue R-250 (w/v, 0.05%) in methanol-acetic acid-water (v/v/v, 25:10:65), and destained in the same solution without the dye. Phosphorylase-b (MW 97.2 kDa), bovine serum albumin (MW 66.2 kDa), Actin (MW 43.0 kDa), Carbonic Anhydrase (MW 31.0 kDa), Trypsin Inhibitor (MW 20.1 kDa), and Lysozyme (MW 14.4 kDa) were used as standards to estimate the molecular weight ranges of polypeptides in each fraction, and to identify the subunits of the major peanut proteins.
Quantification of Protein Fractions from Total Protein Extracts by Densitometry
To quantify the arachin and conarachin fractions and their respective subunits, the gels were rinsed after destaining and the protein bands were analyzed using a densitometric approach. The electrophoretic patterns were analyzed using a transmittance/absorptance scanning densitometer (Alpha Innotech Chem, USA) with Alpha software. The relative protein quantity of each subunit (protein band) was calculated from their respective percent area on the densitograms against the total percent area.
Preparation of Peanut Protein Gels
The peanut protein products were dispersed in 0.01 mol/L sodium phosphate buffer, pH 7.0 at a protein/buffer ratio of 15/100 (w/v). The dispersions were stirred at room temperature for 120 min. Twenty milliliters of each suspension were put into a beaker, sealed with a film, heated at 90°C for 60 min, and then immediately cooled in a bath of cold water. The heated samples were stored at 4°C for 18 h.
Instrumental Texture Profile Analysis (TPA) of the Gels
To evaluate the texture of peanut protein gels, a uniaxial compression test was performed by TA-TX2i Texture Analyzer (Stable Micro Systems Ltd., Godalming, England). The method of Francesca et al.[Citation29] was adapted to suit the requirements of this experiment. A spherical plunger (diameter = 12 mm) was utilized. Samples were compressed to 50% at a rate of 0.1 mm/s.[Citation10] The trigger point was 0.01 N. The program produced a force-displacement curve. The TPA parameters, namely, hardness (peak force on the first compression, N), springiness (related to the height that the gel recovers during the time that elapses between the end of the first compress and the start of the second compress), and cohesiveness (ratio of the active work done under the second force-displacement curve to that done under the first compression curve) were computed.[Citation30,Citation31]
Statistical Analysis
Data were expressed as mean standard deviation (SD) of three replicated determinations. Pearson’s correlation coefficients were used to determine the degree and significance of association among the various attributes.[Citation32] In order to reduce the dimensionality of the data set and to describe all the variability of the system using a smaller number of variables, principal component analysis (PCA) was used. The first principal component (PC) describes the maximum possible variation and the second PC accounts for the most and so on. Unsupervised classification was performed with cluster analysis (CA) to measure the similarity between objects. The statistical analysis of the data was performed using the SPSS (Statistical Product and Service Solutions) 17.0 package for windows.
In the following section, an approach to integrate the three features into one was provided. The three features of the ith subject was denoted by
(here
). Then there is
where
Based on , two matrices were then constructed,
and
, where
RESULTS AND DISCUSSION
SDS-PAGE Profile of Total Peanut Proteins
PAGE is a highly sensitive technique that makes possible the identification of micrograms of protein. It produces better separation of protein mixtures than other available techniques.[Citation34] These characteristics made this technique desirable for quantization and identification of proteins. Electrophoretic patterns of total peanut proteins from 40 different peanut varieties on SDS-polyacrylamide gradient gel are shown in and . Variations can be observed in the peanut proteins. In , four regions, designated as 1, 2, 3, and 4 were observed after SDS-PAGE. Arachin had polypeptides of approximately 20 kDa to 45 kDa. Conarachin II showed a major polypeptide at 61 kDa, whereas the protein of conarachin I consisted mainly of small molecular weight polypeptides. These results were similar to that obtained by Yamada et al.[Citation35] and Monteriro and Prakash.[Citation36,Citation37]
Figure 1 SDS-PAGE patterns of seed protein from peanut varieties. The different tracks showed M: Marker. (1) Zhonghua8; (2) Zhongnong108; (3) Shanhua7; (4) Silihong; (5) Luhua11; (6) Bianhua3; (7) Haihua1; (8) Shanhua9; (9) Shuangji2; (10) Fenghua5.
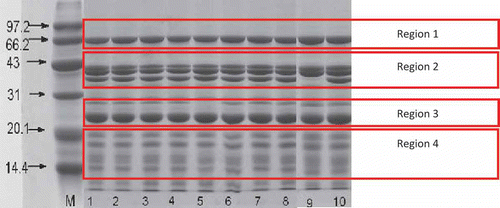
Figure 2 SDS-PAGE patterns of seed protein from peanut varieties. The different tracks showed (11) Yueyou7; (12) Yueyou14; (13) Yueyou20; (14) Yueyou39; (15) Yueyou40; (16) Yueyou45; (17) Yueyou52; (18) Yueyou55; (19) Yueyou86; (20) Minhua9; (21) Guihua771; (22) Zhanhua82; (23) Shanyou250; (24) Longhua243; (25) Heyou11; (26) Quanhua551; (27) Zhongkaihua9; (28) Puhua23; (29) Zhenzhuhong; (30) Zhenzhuhei; (31) Baihuasheng; (32) Huaguanwang; (33) Fenghua1; (34) Fenghua3; (35) Fenghua4; (36) Honghua1; (37) Qinglan8; (38) Huayu8; (39) Luhua14; (40) 9616.
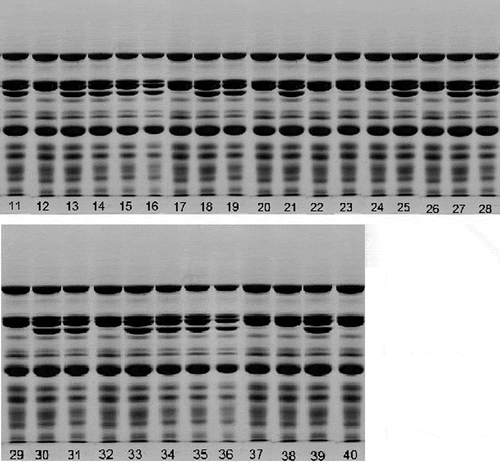
Arachin and conarachin are the major storage proteins in peanuts.[Citation3] As seen from and , different peanut varieties had different storage protein compositions. SDS-PAGE separated arachin (40.5, 37.5, 35.5, and 23.5 kDa subunits) and conarachin (61, 18, 17, and 15.5 kDa subunits) in the peanuts. No major qualitative variation in the conarachin polypeptide (regions 1 and 4 in ) and the arachin basic subunit (region 3 in ) were observed among the genotypes studied. However, the acidic subunits (region 2 in ) of the arachin in the peanut varieties had significant differences. In 13 varieties, including Shuangji2, Yueyou14, Minhua9, Zhanhua82, Shanyou250, Longhua243, and so on (, lane 9; , lanes 12, 17, 20, 22, 23, 24, 26, 29, 32, 37, 38, and 40), the third acidic polypeptide of arachin protein were absent. Even overloading of the sample did not reveal any trace of the third acidic polypeptide of arachin. The number of polypeptides observed was not due to proteolysis because the electrophoresis pattern was identical when the protein was extracted both in the presence and absence of proteolysis inhibitors, such as phenylmethanesulfonyl fluoride (PMSF) (unpublished data).
Quantification of Arachin and Conarachin, and Their Subunits by Densitometry
Table 1 Mean, range, and coefficient of variation for major protein fractions in peanut of 40 varieties
Table 2 Correlation coefficient between arachin, conarachin, and their subunits
PCA
PCA is a bilinear modeling method which gives an interpretable overview of the main information in a multi-dimensional data table. The information carried by the original variables is projected onto a smaller number of underlying (“latent”) variables called PCs. The first PC covers as much of the variation in the data as possible. The second PC is orthogonal to the first and covers as much of the remaining variation as possible, and so on. By plotting the PCs, one can view inter-relationships between different variables, and detect and interpret sample patterns, groupings, similarities or differences.[Citation38] gives the loadings and communality for each subunit and storage protein. PCA was applied to the whole set of data. The first three factors explained 82.29% of the total variability. The contents of arachin, conarachin, and arachin/conarachin had the highest weight on the first PC (explaining 34.44% of the variability). Conarachin I, 23.5 kDa and 15.5 kDa dominated the second PC (explaning 42.22% of variability). 37.5 kDa and 17 kDa contents showed the highest weight on the third PC (explaining 35.69% of variability). The higher the loading of a variable implies a larger contribution to the variation, accounting for the PCs. also gave the score values for each PC for each sample. From the scores on the first PC it can be interpreted that the contents of arachin, conarachin, and archin/conarachin on the first PC loadings are higher for Qinghua8, Shuangji2, Quanhua551, 9616, Huayu8, Huaguanwang, Yueyou14, Longhua243, Zhenzhuhong, and Shanyou250 than the other varieties and are lower for Baihuasheng, Yueyou55, Yueyou20, Yueyou7, and Puhua23 than the other varieties. When the second PC is interpreted, conarachin I, 23.5 kDa and 15.5 kDa contents are higher for Yueyou39, Yueyou52, Huaguanwang, Minhua9, and Shanyou250 and are lower for Luhua11, Bianhua3, Fenghua5, Zhongnong108, Shanhua7, and Quanhua551 than for the other varieties investigated. On the third PC, 37.5 kDa and 17 kDa contents are higher for Zhanhua82, Longhua243, Yueyou40, Shanyou250, Yueyou52, Quanhua551, and Yueyou20 are lower for Yueyou39, Luhua14, Huaguanwang, 9616, Fenghua3, Qinghua8, Honghua1 and than for the other varieties.
Table 3 The loadings and the scores of the first three principal component
shows the two way loadings and score plots. Every PC was plotted against PC1 to show high percentage of the total variance (71.80 to 51.34%). showed the behavior of variables on the PC1 and PC2. As can be seen, there is an association between arachin, arachin/conarachin, and 35.5 kDa. The superposition of the score () and loading () plots for PC1 and PC2 show arachin contents are higher for Yueyou7, Yueyou20, and Yueyou55. 15.5 kDa contents are higher for Shanhua7, Luhua11, Bianhua3, and Fenghua5. It can be interpreted from and from PC1 and PC3 that the conarachin II content is the highest for Huaguanwang, 17 kDa content is highest for Zhanhua82.
Figure 3 The score and loadings (a and c are the loading plots and b and d are the score plots) (1) Zhonghua8; (2) Zhongnong108; (3) Shanhua7; (4) Silihong; (5) 0uhua11; (6) Bianhua3; (7) Haihua1; (8) Shuangji2; (9) Shanhua9; (10) Fenghua5; (11) Yueyou7; (12) Yueyou14; (13) Yueyou20; (14) Yueyou39; (15) Yueyou40; (16) Yueyou45; (17) Yueyou52; (18) Yueyou55; (19) Yueyou86; (20) Minhua9; (21) Guihua771; (22) Zhanhua82; (23) Shanyou250; (24) Longhua243; (25) Heyou11; (26) Quanhua551; (27) Zhongkaihua9; (28) Puhua23; (29) Zhenzhuhong; (30) Zhenzhuhei; (31) Baihuasheng; (32) Huaguanwang; (33) Fenghua1; (34) Fenghua3; (35) Fenghua4; (36) Honghua1; (37) Qinghua8; (38) Huayu8; (39) Luhua14; (40) 9616.
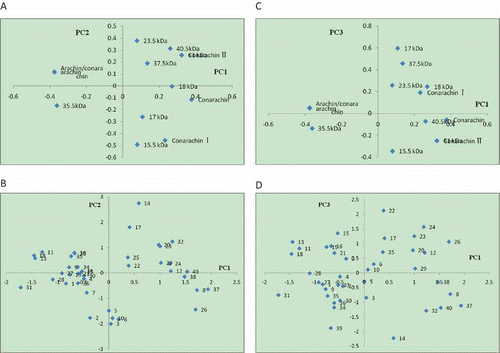
The classification of the peanut varieties from the view point of storage proteins contents can be made using three way PC score graph. showed PC 1-2-3. The PC 1-2-3 graph showed the total variance of about 82.29%. It can be classified into three groups. These groups include:
Figure 4 Three way PCA scores plot PC1-2-3. (1) Zhonghua8; (2) Zhongnong108; (3) Shanhua7; (4) Silihong; (5) Luhua11; (6) Bianhua3; (7) Haihua1; (8) Shuangji2; (9) Shanhua9; (10) Fenghua5; (11) Yueyou7; (12) Yueyou14; (13) Yueyou20; (14) Yueyou39; (15) Yueyou40; (16) Yueyou45; (17) Yueyou52; (18) Yueyou55; (19) Yueyou86; (20) Minhua9; (21) Guihua771; (22) Zhanhua82; (23) Shanyou250; (24) Longhua243; (25) Heyou11; (26) Quanhua551; (27) Zhongkaihua9; (28) Puhua23; (29) Zhenzhuhong; (30) Zhenzhuhei; (31) Baihuasheng; (32) Huaguanwang; (33) Fenghua1; (34) Fenghua3; (35) Fenghua4; (36) Honghua1; (37) Qinghua8; (38) Huayu8; (39) Luhua14; (40) 9616.
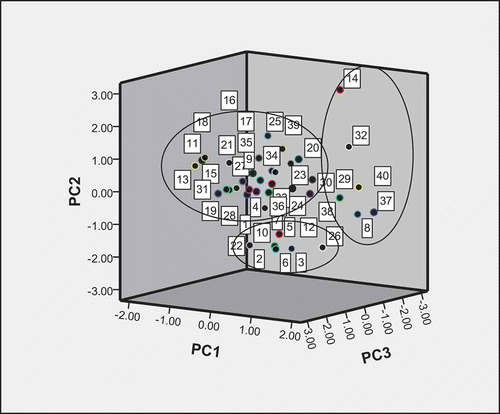
Group 1: Yueyou7, Yueyou20, Yueyou55, Zhonghua8, Silihong, Haihua1, Shanhua9, Yueyou14, Yueyou40, Yueyou45, Yueyou52, Yueyou86, Minhua9, Guihua771, Zhanhua82, Shanyou250, Longhua243, Heyou11, Zhongkaihua9, Puhua23, Zhenzhuhong, Zhenzhuhei, Baihuasheng, Fenghua1, Fenghua3, Fenghua4, Honghua1, and Luhua14.
Group 2: Zhongnong108, Shanhua7, Luhua11, Bianhua3, Fenghua5, and Quanhua551.
Group 3: Shuangji2, Yueyou39, Huaguanwang, Qinghua8, Huayu8, and 9616.
Linear Discriminant Analysis (LDA)
LDA technique is a supervised pattern recognition method. In supervised pattern recognition, objects are classified into groups (or classes or clusters) with pre-determined models for the class. These approaches differ from unsupervised methods, such as CA, where there is no prior class model. The aim of hard-modeling, a form of supervised pattern recognition, is to classify uniquely into a number of pre-determined classes.[Citation39] LDA was performed on the classified three groups resulting from the PCA analyses above for the 13 elements using SPSS 17.0 statistics software. The recognition of these groups was highly satisfactory. All group members determined by PCA are in the predicted group that 100.0% of original grouped cases correctly classified. Two canonical discriminant function were obtained from the data that eigen values are bigger than one. The first canonical discriminant function explains 98.8% of the variance. The discriminant function of the first function is: Z = –2.014 –0.156*arachin + 0.178*conarachin –0.033*conararachinI + 0.259*conarachinII –0.166*arachin/conarachin –0.313*40.5kDa –1.647*37.5 kDa + 0.760* 35.5kDa –1.298* 23.5kDa –0.820* 18kDa –1.438* 17kDa + 1.641* 15.5 kDa
Relationship Between Storage Protein Contents and Gel Property
TPA of peanut protein gel properties
The protein gel properties consisted of three-dimensional matrices or intertwined network, partially associated with polypeptides, in which deionized water was entrapped. The gel property was characterized by a relatively high hardness, springiness, and cohesiveness. The abilities of protein to form gels and to provide a structure for holding water, flavors, sugars, and food ingredients are useful in food applications, and in new-product development that provide an added dimension of protein functionality.
shows that the hardness, springiness, and cohesiveness of the gel made from the PPI varied greatly. The CV of the three indexes reached 40.12, 16.54, and 17.15%, respectively, which showed that hardness plays an important role in evaluating the gel properties among these three indexes. From the TPA results, the first three hardness, springiness, and cohesiveness varieties as followed: Bianhua3 (3.50), Quanhua551 (2.92), and Luhua11 (2.73); Huaguanwang (0.97), Shanhua7 (0.96), and Zhongnong108 (0.89); Yueyou52 (0.60), Yueyou40 (0.59), and Huayu8 (0.51), respectively. However, there was no similar trend for the three indexes of gel properties among the peanut varieties. The gel property of protein by the three indexes could not be evaluated. Therefore, integrating these three features into one was necessary. Using the method given in Section 2.8, the three features were integrated into one feature. The relationship between the new feature and the original three features is:
Table 4 Total variance explained
Table 5 Mean, range, and coefficient of variation for gel properties in peanut of 40 varieties
The correlation coefficients between the newly depicted index and the original ones were 0.87, 0.41, and 0.47, which corresponded to hardness, springiness, and cohesiveness, respectively. So defined this value as gel property was reasonable, and in the next, this value was used to reflect the relationship between gel property and protein content. Using the new index, the variety that had the best gel property (high value) could be evaluated. For example, the first three varieties of gel property were Bianhua3 (1.32), Shanhua7 (1.31), and Yueyou52 (1.28).
Relationship Between Storage Protein Contents and Gel Property
presents the correlation coefficient of the storage protein contents and gel property. The arachin content (r = –0.47) and arachin/conarachin ratio (r = –0.51) were negatively correlated with gel property. However, conarchin I contetnt (r = 0.49), conarchin II content (r = 0.59) and conarchin content (r = 0.51) were positively correlated with gel property. There were some relationships between peanut protein gel property and arachin, conarachin, conarachin I, conarachin II, and the ratio of arachin/conarachin. This result was in general agreement with earlier findings about soybeans, which showed the relationship of quantity composition and gel property, and the positive correlation of soybean glycine with gel properties.[Citation21,Citation40,Citation41] Hence, the result of this article would have further research implications about why they had such relations.
Table 6 Correlation coefficients of gel property and protein components
CA
CA is the most widely used unsupervised pattern recognition technique in chemometrics. This technique involves trying to determine relationships between objects (samples) without using prior information about these relationships. The raw data for CA consist of a number of objects and related measurement.[Citation39] Objects will be grouped in clusters in terms of their nearness or similarity. The CA was applied using the SAS package. CA was performed using the gel property. The measurement is based on the squared Euclidean distance. In this study, the Ward’s method was used as a clustering method. Similar results to PCA were obtained after the application of CA (). Five groupings were obtained from CA. These groups contain:
Figure 5 Dendogram of cluster analysis. (1) Zhonghua8; (2) Zhongnong108; (3) Shanhua7; (4) Silihong; (5) Luhua11; (6) Bianhua3; (7) Haihua1; (8) Shuangji2; (9) Shanhua9; (10) Fenghua5; (11) Yueyou7; (12) Yueyou14; (13) Yueyou20; (14) Yueyou39; (15) Yueyou40; (16) Yueyou45; (17) Yueyou52; (18) Yueyou55; (19) Yueyou86; (20) Minhua9; (21) Guihua771; (22) Zhanhua82; (23) Shanyou250; (24) Longhua243; (25) Heyou11; (26) Quanhua551; (27) Zhongkaihua9; (28) Puhua23; (29) Zhenzhuhong; (30) Zhenzhuhei; (31) Baihuasheng; (32) Huaguanwang; (33) Fenghua1; (34) Fenghua3; (35) Fenghua4; (36) Honghua1; (37) Qinghua8; (38) Huayu8; (39) Luhua14; (40) 9616.
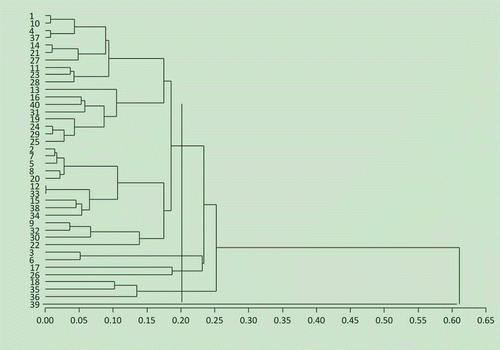
Group 1: Zhonghua8, Fenghua5, Silihong, Qinghua8, Yueyou39, Guihua771, Zhongkaihua9, Yueyou7, Shanyou250, Puhua23, Yueyou20, Yueyou45, 9616, Baihuasheng, Yueyou86, Longhua243, Zhenzhuhong, Heyou11, Zhongnong108, Haihua1, Luhua11, Shuangji2, Minhua9, Yueyou14, Fenghua1, Yueyou40, Huayu8, Fenghua3, Huaguanwang, Zhanhua82
Group 2: Shanhua7, Bianhua3
Group 3: Yueyou52, Quanhua551
Group 4: Yueyou55, Fenghua4, Honghua1
Group 5: Luhua14
CONCLUSIONS
Previous studies focused on amino acid composition of total protein and its fractions, and the relationship between amino acid and protein texture. Different preparation methods affect gel properties. The current research studied the content of arachin and conarachin proteins of peanut varieties and their gel properties. In addition, a new method was built to express gel property. It was found that the acidic subunits of arachin protein in the peanut varieties had significant differences. From the 13 varieties, namely, Shuangji2, Yueyou14, Minhua9, Zhanyou82, Shanyou250, Longhua243, and so on, the third acidic polypeptides of arachin proteins were absent. The different varieties had different arachin and conarachin contents. Yueyou7 had the highest arachin content (62.3%), whereas Qinglan8 had the highest conarachin content (53.5%). The CV of arachin/conarachin ratio reached 15.65%. The study developed an efficient approach to characterize the three gel property indexes into one index, which had correlation coefficients 0.87, 0.41, and 0.47, corresponding to hardness, springiness, and cohesiveness, respectively. The conarachin content (r = 0.51), conarachin I content (r = 0.49), and conarachin II content (r = 0.59) were positively correlated with gel property. The arachin content (r = –0.47) and arachin/conarachin ratio (r = –0.51) were negatively correlated with gel property. PCA, LDA, and CA were used as classification techniques. About 40 varieties were classified into three groups by PCA and all group members determined by PCA are in the predicted group that 100.0% of original grouped cases correctly classified by LDA. CA found some similar results with PCA, but there are a lot of different groupings. At this stage of the study, it is clear that the different varieties may be grouped according to their storage protein contents, but there is insufficient evidence to attribute this to plant physiology or different “external” parameters such as total protein content or amino acids content, and so on. It was also interpreted the low correlation between storage protein content and gel property, but did not show in this article. This will be reported in the later studies.
ACKNOWLEDGMENTS
The authors also wish to thank Ms. Chunhong Li (CAAS, Beijing) for allowing access to her TA-TX2i Texture Analyzer facilities and for her skillful technical assistance with the TA-TX2i Texture Analyzer.
FUNDING
This research was funded by the “Special Fund for Agro-Scientific Research in the Public Interest” (Serial No. 200903043) and the “Special Fund for Basic Scientific Research and Operational Costs of Central-level Public Welfare Scientific Research Institutes of CP in CAAS.” The authors gratefully acknowledge the financial support.
REFERENCES
- FAOSTAT. http://faostat.fao.org/site/567/DesktopDefault.aspx?PageID=567#ancor2009.
- China statistical yearbook. http://www.stats.gov.cn/tjsj/ndsj/2009/indexch.htm2010.
- Prakash, V.; Rao, M.S. Physicochemical properties of oilseed proteins. CRC Critical Reviews in Biochemistry 1986, 20 (3), 286–301.
- Wan, S.B. Peanut quality [M]. China’s Agricultural Science and Technology Press. Beijing: (in Chinese) 2008, pp. 14–58.
- Ahmedna, M.; Prinyawiwatful, W.; Rao, R.M. Solubilized wheat protein isolate: Functional properties and potential food applications. Journal of Agricultural and Food Chemistry 1999, 47 (4), 1340–1345.
- Fuhrmeister, H.; Meuser, F. Impact of processing on functional properties of protein products from wrinkled peas. Journal of Food Engineering 2003, 56, 119–129.
- Yumiko, Y.; Yoshiko, W.; Michael, S.; Andreas, W. Functional and bioactive properties of rapeseed protein concentrate and sensory analysis of food application with rapeseed protein concentrates. LWT-food Science and Technology 2006, 39 (5), 503–512.
- Chandi, G.K.; Sogi, D.S. Functional properties of rice bean protein concentrates. Journal of Food Engineering 2007, 79 (2), 592–597.
- Prinyawiwatkul, W.; Beuchat, L.R.; McWatters, K.H. Functional property changes in partially defatted peanut flour caused by fungal fermentation and heat treatment. Journal of Food Science 1993, 58, 1318–1323.
- Wu, H.W.; Wang, Q.; Ma, T.Z.; Ben, J.J. Comparative studies on the functional properties of various protein concentrate preparations of peanut protein. Food Research International 2009, 42, 343–348.
- Yamada, T.; Aibara, S.; Morita, Y. Dissociation association behavior of arachin between dimeric and monomeric forms. Agricultural and Biological Chemistry 1979, 43 (12), 2549–2556.
- Krishna, T.G.; Pawar, S.E.; Mitra, R. Variation and inheritance of the arachin polypeptides of groundnut (Arachis hypogaea L.). Theoretical and Applied Genetics 1986, 73, 82–87.
- Dawson, R. The nutritive value of groundnut protein. British Journal of Nutrition 1968, 22, 601–607.
- Dawson, R. Comparison of fraction of groundnut proteins by two different methods. Analytical biochemistry 1971, 305–313.
- Neucere, N.J. Isolation of α-arachin, the major peanut globulin. Analytical Biochemistry 1969, 27, 15–24.
- Singh, J.; Dieckert, J.W. Types and molecular weights of subunits of arachin P6. Preparative Biochemistry 1973, 3, 73–82.
- Basha, S.M.M.; Cherry, J.P. Composition, solubility, and gel electrophoretic properties of proteins isolated from Florunner peanut seeds. Journal of Agricultural and Food Chemistry 1976, 24, 359–365.
- Nakamura, T.; Utsumi, S.; Kitamura K. Cultivar differences in gelling characteristics of soybean glycinin. Journal of Agricultural and Food Chemistry 1984, 32, 647–651.
- Riblett, A.L.; Thomas, J.; Schmidt, K.A. Characterization of β-conglycinin and glycinin soy protein fractions from four selected soybean genotypes. Journal of Agricultural and Food Chemistry 2001, 49, 4983–4989.
- Khatib, K.A.; Herald, T.J.; Aramouni, F.M.; Mac Ritchle, F.; Schapaugh, W.T. Characterization and functional properties of soy β-conglycinin and glycinin of selected genotypes. Journal of Food Science 2002, 67, 2923–2929.
- Mujoo, R.; Dianne, T.T.; Perry, K.W.N. Characterization of storage proteins in different soybean varieties and their relationship to tofu yield and texture. Food Chemistry 2003, 82, 265–273.
- Arrese, E.L.; Sorgentini, D.A.; Wagner, J.R. Electrophoretic, solubility, and functional properties of commercial soy protein isolates. Journal of Agricultural and Food Chemistry 1991, 39, 1029–1032.
- Shimada, K.; Matsushita, S. Gel formation of soybean 7S and 11S proteins. Agricultural and biological chemistry 1980, 44 (30), 637–641.
- Cao, X.H.; Li, C.J.; Wen, H.B.; Gu, Z.X. Extraction technique and characteristics of soluble protein in germinated brown rice. International Journal of Food Properties 2010, 13, 810–820.
- Conforti, P.A.; Lupano, C.E. Selected properties of araucaria angustifolia and araucaria araucana seed protein. International Journal of Food Properties 2011, 14, 84–91.
- Adebowale, Y.A.; Schwarzenbolz, U.; Henle, T. Protein isolates from bambara groundnut (voandzeia subterranean L.): Chemical characterization and functional properties. International Journal of Food Properties 2011, 14, 758–775.
- Laemmli, U.K. Cleavage of structural proteins during the assembly of the head of bacteriophage T4. Nature 1970, 227, 680–686.
- Cai, T.; Cheng, K. Processing effect on soybean storage proteins and their relationship with tofu quality. Journal of Agricultural and Food Chemistry 1999, 47, 184–194.
- O’Kane, F.E.; Happe, R.P.; Vareijken, J.M.; Gruppen, H.; Van Bockel, M.A.J.S. Characterization of pea vicillin. 2. Consequences of compositional heterogenecity on heat-induced gelation behavior. Journal of Agricultural and Food Chemistry 2004, 52, 3149–3154.
- Dharmaraj, U.; Ravi, R.; Malleshi, N.G. Physicochemical and textural characteristics of expanded finger millet. International Journal of Food Properties 2012, 15, 336–349.
- Vázquez da Silva, M.; Delgado, J.M.P.Q.; Goncalves, M.P. Impact of MG2+ and tara gum concentrations on flow and textural properties of WPI solutions and cold-set gels. International Journal of Food Properties 2010, 13, 972–982.
- SAS Institute Inc. SAS/STAT User’s Guide, 4th Ed; Cary, NC, Vol. 6, 1990.
- Shi, J.; Malik, J. Normalized cuts and image segmentation. IEEE Transactions on Pattern Analysis and Machine Intelligence 2000, 22 (8), 888–905.
- Fishbein, W.N. Quantitative densitometry of 1-50 μg protein in acrylamide gel slabs with coomassie blue. Analytical Biochemistry 1972, 46 (2), 388–401.
- Yamada, T.; Aibara, S.; Morita, Y. Accumulation pattern of arachin and its subunit in maturation of groundnut seeds. Plant Cell Physiology 1980, 21, 1217–1226.
- Monteiro, P.V.; Prakash, V. Effect of proteases on Arachin, conarachin I and conarachin II from peanut (Arachis hypogaea L.). Journal of Agricultural and Food Chemistry 1994a, 42, 268–273.
- Monteiro, P.V.; Prakash, V. Functional properties of homogeneous protein fractions from peanut (Arachis hypogaea L.). Journal of Agricultural and Food Chemistry 1994b, 42, 274–278.
- CAMO Software AS, Nedre Vollgate 8, N-0158 OSLO, Norway. 1998.
- Brereton, R.G. Chemometrics–Applications of Mathematics and Statistics to Laboratory Systems, Ellis Horwood Limited: West Sussex, UK, 1990.
- Skurray, G.; Cunich, J.; Carter, O. The effect of different varieties of soybean and calcium ion concentration on the quality of tofu. Food Chemistry 1980, 6, 89–95.
- Taira, H. Quality of soybeans for processed foods in Japan. Journal of Agriculture Research Quality 1990, 24, 224–230.