Abstract
Crucial physio-chemical changes occuring in eggs during storage after laying lead to loss of egg freshness. In this research, a new method for prediction of egg freshness using transmission visible near infrared spectroscopy was investigated. For this purpose 300 eggs were stored at two control conditions: refrigerator (4–5°C, 75%RH) and room (24–25°C, 40%RH) then by special egg holder, transmission spectroscopy was measured. For two eggs groups, 25 eggs in each group, in six days were tested by spectroscopy, after that Haugh unit and air cell height was measured directly. The non-destructive visible near infrared spectroscopy spectral measurements from 300 to 1100 nm (832 length of wave) were done as well as Haugh unit, air cell height for each egg and created the database for both environments. Finally a maximum likelihood latent root regression algorithm was developed to predict Haugh unit and air cell height by spectrum observation. The database was randomly divided into two parts. Training data, was used for maximum likelihood latent root regression parameter tuning and training of the model and testing data, was used just for model evaluation. Results indicated that maximum likelihood latent root regression method showed good prediction ability with coefficient of determination (R2) value up to 0.82 and 0.86 for Haugh unit and air cell height, respectively for testing data set. The results showed this method was better in comparison with partial least square regression (R2 up to 0.79 and 0.72 for air cell height and Haugh unit) which was already used for this prediction.
INTRODUCTION
Prediction of important changes occuring in eggs during storage is critical in order to monitor egg freshness. The internal quality change during storage is based on air cell size, albumen quality, yolk quality, and the presence of blood or meat spots. One of the most important interior egg qualities is the albumen height. Albumen (egg white) accounts for most of an egg’s liquid weight, about 67%. Albumen quality can also give an indication of food safety. When a freshly laid egg is broken onto a smooth flat surface, the yolk is generally in a central position surrounded by thick albumen. However, the thick albumen becomes thinner with time resulting in a larger area displacing the yolk placement to one side.[Citation1Citation2] The rate at which these changes occur during storage depends on storage duration, relative humidity, temperature, and hen age and strain.[Citation3Citation5] Studies carried out in order to set up techniques for on-line prediction of the days of storage and the assessment of the egg freshness parameters concerned the dielectric properties analyses, the use of an electronic nose-based system and the Vis-NIR spectroscopy.[Citation6–Citation11] A predictive model of thick albumen height was obtained with a determination coefficient of 0.78 by means of diffuse reflectance Fourier Transform (FT-NIR) spectroscopy.[Citation12] Spectroscopy is the study of the interaction between matter and optic radiated energy. It is a new non-destructive method where the optic light was radiated to the poultry egg in one side and in the other side the energy of each wavelength was measured. It has the advantage of fast and non-contact method. The egg was affected like an optic filter and this energy is changed under the influence quality of poultry egg. The success of this technique is due to the continuous improvements of the sensors and instrumentations capable of supporting complex calculations based on multivariate statistical methods such as principal components analysis (PCA) and partial least squares regression (PLS).[Citation13Citation13] The advantage of optical measurements is that their nature is non-destructive, non-contact, and fast.
The PLS algorithm could consider simultaneously the variable matrix Y (Haugh unit [HU], air cell height) and the variable matrix X (power spectrum). To reduce the dimension and compress the original spectral data, the PCA were considered as new eigenvectors of the original spectra. In the development of PLS model, was used full cross-validation to evaluate the quality and prevent over fitting of calibration model. The number of PLS components (i.e., PCs) can affect the performance of PLS model, and it should be optimized by cross-validation in model calibration.
Maximum likelihood latent root regression (MLLRR) has also significantly demonstrated marginal improvements over maximum likelihood principal components regression (MLPCR), but this will depend on the error structure, particularly as it pertains to the errors in outputs data. MLPCR has demonstrated significant improvement over principle component regression (PCR) and PLS under the right error conditions. An important feature of MLLRR is its tremendous versatility in terms of the error structures that can be accommodated. In some ways, the relationship between MLPCR and MLLRR can be considered analogous to the relationship between PCR and PLS, the latter using the information in output data to obtain a more condensed representation of the factor space better suited for prediction. The analogy is not exact, however, since PLS uses a somewhat different modeling philosophy.[Citation18,Citation19] The objective of this research was to introduce MLLRR method for prediction of egg freshness in terms of internal egg quality of HU and air cell height. This experiment aimed to improve the technique’s reliability.
MATERIALS AND METHODS
Samples
Tests were carried out on 300 medium-sized commercial eggs collected from 33-week-old Boris Brown hens from the Kei farm (Kyoto, Japan) on the day the eggs were laid. On arrival at the laboratory, 50 eggs were analyzed, and the rest were stored under controlled conditions. One group was kept at refrigerator condition (4–5°C, 75% relative humidity), the another group was kept at room condition (24 ± 1°C, 40% relative humidity) in order to cause a variation in HU and air cell height. Twenty-five eggs from each group were selected for analysis every six days over a five-week storage period.
Quality Egg Parameters
The quality parameters considered were: temperature (°C) and humidity (%), air cell height (mm), thick albumen height (mm), and HU. The air cell height is measured from the base of the shell in three equidistant points on the circumference described by the contact line of the membrane to the shell, and at the middle point of the same membrane. The air cell height was obtained averaging the values of these four points. The thick albumen was measured by averaging three measurements carried out at different points of the thick albumen at the distance of 10 mm from the yolk. The HU was calculated by using the following formula[Citation13]:
Vis-NIR spectroscopic data
Vis-NIR transmission data of 300 Borris Brown hen eggs of the same flock fed with a standard ration for each egg were obtained using spectroscope in 832 wavebands. The set up transmittance measured at wavelengths from 300–1100 nm with 1 nm increments. For this purpose a special egg holder was used to hold eggs between incident and transmitted light (). The egg is placed on a foam ring with its blunt end pointed upwards. This configuration was chosen in order to reproduce the typical egg orientation in a grading machine. An optical fiber is placed on one side of the egg and transports the transmitted light into an Avaspec-2048 spectrophotometer (Avantes, Eerbeek, The Netherlands). A xenon lamp was used as a light source and a Teflon block was used for calibration. Because light is not molecularly absorbed and scattered when it passes Teflon, the transmission spectrum of Teflon is nearly flat in the visible and NIR range. This makes it suitable as a reference measurement.
Figure 1 Experimental set-up for the measurements of transmission spectroscopy (LAX-Cute, 100W Xenon light source, Asahi Spectra).
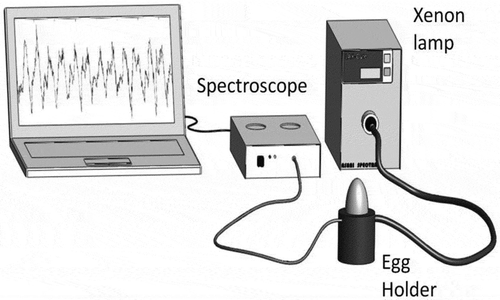
For each egg, a non-destructive spectral transmission measurement and then determination of destructive freshness parameters (HU and air cell height) were performed. The spectral data of each egg was obtained by averaging three measurements and the acquisition time for each measurement was 250 ms. After spectra measurment the eggs were weighed (± 0.01 g) and broken. The albumen height was determined (± 0.25 mm) by means of a vertically mounted micrometer. Based on these measurements, the HU was determined by formula (1). Typically wavelength to response attitude for two random data illustrated in .
Data Processing
The goal of the identification is finding the best function between HU, air cell height, and the Vis-NIR spectroscopy data which already obtained from stored eggs in room and refrigerator conditions. There are 832 wavelengths, which is known as regressor, from Vis-NIR spectroscopy, the air cell height and HU for each egg. In other words, the aim of identification is finding the best two mappings between 832 regressors in one hand and HU and air cell height in the other hand. Sample points and numbers of eggs, is less than the regressors number and so the following parts must be done in this case:
Subset selection: In this part the number of regressors must be reduced by eliminating regressors which has non-significant effect or finding a linear mapping matrix, to reduce the size of regressors data.
Finding a map between reduced regressors, air cell and HU, using MLLRRFootnote1 method.
Subset Selection
For this part SVDFootnote2 the composition is used for regressors reduction by defining a new parameters, λ, which is used for control of number of regressors. Singular value decomposition of an m × n real or complex matrix M is a factorization of the form.
MLLRR
If the statistical characteristic of measured data was known the maximum likelihood method is best estimator because this method searches for best match model. The result of this method is the same as the result of least square (LS) method in this saturation. Parameter estimation methods are widely used because of their desirable statistical characteristics. By this method the parameters determined for the model are the ones most likely to give rise to the observed data, given the statistical characteristics of the measurement uncertainties.[Citation2] In this research the MLLRR was used for pure approach. With this technique, the original calibration matrix of response variables is augmented by the corresponding concentration vector(s). In this way, the reduction of dimensionality and the determination of the calibration model are performed simultaneously. For a single component there is:
MLLRR is more general in its treatment of measurement errors than other method that it includes uncertainties in the concentration values. It is an optimal modeling method in the maximum likelihood sense, subject to the usual restrictions (linear model of known pseudorank, normally distributed errors with a known covariance structure). All algorithms were implemented in Matlab V7.0 (Mathworks,USA) under Windows XP.
RESULTS AND DISCUSSION
Egg Freshness and Storage Time
Egg freshness mainly includes two indexes: HU and air cell height. In order to build the inspection freshness model with optical properties, a tracing test of the egg freshness had also been done for five weeks. shows the relationship between HU and air cell height under the controlled storage conditions. Since egg aging coincides with an increase in air cell height on the one hand and a decrease in the HU on the other hand, a similar but reverse interpretation can be given for the variations of these two parameters. The main reason for liquefaction of thick white is that amino acid based proteins, which are one of the building materials for thick whites, are influenced by aging eggs.
Destructive Analysis
Data analysis
Significant differences (p < 0.05) between mean values of the freshness parameters (air cell, thick albumen heights, and HU) at different storage durations were explored using ANOVA; Duncan’s multiple range test was used if significant differences emerged between variance means (SAS 9.1 for windows). Mean value of freshness parameters measured for each sample are summarized in for different storage conditions.
Table 1 Mean values of main physical attributes of 25 eggs measured at different storage condition
Relation Between Transmission Spectra and Destructive Freshness Parameters
Partial least square (PLS)
Consider the general model as follows:
Table 2 R2 value for egg quality indices at different storage conditions
Spectrum Data
All spectrum data were divided into two subsets. The first subset was used to train, the model build its structure and determine the model parameters. The second set, which was not included in the first set, was used to test the robustness and the generation power of the model. The training set contained 80% of all data where the remaining 20% was kept as test data. In this case there are many input data (830 regressors) which must be selected using subset selection algorithm as mentioned before. For air cell height and HU prediction, two separate models are proposed. So the first simulation for the different value of λ was run and R2 value of HU for training and testing data were calculated and shown in . Similarly, the air cell height model was simulated and R2 values of air cell for both subsets were calculated and shown in . The best value of λ and the number of regressors are reported in . An improvement in prediction of egg quality indices with 14 and 54 regressors compared to full spectrum was found. By eliminating less informative wavelengths and selecting the best regressors, MLLRR can improve the accuracy of model prediction. The difference between this study and previous work can be attributed to different predictive model and development of new experimental setup. According to , an extra test was run for room and refrigerator test data and R2 value was reported in .
Table 3 Best value for λ and number of regressors in train data
Table 4 R2 and λ value for HU and air cell height at different storage conditions
For data of eggs stored in different storage conditions, train and test data a simulation was run and showed the value of HU and air cell height. Results were illustrated in and . Albumen becomes thinner over time which leads to a change in the transmitted spectra. NIR spectra correspond to vibrational modes involving O-H chemical bonds.[Citation10]
Regresion Model Between Measured and Estimated Values
shows the predicted value by the model versus the measured value of HU and air cell height in room condition by performing the cross-validation of the non-destructive measurements of spectral transmittance. The validation was carried out in the full range of spectra. The predictive model produced satisfactory results with a high coefficient of determination between transmittance spectra and egg quality indices (R2 = 0.834, 0.88 for HU and air cell height, respectively). Also a similar trend can be found for eggs stored in refrigerated conditions as presented in
CONCLUSIONS
This work showed that the Vis-NIR transmission of an egg provides quantitative information about egg quality. PLS and MLLR models were built in order to predict the HU and air cell height with non-destructive spectral measurements. For the HU and air cell height the results showed an improvement in prediction with 14 and 54 wavelengths, respectively compared to those obtained with full spectrum. The developed model was used to relate the spectral data to HU and air cell height. For MLLR predictor, the R2 for the validation set were 0.823, 0.857 for HU and air cell height, respectively and values of 0.72, 0.79 for the same in PLS model. R2 value using maximum likelihood method compared to PLS illustrated MLLR method is better for prediction of egg freshness. In conclusion, the MLLR model can improve the predictive ability, decrease the model complexity and reduce the time of analysis.
ACKNOWLEDGMENT
The authors would like to thank the laboratory of Agriculture Process Engineering, Kyoto University for their technical support.
Notes
2. 1Maximum Likelihood Latent Root Regression
3. 2Singular Value Decomposition
REFERENCES
- Shrimpton, D.H. The nutritive value of eggs and their dietary significance. In: Egg Quality-current Problems and Recent Advances; Wells, R.G.; Belyavin, C.G.; Eds.; Butterworth: Toronto, 1987.
- Robinson, D.S.; Mosey, J.B. Changes in the composition of ovomucin during liquifation of thick white. Journal of the Science of Food and Agriculture 1972, 23, 29–38.
- Roberts, J.R. Factors affecting egg internal quality and egg shell quality in laying hens. Journal of Poultry Science 2004, 41, 161–177.
- Scott, T.A.; Silversides, F.G. The effect of storage and strain of hen on egg quality. Poultry Science 2000, 79, 1725–1729.
- Silversides, F.G.; Scott, T.A. Effect of storage and layer age on quality of eggs from two lines of hens. Poultry Science 2001, 80, 1240–1245.
- Ragni, L.; Gradari, P.; Berardinelli, A.; Giunchi, A.; Guarnieri, A.; Predicting quality parameter of shell eggs using a simple technique based on the dielectric properties. Biosystems Engineering 2006, 94 (2), 255–262.
- Dutta, R.; Hines, E.L.; Gardner, J.W.; Udrea, D.D.; Boilot, P. Non-destructive egg freshness determination; an electronic nose based approach. Measurement Science and Technology 2003, 14, 190–198.
- Abdel-Nour., N.; Ngadi, M.; Prasher, M.; Karimi, Y. Prediction of Egg Freshness and Albumen Quality Using Visible/Near Infrared Spectroscopy. Food Bioprocess Technology 2011, 4, 731–736.
- Karoui, R.; Kemps, B.; Bamelis, F.; De Ketelaere, B.; Merten, K.R.; Schoonheydt, R.; Decuypere, E.; De Baerdemaeker, J. Development of a rapid method based on front face fluorescence spectroscopy for the monitoring of egg freshness: 1—evolution of thick and thin egg albumens. European Food Research and Technology 2006, 223, 303–312.
- Karoui, R.; Kemps, B.; Bamelis, F.; De Ketelaere, B.; Merten, K.R.; Schoonheydt, R.; Decuypere, E.; De Baerdemaeker, J. Development of a rapid method based on front-face fluorescence spectroscopy for the monitoring of egg freshness: 2—evolution of egg yolk. European Food Research and Technology 2006, 223, 180–188.
- Narushin, V.G. Non-destructive measurements of egg parameters and quality characteristics. World’s Poultry Science Journal 1997, 53 (2): 141–153.
- Abdel-Nour., N.; Ngadi, M.; Prasher, M.; Karimi, Y. Combined Maximum R2 and Partial Least Square Wavelengths Selection and Analysis of Spectroscopic Data. International Journal of Poultry Science 2009, 8 (2), 170–178.
- Haugh, R.R. A new method for determining the quality of an egg. US Egg Poultry 1937, 39, 27–49.
- Giunchi, A.; Berardinelli, A.; Ragni, L.; Fabbri, A.; Silaghi, F. Non-destructive freshness assessment of shell eggs using FT-NIR spectroscopy. Journal of Food Engineering 2008, 89, 142–148.
- Kemps, B.J.; Bamelis, F.; De Ketelaere, B.; Mertens, K. M.; Decuypere, E.; De Baerdemaeker, J. Visible transmission spectroscopy for the assessment of egg freshness. Journal of Science and Food in Agriculture 2006, 86, 1399–1406.
- Kestens, V.; Charoud-Got, J.; Bau, A.; Bernreuther, A.; Emteborg, H. Online measurement of water content in candidate reference materials by acoustoopticaltuneable filter near-infrared spectrometry (AOTF-NIR) using pork meat calibrants controlled by Karl Fischer titration. Food Chemistry 2008, 106, 1359–1365.
- Balabin, R.M.; Safieva, R.Z.; Lomakina, E.I. Comparison of linear and nonlinear calibration models based on near infrared (NIR) spectroscopy data for gasoline properties prediction. Chemometrics and Intelligent Laboratory Systems 2007, 88, 183–188.
- Wentzell, P.D.; Andrews, D.T.; Kowalski, B.R. Maximum likelihood multivariate calibration. Analytical Chemistry 1997, 69, 2299–2311.
- Wentzell, P.D.; Andrews, D.T.; Hamilton, D.C.; Faber, K.; Kowalski, B.R. Maximum likelihood principal component analysis. Journal of Chemometrics 1997, 11, 339–366.