ABSTRACT
Most typical approaches for measuring color and texture properties are time consuming. An image-based method was used to evaluate the texture and color in freeze-dried potatoes. Microstructure of cooked, cooked freeze-dried and rehydrated potatoes were analyzed by scanning electron microscopy. Texture properties were analyzed by a texture analyzer and image analysis. Color was determined with a spectrocolorimeter and a digital camera. Significant differences (p < 0.05) were obtained for cooked and cooked freeze-dried rehydrated potatoes. A linear trend was applied for instrumental and image features for texture and color values. Results showed that texture features of images, such as contrast, entropy, energy, and homogeneity (calculated from grey level co-occurrence matrix), showed correlations with instrumental texture features, such as hardness, adhesiveness, chewiness, cohesiveness, and resilience. Similar results were also obtained for color features. This study showed that image processing techniques could be applied as a useful tool to analyze quality in potatoes.
Introduction
The potato (Solanum tuberosum L.) is one of the world’s major crops and it is consumed daily by millions of people from diverse cultural backgrounds. Potatoes exhibit a high nutritional value such as high content of carbohydrates, vitamins, pleasant taste, and high digestibility. Among many quality parameters, color is considered the most important visual attribute in the perception of the product quality, it is critically appraised by consumers and often is the basis for their selection or rejection.[Citation1] Consumers tend to associate color with texture, flavor, safety, storage time, and nutrition because it correlates well with physical, chemical, and sensorial properties. Along with the color, texture also plays an important role in the overall acceptance of food quality by consumers. Texture in food has been defined as “all the rheological and structural (geometric and surface) attributes of the product perceptible by means of mechanical, tactile, visual and auditory receptors.”[Citation2]
Quality parameters are usually measured by conventional techniques, such as sensory and instrumental methods, which are time consuming and destructive to the product. The relationship of instrumental variables to the human perception of texture, color, etc. has been a very active area of research, with many researchers addressing this topic.[Citation3,Citation4] An interesting alternative for analyzing the surface of food products and quantifying appearance characteristics is to use computerized image analysis techniques.[Citation5,Citation6] Image analysis can be a useful tool for characterizing food morphology because the highly irregular structures of many food materials elude precise quantification by conventional means. This technique makes it possible to obtain measurements from digitalized images providing objective evaluations of the morpho-colorimetric features of samples, a method that is more quantitative and less biased than the common method of visual perception, which is prone to variation due to the personal opinions of inspectors or trained panels.[Citation7]
Grey level co-occurrence matrix (GLCM) is probably one of the most frequently cited methods for the texture analysis of images. The texture of an image corresponds to the spatial organization of pixels in the image and the co-occurrence matrix describes the occurrence of grey level between two pixels separated in the image by a given distance. GLCM results in the calculation of up to 14 textural features which can be expected to represent the textural characteristics of the studied image.[Citation8–Citation10] When microscopy techniques, such as scanning electron microscopy (SEM) and images analysis, are used together become a powerful tool to evaluate the microstructure changes of a product; cell size and number of cells can then be measured and quantified from the projected image.[Citation11,Citation12] While employing image processing with SEM, some important sensory attributes, such as texture, could be predicted by processing the surface and cross-section images of a product.[Citation13,Citation14] The aim of the present research was to evaluate color and texture in potato cv Spunta submitted to freeze drying for instant meal applying image analysis techniques. Data obtained by image analysis was correlated with instrumental analysis in order to evaluate effectiveness of the method.
Material and methods
Samples, preparation for freeze drying, color, and texture analysis
Samples of potatoes, cv Spunta, were obtained at a local farm. A preliminary evaluation to set treatment conditions, temperatures, and water requirements was performed in order to improve analysis. Parameters applied were as follows: Potatoes (100 g of each sample) were added to 946 mL of boiling water and cooked for 10 min after the water returned to boiling. Cooked potato (CP) were cooled in water for 5 min, drained on paper towels to remove excess of water, spread on plastic trays, and covered with aluminium foil until the freeze-drying process.
CPs were freezed-dried using a non-continuo equipment (Rificor, Argentina). Parameters were set at: freezing temperature: (–50 ± 1°C) for 24 h; drying process: (40 ± 1°C) at maximum vacuum (pressure: 0.346 Pa) during 11 h. Cooked freeze-dried potato (CFDP) were vacuum packaged, individually identified, and stored in a dark place at room temperature until analysis. In order to analyze texture and color parameters of cooked freeze-dried rehydrated samples (CFDRP), rehydration was performed with tap water at 98°C. The duration of rehydration process was fixed in 5 min as after that time, there was no more absorption of water by the sample.
SEM
SEM was used for the observation of the microstructure of the CP, CFDP, and CFDRP. Samples were cross-sectioned using a scalpel; the cut was always performed in the same direction. A dehydration process in the CPs and CFDRPs was performed with ethanol series, acetone, and then dried. Samples were mounted on holders and coated with gold. Microscopic evaluation was performed using a scanning electron microscope (SEM 515, Philips, USA). Observations of the samples at magnifications of 250–5000× were obtained for image analysis (Model Genesis Version 5.21.). Brightness and contrast are the most important variables that must be controlled during the acquisition of images; therefore, the values of these parameters were kept constant for each magnification during the process of image acquisition.
Color parameters
Instrumental analysis
Color parameters (L*, a*, and b*) were measured using a spectrocolorimeter BYK Gardner Spectro guide 45/0 gloss. Among the several existing color scales, CIELAB color space is a three-dimensional spherical system defined by three colorimetric coordinates. The coordinate L* is called the lightness. The coordinates a* and b* form a plane perpendicular to the lightness. The coordinate a* defines the deviation from the achromatic point corresponding to lightness, to red when it is positive and to green if negative. Similarly, the coordinate b* defines the turning to yellow if positive and to blue if negative. Instrumental conditions included a 5 cm diameter aperture, D65-iluminant and 10° standard angle observer. Samples were measured at room temperature. Each color parameter was measured five times and its average value was used for statistical analysis. Values of L*, a*, and b* were used to calculate Hue angle (h*ab), where h*ab = 0° for red hue and h*ab = 90° for yellowish hue; chroma (C*ab) and whiteness index (WI).
Image analysis
Samples were illuminated using a lamp (TL-D Deluxe model, Natural Daylight, 18W/965, Philips, NY, USA) with a color temperature of 6500 K (D65, standard light source) and a color-rendering index (Ra) close to 90%. The four fluorescent tubes (60 cm long) were situated 35 cm above the sample and at an angle with the sample of 45°. Additionally, light diffusers covering each lamp and electronic ballast assured a uniform illumination system. A color digital camera (CDC), Canon Eos Rebel was located vertically over the sample at a distance of 12.5 cm. The angle between the camera lens and the lighting source axis was around 45°. Lamps and CDC were inside a wooden box with internal walls that were painted black to avoid the light and reflection from the room.
Eighteen images from one side of each sample and eight regions of interest were taken on the matte black background using the following camera settings: manual mode with the lens aperture at f of 4.5 and speed 1/125, no zoom, no flash, 3088 × 2056 pixels resolution of the CDC and storage in JPEG format. The algorithms for pre-processing of full images, image segmentation and color quantification were processed by Adobe Photoshop cs6 (v13.0 Adobe Systems Incorporated. 2012). L, a, and b values were transformed to CIE L*, a*, and b*. Values of L*, a*, and b* were used to calculate Hue angle (h*ab), where h*ab = 0° for red hue and h*ab = 90° for yellowish hue, chroma (C*ab), and WI.
Instrumental and image texture parameters
Textural profile analysis (TPA)
The TPA of the CP and CFDRP was performed individually using a texture analyzer (TA-XT—Texture Technologies Corp., UK) with a 5 kg load cell using a two-cycle compression method. Samples were compressed 3 mm with a time interval of 5 s at a speed 5.0 mm.sec−1. Values for hardness (HAR: peak force of the first compression cycle in N), cohesiveness (COH: ratio of the positive force area during the second compression to that during the first compression), springiness (SPRIN: ratio of the time duration of force input during the second compression to that during the first compression), resilience (RES: ratio of the negative force input to positive force input during the first compression), adhesiveness (ADH: negative area under the baseline between the compression cycles), and chewiness (CHEW: hardness multiplied by cohesiveness multiplied by springiness in N) were measured.[Citation15] Results were reported as an average value. Triplicates were analzed.
Image texture analysis: GLCM
Eighteen SEM images samples and eight regions of interest of each acquired SEM images were selected. Textural property was computed from a set of GLCM probability distribution matrices for a given image. The GLCM shows the probability that a pixel of a particular grey level occurs at a specified direction and distance (d = 1) from its neighboring pixels. Gray level co-occurrence matrix is represented by Pd,θ (i, j) where counts the neighboring pair pixels with gray values i and j at the distance of d and the direction of θ.[Citation16,Citation17] Five image texture features (Correlation [COR], Energy [ASM], Homogeneity [HOM], Entropy [ENT], and Contrast [CON]) were calculated using MATLAB 8.4 (The MathWorks, Inc., MA, USA; Eq. [4–8]):
where ux, uy, and
are the means and standard deviations of px and py.
Statistical analysis
Texture and color parameters were subjected to linear normalization prior to principal component analysis (PCA) in order to efficiently suppress quantitative effects on the multivariate data. Mean values were compared by T-Student. Regression equations and correlation coefficients (R2) were performed between instrumental and image texture features using SPSS-Advanced Statistics 12 software (SPSS Inc., Chicago, IL).
Results and discussion
SEM
Micrographs taken from a cross-sectional cut of the CP, CFDP, and CFDRP are shown in . CP () showed an organized structure without gaps; the cell wall is seen as a polymer network of polysaccharides. Cells surfaces of CP walls were smooth, flat, uniform, and regular. Potato cell walls are strong and hard to break. As the cells walls break down they no longer have a strong rigid structure. Cell walls are composites of microstructures of cellulose microfibrils embedded in a matrix of polysaccharides and some proteins.[Citation18] During the cooking process, the breakdown of cells is usually due to cell separation and not cell rupture. Cooking at high temperature can produce larger pore size and bulkier starch matrix.[Citation19]
Figure 1. Scanning electron micrographs performed at 250, 500, and 5000 × of A: cooked; B: cooked freeze-dried; and C: cooked freeze-dried rehydrated potato.
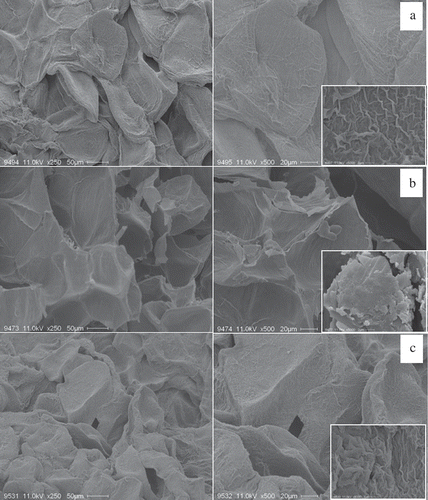
Structures in the CFDP showed that cell walls were dehydrated, separated, and partially fragmented. Fragility of cell walls appeared; especially tearing of cell walls from their base (). High porous size structure with larger and irregular cavities was observed in CFDP. Pore cavities are due to ice sublimation during the freeze-drying process. CFDRP () showed that due to porous size structure water easily reoccupied the empty spaces in all samples. Surfaces of CFDRP were smooth, flat, uniform, and regular, similar to the CP. Porosity in the samples are due to the freeze-drying process which depends on different factors, such as pressure in the freeze dryer, drying temperature, and water uptake. In the freeze-drying process, high porosity helps to maintain the structure without the deformations that are inevitable in other drying methods, allowing for a fast rehydration process because water easily reoccupies the empty spaces.[Citation20] A general view of all the micrographs showed that microstructure of the CP and CFDRP had similar structures, showing that porosity seemed to be gradually dispersed due to a fast and good rehydration process after freeze-drying.
Instrumental and image texture analysis
Instrumental and image texture values for CP and CFDRP are shown in . Significant differences (p < 0.05) were obtained for ASM, CON, ENT, HOM, ADH, CHEW, COH, HARD, and RES in the CP and CFDRP. The CP showed higher texture values when it was related to CFDRP. In image analysis, COR indicates the linearity of the image; for an image with large areas of similar intensities, a high value of correlation is measured. ENT takes low values for smooth images. ASM represents the smoothness of an image. CON is a measure that shows the difference from one pixel to others close to it, representing a measure of local gray variations; the softer the texture the lower the contrast. HOM shows improvement of uniformity and smoothness of the image.[Citation16]
Table 1. Texture parameter values of cooked and cooked freeze-dried rehydrated potato (cv. Spunta).
The image analysis revealed that CFDRP had lower CON (softness) and lower ASM (smoothness in image) values. Smoothness, softness, and low linearity in the image was obtained for the CFDRP. The CP showed higher ASM (roughness) values, hardness, and higher linearity in the image. Instrumental texture values showed that the CP had higher HARD values when compared to CFDRP; the CP showed to be harder than CFDRP. In general, instrumental and image analysis showed that CFDRP was softer than CP. Increases in softness in freeze-dried samples can be attributed to its porous structure. High porosity (amounts of pores) helps to maintain the structure without the deformations that are inevitable in other drying methods. The degree of porosity also has influence in texture and rehydration ability, when the size of the air cells in porous material are bigger, it allows a fast rehydration due that water easily enters and reoccupies the empty spaces.[Citation21] During the subsequent freezing and freeze-drying, the ice sublimation creates pores; the amount of pores (porosity) is related to the water uptake and is higher when the water uptake is increased. The porous structure is also influenced by the freezing process; a cooling process with a high under-cooling procedure leads to smaller ice crystals and a larger inner surface. Due to the high porosity in the freeze-dried cell suspension, there is a high specific surface area; this influences the sorption behavior, as well as the rehydration process.[Citation22]
Sandhu et al.[Citation23] reported that textural changes in potato can also be associated with the gelatinization and retrogradation behavior of starch and breakdown of the cell wall and middle lamellae structural components. Csilla et al.[Citation24] stated that starch is the major component of the dry matter of potatoes therefore, its molecular organization and interactions with non-starch polysaccharides and sugars are important factors influencing texture in potatoes. Results showed that instrumental and image analysis had similar results for the CP and CFDRP. Changes in texture can be due to the points stated previously. If there is a lower amount of pores and a higher pore size, a fast rehydration process is expected; samples are softer, the smoothness and high linearity of the image appears.
Instrumental and image color analysis
The color values for the CP, CFDP, and CFDRP are shown in . The color parameters (L*, a*, b*, C*ab, WI, and h*ab) showed significant differences (p < 0.05). Higher L*, b*, and C*ab values and lower a*, WI, and h*ab values were obtained for the CP. ∆E[Citation25] values for instrumental and image color analysis was 11.5 and 10.9, respectively. The CFDRP samples were shown to be softer, bigger, whiter, and the vividness decreased when it was compared to the CP. The color of potatoes is an extremely important criterion strictly related to consumer perception. The aspect and color of the food surface is the first quality parameter evaluated by consumers and is critical in the acceptance of the product, even before it enters the mouth.[Citation26] Consumers tend to associate color with flavor, safety, storage time, nutrition, and satisfaction level due to the fact that it correlates well with physical, chemical, and sensorial evaluations of food quality.
Table 2. Color parameters of cooked, cooked freeze-dried, and cooked freeze-dried rehydrated potato (cv. Spunta).
Changes in color can be due to dehydrating procedure which affects drying kinetics and rehydration kinetics among the process.[Citation27] Drying methods with higher temperatures in potatoes can affect color, increasing WI, yellowness (b*), and decreasing L* values in dehydrated samples when compared to cooked samples.[Citation28,Citation29] Color can also be affected by the cooking process which leads to a Maillard reaction, resulting in characteristic golden brown zones.[Citation30] Freezing before drying also affects color, it can provide a whiter product with a less uniform porous structure and higher bulk density.[Citation31,Citation32] Moisture also has influence on color; higher moister promotes starch gelatinization, protein denaturation, and the development of darker pigments.[Citation33] The results revealed that applying instrumental or image analysis in color, both techniques showed similar results. Changes in color are due to the points previously stated.
Correlation between instrumental and image texture
In order to correlate instrumental and image analysis a linear trend with a linear correlation was analyzed for texture (instrumental versus texture image features [ADH, CHEW, COH, RES, and HARD versus ASM, CON, ENT, and HOM]) and for color (instrumental versus color image features). For texture analysis, PCA was performed with ADH, CHEW, COH, RES, and HARD (instrumental) and with ASM, CON, ENT, and HOM (image). Two PCs were found for instrumental features accounting 90.7% of the total variance and two PCs were found for image features accounting 89.7% of the total variance. Each PC score was multiplied by the respective variance. PC1 of instrumental and image texture were combined using a linear combination. shows linear regression of PCA scores of texture features (instrumental versus image texture). The linear regression analysis revealed a strong relationship between instrumental and image features (R = 0.918), based on the resulting R2 value, the model explained 84.0% of the variability that associated instrumental with image features.
Figure 2. Linear regressions of PCA scores of texture obtained by instrumental and image analysis of (■) cooked; and (♦) cooked freeze-dried rehydrated potato.
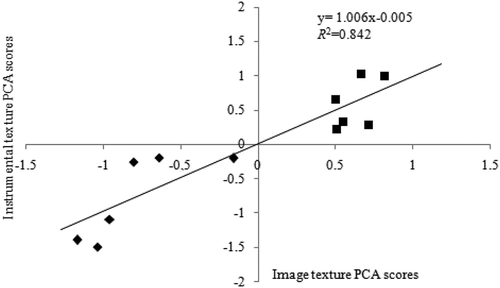
A similar analysis method was applied for color parameters (instrumental and image). PCA analysis was performed; two PCs were found for instrumental features accounting 96.7% of the total variance and two PCs were found for image features accounting 95.1% of the total variance. Each PC score was multiplied by the respective variance. PC1 of instrumental and image features were combined using a linear combination. PC1 of instrumental and image color parameters were combined using a linear combination. Linear regression analysis revealed a strong relationship between instrumental and image (R = 0.907), based on the resulting R2 value, the model explained 87.0% of the variability associated instrumental with image (). Results showed that image analysis can be used to estimate instrumental features, such as color and texture, in potatoes.
Conclusions
An image-based technique was developed to approach texture and color parameters in potatoes. Image texture analysis showed that energy, entropy, contrast, and homogeneity calculated from GLCM had correlations with instrumental texture features (adhesiveness, chewiness, cohesiveness, hardness, and resilience). Similar results were obtained for color parameters. When SEM and image analysis were used together, they became a powerful tool because important sensory attributes could be predicted by processing the surface and cross-section images of the product. Results obtained in this research showed that image analysis can be used to predict texture and color in potatoes; it can also be performed easily as a quantitative and non-invasive technique that could be related in future studies for quality. The authors recommend that other cultivars are also investigated to increase the number of samples in future research in order to establish a general trend and to improve the research.
References
- Carmo, J.; Barbosa de Lima, A.Drying of Lentils Including Shrinkage: A Numerical Simulation. Dry Technology 2005, 23, 1977–1992.
- Lawless, H.; Heymann, H. Sensory Evaluation of Food: Principals and Practices. Chapman and Hall: New York, NY, 1998.
- Luckett, C.; Kuttappan, V.; Johnson, L.; Owens, C.; Seo, H. Comparison of Three Instrumental Methods for Predicting Sensory Attributes of Poultry Deli Meat. Journal of Sensory Studies 2014, 29, 171–181. https//doi:10.1111/joss.12092
- Guzek, D.; Głąbska, D.; Pogorzelska, E.; Pogorzelski, G.; Wierzbick, A. Instrumental Measurement of Meat in a Laboratory Research and on a Production Line. Advances in Science and Technology Research Journal 2013, 7, 5–11.
- Mendoza, F.; Lu, R.; Diwan, A.; Cen, H.; Bailey, B. Integrated Spectral and Image Analysis of Hyperspectral Scattering Data for Prediction of Apple Fruit Firmness and Soluble Solids Content. Postharvest Biology and Technology 2012, 62, 149–160.
- Tournier, C.; Grass, M.; Zope, D.; Salles, C.; Bertrand, D. Characterization of Bread Breakdown During Mastication by Image Texture Analysis. Journal of Food Engineering 2012, 113, 615–622.
- Kono, S.; Kawamura, I.; Yamagami, S.; Araki, T.; Sagara, Y. Optimum Storage Temperature of Frozen Cooked Rice Predicted by Ice Crystal Measurement, Sensory Evaluation and Artificial Neural Network. International Journal of Refrigeration 2014, 56, 165–172.
- Saini, M.; Singh, J.; Prakash, N. Analysis of Wheat Grain Varieties Using Image Processing: A Review. International Journal of Science and Research 2014, 3, 490–495.
- Haralick, R.; Shanmugam, K.; Dinstein, I. Texture Features for Image Classification. IEEE Transaction on Systems Man and Cybernetic 1973, 3, 610–621.
- Tahir, A.; Neethirajan, S.; Jayas, D.; Shanin, M.; Symons, S.; White, N. Evaluation of the Effect of Moisture Content on Cereal Grains by Digital Image Analysis. Food Research International 2007, 40, 1140–1145.
- Naganathan, G.; Grimes, L.; Subbiah, J.; Calkins, C.; Samal, A.; Meyer, G. Visible/Near-Infrared Hyperspectral Imaging for Beef Tenderness Prediction. Computers and Electronic in Agriculture 2008, 64, 225–233.
- Kamruzzamana, M.; ElMasrya, G.; Suna, D.; Allenb, P. Prediction of Some Quality Attributes of Lamb Meat Using Near-Infrared Hyperspectral Imaging and Multivariate Analysis. Analytica Chimica Acta 2012, 714, 57–67.
- Barrera, G.; Calderón-Domínguez, G.; Chanona-Pérez, J.; Gutiérrez-López, G.; Leóna, A.; Ribott, P. Evaluation of the Mechanical Damage on Wheat Starch Granules by SEM, ESEM, AFM and Texture Image Analysis. Carbohydrate Polymers 2013, 98, 1449–1457.
- Xiong, Z.; Sun, D.; Pu, H.; Zhu, Z.; Luo, M. Combination of Spectra and Texture Data of Hyperspectral Imaging for Differentiating Between Free-Range and Broiler Chicken Meats. LWT–Food Science and Technology 2015, 60, 649–655.
- Caine, W.; Aalhus, J.; Best, D.; Dugan, M.; Jeremiah, L. Relationship of Texture Profile Analysis and Warner-Bratzler Shear Force with Sensory Characteristics of Beef Rib Steaks. Meat Science 2003, 64, 333–339.
- Karimi, M.; Fathi, M.; Sheykholeslam, Z.; Sahraiyan, B.; Naghipoor, F. Effect of Different Processing Parameters on Quality Factors and Image Texture Features of Bread. Journal of Bioprocess and Biotechnology 2012, 2, 12–17.
- Azizi, A.; Abbaspour-Gilandeh, Y.; Nooshyar, M.; Afkari-Sayah, A. Identifying Potato Varieties Using Machine Vision and Artificial Neural Networks. International Journal of Food Properties 2016, 19, 618–635.
- Mohd Hanim, A.; Chin, N.; Yusof, Y. Rheological Characterisation of Malaysian Varieties of Sweet Potato Doughs Using Large and Small Deformation Measurements. International Journal of Food Properties 2015, 18, 963–977.
- Leelayuthsoontorn, P.; Thipayarat, A. Textural and Morphological Changes of Jasmine Rice Under Various Elevated Cooking Conditions. Food Chemistry 2006, 96, 606–613.
- Oikonomopoulou, V.; Krokida, M.; Karathanos, V. The Influence of Freeze Drying Conditions on Microstructural Changes of Food Products. Procedia Food Science 2011, 1, 647–654.
- Oikonomopoulou, V.; Krokida, M.; Karathanos, V. Structural Properties of Freeze-Dried Rice. Journal of Food Engineering 2011, 107, 326–333.
- Leelayuthsoontorn, P.; Thipayarat, A. Textural and Morphological Changes of Jasmine Rice Under Various Elevated Cooking Conditions. Food Chemistry 2006, 96, 606–613.
- Mounir, S. Texturing of Chicken Breast Meat as an Innovative Way to Intensify Drying: Use of a Coupled Washing/Diffusion CWD Phenomenological Model to Enhance Kinetics and Functional Properties. Drying Technology 2015, 33, 1369–1381.
- Sandhu, K.; Sharma, L.; Kaurb, M. Effect of Granule Size on Physicochemical, Morphological, Thermal and Pasting Properties of Native and 2-Octenyl-1-Ylsuccinylated Potato Starch Prepared by Dry Heating Under Different pH Conditions. LWT–Food Science and Technology 2015, 61, 224–230.
- Csilla, U.; Juhásza, Z.; Polgárb, Z.; Bánfalvi, Z. GC–MS-Based Metabolic Study on the Tubers of Commercial Potato Cultivars upon Storage. Food Chemistry 2014, 159, 287–292.
- Kim, S.; Lee, M.; Yang, S.; Choi, Y.; Jegal, S.; Sung, C.; Mo, E. Physicochemical Characteristics and Antioxidant Capacity of Rice Cake (Sulgitteok) Supplemented with Lyophilized Sedum Sarmentosum (Dolnamul) Powder. Preventive Nutrition Food Science 2012, 17, 152–157.
- Pedreschi, J.; Domingo, M.; Moyano, P. Development of a Computer Vision System to Measure the Color of Potato Chips. Food Research International 2006, 39, 1092–1098.
- Jiao, A.; Su, X.; Jin, Z. Modelling of Dehydration-Rehydration of Instant Rice in Combined Microwave-Hot Air Drying. Food and Bioproducts Processing 2014, 92, 256–265.
- Opoku, A.; Tabil, L.; Meda, V. Effect of Microwave Drying and Storage on the Color, Breakage, Dehulling and Cooking Quality of Two Red Lentil Varities. CSBE 2009, 8, 134–142.
- Karbassi, A.; Mehdizadeh, Z. Drying Rough Rice in a Fluidized Bed Dryer. Journal of Agricultural Science and Technology 2010, 10, 233–241.
- Chiavaro, E.; Barbanti, D.; Vittadini, E.; Massini, J. The Effect of Different Cooking Methods on the Instrumental Quality of Potatoes (cv. Agata). Journal of Food Engineering 2006, 77, 169–178.
- Sripinyowanich, J.; Noomhorm, A. A New Model and Quality of Unfrozen and Frozen Cooked Rice Dried in a Microwave Vibro-Fluidized Bed Dryer. Drying Technology 2011, 29, 735–748.
- Sripinyowanich, J.; Noomhorm, A. Effects of Freezing Pretreatment, Microwave Assisted by Vibro-Fluidized Bed Drying and Drying Temperature on Instant Rice Production and Quality. Journal of Food Process and Preservation 2013, 37, 314–324.
- Arntfield, S.; Scanlon, M.; Malcolmson, L.; Watts, B.; Ryland, D.; Savoie, V. Effect of Tempering and End Moisture Content on the Quality of Micronized Lentils. Food Research International 1997, 30, 371–380.