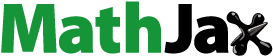
ABSTRACT
An essential component of a three-dimensional air quality model is its gas-phase mechanism. We present an overview of the necessary atmospheric chemistry and a discussion of the types of mechanisms with some specific examples such as the Master Chemical Mechanism, the Carbon Bond, SAPRC and the Regional Atmospheric Chemistry Mechanism (RACM). The first versions of the Carbon Bond and SAPRC mechanisms were developed through a hierarchy of chemical species approach that relied heavily on chemical environmental chamber data. Now a new approach has been proposed where the first step is to develop a highly detailed explicit mechanism such as the Master Chemical Mechanism and the second step is to test the detailed explicit mechanism against laboratory and field data. Finally, the detailed mechanism is condensed for use in a three-dimensional air quality model. Here it is argued that the development of highly detailed explicit mechanisms is very valuable for research, but we suggest that combining the hierarchy of chemical species and the detailed explicit mechanism approaches would be better than either alone.
Implication: Many gas-phase mechanisms are available for urban, regional and global air quality modeling. A “hierarchy of chemical species approach,” relying heavily on smog-chamber data was used for the development of the early series of mechanisms. Now the development of large, explicit master mechanisms that may be condensed is a significant, trend. However, a continuing problem with air quality mechanism development is due to the high complexity of atmospheric chemistry and the current availability of laboratory measurements. This problem requires a balance between completeness and speculation so that models maintain their utility for policymakers.
Introduction
Air quality models are used by governments, regulatory agencies and the private sector to perform simulations to support the development of public policies to improve air quality (Kaduwela et al. Citation2015). The chemical species that are simulated for regulatory purposes include ozone (O3), particulate matter (PM), atmospheric acids, including nitric acid (HNO3) and sulfuric acid (H2SO4), and toxic compounds. A gas-phase chemical mechanism is a required component of an air quality model to simulate the chemical transformation of emissions into their products (e.g. Calvert et al. Citation2015; Reynolds, Seinfeld, and Roth Citation1973; Seinfeld Citation1988). Much of the support to develop high-quality gas-phase mechanisms is derived from these regulatory applications in addition to scientific interest (Kaduwela et al. Citation2015).
Air quality models that are commonly used for the development of public policy include point or box models, Lagrangian models also known as trajectory models, and Eulerian models (Collett and Oduyemi Citation1997). Box models simulate only chemistry at a given point although they may include simple factors such as dilution, varying temperatures or photolysis frequencies (Derwent Citation2017). Lagrangian models simulate chemistry along trajectories (Derwent et al. Citation2010c) that follow wind flow patterns and Eulerian models make their simulations over fixed three-dimensional grids (Byun and Schere Citation2006). Chemical box models and Lagrangian models have important public policy applications that include ozone reactivity calculations and mechanism comparisons (Carter Citation1994; Derwent Citation2017; Derwent et al. Citation2010a). However, in this review, our primary focus is on gas-phase chemical mechanisms for Eulerian air quality models that are used in the Community Multi-scale Air Quality Model (CMAQ) to simulate O3, PM, and atmospheric acids for regulatory purposes (Byun and Schere Citation2006). We acknowledge that the simulation of air toxics is an important subfield although this is beyond the scope of our review. Our focus is on Eulerian air quality models because these models are very widely used to perform simulations to evaluate the effects of emission reduction strategies for the development of public policy especially in the United States (e.g. Astitha et al. Citation2017; Stewart et al. Citation2019).
First, we present a very generalized overview of the atmospheric chemistry required to simulate O3, PM, and atmospheric acids to define the scientific problem to be solved. Second, we provide a presentation of the common types of gas-phase mechanisms. Third, we discuss some of the current gas-phase mechanisms that used for urban, regional and global scale modeling with a focus on those used in Eulerian models. Fourth, we believe that the gas-phase mechanism development strategies used during the 1970s and 1980s and the proposed future strategies are somewhat opposites. The early strategies focused on adding complexity step by step to a gas-phase mechanism followed by environmental chamber testing at each step. Many future strategies focus on developing a detailed explicit mechanism first before testing and condensation are performed. Both the past mechanism development paradigms and the proposed future development strategies are discussed in this review. This review concludes with a perspective on possible future directions and alternative paths for mechanism development. We suggest that mechanism development may benefit from a merger of the past and proposed future strategies.
Overview of tropospheric atmospheric chemistry
Tropospheric ozone is formed by the photolysis of nitrogen dioxide (NO2) to produce a ground state oxygen atom (O(3P)). The O(3P) reacts rapidly with molecular oxygen (O2) to produce ozone.
where: in Reaction 1, the symbol hν represents a photon of solar radiation and in Reaction 2, M represents a third body, such as another oxygen or nitrogen molecule, that is required to carry-away excess collision energy so that an O3 molecule can stabilize. The O3 reacts with nitric oxide (NO) to reproduce NO2 and O2. The rate of Reaction 2 is fast in the troposphere so if the rates of Reactions 1 and 3 are about equal the Ozone Photostationary State Approximation equation results (Calvert and Stockwell Citation1983; Leighton Citation1961):
where ,
and
are the concentrations of O3, NO2 and NO, respectively,
is the photolysis frequency of NO2 and
is the rate coefficient for Reaction 3. Although Equation (4) is not very useful for estimating ozone concentrations, it is a useful heuristic tool for understanding tropospheric ozone formation.
Equation (4) shows that the concentration of O3 depends on the photolysis frequency of NO2 and the ratio of NO2 to NO. The rate of O3 production is directly proportional to the rate of NO2 photolysis which is proportional to and
, Reaction 1. The rate of O3 consumption by Reaction 3 is directly proportional to
and
. Chemical processes that increase the concentration ratio of NO2 to NO produce greater O3 concentrations. The most important reactions that convert NO to NO2 are the reactions of peroxy radicals. These peroxy radicals include hydroperoxy radicals (HO2) and organic peroxy radicals (RO2) (Demerjian, Kerr and Calvert Citation1974; Stockwell et al. Citation2012).
The photolysis of O3 is a major source of hydroxyl radicals (HO). HO reacts with CO, VOC and other species to produce HO2 and/or RO2 radicals that convert NO to NO2. This leads to additional reactions that reproduce HO which makes the overall process of ozone production a chain-mechanism. One of the O3 photolysis reactions produces energetically excited oxygen atoms (O(1D)), Reaction 5.
Most of the O(1D) collide with other molecules (M) in the atmosphere to produce O(3P) that react with molecular oxygen to reproduce O3 but a few percent reacts with water to produce HO radicals.
Other primary sources of HO include the photolysis of formaldehyde, nitrous acid (HONO; Stockwell et al. Citation2012) and during the nighttime, the reaction of O3 with alkenes (Finlayson, Pitts, and Akimoto Citation1972).
The HO radical reacts with many gas-species and in general, its reactions with VOC (RH) produce organic peroxy radicals, RO2 (Atkinson Citation1990, Citation2000; Calvert et al. Citation2015). If the RH is a saturated hydrocarbon HO generally reacts with to abstract a hydrogen atom (Reaction 8) while if the RH contains a double bond then HO may add to the double bond in the RH (not shown), in either case, the reaction product reacts very rapidly to produce an organic peroxy radical. The RO2 reacts with NO to produce carbonyl compounds, species that contain a carbonyl group (C = O). These are represented by CARBONYL in Reaction 9.
CARBONYL includes aldehydes such as formaldehyde (CH2O), acetaldehyde (CH3CHO), ketones such as acetone (CH3COCH3) and their higher carbon number analogs. The reaction of RO2 with NO also produces some organic peroxy radicals through decomposition, as well as organic nitrates. In both cases, the yields are complicated functions of temperature, pressure and the molecular weight of the parent compounds (Atkinson Citation2000).
The HO2 radical produces more conversions of NO to NO2, Reaction 10.
The photolysis of aldehydes produces more peroxy radicals. For example, one of the photolysis reactions of formaldehyde produces HO2 and this can be a major radical source in polluted urban regions (Calvert et al. Citation2015; Calvert and Pitts Citation1966; Gao, Stockwell, and Milford Citation1995; Leighton Citation1961; Stockwell et al. Citation2012).
Another significant reaction involving acetaldehyde and higher molecular aldehydes involves the production of peroxyacetyl nitrate (PAN) and higher molecular weight analogs (PAN; Leighton Citation1961; Seinfeld and Pandis Citation2016). For example, the reaction of HO with acetaldehyde produces the acetyl peroxy radical (CH3CO3).
One of the reactions of CH3CO3 is with NO2 to form PAN. PAN is not a stable species, except at low temperatures, and it is usually in equilibrium with CH3CO3 and NO2.
PAN is a reservoir for acetyl peroxy radicals and NO2 that may reduce O3 formation where it is formed but increase O3 formation downwind. The above scheme focuses primarily on the chemistry of the daytime atmosphere, but significant chemistry occurs during the nighttime (Calvert et al. Citation2015). Other reactions that are important under cleaner conditions include the self and cross reactions of HO2 and RO2 radicals which produce a large number of compounds; some of these are hydroperoxides, other organic peroxides, and organic acids.
VOC compounds have a range of degradation mechanisms that are generally more complicated than the generalized and simplified scheme presented above (e.g. Atkinson Citation2000; Calvert et al. Citation2015). HO radicals abstract hydrogen atoms from alkanes while HO radicals react more rapidly to add to alkenes and aromatic molecules than through abstraction. Although most of these reactions produce RO2 radicals that convert NO to NO2, differences between the VOC degradation mechanisms lead to the production of different amounts of O3. Note that these differences may be represented by the concept of the O3 incremental reactivity (IR) that is defined by Equation (14) (Carter Citation1994).
where Δ[O3] is the change in the O3 mass concentration affected by an incremental change in the mass concentration of a VOC, Δ[VOC]. An alternative to incremental reactivity is the photochemical ozone creation potential (POCP; Derwent, Jenkin, and Saunders Citation1996). POCP is defined as an organic compound’s contribution to ozone formation relative to ethene as calculated from multi-day, 1-dimensional trajectory model simulations, Equation (15).
First, a base-case simulation is made. Second, another simulation is made with a small mass increment in ethene emissions. Then, to calculate POCPs, simulations are made with a small mass increment to the emissions for each VOC compound and Equation (15) is applied.
A gas-phase chemical mechanism must account for reactivity differences if it is to estimate the effects of VOC emissions on O3 concentrations and simulate its formation accurately. Mechanisms for incremental reactivity or POCP calculations may require more detail than those used for Eulerian air quality modeling in order to account for an organic compound’s degradation mechanism and these simulations are usually made with box or 1-dimensional trajectory models (Carter Citation1994; Derwent et al. Citation2007; Derwent, Jenkin, and Saunders Citation1996; Derwent et al. Citation2001).
Gas-phase chemistry generates inorganic and organic precursors with low-vapor pressures that condense to form particulate matter (PM; Calvert et al. Citation2015; Haagen-Smit Citation1952; Jang and Kamens Citation2001; Tobias and Ziemann Citation2000). The major inorganic precursors are sulfuric acid (H2SO4=) and nitric acid (HNO3). Sulfuric acid is produced by the oxidation of sulfur dioxide (SO2) by HO, Reactions 16– 18.
Reaction 17 produces HO2 radicals that may react with NO to produce more HO radicals (Stockwell and Calvert Citation1983a). H2SO4(aq) has a very low vapor pressure and is hygroscopic, therefore it condenses to an aerosol on a very rapid time scale. Solid sulfate aerosol is produced when H2SO4(aq) reacts with NH3 through reversible reactions to produce solid ammonium bisulfate (NH3HSO4(s)) and ammonium sulfate ((NH3)2SO4(s)) (Seinfeld and Pandis Citation2016).
The major source of tropospheric HNO3 is the reaction of HO radical with NO2, Reaction 21 (Seinfeld and Pandis Citation2016).
Reaction 21 is also an important termination reaction in the chain mechanism that produces O3. Other sources of HNO3 include nighttime reactions of the nitrate radical (NO3 and N2O5; Calvert et al. Citation2015; Stockwell and Calvert Citation1983b).
Similar to H2SO4(aq), HNO3 reacts reversibly with ammonia (NH3) to produce gas-phase ammonium nitrate (NH4NO3(g)).
A fraction of the NH4NO3(g) may condense into the solid phase, NH4NO3(s) as shown by Reaction 23,
The fraction of NH4NO3 found in the solid and gas-phases phase depends on the temperature, relative humidity and ambient sulfate concentrations (Kim and Seinfeld Citation1995; Kim, Seinfeld, and Saxena Citation1993a, Citation1993b).
A gas-phase mechanism for an air quality model needs to include sufficient VOC oxidation chemistry to describe the formation of secondary organic aerosol (SOA). Many organic compounds with carbon numbers of five or higher react with HO and, with O3 if they are alkenes, to produce high molecular weight oxygenated products with sufficiently low vapor pressures to condense to form particulate matter. For example, isoprene (C5H8) is a common, biogenically emitted hydrocarbon that is a major contributor to global SOA production (Guenther et al. Citation2006; Lin et al. Citation2012). Isoprene chemistry has been reviewed by Carlton, Wiedinmyer, and Kroll (Citation2009) and by Bates and Jacob (Citation2019). Its oxidation products include compounds such as isoprene β-epoxydiol that form SOA, (Lin et al. Citation2012). Note that isoprene β-epoxydiol is an epoxide with three oxygen atoms.
Figure 1. Isoprene and one of its highly oxidized products, isoprene β-epoxydiol, that forms particulate matter in the atmosphere. The white spheres are hydrogen atoms, the black spheres are carbon atoms and the red spheres are oxygen atoms.
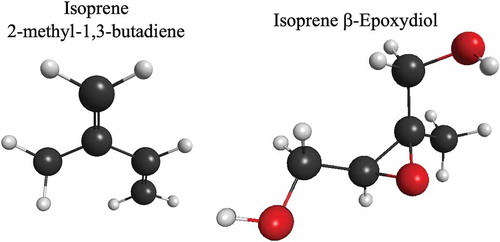
The oxidation process of high molecular weight VOC may include many oxidation cycles that add oxygen, nitrogen, and other atoms to form low volatility organic molecules. In addition, chain-terminating reactions between high molecular weight organic peroxy-radicals can result in highly oxygenated, high molecular weight compounds. These processes produce highly oxygenated high molecular weight compounds with a lower vapor pressure that may be oxidized further until they condense to form SOA (Calvert et al. Citation2015). Therefore, a mechanism needs to include the chemistry of high molecular weight VOC with carbon atoms up to C25 and low vapor pressure products (Lee-Taylor et al. Citation2011). The formation of SOA is much more complicated than O3 because it is more strongly affected by the range of physical and chemical properties of organic compounds than O3 (Johnson et al. Citation2004; Zhang et al., Citation2007). The organic chemistry of compounds with five or more than carbon atoms and the dependence of SOA on NOx concentrations are continuing important topics of research (Calvert et al. Citation2015; Hallquist et al. Citation2009; Hodzic et al. Citation2010a, Citation2010b; Lee-Taylor et al. Citation2011; Virtanen et al. Citation2010; Volkamer et al. Citation2006; Zhang et al. Citation2013).
Types of gas-phase chemical mechanisms
Explicit mechanisms
Most chemists think in terms of explicit chemical mechanisms. Explicit mechanisms are composed of specific reactions for individual chemical compounds (Calvert et al. Citation2015; Stockwell et al. Citation2012). There are fewer inorganic species and reactions in the lower troposphere that need to be represented in an air quality mechanism compared with atmospheric organic chemistry. Therefore, it has long been possible to include explicit schemes for ozone (O3), nitric oxide (NO), nitrogen dioxide (NO2), ammonia (NH3), hydroxyl radical (HO), hydroperoxyl radical (HO2), sulfur dioxide (SO2) and related species in most chemical mechanisms for air quality. An explicit inorganic mechanism component of an air quality mechanism does not add a serious computational burden to the overall chemical mechanism because usually the inorganic chemistry is expressed in terms of fewer than 20 chemical species with fewer than 50 reactions (Calvert et al. Citation2015; Stockwell et al. Citation2012).
Atmospheric chemistry is complicated due to the emissions of many thousands of Volatile Organic Compounds (VOC) in the atmosphere (Bowman and Seinfeld Citation1994; Calvert et al. Citation2015; Seinfeld and Pandis Citation2016; Warneck Citation2000). There are thousands of both natural (e.g. Fuentes et al. Citation2000; Yee et al. Citation2018) and anthropogenic (e.g. Atkinson Citation1990, Citation2000; Murrells et al. Citation2010; Pétron et al. Citation2012; Simon et al. Citation2010) compounds emitted and much of the gas-phase chemistry of these compounds remain uncertain or unknown. Extending the explicit approach to the thousands of emitted VOC compounds would result in an explicit tropospheric mechanism that would contain millions of reactions (Szopa, Aumont, and Madronich Citation2005a).
The level of detail and computational efficiency are two competing constraints on developers of mechanisms for Eulerian air quality modeling. The mechanism must represent the most significant aspects of the chemical system to be simulated but it should be computationally efficient too and this implies that the mechanism needs to be condensed in size (Calvert et al. Citation2015). Three-dimensional air quality models such as CMAQ (Byun and Schere Citation2006) make calculations for modeling domains that may consist of 105 or more grid-boxes. Prognostic species include those chemical species with lifetimes long enough to be transported through the atmosphere. The concentrations of prognostic species are calculated explicitly and due to this each prognostic species adds one storage location and one differential equation that must be solved for every grid-box.
Each prognostic species increases computational demands to complete transport calculations as well (Jacobson Citation1999). Overall, there is a demand for balance in the model between the need for detailed chemistry and its meteorological components. The practical size of a gas-phase mechanism should be a function of available computational resources and the efficiency of numerical methods. Available computer power has followed Moore’s law (Moore Citation1965) which projects that power should double about every 1.5 years and this has been true of grid-based models as well (Voller and Porté-Agel Citation2002). The increase in computer power should allow for larger chemical mechanisms to be used in Eulerian models. However, air quality modelers have often favored improving a model’s spatial resolution and to make other revisions to meteorological aspects of the model rather than increase the size of the chemical mechanism. A practical-condensed mechanism for air quality modeling consists of a few hundred chemical species and reactions (Makar, Stockwell, and Li Citation1996).
Although explicit mechanisms can be used in three-dimensional Eulerian air quality models for research, they are not very practical to use in the models for public policy development because comparatively large computational resources are required that may not be available to most state and local agencies. However, explicit mechanisms have very important applications in zero-dimensional box models and trajectory models, especially for estimating incremental reactivity and photochemical ozone creation potentials.
Surrogate chemical mechanisms
A surrogate mechanism represents all atmospheric organic chemistry with explicit chemical mechanisms for a very few selected species (Dimitriades and Dodge Citation1983). The Dimitriades and Dodge surrogate mechanism made large numbers of simulations practical with the computers available during the 1980s. Dimitriades and Dodge choose to use butane and propene as their surrogates. The pairing of a lower-reactivity alkane represented by the explicit chemistry of butane and a greater-reactivity alkene, represented by the explicit chemistry of propene, allowed the mechanism to represent a wide range of atmospheric VOC reactivities.
Aggregated condensed chemical mechanisms
Typically, mechanisms are condensed through the aggregation of VOC and therefore the major difference between mechanisms is in the applied method of aggregation. VOC aggregation uses generalized model species to represent groups or classes of organic compounds with similar chemical structure and reactions. There are two approaches that are commonly used: one is aggregation by chemical moiety and the other is aggregation by molecule.
The aggregation by chemical moiety for VOC was developed by Whitten and Hogo (Citation1999) and Whitten, Hogo, and Killus (Citation1980a). The aggregation by chemical moiety approach, also known as the Carbon Bond approach, uses model species to represent the concentrations of molecular groups (chemical moieties). There is much less weight given to the specific molecule to which the moieties are attached than used in the molecule aggregation approach. For example, a mechanism using the chemical moiety approach might include PAR (alkane carbon atoms), OLE (double-bonded carbon atoms), ARO (aromatic rings) and CAR (carbonyl groups). In that case, 1 ppb (by volume) of propane (CH3CH2CH3) would be represented by 3 ppb of PAR, 1 ppb of propene (CH2=CHCH3) would be represented by 1 ppb of OLE and 1 ppb of PAR; and 1 ppb of toluene (CH3C6H5) would be represented by 1 ppb of PAR and 1 ppb of ARO. This approach provides three major advantages: (1) it was relatively easier to aggregate speciated emissions into model species compared with the molecule aggregation approach, (2) fewer model species than were required than compared with the aggregation by molecule approach making the mechanism smaller than others and (3) the number of carbon atoms in the simulated atmosphere could be conserved.
The aggregation by molecule approach groups similar chemical compounds into model species. For example, the mechanism could use a model species such as “BUTA” to represent ethane, propane, and isomers of butane while “ALD” might represent all aldehydes higher than formaldehyde. Some mechanisms employ weighting factors to adjust for differences in chemical reactivity or carbon mass between the compounds being aggregated (e.g. Stockwell et al. Citation1997). Often the mechanisms used in Eulerian three-dimensional air quality models have explicit, surrogate and aggregated elements.
Selected gas-phase chemical mechanisms for urban and regional air quality modeling
lists several of the most widely used gas-phase mechanisms for air quality modeling. In this section, we focus on selected tropospheric gas-phase mechanisms that are used for urban and regional air quality modeling. In the next section, we present selected gas-phase mechanisms that are used for hemispheric and global scale atmospheric chemistry models because these models may be used to prepare chemical boundary conditions for regional air quality modeling.
Table 1. Selected gas-phase chemical mechanisms used in air quality models. The symbol + indicates that the information was not apparent from the mechanism description (following the format of Baklanov et al. Citation2014).
The choice of gas-phase mechanism to be used in an air quality model will affect predicted concentrations and therefore the decision must include a consideration of a mechanism’s strengths and weakness for the purpose of the simulations. For example, Knote et al. (Citation2015) used a box model to intercompare seven gas-phase mechanisms that are commonly used in 3-deminsional air quality models including versions of the Carbon Bond Mechanism, version 5 (CB05; Yarwood et al. Citation2005), versions of the Regional Acid Deposition Model (RADM; Stockwell et al. Citation1990) and Regional Atmospheric Chemistry Mechanism (RACM, Stockwell et al. Citation1997) series and the Model for Ozone and Related chemical Tracers (MOZART; Emmons et al. Citation2010). This comparison found differences in simulated concentrations for O3 (5%) HO2 (20%) HO (40%), NOx (25%) PAN (50%) and CH2O (20%) among the mechanisms included in their study. The greatest differences were over 100% and these large differences were found for biogenically emitted VOCs such as isoprene and for chemical species that are very important for simulating nighttime chemistry such as NO3 and N2O5. Clearly, this study illustrates the significance of the mechanism choice for air quality modeling.
The master chemical mechanism (MCM) and mechanisms derived from it
The Master Chemical Mechanism (Jenkin, Saunders, and Pilling Citation1997; MCM v3.2 Citation2019; Saunders et al. Citation2003) is an explicit mechanism that was first developed in the United Kingdom (UK). It has been a focus for research and application in Europe for over 20 years and it was cited within the EUROTRAC project (Ebel, Friedrich, and Rodhe Citation1997; Midgley and Reuther Citation2003). Initially, it was applied in an ongoing application to simulating photochemical ozone creation potential (POCP) calculations (e.g. Derwent et al. Citation2007, Citation2001) and more recently to reactivity calculation to PM (Derwent et al. Citation2010b). Although the MCM is considered to be an explicit mechanism it illustrates that most gas-phase mechanisms are not perfectly explicit or perfectly aggregated. Examination of the MCM shows that almost all organic peroxy-radicals reactions are characterized very similarly by the same rate constants and with analogous products.
The Master Chemical Mechanism (MCM; Bloss et al. Citation2005a, Citation2005b; Jenkin, Saunders, and Pilling Citation1997; Jenkin et al. Citation2003, Citation2008, Citation2012; Jenkin, Young, and Rickard Citation2015; Saunders et al. Citation2003) that represents the chemistry of 143 emitted organic compounds (including methane) and with over 2400 species and 7100 reactions (MCM v3.2 Citation2019). The explicit MCM is too large for many Eulerian air quality models so a number of condensed versions have been based on MCM and these are the Common Representative Intermediates (CRI) mechanisms (Jenkin et al. Citation2008; Watson et al. Citation2008). CRI mechanisms have been implemented in the CMAQ, WRF-CHEM, and EMEP 3-D air quality policy models. McFiggans et al. (Citation2019) used the EMEP 3-D model to find that the CRI mechanism provides improved PM simulations.
The Dimitriades and Dodge (Citation1983) surrogate mechanism
The Dimitriades and Dodge (Citation1983) mechanism was not widely used in three-dimensional air quality models; rather it was used in box models for most applications. Emissions inventories were used to estimate representative mole fractions of butane and propene for the site to be modeled. Simulations were made with varied initial concentrations and emissions rates of NOx and VOC to generate contour diagrams of maximum O3 concentrations as functions of NOx and VOC. These plots were known as Empirical Kinetics Modeling Approach (EKMA) or ozone isopleths even if mechanisms other than the Dimitriades and Dodge are used to make the simulations. EKMA plots can be used to estimate the relative effectiveness of NOx control strategies versus VOC control strategies for reducing O3 concentrations.
The carbon bond mechanism series
The earliest versions of the Carbon Bond mechanism were based on the aggregation by chemical moiety approach (Whitten, Hogo, and Killus Citation1980a). It was designed originally for the modeling of urban areas with high NOx concentrations. Environmental chamber testing was integral to its development (Whitten, Killus, and Johnson Citation1985). The Carbon Bond mechanism, version four (CB4; Gery et al. Citation1989) was very widely used in 3-deminsional air quality models. The generally smaller size of the Carbon Bond mechanisms compared with other mechanisms resulted in three-dimensional air quality simulations that required less computer time. This and the relative simplicity of preparation of emissions inventories made the Carbon Bond mechanism very popular among air quality modelers. However, successive versions of these mechanisms have incorporated new species and reactions that are more like those found in mechanisms that employ the aggregation by molecule approach. These modifications have improved simulation performance and this is consistent with known atmospheric chemistry because product species and the rates of chemical reactions depend on overall molecular structure (the constituent moieties of a molecule affect its reactivity) along with a compound’s total molecular weight, especially for secondary aerosol formation (de Gouw and Jimenez Citation2009; Donahue et al. Citation2006; Lee-Taylor et al. Citation2011; Yarwood et al. Citation2005; Zhang et al., Citation2007).
The most up-to-date versions of the Carbon Bond series are Carbon Bond, version 5 (CB05; Yarwood et al. Citation2005) and Carbon Bond, version 6 (CB6, Yarwood et al. Citation2010). CB05 was developed along a path that was similar to SAPRC in that CB4 was modified so that the new version could be used to simulate regional, lower NOx conditions, as well as highly polluted urban sites (Yarwood et al. Citation2005). More explicit chemistry was added to CB05, for example, it explicitly treats methane, ethane chemistry and the methylperoxy radical rather than by the original Carbon Bond approach. CB05 treats alkenes with internal double bonds (R-HC=CH-R) differently than those with primary double bonds (H2C=CH-R) and this is another deviation from the original Carbon Bond approach. Other species that are treated more explicitly in CB05 include aggregated species for terpene and similar biogenically emitted compounds, higher organic peroxides, formic acid, and organic acids, acetaldehyde, an aggregated species for higher molecular weight aldehyde, PAN and a higher molecular weight analog (PANX). The revised CB05 mechanism consists of 51 model species with 156 reactions. In addition to these updates, Yarwood et al. (Citation2005) provided two extensions. One extension is a set of reactions that characterize reactive chlorine chemistry that may be important to simulate air near oceanic coasts. The second CB05 extension includes explicit reactions for air-toxics and Secondary Organic Aerosol (SOA) precursors.
The development of CB6 from CB05 involved adding explicit chemistry for propane, benzene, acetone, other ketones, acetylene, benzene, glyoxal, glycolaldehyde, and methylglyoxal (Yarwood et al. Citation2010). The revised chemistry for propane, benzene, acetone, and other ketones improved the performance of CB6 in simulating O3 while the addition of acetylene, benzene, glyoxal, glycolaldehyde and methylglyoxal improved its performance in simulating SOA precursors.
A number of other mechanism developers have produced modified versions of the Carbon Bond mechanism. Zaveri and Peters (Citation1999) developed one of the first versions of the Carbon Bond mechanism designed for regional air quality modeling called CBM–Z. Kim et al. (Citation2011) successfully modified Carbon Bond to improve its performance in simulating secondary organic aerosol formation.
Statewide air pollution research center (SAPRC) mechanisms
The SAPRC mechanism series includes SAPRC-90, SAPRC-99, SAPRC-07, SAPRC-11 and SAPRC-16 air quality mechanisms (Carter Citation1990, Citation2000, Citation2010a, Citation2010b; Carter and Heo Citation2013; Carter Citation2016). The SAPRC series of chemical mechanisms was one of the earlier air quality mechanisms along with Carbon Bond. It was originally developed with close integration of mechanism development with testing and fitting of data from environmental chambers (e.g., Carter Citation1995; Carter and Lurmann Citation1991).
Like the Carbon Bond mechanism, early versions of SAPRC were specifically designed for the modeling of highly polluted urban areas with high NOx concentrations (e.g. Carter Citation1990). For example, the reaction of a VOC with HO, in general, produces organic peroxy radicals. To reduce the complexity of the mechanism, rather than treat many organic peroxy radicals in the mechanism, early SAPRC developers included the reaction products of subsequent peroxy organic radical reactions with NO as products of the primary HO – VOC reaction. To account for the conversion of NO to NO2 an operator radical was produced that reacted with NO and other mechanism species. This simplification made it difficult to accurately simulate the VOC products under low NOx conditions but in a very polluted urban area the error was probably not too great.
However, the spatial domain simulated by air quality models usually includes the regional scale where NO concentrations are lower than in the urban core and therefore later versions of SAPRC were revised to better simulate regional atmospheric chemistry. For example, SAPRC07 and more recent versions represent peroxy-peroxy reactions more explicitly and these modifications allow the effects of NOx on the formation of organic products to be simulated more accurately (Cai et al. Citation2011). Another important effort has been to develop better representations of aromatic chemistry in SAPRC with revisions included in SAPRC07, SAPRC-11, and SAPRC16.
Improvements and increased complexity of mechanisms will change the simulated O3 and its production efficiency in three-dimensional air quality models. Versions of SAPRC have been implemented in CMAQ and compared (Cai et al. Citation2011). The comparisons of Cai et al. showed that SAPRC07 yielded generally lower simulations of O3 than SAPRC99. Cai et al. defined ozone production efficiency (OPE) as the slope of the regression line between O3 and NOz (the sum of all of the oxidation products of NOx) between the hours of 10:00 a.m. to 5:00 p.m. at specified sites. The ozone production efficiency of SAPRC07 was found to be lower than SAPRC99 by up to about 22%.
The SAPRC series includes versions with an adjustable number of organic species and generalized kinetic and mechanistic parameters that can be modified as appropriate for a particular mix of pollutants. The larger SAPRC versions may be run, usually in single box models, with very explicit representation of the organic chemistry of over 1000 organic compounds. Most versions of SAPRC that are used in Eulerian three-dimensional air quality models have representations of VOC that are more condensed (Carter Citation2016; Carter 2018; Venecek et al. Citation2018).
The regional acid deposition model (RADM) and regional atmospheric chemistry mechanism (RACM) series
This series of gas-phase chemical mechanisms uses an aggregation by molecule approach for VOC that is similar to SAPRC. The RADM/RACM series is designed: (1) to be valid for both high and low NOx conditions; (2) to simulate the production of peroxides that result from peroxy radical – peroxy radical reactions at lower NOx concentrations found aloft and on the regional scale; (3) to simulate the production of acids and (4) to simulate the oxidation of less reactive organic compounds that may occur over long range transport. The simulation of peroxy radical – peroxy radical reactions was particularly important for the Regional Acid Deposition Mechanism, version 1 (RADM1; Stockwell Citation1986) and the Regional Acid Deposition Mechanism, version 2 (RADM2; Middleton, Stockwell, and Carter Citation1990; Stockwell et al. Citation1990) because of the necessity to simulate the production of hydrogen peroxide (H2O2), methyl hydroperoxide (CH3OOH) and other organic peroxides. H2O2 and organic peroxides oxidize sulfur dioxide to sulfate very rapidly in the aqueous phase (Lind, Lazrus, and Kok Citation1987). Peroxy radical-peroxy radical reactions also have some influence on simulated O3 concentrations (Stockwell et al. Citation1990). For these reasons, the RADM/RACM series includes an explicit, reduced set of peroxy radical – peroxy radical reactions which include reactions of HO2, methyl peroxy radicals and acetyl peroxy radicals with all other organic peroxy radicals in the mechanisms. Sensitivity tests showed that other cross-reactions of organic peroxy radicals, such as the self-reaction of the ethyl peroxy radical, have only a small effect on O3, H2O2, and organic peroxide concentrations.
RADM2 was used in the United States, Europe and in Asia and the series was updated to include the Euro-RADM Mechanism (Stockwell and Kley Citation1994), the Regional Atmospheric Chemistry Mechanisms, version 1 (RACM1; Stockwell et al. Citation1997) and the Regional Atmospheric Chemistry Mechanisms, version 2 (RACM2; Goliff, Stockwell, and Lawson Citation2013). The RACM series development was international in that RACM1 was developed in Germany as part of the Förderschwerpunkt Troposphärenforschung (TFS) and the EUROTRAC projects (Ebel, Friedrich, and Rodhe Citation1997; Geiger et al. Citation2002; Midgley and Reuther Citation2003) while RACM2 was developed with American support from the U.S. EPA and NASA.
Revision of the RADM/RACM series has followed a path that is similar to SAPRC and Carbon Bond. Over the years there has been a significant improvement in the understanding of the chemistry of alkanes, alkenes (including isoprene and other biogenically emitted compounds) and aromatic compounds. Nighttime chemistry has been recognized as important and therefore processes such as the production of HO from the ozonolysis of alkenes and reactions of NO3 with organic-peroxy radicals and NO3 with alkenes have been added to mechanisms. Mechanism developers have included more detailed chemistry as it has become known and computational resources increase. In RACM2 acetaldehyde and acetone are now treated as explicit species while generalized aldehydes and ketones represent classes of higher analogs and in RACM methanol and ethanol are treated more explicitly. RACM2 is the current version of this series and it consists of 118 model chemical species that react in a total of 356 reactions. The computational requirements of CMAQ with RACM2 are acceptable for routine simulations (Sarwar et al. Citation2013).
Simulations made with these mechanisms have been compared with environmental chamber experiments and field observations (e.g. Goliff, Stockwell, and Lawson Citation2013; Sarwar et al. Citation2013). Chamber experiments are simulated better by RACM2 compared with RACM1 (Goliff, Stockwell, and Lawson Citation2013). CMAQ simulations with RACM2 and CB05 were compared (Sarwar et al. Citation2013). The simulated CMAQ-RACM2 O3 concentrations were higher than CMAQ-CB05 at all levels. RACM2 provided improved performance at higher measured O3 concentrations while producing overestimates at low-measured O3 concentrations compared with CB05. RACM2 simulations predicted higher concentrations of inorganic and organic aerosols that agreed better with observed data than CB05. However, the differences may not greatly affect air quality abatement strategies derived from CMAQ with the three different mechanisms.
Selected gas-phase chemical mechanisms for global atmospheric chemistry modeling
Global atmospheric chemistry models are becoming increasingly important as sources of boundary conditions for air quality models (Henderson et al. Citation2014; Saunders Citation2017). The mechanisms employed in global models require different chemical mechanisms than mechanisms prepared for regional models. Global models require chemical mechanisms that are valid over oceans as well as over land masses. Oceans are significant sources of halogens and reduced sulfur compounds that have complex chemistries (Warneck Citation2000). However, there are differences in the VOC emissions since anthropogenic emissions are not as prevalent over the ocean although polluted plumes from continental sources can cover significant areas of the oceans (Lelieveld et al. Citation2001). Global atmospheric chemistry models may include a significant amount of stratospheric chemistry. In this section, we discuss the GEOS-Chem Mechanism, the Model for Ozone and Related chemical Tracers (MOZART) mechanism and the Global Atmospheric Chemistry Mechanism (GACM). GACM is part of the RADM/RACM mechanism series.
GEOS-chem mechanism
Early versions of the GEOS-Chem included inorganic chemistry to simulate H2O2 and other products that are produced at low NOx concentrations. It also included inorganic species for NOx, HNO3, three peroxyacyl nitrates, and two different organic nitrate compounds were included because of their importance in simulating O3 formation and the long-range transport of nitrogen oxides (Horowitz et al. Citation1998). GEOS-Chem treated less reactive alkanes, methane, ethane, and propane while extensive use was made of surrogate species to represent other alkane chemistry (Horowitz et al. Citation1998). Propene represented all alkenes with three or more carbon atoms while butane was used to represent all VOCs with four or more carbon atoms. VOCs were summed into butane or propene according to class and the number of carbon atoms of the aggregated VOC species. Many of VOCs emitted in highly polluted urban areas, such as aromatic hydrocarbons, were ignored because the mechanism developers’ focus was on the simulation of global ozone concentrations. The version of GEOS-Chem developed by Horowitz et al. (Citation1998) includes isoprene but not terpenes to represent biologically emitted compounds. The isoprene scheme is rather extensive. There are some emissions of aldehydes and ketones which are also oxidation products of hydrocarbons. These compounds are represented in the GEOS-Chem mechanism by formaldehyde, acetaldehyde, methacrolein, higher aldehydes (RCHO), acetone, methyl vinyl ketone and higher ketones (MEK). GEOS-Chem includes an extensive scheme for the reactions of HO2 and organic peroxy radicals which is necessary for modeling low NOx conditions.
Further updates to the GEOS-Chem mechanism have been made by (Bates and Jacob Citation2019; Bey et al. Citation2001). Now there are many current versions that may be found at the GEOS-Chem website (GEOS-Chem Citation2019). Currently available mechanisms include chemistry for the troposphere, coupled tropospheric and stratosphere mechanisms, a mechanism for SOA formation, mercury and perhaps unexpectedly, radionuclides.
The model for ozone and related chemical tracers (MOZART) mechanism
The Model for Ozone and Related chemical Tracers (MOZART) is a global chemical transport model that can be used to prepare lateral chemical boundary conditions for regional air quality models such as WRF-Chem. The gas-phase chemical mechanism in MOZART is relatively comprehensive and covers both the stratosphere and troposphere (Emmons et al. Citation2010). It includes explicit chemical reactions for ethane, propane, formaldehyde, acetaldehyde, acetone, methanol, and isoprene. The MOZART species BIGALK represents alkanes that have four or more carbon atoms and BIGENE represents alkenes with four or more carbon atoms. BIGALK and BIGENE may represent other classes of higher-carbon VOCs with similar reactivity. Benzene, toluene, and xylenes are represented by the species TOLUENE. The standard version of MOZART includes 85 gas-phase species, 39 photolytic reactions, and 157 gas-phase reactions.
The global atmospheric chemistry mechanism (GACM)
The GEOS-Chem and MOZART chemical mechanisms have been used in global atmospheric chemistry mechanisms to supply lateral chemical boundary conditions to regional air quality models, but they were not designed for this purpose. Inconsistencies between global atmospheric chemistry mechanisms and those used for regional modeling may require considerable effort to map the global model’s chemical species into the regional model’s species. The Regional Atmospheric Chemistry Mechanism, Version 2 (RACM2) is an option in CMAQ and the Weather Research and Forecasting model coupled with Chemistry (WRF-Chem). The goal for the development of the Global Atmospheric Chemistry Mechanism (GACM) was to have gas-phase chemistry that is consistent with RACM2 (Saunders Citation2017). GACM includes additional chemistry for marine environments, including chlorine and reduced sulfur compounds, while reducing its treatment of the VOC chemistry needed for highly polluted urban regions. This kept GACM’s size small enough to allow it to be used efficiently in global models. GACM’s chemistry of volatile organic compounds (VOC) is highly compatible with the VOC chemistry in RACM2 allowing a global model with GACM to provide VOC boundary conditions to a regional scale model with RACM2 with reduced error.
A GACM-RACM2 system of mechanisms may yield more accurate forecasts by regional air quality models such as WRF-Chem because if the global and regional air quality models use a highly compatible set of chemical mechanisms, uncertainty in the translation of chemical species between the two models is reduced (Saunders Citation2017). The compatibility of the GACM and RACM2 mechanisms has been tested with WRF-Chem and they were shown to yield similar concentrations along the California Coast.
Methods initially used for the development of gas-phase chemical mechanisms for air quality
There are few discussions of the history of gas-phase air quality mechanism development (e.g. Heymann Citation2010). Initially, many mechanism developers used a hierarchy of chemical species approach (Demerjian et al., Citation1974; Whitten, Killus, and Hogo Citation1980b). We characterize the hierarchy of chemical species approach as “bottom up” in that a very simple core mechanism is developed and tested. Following the development of the initial simple core, new species and reactions are added followed by additional testing at each step. The bottom-up approach alone was not perfect, and errors could be introduced through an overreliance on testing as will be shown.
In this section, we present the hierarchy of chemical species approach and other methods for developing gas-phase air quality mechanisms that did not involve the development of very large explicit mechanisms. Recently, mechanism developers have placed greater and greater reliance on approaches that we characterize as “top down”. Top-down approaches proceed from the development of very large explicit mechanisms that are condensed to create mechanisms for a particular purpose (e.g. Kaduwela et al. Citation2015). In the next section, we discuss the top-down approach and we will assert our belief that future mechanism development will be best served with a mixture of the bottom up and top-down approaches.
Two early attempts to produce comprehensive atmospheric chemistry mechanisms were published by Niki, Daby, and Weinstock (Citation1972) and Demerjian et al. (Citation1974). The Demerjian et al. study was particularly noteworthy in that it provided computer simulations where chemistry was added step by step so that the importance of various types of reactions and species could be estimated. Protocols were established during the early years to help ensure that air quality mechanisms included sufficient chemistry that was accurate enough to allow the mechanism to simulate environmental chamber and field measurements. Also, due to relatively limited knowledge, there was a concern that mechanisms might include compensating errors.
shows a scheme for developing gas-phase chemical mechanisms for air quality modeling that was state-of-the-science from the middle 1970s through the early 2000s. The available data originated from laboratory experiments, environmental chemical reaction chambers (previously known as “smog chambers”) and field measurements. However, during the relatively early days of gas-phase chemical mechanism development, there were large gaps in atmospheric chemistry knowledge. Key sources of laboratory measurements of gas-phase chemical reactions, their rate coefficients and product yields during the early period included (Atkinson Citation1990, Citation1994; Atkinson et al. Citation1997, Citation1992; Atkinson and Carter Citation1984; Baulch et al. Citation1982, Citation1980; Wilson Citation1972) and more recently, Atkinson et al., (Citation2006). Many of these provided datasheets that included many rate constant measurements for each reaction listed and the mechanism developer might have to further evaluate the measurements to choose the most reliable data. For example, the accepted value of the rate constant for the reaction of HO with CO varied by a factor of 1000 between the years 1962 and 1972 (Wilson Citation1972). This uncertainty in the available data required that chemical mechanisms be evaluated extensively with environmental chamber experiments.
Figure 2. Flowchart of an early scheme for developing gas-phase chemical mechanisms for air quality modeling that was state-of-the-science from the middle 1970s through the early 2000s Whitten et al. (Citation1980b).
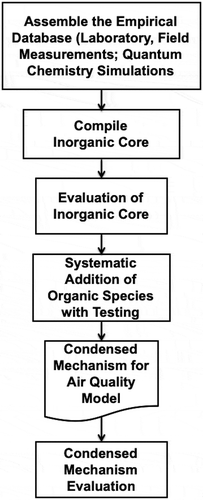
Data from environmental chambers were an integral component of chemical mechanism development during the first few decades of atmospheric chemistry (Whitten, Hogo, and Killus Citation1980a, Citation1980b; Citation1985). and show two different examples of approaches to environmental chemical reaction chambers. shows an indoor chamber with artificial irradiation lamps. Gaseous reaction mixtures are introduced into the chamber, irradiated and changes in chemical composition are monitored by gas-chromatography, mass-spectrometry, spectroscopy, and other instrumentation. However, usually the artificial irradiation did not provide actinic fluxes that well matched the real solar actinic fluxes and it was feared that this could introduce bias into the photochemical measurements. shows an outdoor chamber at the University of North Carolina (UNC chamber). Outdoor chambers use natural solar irradiation but there can be high levels of uncertainty introduced due to difficulties in the measurement of the natural solar flux and its response to solar zenith angle, haze, clouds, plastic exterior coverings, etc. (Finlayson-Pitts and Pitts Citation1986, Citation2000; Jeffries, Fox, and Kamens Citation1976; Jeffries, Kamens, and Sexton Citation2013). Dodge (Citation2000) provides a comprehensive guide to environmental chambers and they are used in comparing chemical mechanisms. A very recent review of environmental chambers is found in Hidy (Citation2019).
Figure 3. An indoor chamber with artificial irradiation lamps. The left panel shows the irradiation lamps surrounding the Pyrex glass chamber. Gas concentrations were monitored by Fourier transform infrared spectroscopy (contained in box to the extreme left of the panel). The author (WRS) is assembling the chamber at the national center for atmospheric research. The right panel shows the chamber illuminated by the irradiation lamps.
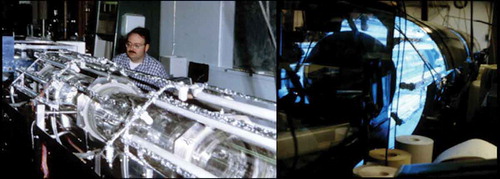
Figure 4. An outdoor chamber located at a field site at the University of North Carolina. Note that it has a somewhat similar design to an A-frame house. A history of this chamber is given by Jeffries, Kamens, and Sexton (Citation2013).
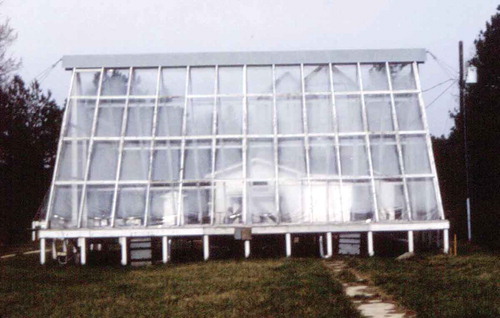
Developers producing mechanisms under the concept of a hierarchy of chemical species approach (Whitten, Hogo, and Killus Citation1980a) made environmental chamber testing an integral part of mechanism development. Every additional level of detail in a chemical mechanism was evaluated as it was added to the mechanism to reduce the strong possibility of compensating errors between levels in new mechanisms, . The hierarchy levels followed those of Demerjian et al. (Citation1974) where the lowest hierarchy level of species included ozone, nitrogen dioxide, nitric oxide, carbon monoxide and other inorganic compounds that were necessary to describe the chemistry of almost all tropospheric conditions. This was very reasonable considering the uncertainties in the most basic inorganic reactions at that time. An explicit inorganic mechanism was created based on laboratory experiments, environmental chamber experiments, theoretical estimates and atmospheric observations, . Chamber experiments containing inorganic mixtures of nitric oxide and nitrogen dioxide were performed. Computer simulations were made with the inorganic mechanism and compared with the data from the experiments. If the simulations did not reproduce the chamber measurements well then additional sensitivity simulations could be made to determine what modifications to make to the mechanism to improve its performance, . Additional cycles of simulation, performance evaluation, and modification were made until the performance was judged to be satisfactory (Whitten, Hogo, and Killus Citation1980a, Citation1980b). It should be noted that chamber wall effects on the chemistry need to be included in the simulations and these effects can introduce uncertainties into the mechanism development and evaluation process (Besemer and Nieboer Citation1985; Carter et al. Citation1981).
Figure 5. Flowchart of a chemical mechanism development following a hierarchy of chemical species scheme following the discussion found in Whitten et al. (Citation1980b). Part A shows an overview of the complete development and testing process. Part B shows the detail of the development and addition of species and reactions by hierarchy.
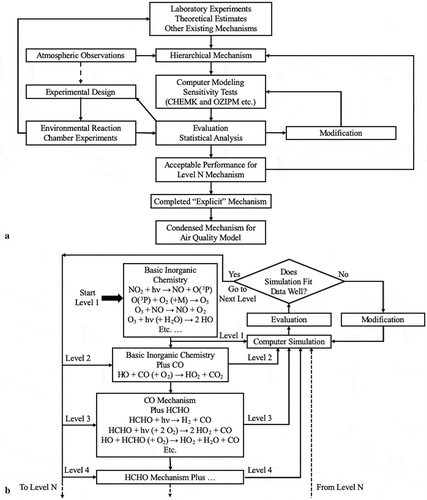
Once the mechanism was completed for nitric oxide and nitrogen dioxide, Level 1 in , carbon monoxide was added to the mechanism. Simulations were made with the resulting Level 2 mechanism and when the Level 2 was determined to perform adequately in simulating the chamber experiments, a few additional reactions were added to the mechanism to represent formaldehyde chemistry (Level 3). The new mechanism Level 3 with formaldehyde was tested against chamber experiments that contained mixtures of formaldehyde, nitrogen oxides, and carbon monoxide and refined again. The next level might be to add acetaldehyde to the mechanism, perform chamber experiments and repeat the process through many layers until a complete, explicit mechanism was developed (Whitten, Killus, and Hogo Citation1980b).
Clearly, the hierarchy of chemical species approach required a very large number of expensive chamber experiments. The process involving evaluation and modification was criticized as “tuning” the mechanism to fit chamber data (Heymann Citation2010). The process could lead to seriously incorrect mechanisms. Whitten and Gery (Citation1986) developed the Carbon Bond Mechanism, version 4 (CB4) with PAN chemistry that was derived from simulations of acetaldehyde/NOx and biacetyl/NOx chamber experiments that were performed at the University of North Carolina (UNC) chamber (Gery, Whitten, and Killus Citation1988). The fit of the chamber data provided a large negative activation energy for the reaction of NO2 with the CH3CH2O2 that made PAN formation very sensitive to temperature (Dodge Citation1989). At lower temperatures, PAN formation increased and this reduced ozone formation due to the sequestration of nitrogen oxides by PAN. This was not in agreement with field measurements and the activation energy for the PAN formation reaction was updated with values from laboratory measurements (Dodge Citation1989). This is one example of the dangers in an overreliance on environmental chamber testing.
A perspective on bottom-up, top-down and the future development of chemical mechanisms for policy relevant air quality models
Gaps in the available experimental kinetics databases need to be filled by theoretical estimates. During the period when the first air quality mechanisms were developed, estimates of reaction properties involving thermochemical approaches to chemical kinetics (Benson Citation1976) would have been considered to be a relatively rigorous approach but given the limited laboratory database, mechanism developers had a relatively free hand in making educated guesses to fill in the gaps. Also, existing gas-phase mechanisms for air quality modeling were used as sources in the construction of new mechanisms. The NCAR Master mechanism Madronich and Calvert (Citation1990), Leone and Seinfeld (Citation1985) and CBM-X (Whitten and Gery Citation1986) are examples of mechanisms that were used for this purpose at that time. This was particularly true of the development of the first version of the RADM mechanism (Stockwell Citation1986) which relied on the evaluation, comparison and condensation of the Leone and Seinfeld, and CBM-X mechanisms.
Now, detailed explicit chemical mechanisms have become recognized as standards by many mechanism developers. These include the MCM (Jenkin, Saunders, and Pilling Citation1997; MCM v3.2 Citation2019; Saunders et al. Citation2003) and the Generator of Explicit Chemistry and Kinetics of Organics in the Atmosphere mechanism (GECKO-A; Mouchel-Vallon et al. Citation2013; Szopa, Aumont, and Madronich Citation2005a, Citation2005b). Some research versions of SAPRC and GECKO-A have highly explicit VOC oxidation mechanisms that are generated by computer. GECKO-A is a protocol that can generate millions of reactions and it is especially focused on improving the simulation of aerosol precursors and linking gas and aqueous-phase chemistry (Mouchel-Vallon et al. Citation2013). All of these mechanisms have a very high level of detail that exceeds the available published laboratory chemical kinetics database (Stockwell et al. Citation2012). Analogy, structure activity relationships (SARs) and more recently, quantum chemistry calculations are used to fill in database gaps. A detailed perspective on the development of SARs for gas-phase atmospheric chemistry is presented by Vereecken et al. (Citation2018). An example of quantum chemistry calculations of reaction rate constants and products used to estimate reaction properties for atmospheric chemistry is given in Gross, Mikkelsen, and Stockwell (Citation2001a, Citation2001b) and discussed more generally elsewhere (Barker and Golden Citation2003; Burke et al. Citation2015; Vereecken, Glowacki, and Pilling Citation2015). Large explicit mechanisms may play a critical role in the development of new mechanisms such as the CRI mechanism.
A new scheme for developing chemical mechanisms for air quality models
Mechanism development (like all modeling) requires simplifications: generalization, distortion, and deletion in the face of uncertainty. Ideal mechanisms would have as few simplifications as possible. Kaduwela et al. (Citation2015) hold that mechanisms such as MCM and GECKO-A are the most explicit, complete and therefore should be used as reference mechanisms. Kaduwela et al. (Citation2015) present a scheme for the development of chemical mechanisms for air quality models to make policy relevant simulations of O3, toxic air pollutants and PM that may become a template for much of gas-phase mechanism development. This scheme seeks to ensure that mechanisms are as complete as possible to simulate changes in pollutant concentrations for the right reasons.
The scheme proposed by Kaduwela et al. (Citation2015) is based on the five stages, . The five stages of chemical mechanism development are Stage 1: Assemble the empirical database; especially laboratory measurements; Stage 2: Mechanism compilation; Stage 3: Evaluation; Stage 4: Mechanism reduction; and Stage 5: Testing of the final condensed mechanism.
Figure 6. Flowchart of the new directions scheme based on five stages proposed by Kaduwela et al. (Citation2015).
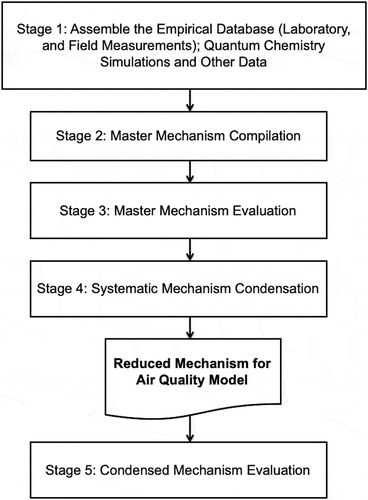
The activities of stage 1, the assembly of the empirical database of laboratory measurements and other data is critical to any development of chemical mechanisms for air quality modeling. Databases are available from the International Union of Pure and Applied Chemistry (IUPAC), IUPAC Task Group on Atmospheric Chemical Kinetic Data Evaluation (IUPAC Citation2019), NASA-Jet Propulsion Laboratory (NASA-JPL, Burkholder et al. Citation2015) and independent reviews such as those supported by the Coordinating Research Council CRC (e.g. Calvert et al. Citation2000, Citation2002, Citation2008, Citation2011, Citation2015).
The stage 2 mechanism compilation, as proposed by Kaduwela et al. (Citation2015) is a master mechanism type approach that may rely on the extensive use of computer programs to generate detailed mechanisms, such as the explicit organic mechanism generator used for SAPRC (e.g. Carter Citation2019) and the more extensive codes for GECKO-A (Aumont, Szopa, and Madronich Citation2005). Developers of self-generating mechanisms assume that including as many as possible of the chemical processes that may be hypothesized reasonably to occur in the atmosphere is more important than the possibility of distortion of simulations introduced by adding a large number of uncertain reactions whose number that greatly exceeds the available empirical chemical database.
We believe that the situation resembles . This figure shows a hypothetical plot of the relationship between the number of reactions in a mechanism and the uncertainty of an air quality model simulation made with the mechanism. If too few reactions are included, then the simulation results will be very uncertain and the addition of reactions from the known database would be expected to decrease the simulation uncertainty. For a given state of knowledge, at some point, there will be a minimum in the uncertainty of the simulations. However, after the minimum point, the addition of a greater number of more uncertain reactions, such as those that are estimated, the simulation uncertainty may increase. Finally, after the number of reactions exceeds the kinetic database the simulations may become highly uncertain.
Figure 7. A hypothesized relationship between gas-phase mechanism size, computational requirements, and uncertainty. The thick vertical line denotes the available empirical chemical kinetic database. The thin vertical lines denote three regions where region 1 denotes mechanisms that are incomplete or overly condensed, region 2 denotes mechanisms that are reasonably condensed and region 3 denotes mechanisms that overly exceed the available data. Additions to the chemical kinetic database will shift the thick line to the right and support more detailed chemical mechanisms. The intention of this figure is to provide a qualitative representation of our text.
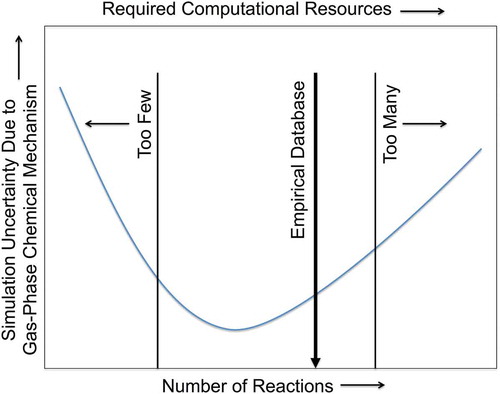
Although all mechanisms used in air quality models must use some assumptions to fill in gaps in chemical knowledge, it appears to us that there is a danger with including too much poorly known chemistry based on untested extrapolations. We are concerned that it is very possible that the requirement to make a very large explicit mechanism as the first step may involve very extensive extrapolation of SAR data that may introduce uncertainty into the process. In some cases, it might be better to exclude some unknown processes because if a reaction is unrealistically fast, slow or has products with unrealistic reactivities, the inclusion of the unknown reaction may result in misleading simulations.
To add confidence in the stage 2 mechanism compilation, research needs to be performed to determine the optimal size of the initial explicit mechanism that will be condensed given the size of the current chemical database. We assume that as the size of the chemical database increases that the optimal size of the initial explicit mechanism should increase too. Research is needed to determine the relationship between the uncertainty in an initial explicit mechanism and the uncertainty in its derived condensed mechanisms.
Evaluation of the master mechanism is stage 3 of the Kaduwela et al. (Citation2015) scheme and it is a critical part of the scheme. In this stage, simulations are made with the master mechanism and the simulations are compared with measurements from field campaigns, environmental chamber experiments and the laboratory. Although it is clear that this evaluation and mechanism refinement is critical, it is not apparent that current field campaign data can be used to improve significantly a chemical mechanism used in a model. Many of the same questions can be raised about the use of environmental chamber data to evaluate a detailed mechanism. In both cases how will the individual VOC oxidation schemes be evaluated if one starts with a “complete” mechanism with potentially thousands of species and thousands or millions of reactions? What does a mechanism developer change to create a better mechanism in response to the evaluation? Stage 3 seems more suited to be used in the concept of mechanism development through a hierarchy of chemical species approach.
Stage 4 proposed by Kaduwela et al. (Citation2015) involves the automatic computer reduction applied to the evaluated detailed mechanism to produce a sufficiently compact mechanism that can be routinely run in a three-dimensional air quality model. The validity of this step depends on the credibility of the detailed mechanism.
We believe that the scheme of Kaduwela et al. (Citation2015) has great merit if it does not become a strict regulatory requirement of government agencies for policy relevant mechanism development. Shutting down other approaches of mechanism development has the strong possibility of hindering progress. We suggest that it may be best to combine the scheme of Kaduwela et al. (Citation2015) with the historical hierarchy of species approaches of Demerjian et al. (Citation1974) and Whitten, Killus, and Hogo (Citation1980b). We see no need to postpone the testing of subsections until a complete large explicit reaction mechanism is completed. For example, probably most mechanism developers create detailed reaction schemes for mechanism subunits that are later condensed down either by hand or by computer-based methods. Making this process more explicit and developing larger subunits along the lines of Kaduwela et al.’s first step would be good, but these subsections should be evaluated against chamber and field data as they are created. Some condensation of subsections as they are developed and tested might reduce the effort required to construct the final condensed mechanism for an air quality model.
We believe that with new technology it should be possible to enhance the chamber database to be used for mechanism development and that new support for environmental chamber experiments should be provided (Hidy Citation2019). Making the environmental chamber database more accessible would promote a closer coupling between the development of mechanisms and testing and therefore greater funding for this purpose and for collecting new empirical data is recommended.
Additional considerations for future research and support of air quality mechanism development
First, it is important to maintain a community of scientists who will conduct independent studies, mechanism development and implement them for evaluation in air quality models because a diverse community provides an important check and balance to the mainstream trends. Funding is needed for the applied research that is necessary to build the kinetics database. For example, there have been many studies to examine the sensitivity of O3 concentrations to rate coefficients (e.g. Gao, Stockwell, and Milford Citation1995, Citation1996). In many cases, the O3 concentrations were sensitive to reactions that are relatively well known, but reducing the uncertainty in rate coefficients from, say 10%, to 5% would help improving confidence in simulations. However, it is very difficult and even unlikely to receive funding from agencies that rely on peer review because such studies would not be considered to be sufficiently creative. Also, funding for many atmospheric chemistry kinetics studies may not fit well with the priorities of funding agencies. In the United States our experience has been that a worthy proposal could easily be regarded as insufficiently fundamental for funding by the National Science Foundation, insufficiently applied for the U.S. EPA and too low in the troposphere for NASA.
There are many conferences that bring various members of the community together. These include the biennial Atmospheric Chemistry Mechanism (ACM) and the International Aerosol Modeling Algorithms (IAMA) meeting that are held at the University of California, Davis. The Gordon Research Conference on Atmospheric Chemistry and the Gordon Research Conference on Biogenic Hydrocarbons and the Atmosphere are two important meetings, but attendance is limited to the Gordon Research Conference selection rules. Many professional societies for chemistry, geosciences, meteorology and the Air and Waste Management Association host excellent international meetings. What seems to be missing is a widely popular venue that brings atmospheric chemists, air quality modelers and those making public policy together effectively. Greater mixing between these groups would encourage greater balance in all three areas. Maybe it would help air quality modelers to be more open to new developments in mechanisms, encourage atmospheric chemists to develop reasonably condensed mechanisms and finally to enlighten policymakers to the strengths and weaknesses of the state of the science. It is an open question if this objective would be served best by modifying the existing meetings or by creating a new one.
We suggest that some attention to the possibility that there may be alternatives to current protocols to create chemical modules for air quality modeling. Here we are asking the unorthodox question: Does a mechanism, in the traditional sense consisting of reactions with their reactants, products (with yields) and rate coefficients, need to be created to have a credible chemical module to simulate O3, PM and other atmospheric constituents of regulatory interest? We suggest that an answer might be found in cheminformatics and machine learning (e.g. Leach and Gillet Citation2007; Wang, Men, and Lu Citation2008). Cheminformatics (also known as Chemoinformatics) is a field that applies information science, data mining and similar fields to chemistry. Perhaps the first problem should be to investigate the possibility of creating a chemical module that makes calculations for higher molecular weight compounds that are based on SAR data directly rather than by estimating explicitly the reactants, products, product yields and rate coefficients of unmeasured reactions.
It may also be time to acknowledge that current gas-phase mechanisms are sufficient without much more development for Eulerian models to make the simulations that are used to evaluate emission reduction strategies for O3. Nikolaou et al. (Citation2018) have used the WRF-Chem model to show that skeletal mechanisms with about 50 species can perform nearly as well as current air quality mechanisms in simulating ozone concentrations but greater detail in the chemical mechanism is much more significant for modeling PM formation (Mouchel-Vallon et al. Citation2013). However, considerable research is needed still to produce air quality mechanisms that accurately simulate PM and especially SOA because its formation is very dependent on the detailed reaction of VOC as suggested in our overview of atmospheric chemistry. Another possible problem with modeling PM is that gas-phase chemistry modules and aerosol modules are not closely coupled in many air quality Eulerian models. We suggest that more research should be conducted to find the effects of the degree of coupling between these modules on overall air quality model performance.
The possibility should be considered that it may be unwise to rely on a single mechanism for air quality modeling for public policy purposes. For example, multiple simulations made with variations in mechanism parameters, initial conditions, emission rates, and other model inputs have been used to estimate the uncertainty associated with air quality simulations (Gao, Stockwell, and Milford Citation1995; Russell et al. Citation1995). Gao, Stockwell, and Milford (Citation1995) successfully used Latin-hypercube sampling to select a set of Monte Carlo simulations that were used to perform an analysis to estimate the effect of uncertainties in rate coefficients and product yields on simulated O3 concentrations. In addition, they estimated the uncertainties in changes in O3 concentrations associated with 25% reductions in VOC and NOx initial concentrations. Russel et al. focused more directly on applying their analysis to the evaluation of urban O3 control strategies. They found that within modeling uncertainty that selective control of more reactive VOC species would be cheaper and more effective than traditional mass-based emission control strategies.
Our current proposal is an extension of Russell et al. (Citation1995) and Gao, Stockwell, and Milford (Citation1995) and we believe that it is worthy of evaluation by regulatory modelers as a potential future approach. Meteorological and air quality models have multiple parameterizations for the boundary layer, microphysics, etc. Why should one “right” mechanism be required when ensemble forecasts are widely used in meteorological forecasting (Lewis Citation2005)? We believe that it is possible that ensemble simulations with multiple mechanisms and emissions inventories will produce a more robust analysis of simulation uncertainties for policymakers than current methods.
A concept for an ensemble air quality simulation system for air quality is shown in . The left-most column represents a set of meteorological fields that are produced by running a meteorological model several variations to provide an ensemble. Ensemble meteorological simulation is an established technique in meteorological forecasting (Lewis Citation2005). The meteorological simulations are made with different physics options, different initializations, different met inputs, etc. Variations in simulated pollutant mixing heights, temperature, moisture and cloud cover are particularity important. The next column to the right illustrates the production of a set of emissions inventories for each chemical mechanism employed in the study. This is a critical step because there is a high level of uncertainty in emissions inventories, even for highly studied urban areas such as the South Coast Air Basin (Fujita et al. Citation2016). The next column to the right shows a number of possible air quality model configurations. The configurations would include different chemical mechanisms, different atmospheric physics selections, etc.
Figure 8. A scheme for ensemble air quality simulations. That shows the generation of sets of meteorological fields, emissions inventories, air quality configurations with variations in chemical mechanisms and other physical variables. An automatic Latin-Hypercube system is used to build and run a “random” series of air quality simulations.
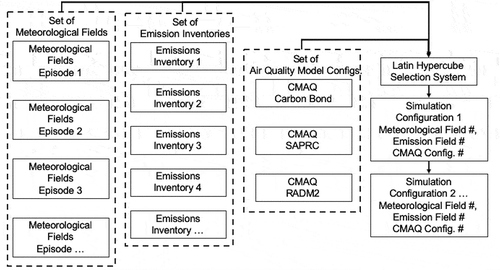
An automatic system to choose between meteorological fields, emission inventories and air quality model configurations (e.g. run-scripts) and mechanisms (previously implemented in the model) could be based on Latin-hypercube sampling (Gao, Stockwell, and Milford Citation1995; McKay, Beckman, and Conover Citation1979) would be used to determine a range of model configurations and episodes to simulate as shown by the right column of . The Latin hypercube sampling (LHS) system will use statistical methods to generate a sample of parameter values from multidimensional distributions that are nearly random. However, it is not possible for the selection to be completely random because of the necessary pairing between emission inventories and chemical mechanisms.
The aggregation of an ensemble of simulations is a complex task and comprehensive plots are needed to display a global sensitivity analysis. An ensemble set of air quality model simulations allow differences to be examined between the various model-configuration simulations. For example, Russell et al. (Citation1995) used cumulative frequency distribution diagrams to display the effects of uncertainty on simulated air pollutant concentrations and the probability of a particular prediction. The authors believe that the major technical barrier to the general adoption of ensemble forecasting for air quality is due to the large demands on required computational resources. However, the computational resources for ensemble air quality simulations might not be that much greater than those required for a large explicit master mechanism approach. There may be difficulties in changing the air quality analysis protocols required by governmental agencies as well.
Observation-based comparisons between data and meteorological and air quality model simulations need to remain the most important methodology of evaluating model performance. However, no simulation made by an atmospheric model will be completely accurate even if the model formulation including its physics, chemistry, numerical methods, emissions, and other input data are “perfect” (Rao et al. Citation2019). Even if the models were perfect the stochastic nature of the atmosphere on both short and long-time scales limit their accuracy and these limitations should be recognized by polity makers.
Conclusion
Gas-phase mechanism development for air quality modeling has made much progress since its early days in the 1960s and 1970s. Basic chemical knowledge was missing, but useful chemical mechanisms were developed through bottom-up methods that relied heavily on environmental chamber experiments (Whitten, Killus, and Hogo Citation1980b). The state of the science of atmospheric chemistry has developed and has been incorporated in the gas-phase chemistry mechanisms that are widely used for air quality modeling that include the Carbon Bond series, SAPRC and RADM/RACM/GACM.
Today’s database is much larger and not yet complete so much of the focus of mechanism developers remains on expanding the laboratory and computational chemistry database (Hidy Citation2019; Vereecken et al. Citation2018), the construction of master chemical mechanisms and reduction of the master mechanisms. We agree with Kaduwela et al. that mechanisms should use the “best, most up-to-date atmospheric chemistry data” and be developed through a transparent process. The current air quality mechanisms developed to be used for policymaking have a clear connection to the literature, often more than mechanisms applied more exclusively to research. Mechanism developers should consider methods such as cheminformatics as an integral part of development and documentation can help strengthen the connection to literature.
We believe that environmental chambers from the major sites in Europe and the United States should be made more accessible either through a single site or a closely coupled network of sites (Hidy Citation2019). New atmospheric chemistry studies involving environmental chambers and field studies should be supported. The environmental chamber experiments should focus on improved studies of both simple and complex mixtures of VOCs so that the new studies will support both top-down and bottom approaches to mechanism development. Detailed evaluations should be performed after the mechanisms are implemented in models and several protocols have been published for this purpose (Astitha et al. Citation2017; Eder and Yu Citation2006; Emery et al. Citation2017; Simon, Baker, and Phillips Citation2012).
The mechanism development communities and the regulatory communities need to come to some consensus regarding the need and frequency of updates for the existing gas-phase mechanisms. Here we have focused primarily on those used for Eulerian modeling of O3 and PM. The need for updates to enhance mechanisms’ ability to simulate PM is greater than for O3 so it seems to us that new mechanism versions should be produced more frequently for PM. However, detailed mechanisms for research and regulatory applications, such as those needed for O3 reactivity modeling, should be produced as new data become available. We suggest that both bottom-up and top-down approaches may face significant challenges in meeting the demand for detailed mechanisms.
Finally, new methods of applying air quality models should be considered. For example, ensemble modeling methods with multiple mechanisms developed under varying assumptions and their emissions inventories should be considered for the analysis of uncertainty in air quality simulations. This will provide a clearer picture of robustness of the analysis for policymakers.
Acknowledgment
The authors thank the China Section of the Air & Waste Management Association for the generous scholarship they received to cover the cost of page charges and make the publication of this review possible. The authors also thank the peer reviewers for their wise and helpful comments.
Additional information
Funding
Notes on contributors
William R. Stockwell
William R. Stockwell is a Research Professor in the Physics Department of the University of Texas at El Paso (UTEP) and an Affiliated Faculty in the Division of Atmospheric Sciences at the Desert Research Institute.
Emily Saunders
Emily Saunders is a Senior Research Scientist in the Global Modeling and Assimilation Office at the NASA Goddard Space Flight Center.
Wendy S. Goliff
Wendy S. Goliff is an Associate Faculty in the Chemistry Department of Riverside City College.
Rosa M. Fitzgerald
Rosa M. Fitzgerald is a Professor in the Physics Department at UTEP.
References
- Astitha, M., H. Luo, S. T. Rao, C. Hogrefe, R. Mathur, and N. Kumar. 2017. Dynamic evaluation of two decades of WRF-CMAQ ozone simulations over the contiguous United States. Atmos. Environ. 164:102–16. doi:10.1016/j.atmosenv.2017.05.020.
- Atkinson, R. 1990. Gas-phase tropospheric chemistry of organic compounds: A review. Atmos. Environ. 24A:1–41. doi:10.1016/0960-1686(90)90438-S.
- Atkinson, R. 1994. Gas-phase tropospheric chemistry of organic compounds. J. Phys. Chem. Ref. Data, Monograph No. 2.
- Atkinson, R. 2000. Atmospheric chemistry of VOCs and NOx. Atmos. Environ. 34:2063–101. doi:10.1016/S1352-2310(99)00460-4.
- Atkinson, R., D. L. Baulch, R. A. Cox, J. N. Crowley, R. F. Hampson, R. G. Hynes, M. E. Jenkin, M. J. Rossi, J. Troe, and IUPAC Subcommittee. 2006. Evaluated kinetic and photochemical data for atmospheric chemistry: Volume II – Gas phase reactions of organic species. Atmos. Chem. Phys. 6:3625–4055. doi: acp-6-/acp-6-3625-2006.
- Atkinson, R., D. L. Baulch, R. A. Cox, R. F. Hampson Jr., J. A. Kerr, M. J. Rossi, and J. Troe. 1997. Evaluated kinetic and photochemical data for atmospheric chemistry: Supplement V. IUPAC subcommittee on gas kinetic data evaluation for atmospheric chemistry. J. Phys. Chem. Ref. Data. 26:521–1011. doi:10.1063/1.556011.
- Atkinson, R., D. L. Baulch, R. A. Cox, R. F. Hampson Jr., J. A. Kerr, and J. Troe. 1992. Evaluated kinetic and photochemical data for atmospheric chemistry: Supplement IV. IUPAC subcommittee on gas kinetic data evaluation for atmospheric chemistry. J. Phys. Chem. Ref. Data. 21:1125–568. doi:10.1063/1.555918.
- Atkinson, R., and W. P. L. Carter. 1984. Kinetics and mechanisms of the gas-phase reactions of ozone with organic compounds under atmospheric conditions. Chem. Rev. 84:437–70. doi:10.1021/cr00063a002.
- Aumont, B., S. Szopa, and S. Madronich. 2005. Modelling the evolution of organic carbon during its gas-phase tropospheric oxidation: Development of an explicit model based on a self generating approach. Atmos. Chem. Phys. 5:2497–517. doi:10.5194/acp-5-2497-2005.
- Badia, A., and O. Jorba. 2015. Gas-phase evaluation of the online NMMB/BSC-CTM model over Europe for 2010 in the framework of the AQMEII-Phase2 project. Atmos. Environ. 115:657–69. doi:10.1016/j.atmosenv.2014.05.055.
- Baklanov, A., U. S. Korsholm, R. Nuterman, A. Mahura, K. P. Nielsen, B. H. Sass, A. Rasmussen, A. Zakey, E. Kaas, A. Kurganskiy, et al. 2017. Enviro-HIRLAM online integrated meteorology–Chemistry modelling system: Strategy, methodology, developments and applications (v7.2). Geosci. Model Dev. 10:2971–99. doi:10.5194/gmd-10-2971-2017.
- Baklanov, A., K. Schlünzen, P. Suppan, J. Baldasano, D. Brunner, S. Aksoyoglu, G. Carmichael, J. Douros, J. Flemming, R. Forkel, et al. 2014. Online coupled regional meteorology chemistry models in Europe: Current status and prospects. Atmos. Chem. Phys. 14:317–98. doi:10.5194/acp-14-317-2014.
- Barker, J. R., and D. M. Golden. 2003. Master equation analysis of pressure-dependent atmospheric reactions. Chem. Rev. 103:4577–92. doi:10.1021/cr020655d.
- Bates, K. H., and D. J. Jacob. 2019. A new model mechanism for atmospheric oxidation of isoprene: Global effects on oxidants, nitrogen oxides, organic products, and secondary organic aerosol. Atmos. Chem. Phys. 19:9613–40. doi:10.5194/acp-19-9613-2019.
- Baulch, D. L., R. A. Cox, P. J. Crutzen, R. F. Hampson Jr., J. A. Kerr, J. Troe, and R. T. Watson. 1982. Evaluated kinetic and photochemical data for atmospheric chemistry: Supplement 1. CODATA Task Group on Chemical Kinetics. J. Phys. Chem. Ref. Data. 11:327–496. doi:10.1063/1.555664.
- Baulch, D. L., R. A. Cox, R. F. Hampson Jr., J. A. Kerr, J. Troe, and R. T. Watson. 1980. Evaluated kinetic and photochemical data for atmospheric chemistry. J. Phys. Chem. Ref. Data. 9:295–471. doi:10.1063/1.555619.
- Benson, S. W. 1976. Thermochemical kinetics: Methods for the estimation of thermochemical data and rate parameters. 2nd ed. New York: John Wiley & Sons.
- Besemer, A. C., and H. Nieboer. 1985. The wall as a source of hydroxy radicals in smog chambers. Atmos. Environ. 19:507–13. doi:10.1016/0004-6981(85)90171-4.
- Bey, I., D. J. Jacob, R. M. Yantosca, J. A. Logan, B. D. Field, A. M. Fiore, Q. Li, H. Y. Liu, L. J. Mickley, and M. G. Schult. 2001. Global modeling of tropospheric chemistry with assimilated meteorology: Model description and evaluation. J. Geophys. Res. 106:23073–95. doi:10.1029/2001JD000807.
- Bloss, C., V. Wagner, A. Bonzanini, M. E. Jenkin, K. Wirtz, M. Martin-Reviejo, and M. J. Pilling. 2005a. Evaluation of detailed aromatic mechanisms (MCMv3 and MCMv3.1) against environ- mental chamber data. Atmos. Chem. Phys. 5:623–39. doi:10.5194/acp-5-623-2005.
- Bloss, C., V. Wagner, M. E. Jenkin, R. Volkamer, W. J. Bloss, J. D. Lee, D. E. Heard, K. Wirtz, M. Martin-Reviejo4, G. Rea, et al. 2005b. Development of a detailed chemical mechanism (MCMv3.1) for the atmospheric oxidation of aromatic hydrocarbons. Atmos. Chem. Phys. 5:641–64. doi:10.5194/acp-5-641-2005.
- Bowman, F. M., and J. H. Seinfeld. 1994. Ozone productivity of atmospheric organics. J. Geophys. Res. 99:5309–24. doi:10.1029/93JD03400.
- Burke, M. P., C. F. Goldsmith, S. J. Klippenstein, O. Welz, H. Huang, I. O. Antonov, J. D. Savee, D. L. Osborn, J. Zádor, C. A. Taatjes, et al. 2015. Multiscale informatics for low-temperature propane oxidation: Further complexities in studies of complex reactions. J. Phys. Chem. A. 119:7095–115. doi:10.1021/acs.jpca.5b01003.
- Burkholder, J. B., S. P. Sander, J. Abbatt, J. R. Barker, R. E. Huie, C. E. Kolb, M. J. Kurylo, V. L. Orkin, D. M. Wilmouth, and P. H. Wine. 2015. Chemical kinetics and photochemical data for use in atmospheric studies, evaluation No. 18. JPL Publication 15-10, Jet Propulsion Laboratory, Pasadena. Accessed May 8, 2019. http://jpldataeval.jpl.nasa.gov.
- Byun, D., and K. L. Schere. 2006. Review of the governing equations, computational algorithms, and other components of the Models-3 Community Multiscale Air Quality (CMAQ) modeling system. Appl. Mech. Rev. 59:51–77. doi:10.1115/1.2128636.
- Cai, C., J. T. Kelly, J. C. Avise, A. P. Kaduwela, and W. R. Stockwell. 2011. Photochemical modeling in California with the SAPRC07C and SAPRC99 chemical mechanisms: Model intercomparison and response to emission reductions. J. Air Waste Manage. Assoc. 61:559–72. doi:10.3155/1047-3289.61.5.559.
- Calvert, J. G., R. Atkinson, K. H. Becker, R. M. Kamens, J. H. Seinfeld, T. J. Wallington, and G. Yarwood. 2002. The mechanisms of atmospheric oxidation of aromatic hydrocarbons. Oxford, UK: Oxford University Press.
- Calvert, J. G., R. Atkinson, J. A. Kerr, S. Madronich, G. K. Moortgat, T. J. Wallington, and G. Yarwood. 2000. The mechanisms of the atmospheric oxidation of the alkenes. Oxford, UK: Oxford University Press.
- Calvert, J. G., R. G. Derwent, J. J. Orlando, G. S. Tyndall, and T. J. Wallington. 2008. Mechanisms of atmospheric oxidation of the alkanes. Oxford, UK: Oxford University Press, Oxford.
- Calvert, J. G., A. Mellouki, J. J. Orlando, M. J. Pilling, and T. J. Wallington. 2011. The mechanisms of the atmospheric oxidation of the oxygenates. Oxford, UK: Oxford University Press.
- Calvert, J. G., J. J. Orlando, W. R. Stockwell, and T. J. Wallington. 2015. The mechanisms of reactions influencing atmospheric ozone. Oxford, UK: Oxford University Press.
- Calvert, J. G., and J. N. Pitts. 1966. Photochemistry. New York: Wiley.
- Calvert, J. G., and W. R. Stockwell. 1983. Deviations from the O3-NO-NO2 photostationary state in tropospheric chemistry. Can. J. Chem. 61:983–92. doi:10.1139/v83-174.
- Carlton, A., C. Wiedinmyer, and J. Kroll. 2009. A review of Secondary Organic Aerosol (SOA) formation from isoprene. Atmos. Chem. Phys. 9:4987–5005. doi:10.5194/acp-9-4987-2009.
- Carter, W. P. L. 1990. A detailed mechanism for the gas-phase atmospheric reactions of organic compounds. Atmos. Environ. 24A:481–518. doi:10.1016/0960-1686(90)90005-8.
- Carter, W. P. L. 1994. Development of ozone reactivity scales for volatile organic compounds. J. Air Waste Manage. Assoc. 44:881–99. doi:10.1080/1073161X.1994.10467290.
- Carter, W. P. L. 1995. Computer modeling of environmental chamber measurements of maximum incremental reactivities of volatile organic compounds. Atmos. Environ. 29:2513–27. doi:10.1016/1352-2310(95)00150-W.
- Carter, W. P. L. 2000. Documentation of the SAPRC-99 chemical mechanism for VOC reactivity assessment. Final Report to California Air Resources Board Contract No. 92-329, and 95-308, California Environmental Protection Agency, Air Resources Board, Research Division, Sacramento, CA, USA. Accessed May 8, 2019. http://www.cert.ucr.edu/~carter/absts.htm#saprc99.
- Carter, W. P. L. 2010a. Development of the SAPRC-07 chemical mechanism. Atmos. Environ. 44:5324–35. doi:10.1016/j.atmosenv.2010.01.026.
- Carter, W. P. L. 2010b. Development of a condensed SAPRC-07 chemical mechanism. Atmos. Environ. 44:5336–45. doi:10.1016/j.atmosenv.2010.01.024.
- Carter, W. P. L. 2016. Preliminary documentation of the SAPRC16 mechanism. Accessed May 8, 2019. http://www.cert.ucr.edu/~carter/SAPRC/16/S16doc.pdf.
- Carter, W. P. L. 2019. Description of the SAPRC-16 mechanism generation system for the atmospheric reactions of VOCs in the presence of NOx. Gateway to the SAPRC-16 mechanism. Accessed September 19, 2019. http://mechgen.cert.ucr.edu/.
- Carter, W. P. L., R. Atkinson, A. M. Winer, and J. N. Pitts Jr. 1981. Evidence for chamber dependent radical sources: Impact on kinetic computer models for air pollution. Int. J. Chem. Kinet. 13:735–40. doi:10.1002/(ISSN)1097-4601.
- Carter, W. P. L., and G. Heo. 2013. Development of revised SAPRC aromatics mechanisms. Atmos. Environ. 77:404–14. doi:10.1016/j.atmosenv.2013.05.021.
- Carter, W. P. L., and F. W. Lurmann. 1991. Evaluation of a detailed gas phase atmospheric reaction mechanism using environmental chamber data. Atmos. Environ. 25:2771–806. doi:10.1016/0960-1686(91)90206-M.
- Collett, R. S., and K. Oduyemi. 1997. Air quality modelling: A technical review of mathematical approaches. Meteorol. Appl. 4:235–46. doi:10.1017/S1350482797000455.
- de Gouw, J., and J. L. Jimenez. 2009. Organic aerosols in the Earth’s atmosphere. Environ. Sci. Technol. 43:7614–18. doi:10.1021/es9006004.
- Demerjian, K. L., J. A. Kerr, and J. G. Calvert. 1974. The mechanism of photochemical smog formation. Adv. Environ. Sci. Technol. 4:1–262.
- Derwent, R. 2017. Intercomparison of chemical mechanisms for air quality policy formulation and assessment under North American conditions. J. Air Waste Manage. Assoc. 67:789–96. doi:10.1080/10962247.2017.1292969.
- Derwent, R. G., M. E. Jenkin, N. R. Passant, and M. J. Pilling. 2007. Reactivity-based strategies for photochemical ozone control in Europe. Environ. Sci. Policy 10:445–53. doi:10.1016/j.envsci.2007.01.005.
- Derwent, R. G., M. E. Jenkin, M. J. Pilling, W. P. L. Carter, and A. Kaduwela. 2010a. Reactivity scales as comparative tools for chemical mechanisms. J. Air Waste Manage. Assoc. 60:914–24. doi:10.3155/1047-3289.60.8.914.
- Derwent, R. G., M. E. Jenkin, and S. M. Saunders. 1996. Photochemical ozone creation potentials for a large number of reactive hydrocarbons under European conditions. Atmos. Environ. 30:181–99. doi:10.1016/1352-2310(95)00303-G.
- Derwent, R. G., M. E. Jenkin, S. M. Saunders, and M. J. Pilling. 2001. Characterization of the reactivities of volatile organic compounds using a master chemical mechanism. J. Air Waste Manage. Assoc. 51:699–707. doi:10.1080/10473289.2001.10464297.
- Derwent, R. G., M. E. Jenkin, S. R. Utembe, D. E. Shallcross, T. P. Murrells, and N. R. Passant. 2010b. Secondary organic aerosol formation from a large number of reactive man-made organic compounds. Sci. Total Environ. 408:3374–81. doi:10.1016/j.scitotenv.2010.04.013.
- Derwent, R. G., C. S. Witham, S. R. Utembe, M. E. Jenkin, and N. R. Passant. 2010c. Ozone in Central England: The impact of 20 years of precursor emission controls in Europe. Environ. Sci. Policy. 13:195–204. doi:10.1016/j.envsci.2010.02.001.
- Dimitriades, B., and M. Dodge (Eds.). 1983. Proceedings of the empirical kinetics modeling approach (EKMA) validation workshop. EPA Report EPA-600/9-83-014, Environmental Sciences Research Lab, Research Triangle Park, NC, USA.
- Dodge, M. C. 1989. A comparison of three photochemical oxidant mechanisms. J. Geophys. Res. 94:5121–36. doi:10.1029/JD094iD04p05121.
- Dodge, M. C. 2000. Chemical oxidant mechanisms for air quality modeling: Critical review. Atmos. Environ. 34:2103–30. doi:10.1016/S1352-2310(99)00461-6.
- Donahue, N. M., A. L. Robinson, C. O. Stanier, and S. N. Pandis. 2006. Coupled partitioning, dilution, and chemical aging of semivolatile organics. Environ. Sci. Technol. 40:2635–43. doi:10.1021/es052297c.
- Ebel, A., R. Friedrich, and H. Rodhe, Eds. 1997. Transport and chemical transformation of pollutants in the troposphere, vol. 7, tropospheric modelling and emission estimation. New York: Springer Verlag.
- Eder, B., and S. Yu. 2006. A performance evaluation of the 2004 release of models-3 CMAQ. Atmos. Environ. 40:4811–24. doi:10.1016/j.atmosenv.2005.08.045.
- Emery, C., Z. Liu, A. G. Russell, M. T. Odman, G. Yarwood, and N. Kumar. 2017. Recommendations on statistics and benchmarks to assess photochemical model performance. J. Air Waste Manage. Assoc. 67:582–98. doi:10.1080/10962247.2016.1265027.
- Emmons, L. K., S. Walters, P. G. Hess, J.-F. Lamarque, G. G. Pfister, D. Fillmore, C. Granier, A. Guenther, D. Kinnison, T. Laepple, et al. 2010. Description and evaluation of the model for ozone and related chemical tracers, version 4 (MOZART-4). Geosci. Model Dev. 3:43–67. doi:10.5194/gmd-3-43-2010.
- Finlayson, B. J., J. N. Pitts Jr., and H. Akimoto. 1972. Production of vibrationally excited OH in chemiluminescent ozone-olefin reactions. Chem. Phys. Letters. 12:495–98. doi:10.1016/0009-2614(72)90016-4.
- Finlayson-Pitts, B. J., and J. N. Pitts Jr, eds. 1986. Chapter 6. Environmental chambers. In Atmospheric chemistry: Fundamental and experimental techniques, 330–402. New York: John Wiley & Sons.
- Finlayson-Pitts, B. J., and J. N. Pitts Jr. 2000. Chemistry of the upper and lower atmosphere: Theory, experiments and applications. New York: Academic Press.
- Flemming, J., V. Huijnen, J. Arteta, P. Bechtold, A. Beljaars, A.-M. Blechschmidt, M. Diamantakis, R. J. Engelen, A. Gaudel, A. Inness, et al. 2015. Tropospheric chemistry in the integrated forecasting system of ECMWF. Geosci. Model Dev. 8:975–1003. doi:10.5194/gmd-8-975-2015.
- Fuentes, J. D., M. Lerdau, R. Atkinson, D. Baldocchi, J. W. Botteneheim, P. Ciccioli, B. Lamb, C. Geron, L. Gu, A. Guenther, et al. 2000. Biogenic hydrocarbons in the atmospheric boundary layer: A review. Bull. Amer. Meteor. Soc. 81:1537–75. doi:10.1175/1520-0477(2000)081<1537:BHITAB>2.3.CO;2.
- Fujita, E. M., D. E. Campbell, W. R. Stockwell, E. Saunders, R. Fitzgerald, and R. Perea. 2016. Projected ozone trends and changes in the ozone-precursor relationship in the South Coast Air Basin in response to varying reductions of precursor emissions. J. Air Waste Manage. Assoc. 66:201–14. doi:10.1080/10962247.2015.1106991.
- Gao, D., W. R. Stockwell, and J. B. Milford. 1995. First order sensitivity and uncertainty analysis for a regional scale gas-phase chemical mechanism. J. Geophys. Res. 100:23153–66. doi:10.1029/95JD02704.
- Gao, D., W. R. Stockwell, and J. B. Milford. 1996. Global uncertainty analysis of a regional scale gas-phase chemical mechanism. J. Geophys. Res. 101:9107–19. doi:10.1029/96JD00060.
- Geiger, H., I. Barnes, K. H. Becker, B. Bohn, T. Brauers, B. Donner, H.-P. Dorn, M. Elend, C. M. F. Dinis, D. Grossmann, et al. 2002. Chemical mechanism development: Laboratory studies and model applications. J. Atmos. Chem. 42:323–57. doi:10.1023/A:1015708517705.
- Geiger, H., I. Barnes, I. Bejan, T. Benter, and M. Spittler. 2003. The tropospheric degradation of isoprene: An updated module for the regional atmospheric chemistry mechanism. Atmos. Environ. 37:1503–19. doi:10.1016/S1352-2310(02)01047-6.
- GEOS-Chem. 2019. Accessed May 8, 2019. http://wiki.seas.harvard.edu/geos-chem/index.php/GEOS-Chem_chemistry_mechanisms.
- Gery, M. W., G. Z. Whitten, and J. P. Killus. 1988. Development and testing of the CBM-1V for urban and regional modeling. Rep. EPA/600/3-88/OI2, U.S. Environ. Prot. Agency, Research Triangle Park, N.C., USA.
- Gery, M. W., G. Z. Whitten, J. P. Killus, and M. C. Dodge. 1989. A photochemical kinetics mechanism for urban and regional scale computer modeling. J. Geophys. Res. 94:12925–56. doi:10.1029/JD094iD10p12925.
- Goliff, W. S., W. R. Stockwell, and C. V. Lawson. 2013. The regional atmospheric chemistry mechanism, version 2. Atmos. Environ. 68:174–85. doi:10.1016/j.atmosenv.2012.11.038.
- Grell, G. A., S. Emeis, W. R. Stockwell, T. Schoenemeyer, R. Forkel, J. Michalakes, R. Knoche, and W. Seidl. 2000. Application of a multiscale, coupled MM5/chemistry model to the complex terrain of the VOTALP valley campaign. Atmos. Environ. 34:1435–53. doi:10.1016/S1352-2310(99)00402-1.
- Grell, G. A., S. E. Peckham, R. Schmitz, S. A. McKeen, G. Frost, W. C. Skamarock, and B. Eder. 2005. Fully coupled “online” chemistry within the WRF model. Atmos. Environ. 39:6957–75. doi:10.1016/j.atmosenv.2005.04.027.
- Griffin, R. J., D. Dabdub, and J. H. Seinfeld. 2002. Secondary organic aerosol 1. Atmospheric chemical mechanism for production of molecular constituents. J. Geophys. Res. 107:4342. doi:10.1029/2001JD000541.
- Gross, A., K. V. Mikkelsen, and W. R. Stockwell. 2001a. A phase-space method for arbitrary bi-molecular gas-phase reactions: Theoretical description. Int. J. Quantum Chem. 84:479–92. doi:10.1002/(ISSN)1097-461X.
- Gross, A., K. V. Mikkelsen, and W. R. Stockwell. 2001b. A phase-space method for arbitrary bi-molecular gas-phase reactions: Application to the CH3CHO + HO and CH3OOH + HO reactions. Int. J. Quantum Chem. 84:493–512. doi:10.1002/(ISSN)1097-461X.
- Guenther, A., T. Karl, P. Harley, C. Wiedinmyer, P. Palmer, and C. Geron. 2006. Estimates of global terrestrial isoprene emissions using MEGAN (model of emissions of gases and aerosols from nature). Atmos. Chem. Phys. 6:3181–210. doi:10.5194/acp-6-3181-2006.
- Haagen-Smit, A. J. 1952. Chemistry and physiology of Los Angeles smog. Ind. Eng. Chem. 44:1342–46. doi:10.1021/ie50510a045.
- Hallquist, M., J. C. Wenger, U. Baltensperger, Y. Rudich, D. Simpson, M. Claeys, J. Dommen, N. M. Donahue, C. George, A. H. Goldstein, et al. 2009. The formation, properties and impact of secondary organic aerosol: Current and emerging issues. Atmos. Chem. Phys. 9:5155–236. doi:10.5194/acp-9-5155–2009.
- Henderson, B. H., F. Akhtar, H. O. T. Pye, S. L. Napelenok, and W. T. Hutzell. 2014. A database and tool for boundary conditions for regional air quality modeling: Description and evaluation. Geosci. Model Dev. 7:339–60. doi:10.5194/gmd-7-339-2014.
- Henrot, A. J., T. Stanelle, S. Schroeder, C. Siengenthaler, D. Taraborrelli, and M. G. Schultz. 2017. Implementation of the biogenic emission model MEGAN into the ECHAM6-HAMMOZ chemistry climate model. Basic results and sensitivity tests. Geosci. Model Dev. 10:903–26. doi:10.5194/gmd-10-903-2017.
- Heymann, M. 2010. Lumping, testing, tuning: The invention of an artificial chemistry in atmospheric transport modeling. Stud. Hist. Philos. M. P. 41:218–32. doi:10.1016/j.shpsb.2010.07.002.
- Hidy, M. G. 2019. Atmospheric chemistry in a box or a bag. Atmosphere 10:401. doi:10.3390/atmos10070401.
- Hinneburg, D., E. Renner, and R. Wolke. 2009. Formation of secondary inorganic aerosols by power plant emissions exhausted through cooling towers in Saxony. Env. Sci. Pollut Res. 16:25–35. doi:10.1007/s11356-008-0081-5.
- Hodzic, A., J. L. Jimenez, S. Madronich, M. R. Canagaratna, P. F. DeCarlo, L. Kleinman, and J. Fast. 2010b. Modeling organic aerosols in a megacity: Potential contribution of semi-volatile and intermediate volatility primary organic compounds to secondary organic aerosol formation. Atmos. Chem. Phys. 10:5491–514. doi:10.5194/acp-10-5491-2010.
- Hodzic, A., J. L. Jimenez, A. S. H. Prévôt, S. Szidat, J. D. Fast, and S. Madronich. 2010a. Can 3-D models explain the observed fractions of fossil and non-fossil carbon in and near Mexico City? Atmos. Chem. Phys. 10:10997–1016. doi:10.5194/acp-10-10997-2010.
- Horowitz, L. W. 2003. A global simulation of tropospheric ozone and related tracers: Description and evaluation of MOZART, version 2. J. Geophys. Res. 108:4784. doi:10.1029/2002JD002853.
- Horowitz, L. W., J. Liang, G. M. Gardner, and D. J. Jacob. 1998. Export of reactive nitrogen from North America during summertime: Sensitivity to hydrocarbon chemistry. J. Geophys. Res. 103:13451–76. doi:10.1029/97JD03142.
- Inness, A., F. Baier, A. Benedetti, I. Bouarar, S. Chabrillat, H. Clark, C. Clerbaux, P. Coheur, R. J. Engelen, Q. Errera, et al., the MACC team. 2013. The MACC reanalysis: An 8 yr data set of atmospheric composition. Atmos. Chem. Phys. 13:4073–109. doi: 10.5194/acp-13-4073-2013.
- Inness, A., A.-M. Blechschmidt, I. Bouarar, S. Chabrillat, M. Crepulja, R. J. Engelen, H. Eskes, J. Flemming1, A. Gaudel, F. Hendrick, et al. 2015. Data assimilation of satellite-retrieved ozone, carbon monoxide and nitrogen dioxide with ECMWF’s composition-IFS. Atmos. Chem. Phys. 15:5275–303. doi:10.5194/acp-15-5275-2015.
- IUPAC. 2019. Accessed May 8, 2019. http:iupac.pole-ether.fr.
- Jacob, D., and R. Podzun. 1997. Sensitivity studies with the regional climate model REMO. Meteorl. Atmos. Phys. 63:119–29. doi:10.1007/BF01025368.
- Jacobson, M. Z. 1999. Fundamentals of atmospheric modeling. 2nd ed. Cambridge, UK: Cambridge University Press.
- Jang, M., and R. M. Kamens. 2001. Characterization of secondary aerosol from the photooxidation of toluene in the presence of NOx and 1-propene. Envir. Sci. Technol. 35:3626–39.
- Jeffries, H., D. Fox, and R. Kamens. 1976. Outdoor smog chamber studies: Light effects relative to indoor chambers. Envir. Sci. Technol. 10:1006–11. doi:10.1021/es60121a016.
- Jeffries, H. E., R. M. Kamens, and K. Sexton. 2013. Early history and rationale for outdoor chamber work at the University of North Carolina. Environ. Chem. 10:349–64. doi:10.1071/EN13901.
- Jenkin, M. E., S. M. Saunders, and M. J. Pilling. 1997. The tropospheric degradation of volatile organic compounds: A protocol for mechanism development. Atmos. Environ. 31:81–104. doi:10.1016/S1352-2310(96)00105-7.
- Jenkin, M. E., S. M. Saunders, V. Wagner, and M. J. Pilling. 2003. Protocol for the development of the master chemical mechanism, MCM v3 (Part B): Tropospheric degradation of aromatic volatile organic compounds. Atmos. Chem. Phys. 3:181–93. doi:10.5194/acp-3-181-2003.
- Jenkin, M. E., L. A. Watson, S. R. Utembe, and D. E. Shallcross. 2008. A common representative intermediates (CRI) mechanism for VOC degradation. Part 1: Gas phase mechanism development. Atmos. Environ. 42:7185–95. doi:10.1016/j.atmosenv.2008.07.028.
- Jenkin, M. E., K. P. Wyche, C. J. Evans, T. Carr, P. S. Monks, M. R. Alfarra, M. H. Barley, G. B. McFiggans, J. C. Young, and A. R. Rickard. 2012. Development and chamber evaluation of the MCM v3.2 degradation scheme for β-caryophyllene. Atmos. Chem. Phys. 12:5275–308. doi:10.5194/acp-12-5275-2012.
- Jenkin, M. E., J. C. Young, and A. R. Rickard. 2015. The MCM v3.3.1 degradation scheme for isoprene. Atmos. Chem. Phys. 15:11433–59. doi:10.5194/acp-15-11433-2015.
- Johnson, D., M. E. Jenkin, K. Wirtz, and M. Martin-Reviejo. 2004. Simulating the formation of secondary organic aerosol from the photooxidation of toluene. Environ. Chem. 1:150–65. doi:10.1071/EN04069.
- Kaduwela, A., D. Luecken, W. Carter, and R. Derwent. 2015. New directions: Atmospheric chemical mechanisms for the future. Atmos. Environ. 122:609–10. doi:10.1016/j.atmosenv.2015.10.031.
- Khosrawi, F., O. Kirner, G. Stiller, M. Höpfner, M. L. Santee, S. Kellmann, and P. Braesicke. 2018. EMAC simulations vs. satellite observations. Atmos. Chem. Phys. 18:8873–92. doi:10.5194/acp-18-8873-2018.
- Kim, Y., F. Couvidat, K. Sartelet, and C. Seigneur. 2011. Comparison of different gas-phase mechanisms and aerosol modules for simulating particulate matter formation. J. Air Waste Manage. Assoc. 61:1218–26. doi:10.1080/10473289.2011.603999.
- Kim, Y. P., and J. H. Seinfeld. 1995. Atmospheric gas–Aerosol equilibrium III. Thermodynamics of crustal elements Ca2+; K+; and Mg2+. Aerosol Sci Technol 22:93–110. doi:10.1080/02786829408959730.
- Kim, Y. P., J. H. Seinfeld, and P. Saxena. 1993a. Atmospheric gas-aerosol equilibrium I. Thermodynamic model. Aerosol Sci Technol 19:157–81. doi:10.1080/02786829308959628.
- Kim, Y. P., J. H. Seinfeld, and P. Saxena. 1993b. Atmospheric gas-aerosol equilibrium II. Analysis of common approximations and activity coefficient calculation methods. Aerosol Sci Technol 19:182–98. doi:10.1080/02786829308959629.
- Kinnison, D. E., G. P. Brasseur, S. Walters, R. R. Garcia, D. R. Marsh, F. Sassi, V. L. Harvey, C. E. Randall, L. Emmons, J. F. Lamarque, et al. 2007. Sensitivity of chemical tracers to meteorological parameters in the MOZART-3 chemical transport model. J. Geophys. Res. 112:D03303. doi:10.1029/2008JD010739.
- Knote, C., P. Tuccella, G. Curci, L. Emmons, J. J. Orlando, S. Madronich, R. Baró, P. Jiménez-Guerrero, D. Luecken, C. Hogrefe, et al. 2015. Influence of the choice of gas-phase mechanism on predictions of key gaseous pollutants during the AQMEII phase-2 intercomparison. Atmos. Environ. 115:553–68. doi:10.1016/j.atmosenv.2014.11.066.
- Kushta, J., M. Astitha, S. Solomos, and G. Kallos. 2013. Chemical weather forecasting using the online fully integrated modeling system RAMS/ICLAMS – Comparison with the offline approach. EGU general assembly 2013. Geophys. Res. Abstr. 15:EGU2013–5573.
- Lafore, J. P., J. Stein, N. Asencio, P. Bougeault, V. Ducrocq, J. Duron, C. Fischer, P. Héreil, P. Mascart, V. Masson, et al. 1998. The Meso-NH atmospheric simulation system. Part I: Adiabatic formulation and control simulations. Ann. Geophys. 16:90–109. doi:10.1007/s00585-997-0090-6.
- Leach, A. R., and V. J. Gillet. 2007. An introduction to chemoinformatics. Revised ed. New York: Springer.
- Lee-Taylor, J., S. Madronich, B. Aumont, A. Baker, M. Camredon, A. Hodzic, G. S. Tyndall, E. Apel, and R. A. Zaveri. 2011. Explicit modeling of organic chemistry and secondary organic aerosol partitioning for Mexico City and its outflow plume. Atmos. Chem. Phys. 11:13219–41. doi:10.5194/acp-11-13219-2011.
- Leighton, P. A. 1961. Photochemistry of air pollution. New York: Academic Press.
- Lelieveld, J., P. J. Crutzen, V. Ramanathan, M. O. Andreae, C. A. M. Brenninkmeijer, T. Campos, G. R. Cass, R. R. Dickerson, H. Fischer, J. A. de Gouw, et al. 2001. The Indian ocean experiment: Widespread air pollution from South and Southeast Asia. Science 291:1031–36. doi:10.1126/science.1057103.
- Leone, J. A., and J. H. Seinfeld. 1985. Comparative analysis of chemical reaction mechanisms for photochemical smog. Atmos. Environ. 19:437–64. doi:10.1016/0004-6981(85)90166-0.
- Lewis, J. M. 2005. Roots of ensemble forecasting. Mon. Wea. Rev. 133:1865–85. doi:10.1175/MWR2949.1.
- Lin, Y.-H., Z. Zhang, K. S. Docherty, H. Zhang, S. H. Budisulistiorini, C. L. Rubitschun, S. L. Shaw, E. M. Knipping, E. S. Edgerton, T. E. Kleindienst, et al. 2012. Isoprene epoxydiols as precursors to secondary organic aerosol formation: Acid-catalyzed reactive uptake studies with authentic compounds. Environ. Sci. Technol. 46:250–58. doi:10.1021/es202554c.
- Lind, J. A., A. L. Lazrus, and G. L. Kok. 1987. Aqueous phase oxidation of sulfur(IV) by hydrogen peroxide, methylhydroperoxide, and peroxyacetic acid. J. Geophys. Res. Atmos. 92:4171–77. doi:10.1029/JD092iD04p04171.
- Madronich, S., and J. G. Calvert. 1990. The NCAR master mechanism of the gas phase chemistry—Version 2.0. NCAR/TN-333+SRT, National Center for Atmospheric Research, Boulder Colorado, USA.
- Makar, P. A., W. R. Stockwell, and S. M. Li. 1996. Gas-phase chemistry mechanisms compression strategies: Treatment of reactants. Atmos. Environ. 30:831–42. doi:10.1016/1352-2310(95)00357-6.
- Maurizi, A., M. D’Isidoro, and M. Mircea. 2010. BOLCHEM: An integrated system for atmospheric dynamics and composition. In Integrated systems of meso-meteorological and chemical transport Models, ed. A. Baklanov, M. Alexander, and R. Sokhi, 89–94. Berlin, Heidelberg: Springer. doi:10.1007/978-3-642-13980-2_8
- McFiggans, G., T. F. Mentel, J. Wildt, I. Pullinen, S. Kang, E. Kleist, S. Schmitt, M. Springer, R. Tillmann, C. Wu, et al. 2019. Secondary organic aerosol reduced by mixture of atmospheric vapours. Nature 565:587–93. doi:10.1038/s41586-018-0871-y.
- McKay, M. D., R. J. Beckman, and W. J. Conover. 1979. A comparison of three methods for selecting values of input variables in the analysis of output from a computer code. Technometrics 21:239–45.
- MCM v3.2. 2019. Master chemical mechanism, MCM v3.2. Accessed April 20, 2019. http://mcm.leeds.ac.uk/MCM.
- Middleton, P., W. R. Stockwell, and W. P. L. Carter. 1990. Aggregation and analysis of volatile organic compound emissions for regional modeling. Atmos. Environ. 24A:1107–33. doi:10.1016/0960-1686(90)90077-Z.
- Midgley, P. M., and M. Reuther, Eds. 2003. Towards cleaner air for Europe – Science, tools and applications, part 2, overviews from the final reports of the EUROTRAC-2 subprojects. New York: Springer Verlag.
- Moore, G. E. 1965. Cramming more components onto integrated circuits. Electronics 38:114–17.
- Mouchel-Vallon, C., P. Bräuer, M. Camredon, R. Valorso, S. Madronich, H. Herrmann, and B. Aumont. 2013. Explicit modeling of volatile organic compounds partitioning in the atmospheric aqueous phase. Atmos. Chem. Phys. 13:1023–37. doi:10.5194/acp-13-1023-2013.
- Moussiopoulos, N., P. Sahm, K. Karatzas, S. Papalexiou, and A. Karagiannidis. 1997. Assessing the impact of the new Athens airport to urban air quality with contemporary air pollution models. Atmos. Environ. 31:1497–511. doi:10.1016/S1352-2310(96)00283-X.
- Mues, A. C. 2013. Modeling air quality in a changing climate. dissertation. Berlin: Freien Universität Berlin.
- Murrells, T. P., N. R. Passant, G. Thistlethwaite, A. Wagner, Y. Li, T. Bush, J. Norris, P. J. Coleman, C. Walker, R. A. Stewart, et al. 2010. UK emissions of air pollutants 1970 to 2007, AEAT/ENV/R/2856. Oxford, UK: AEA. Accessed July 11, 2019. https://uk-air.defra.gov.uk/assets/documents/reports/cat07/1010140907_2007_Report_Final_9.pdf.
- Niki, H., E. E. Daby, and B. Weinstock. 1972. Mechanisms of smog reactions. Adv. Chem. Ser. 113:16–57. doi:10.1021/ba-1972-0113.ch002.
- Nikolaou, Z. M., J. Y. Chen, Y. Proestos, J. Lelieveld, and R. Sander. 2018. Accelerating simulations using REDCHEM_v0.0 for atmospheric chemistry mechanism reduction. Geosci. Model Dev. 11:3391–407. doi:10.5194/gmd-11-3391-2018.
- Pétron, G., G. Frost, B. R. Miller, A. I. Hirsch, S. A. Montzka, A. Karion, M. Trainer, C. Sweeney, A. E. Andrews, L. Miller, et al. 2012. Hydrocarbon emissions characterization in the colorado front range: A pilot study. J. Geophys. Res. 117:D04304. doi:10.1029/2011JD016360.
- Pozzoli, L., I. Bey, S. Rast, M. G. Schultz, P. Stier, and J. Feichter. 2008a. Trace gas and aerosol interactions in the fully coupled model of aerosol‐chemistry‐climate ECHAM5‐HAMMOZ: 1. Model description and insights from the spring 2001 TRACE‐P experiment. J. Geophys. Res. 113:D07308. doi:10.1029/2007JD009007.
- Pozzoli, L., I. Bey, S. Rast, M. G. Schultz, P. Stier, and J. Feichter. 2008b. Trace gas and aerosol interactions in the fully coupled model of aerosol‐chemistry‐climate ECHAM5‐HAMMOZ: 2. Impact of heterogeneous chemistry on the global aerosol distributions. J. Geophys. Res. 113:D07309. doi:10.1029/2007JD009008.
- Rao, S. T., H. Luo, M. Astitha, C. Hogrefe, V. Garcia, and R. Mathur. 2019. On the limit to the accuracy of regional-scale air quality models. Atmos. Chem. Phys. Discuss. preprint. doi:10.5194/acp-2019-642.
- Reynolds, S. D., J. H. Seinfeld, and P. M. Roth. 1973. Mathematical modeling of photochemical air pollution–I. Formulation of the model. Atmos. Environ. 7:1033–61. doi:10.1016/0004-6981(73)90214-X.
- Russell, A., J. B. Milford, M. S. Bergin, S. McBride, L. McNair, Y. Yang, W. R. Stockwell, and B. Croes. 1995. Urban ozone control and atmospheric reactivity of organic gases. Science 269:491–95. doi:10.1126/science.269.5223.491.
- Sander, R., A. Kerkweg, P. Jöckel, and J. Lelieveld. 2005. Technical note: The new comprehensive atmospheric chemistry module MECCA. Atmos. Chem. Phys. 5:445–50. doi:10.5194/acp-5-445-2005.
- Sarwar, G., J. Godowitch, B. Henderson, K. Fahey, G. Pouliot, W. Hutzell, R. Mathur, D. Kang, W. S. Goliff, and W. R. Stockwell. 2013. A comparison of atmospheric composition using the carbon bond and regional atmospheric chemistry mechanisms. Atmos. Chem. Phys. 13:1–18. doi:10.5194/acp-13-1-2013.
- Sarwar, G., D. Luecken, G. Yarwood, G. Z. Whitten, and W. P. L. Carter. 2008. Impact of an updated carbon bond mechanism on predictions from the CMAQ modeling system: Preliminary assessment. J. Appl. Meteorol. Clim. 47:3–14. doi:10.1175/2007JAMC1393.1.
- Saunders, E., 2017. Modeling regional & global atmospheric chemistry mechanisms: Observing adverse respiratory health effects due to tropospheric ozone air pollution from modeling output. dissertation. Washington, DC: Howard University. Available ProQuest Dissertations Publishing, Ann Arbor, Michigan.
- Saunders, S. M., M. E. Jenkin, R. G. Derwent, and M. J. Pilling. 2003. Protocol for the development of the master chemical mechanism, MCM v3 (Part A): Tropospheric degradation of non-aromatic volatile organic compounds. Atmos. Chem. Phys. 3:161–80. doi:10.5194/acp-3-161-2003.
- Savenije, M., L. H. van Ulft, E. van Meijgaard, J. S. Henzing, J. M. J. Aan de Brugh, A. M. M. Manders-Groot, and M. Schaap. 2012. Two-way coupling of RACMO2 and LOTOS- EUROS: Implementation of the direct effect of aerosol on radiation. TNO Report: TNO-060-UT-2012-00508, Utrecht, The Netherlands.
- Seinfeld, J. H. 1988. Ozone air quality models. J. Air Pollut. Control Assoc. 38 (5):616–45. doi:10.1080/08940630.1988.10466404.
- Seinfeld, J. H., and S. N. Pandis. 2016. Atmospheric chemistry and physics: From air pollution to climate change. 3rd ed. New York: John Wiley & Sons.
- Shalaby, A., A. S. Zakey, A. B. Tawfik, F. Solmon, F. Giorgi, F. Stordal, S. Sillman, R. A. Zaveri, and A. L. Steiner. 2012. Implementation and evaluation of online gas-phase chemistry within a regional climate model (RegCM-CHEM4). Geosci. Model Dev. 5:741–60. doi:10.5194/gmd-5-741-2012.
- Simon, H., K. R. Baker, and S. Phillips. 2012. Compilation and interpretation of photochemical model performance statistics published between 2006 and 2012. Atmos. Environ. 61:124–39. doi:10.1016/j.atmosenv.2012.07.012.
- Simon, H., L. Beck, P. V. Bhave, F. Divita, Y. Hsu, D. Luecken, J. D. Mobley, G. A. Pouliot, A. Reff, G. Sarwar, et al. 2010. The development and uses of EPA’s SPECIATE database. Atmos. Pollut. Res. 1:196–206. doi:10.5094/APR.2010.026.
- Stern, R., P. Builtjes, M. Schaap, R. Timmermans, R. Vautard, A. Hodzic, M. Memmesheimer, H. Feldmann, E. Renner, R. Wolke, et al. 2008. A model intercomparison study focusing on episodes with elevated PM10 concentrations. Atmos. Env. 42:4567–88. doi:10.1016/j.atmosenv.2008.01.068.
- Stewart, D. R., E. Saunders, R. Perea, R. Fitzgerald, D. E. Campbell, and W. R. Stockwell. 2019. Projected changes in particulate matter concentrations in the South Coast Air Basin due to basin-wide reductions in nitrogen oxides, volatile organic compounds and ammonia emissions. J. Air Waste Manage. Assoc. 69:192–208. doi:10.1080/10962247.2018.1531795.
- Stockwell, W. R. 1986. A homogeneous gas phase mechanism for use in a regional acid deposition model. Atmos. Environ. 20:1615–32. doi:10.1016/0004-6981(86)90251-9.
- Stockwell, W. R., and J. G. Calvert. 1983a. The mechanism of the HO-SO2 reaction. Atmos. Environ. 17:2231–35. doi:10.1016/0004-6981(83)90220-2.
- Stockwell, W. R., and J. G. Calvert. 1983b. The mechanism of NO3 and HONO formation in the nighttime chemistry of the urban atmosphere. J. Geophy. Res. 88:6673–82. doi:10.1029/JC088iC11p06673.
- Stockwell, W. R., F. Kirchner, M. Kuhn, and S. Seefeld. 1997. A new mechanism for regional atmospheric chemistry modeling. J. Geophys. Res. 102:25847–79. doi:10.1029/97JD00849.
- Stockwell, W. R., and D. Kley. 1994. The Euro-RADM mechanism: A gas-phase chemical mechanism for European air quality studies. Jülich, Germany: Forschungszentrum.
- Stockwell, W. R., C. V. Lawson, E. Saunders, and W. S. Goliff. 2012. A review of tropospheric atmospheric chemistry and gas-phase chemical mechanisms for air quality modeling. Atmosphere 3:1–32. doi:10.3390/atmos3010001.
- Stockwell, W. R., P. Middleton, J. S. Chang, and X. Tang. 1990. The second generation regional acid deposition model chemical mechanism for regional air quality modeling. J. Geophys. Res. 95:16343–67. doi:10.1029/JD095iD10p16343.
- Szopa, S., B. Aumont, and S. Madronich. 2005a. Modelling the evolution of organic carbon during its gas-phase tropospheric oxidation: Development of an explicit model based on a self generating approach. Atmos. Chem. Phys. 5:2497–517. doi:10.5194/acp-5-2497-2005.
- Szopa, S., B. Aumont, and S. Madronich. 2005b. Assessment of the reduction methods used to develop chemical schemes: Building of a new chemical scheme for VOC oxidation suited to three-dimensional multiscale HOx-NOx-VOC chemistry simulations. Atmos. Chem. Phys. 5:2519–38. doi:10.5194/acp-5-2519-2005.
- Tobias, H. J., and P. J. Ziemann. 2000. Thermal desorption mass spectrometric analysis of organic aerosol formed from reactions of 1-tetradecene and O3 in the presence of alcohols and carboxylic acids. Envir. Sci. Technol. 34:2105–15. doi:10.1021/es9907156.
- Trukenmüller, A., D. Grawe, and H. Schlünzen. 2004. A model system for the assessment of ambient air quality conforming to EC directives. Meteorol. Z. 13:387–94. doi:10.1127/0941-2948/2004/0013-0387.
- Venecek, M. A., C. Cai, A. Kaduwela, J. Avise, W. P. L. Carter, and M. J. Kleeman. 2018. Analysis of SAPRC16 chemical mechanism for ambient simulations. Atmos. Environ. 192:136–50. doi:10.1016/j.atmosenv.2018.08.039.
- Venkatram, A., P. K. Karamchandani, and P. K. Misra. 1988. Testing a comprehensive acid deposition model. Atmos. Environ. 22:737–47. doi:10.1016/0004-6981(88)90011-X.
- Vereecken, L., B. Aumont, I. Barnes, J. W. Bozzelli, M. J. Goldman, W. H. Green, S. Madronich, M. R. McGillen, A. Mellouki, J. J. Orlando, et al. 2018. Perspective on mechanism development and structure-activity relationships for gas-phase atmospheric chemistry. Int. J. Chem. Kinet. 50:435–69. doi:10.1002/kin.21172.
- Vereecken, L., D. R. Glowacki, and M. J. Pilling. 2015. Theoretical chemical kinetics in tropospheric chemistry: Methodologies and applications. Chem. Rev. 115:4063–114. doi:10.1021/cr500488p.
- Virtanen, A., J. Joutsensaari, T. Koop, J. Kannosto, P. Yli-Pirila, J. Leskinen, J. M. Makela, J. K. Holopainen, U. Pöschl, M. Kulmala, et al. 2010. An amorphous solid state of biogenic secondary organic aerosol particles. Nature 467:824–27. doi:10.1038/nature09455.
- Volkamer, R., J. L. Jimenez, F. S. Martini, K. Dzepina, Q. Zhang, D. Salcedo, L. T. Molina, D. R. Worsnop, and M. J. Molina. 2006. Secondary organic aerosol formation from anthropogenic air pollution: Rapid and higher than expected. Geophys. Res. Lett. 33:L17811. doi:10.1029/2006GL026899.
- Voller, V. R., and F. Porté-Agel. 2002. Moore’s law and numerical modeling. J. Comput. Phys. 179:698–703. doi:10.1006/jcph.2002.7083.
- Wang, W., C. Men, and W. Lu. 2008. Online prediction model based on support vector machine. Neurocomputing 71:550–58. doi:10.1016/j.neucom.2007.07.020.
- Warneck, P. 2000. Chemistry of the natural atmosphere. 2rd ed. New York: Academic Press.
- Watson, L. A., D. E. Shallcross, S. R. Utembe, and M. E. Jenkin. 2008. A Common Representative Intermediates (CRI) mechanism for VOC degradation. Part 2: Gas phase mechanism reduction. Atmos. Environ. 42:7196–204. doi:10.1016/j.atmosenv.2008.07.034.
- Whitten, G. Z., and M. W. Gery. 1986. Development of CBM-X mechanisms for urban and regional AQSMs, Rep. EPA/600/3-86/012. US Environmental Protection Agency, Research Triangle Park, NC, USA.
- Whitten, G. Z., and H. Hogo. 1999. Mathematical modeling of simulated photochemical smog. EPA Report EPA-600/3-77-011, US Environmental Protection Agency, Research Triangle Park, NC.
- Whitten, G. Z., H. Hogo, and J. P. Killus. 1980a. The carbon-bond mechanism: A condensed kinetic mechanism for photochemical smog. Environ. Sci. Technol. 14:690–700. doi:10.1021/es60166a008.
- Whitten, G. Z., J. P. Killus, and H. Hogo. 1980b. Modeling of simulated photochemical smog with kinetic mechanisms. Volume 1. Final report, July 1978-September 1979. OSTI ID: 6871747, Systems Applications, Inc., San Rafael, CA, USA.
- Whitten, G. Z., J. P. Killus, and R. G. Johnson. 1985. Modeling of auto exhaust for smog chamber data for EKMA development. San Rafael, CA., USA: U.S. Environmental Protection Agency Contract Number 68- 02-3735,Systems Applications, Inc.
- Wilson, W. E., Jr. 1972. A critical review of the gas-phase reaction kinetics of the hydroxyl radical. J. Phys. Chem. Ref. Data. 1:535–73. doi:10.1063/1.3253102.
- Yarwood, G., J. Jung, G. Z. Whitten, G. Heo, J. Mellberg, and M. Estes. 2010. Updates to the carbon bond mechanism for version 6 (CB6), Presented at the 9th Annual CMAS Conference, Chapel Hill, NC, October 11-13.
- Yarwood, G., S. Rao, M. Yocke, and G. Whitten. 2005. Updates to the carbon bond chemical mechanism: CB05. Final report to the US EPA. EPA Report RT-0400675. Accessed May 8, 2019. http://www.camx.com/publ/pdfs/CB05_Final_Report_120805.pdf.
- Yee, L. D., G. Isaacman-VanWertz, R. A. Wernis, M. Meng, V. Rivera, N. M. Kreisberg, S. V. Hering, M. S. Bering, M. Glasius, M. A. Upshur, et al. 2018. Observations of sesquiterpenes and their oxidation products in central Amazonia during the wet and dry seasons. Atmos. Chem. Phys. 18:10433–57. doi:10.5194/acp-18-10433-2018.
- Zaveri, R., and L. K. Peters. 1999. A new lumped structure photochemical mechanism for large-scale applications. J. Geophys. Res. 104:30387–415. doi:10.1029/1999JD900876.
- Zhang, Q., J. L. Jimenez, M. R. Canagaratna, J. D. Allan, H. Coe, I. Ulbrich, M. R. Alfarra, A. Takami, A. M. Middlebrook, Y. L. Sun, et al. 2007. Ubiquity and dominance of oxygenated species in organic aerosols in anthropogenically-influenced Northern Hemisphere midlatitudes. Geophys. Res. Lett. 34:L13801. doi:10.1029/2007GL029979.
- Zhang, Q. J., M. Beekmann, F. Drewnick, F. Freutel, J. Schneider, M. Crippa, A. S. H. Prévôt, U. Baltensperger, L. Poulain, A. Wiedensohler, et al. 2013. Formation of organic aerosol in the Paris region during the MEGAPOLI summer campaign: Evaluation of the volatility-basis-set approach within the CHIMERE model. Atmos. Chem. Phys. 13:5767–90. doi:10.5194/acp-13-5767-2013.