Abstract
This article studies the importance of structural unemployment in the Croatian labour market via occupational mismatch between vacancies and unemployment in the period 2004–2011. The matching function which incorporates the effect of occupational mismatch on the flow of filled vacancies is used for the aggregate market as well as for different submarkets based on the grouping of similar occupations. It appears that occupational mismatch does not have an impact on the aggregate flow of filled vacancies. The portion of total unemployment that can be attributed to occupational mismatch is estimated to be only up to 6%, while the portion of unemployment attributed to mismatch in different submarkets varies greatly.
1. Introduction
Croatia is among those countries which have experienced tremendous changes in their labour markets after the collapse of the former socialist system and the transformation to a market economy. This period of rapid structural change in all areas of the economy was characterised by the slow adjustment of the skills structure of the workforce. Nevertheless, even in the transition economies it was expected that after the initial fall in the employment rate, the emergence of new (private) firms would reverse the process.Footnote1 However, this was for the large part halted by the extremely low mobility of workers across different occupations, industries, and locations (Boeri, Citation2000). Brixiova, Li, and Yousef (Citation2009) further emphasise that skill shortages in Central and East Europe (CEE) serve as the most important obstacle to faster labour reallocation and convergence to the EU-15 employment structures.
Schioppa (Citation1991), on the other hand, states how many studies show that those countries which are the least flexible in matching their unemployed with available vacancies are actually those that have persistently high unemployment rates. According to Schioppa, labour market mismatch is usually the consequence of inadequate education and training or insufficient geographical and occupational labour mobility (Citation1991). Therefore, this article starts from the premise that the reason for high and persistent unemployment in Croatia is the shortage of adequate skills in the labour market, i.e. skills and knowledge of the labour force supply do not match the skills and knowledge that employers seek (demand). This means that the highest portion of unemployment in Croatia is structural unemployment. Thus, the main research question is to what extent can the existing level of unemployment be attributed to structural/occupational mismatch or by how much would unemployment fall were structural balance to be achieved?
In order to investigate this, the matching function approach is used by adopting a model first introduced in Dur (Citation1999). The model estimates the matching function that explicitly incorporates the effect of mismatch instead of adding an arbitrary mismatch index into the matching function. However, in this article the matching process is assumed not to be the same across submarkets. One of the limitations of the educational mismatch study by Dur (Citation1999) is that it estimates only the aggregate matching function that covers the entire labour market.Footnote2 This study estimates, besides the aggregate function, the disaggregated matching functions, based on the grouping of (similar) occupations, that explicitly incorporate the mismatch index for different submarkets. Also, this study uses occupations as a proxy for skills instead of the educational levels used in Dur (Citation1999). This is justified on the grounds that occupations typically define the skill requirements of a vacancy and they characterise the skills of a job-seeker much better than the level of education. Furthermore, this is one of the first studies that tries to estimate the existence of the occupational mismatch in the Croatian labour market and thus should provide valuable policy information. Namely, the assumption that the main source of high and persistent unemployment in Croatia is incongruity between supply and demand in the labour market is not new; it is something used on a daily basis for political purposes. However, rigorous empirical testing is missing. The results of this study could also be important for other transition economies since the attributes inherited from the previous system as well as the roads of transition and joining the EU were, to some extent, similar for all CEE countries.
The article is organised as follows. Section 2 provides the background for the topic by explaining the importance of structural unemployment with emphasis on transition countries. Additionally, this section outlines the main issues in the Croatian labour market and gives the description of the data used in the subsequent analysis. In section 3, a short description of the model adopted from Dur (Citation1999) is given while section 4 provides estimation results together with the relative importance of occupational mismatch for Croatian unemployment in the period 2004–2011. Section 5 concludes.
2. Background and data description
2.1. Structural unemployment
The structural imbalance or mismatch entails a situation in which the characteristics of unemployed workers, particularly in terms of skills, work experience or location, differ from those of the jobs that are available (Jackman & Roper, Citation1987, p. 10). In other words, there is a mismatch between vacant jobs and unemployed workers such that if the latter were available with different skills and/or in different places the level of unemployment would fall (Turvey, Citation1977, p. 210).Footnote3 But, why would this represent a problem?
Sattinger (Citation1993), for instance, shows that the quality of a job match determines the productivity level and earnings in a job. Barcena-Martín, Santiago Budría, and Moro-Egido (Citation2012), on a sample of European university graduates, further show that the mismatched earn on average 11.7% less than their well-matched counterparts. Evidently, mismatch of skills, whether vertical (mismatch in the level of education) or horizontal (mismatch in the type of education), can be a source of unemployment in the labour market. This is confirmed in several empirical studies, predominantly for the US labour market.Footnote4
Increased presence of mismatch in transition countries is the result of significant changes during the 1990s in the structure of product markets, which have led to changes in the structure of labour demand, that were not aligned with the labour supply (Obadić, Citation2005). Furthermore, the persistence of structural unemployment could be caused by factors such as deterioration of human capital of the unemployed or a negative perception of the unemployed on the part of the potential employers (Šergo, Poropat, & Gržinić, Citation2009). Yet, although important, studies on skill, educational or occupational mismatch in former transition countries are scarce. This is primarily a result of the lack of adequate data (Kucel, Vilalta-Bufi, & Robert, Citation2011). In addition, most of the studies that exist in this area usually cover school-to-work transition, sometimes differentiating between vertical and horizontal mismatch (see, for instance, Farčnik & Domadenik, Citation2012; Kogan & Unt, Citation2005; Roberts, Citation1998). However, several recent studies on the transition from centrally planned economy towards market economy shed important insights on how the mismatch could have developed in some of these countries (Bartlett, Citation2012; Jeong, Kejak, & Vinogradov, Citation2008; Kucel et al., Citation2011; Lamo & Messina, Citation2010).
The problem of structural unemployment in Croatia is tackled by, for example, Obadić (Citation2006a, Citation2006b), where via calculation of regional mismatch she indicates the existence of structural unemployment due to mismatch between the demand in the labour market and the location of workers seeking employment. Botrić (Citation2011), on the other hand, tries to explain the basic determinants of structural unemployment in the countries of Southeast Europe, and suggests that a high fiscal burden, the overall restructuring of the economy and remittances are primary determinants of high structural unemployment in the region. However, the only paper, until recently, that tried to estimate some kind of skills mismatch for Croatia is the one by Obadić (Citation2005). In the paper, she estimates disaggregated matching functions according to qualification level and economic activity for Croatia in the period 1992(98)–2002. She shows that in qualifications such as skilled and highly-skilled, secondary-school level and non-university level, there is excess of both supply and demand for labour, i.e. a mismatch. As far as economic activities are concerned, there is a mismatch in the manufacturing industry, wholesale and retail trade, and other social and personal service activities. Matković (Citation2011), in his recent work, indicates that there exists horizontal mismatch in the Croatian labour market between field of education and acquired job (occupation).
Currently, the labour market in Croatia is characterised by low activity, low employment, and high unemployment rates. This indicates a lack of flexibility of the Croatian labour market, but also internal structural problems. This situation is not only the consequence of the ongoing economic and financial crisis; it is a constant process ever since the collapse of the socialist system at the beginning of the 1990s. Nevertheless, there is much heterogeneity among the participants in the labour market in Croatia. Employment and unemployment by region reveal that there are significant regional disparities (Tomić, Citationin press). The same holds for differences in skills, occupations or sectors of economic activity. The bulk of the unemployed population in Croatia is composed of people with one to three-year vocational secondary school education, followed by people with four-year vocational secondary and grammar school education and those with only elementary school education. The share of those who have not completed elementary school is decreasing, whilst the share of those with higher education is increasing, especially after the beginning of the crisis in the second half of 2008.
2.2. Data
The data used in this article are monthly data from the Croatian (public) Employment Service (CES) on: (1) the number of registered unemployed persons (U); (2) the number of reported vacancies (V); and (3) the number of employed persons from the Service registry (M) in the period from January 2004 until December 2011. Apart from the exceptionally large number of the unemployed, the employment records from the Service are generally slightly higher than the reported vacancies in the same month. However, this is expected given that since 2002 employers were no longer legally obliged to report vacancies to the CES. The CES states that after 2004 all the transitional effects of changes in legal obligations on reporting vacancies were no longer visible (CNB, Citation2010). However, the vacancy series in this article are based only on vacancies posted at the CES during the respective months.Footnote5
To be able to detect the existence of mismatch in the labour market, all variables are divided according to the nine broad occupational groups:Footnote6 (1) Legislators, senior officials and managers; (2) Professionals; (3) Technicians and associate professionals; (4) Clerks; (5) Service workers and shop and market sales workers; (6) Skilled agricultural and fishery workers; (7) Craft and related trades workers; (8) Plant and machine operators and assemblers; and (9) Elementary occupations.
Even though skills mismatch is usually examined via educational groups (for instance, Dur, Citation1999), due to unavailability of that kind of data for vacancies and number of hires, we decided to analyse structural unemployment in Croatia via occupational mismatch. In the context of the Croatian labour market, as well as its economic and educational structure, we believe that occupational (mis)match may provide even better information. Fahr and Sunde (Citation2001), for example, argue how separating labour markets by occupations allows looking at the relevant comparable measures for flows and stocks, supporting their argument with evidences which suggest that virtually all job-seekers stay within their profession (occupation) which is not true for the industry or region. In addition, the same authors explain how occupation usually defines the skill requirements of vacancy and characterises the skill of a person which may point to better matching quality in searching for a job (Fahr & Sunde, Citation2002). Additionally, the level of education is usually the same in a specific occupational group (Table ), which means that the use of occupation categories as a proxy for skills is justifiable in this case. Evidently, information on job openings by occupation is not important only for those looking for jobs, but also for those considering education and training options and for policymakers (Shah & Burke, Citation2001).
Table 1. Relation between occupational and educational groups of unemployed – 2011.
The assumption here is that each of the occupations represents a separate submarket in the overall labour market. Namely, this assumption implies that jobs within each submarket are reasonably homogeneous but across submarkets differ significantly, so that job-seekers in one specific submarket (occupation) never apply for a job in any other submarket (occupation), and vice versa. Even in the absence of structural imbalance, the unemployment rate is usually not equal to the vacancy rate in aggregate or in any particular submarket so we measure occupational mismatch relative to the existing aggregate levels of unemployment and vacancies in the economy. CES data show that in the case of low-skilled workers (elementary occupations) the share of the unemployed in total unemployment is higher than the proportion of vacancies in this occupation in relation to the total vacancies. On the other hand, in the case of highly skilled workers (professionals), the share of vacancies is higher than the proportion of the unemployed. In some other professions (for example, technicians and associate professionals), these shares are actually very similar. Clearly, one could say that there exists a mismatch between supply (the unemployed) and demand (vacancies) in some of the submarkets, while in others there is no visible mismatch.
However, the share of some submarkets (occupations) in the total number of both vacancies and unemployment is too smallFootnote7 to be able to bring any strong conclusions. In addition, some of these occupations are too distinct from each other and the labour (sub)markets probably function in a completely different way. Therefore, we have grouped these nine occupations into two main categories:
white-collar occupations that includes legislators, senior officials and managers; professionals; technicians and associate professionals; and clerks, and
blue-collar occupations that includes service workers and shop and market sales workers; skilled agricultural and fishery workers; craft and related trades workers; plant and machine operators and assemblers; and elementary occupations.
The empirical analysis will be conducted for these two submarkets separately in order to account for possible differences in the functioning of the labour market (including the matching process) for different (more similar) groups of occupations.
3. Empirical strategy
The model in this article is based on procedure introduced in Dur (Citation1999) which actually stems from the paper by Jackman and Roper (Citation1987).
It all starts with the concept of the matching function. Hujer, Blien, Caliendo, and Zeiss (Citation2002) explain how the matching process actually serves as a proxy for the differences in the geographic and skill characteristics between the vacant jobs and the job-seekers:(1)
where M denotes the number of successful matches, U represents the number of unemployed, V is the number of vacancies, while the coefficient i indicates the occupation in this case.
The above expression is often written in the form of a Cobb-Douglas function:(2)
where k indicates the efficiency of the labour market, and α and β are the coefficients of (partial) elasticities that indicate the relative importance of supply (U) and demand (V) in the labour market.
As mentioned before, it is assumed that the aggregate labour market consists of a number of completely separable submarkets differentiated by the type of occupation. This means that job-seekers belonging to occupation i cannot (or do not) search for a job in any other occupation. The same goes for vacancies – a vacancy that belongs to occupation i is never filled by a job-seeker that belongs to some other occupation. Thus, the aggregate matching function is just the sum of the matching functions across the whole labour market:(3)
Equation Equation3(3) shows that the aggregate number of filled vacancies (matches) depends on the stocks of aggregate unemployment and vacancies, the efficiency parameter k and the distribution of unemployment and vacancies over submarkets (occupations).
The term in expression Equation3
(3) is equal to one if, for each submarket (occupation) i, the share of the unemployed that belongs to the submarket i in aggregate unemployment (Ui/U) is equal to the share of vacancies belonging to the submarket i in aggregate vacancies (Vi/V). If this term is actually equal to one, i.e. the labour market situation is equally (un)favourable in each submarket, it is called perfect structural balance (Dur, Citation1999; based on Jackman & Roper, Citation1987). The difference between actual unemployment (U) and unemployment in perfect structural balance (US) represents an indicator of mismatch in the labour market. From equation Equation3
(3) this difference equals to:Footnote8
(4)
where mm represents the mismatch indicator that can be interpreted as the share of total unemployment that can be attributed to mismatch. Obviously, the importance of mismatch on the overall level of unemployment depends on the distribution of both unemployment and vacancies over submarkets (occupations), but also on the size of the particular submarket. This means that if both U and V are high in one submarket and low in another, shifting the unemployed from the first submarket to the second one does not tremendously increase the number of matches, or that relatively high unemployment and low vacancies in a quite small submarket leads to only a moderately higher level of mm (Dur, Citation1999). Based on this expression, one can observe how equation Equation3(3) represents the matching function that incorporates the effect of occupational mismatch on the (aggregate) flow of filled vacancies.
In order to estimate how much of (total) unemployment can be attributed to occupational mismatch in the period January 2004–December 2011, we use the matching function derived in the equation Equation3(3) . Its empirical counterpart looks like the following:
(5)
where index t is introduced in order to distinguish between different time periods (months). The error term is assumed to have all the usual characteristics.
Parameter kt in equation Equation5(5) represents a set of variables that might affect the search behaviour of both the unemployed and employers, i.e. the efficiency of the matching process. In this case, we use (linear) time trend to account for changes in search behaviour related to unobserved characteristics. In addition, we use the share of the number of users of unemployment benefits in total unemployment, a variable that should affect the search behaviour of the unemployed.Footnote9
4. Estimation results
The model in this article is estimated using non-linear least squares (NLS) estimation, but because of possible simultaneity, we also estimate the model using nonlinear two-stage least squares instrumental variable (TSLS IV) estimation, treating the unemployment, vacancies and the share of the number of users of unemployment benefits in total unemployment as endogenous. In addition to exogenous and lagged endogenous variables as additional instruments we use logs of the index of construction works, the share of the average net in the average gross wage and the spread between interest rates on short-term loans for enterprises and interest rates on foreign currency deposits for enterprises.Footnote10
Furthermore, since these are relatively high frequency data, controlling for seasonal variation seems particularly important. Therefore, estimations include monthly dummies to control for differentiated vacancies and outflows throughout each year. Given that we have data on the flow of filled vacancies by occupations, we estimate the matching function not only on the aggregate level, but also for each of the submarkets defined in the previous section – white-collar and blue-collar occupations – using the same approach as for the aggregate function. Additionally, since the model assumes constant returns to scale we present here only the restricted (α + β = 1) model specification (Table ). First, let us discuss the results for the aggregate function.
Table 2. Estimation results for the restricted estimation.
At first glance, it seems that there are no major differences between NLS and IV estimation. For both the NLS and TSNLS IV estimation the coefficient of elasticity (α) for the unemployment is larger than the coefficient of elasticity for vacancies (β) which means that firms are less successful in finding workers than workers in finding jobs. The reason for this may be the limitation in labour supply, mismatch, the asymmetry of information, etc. Additionally, for both the NLS and TSNLS IV estimation, the coefficient for the incorporated mismatch index (equation Equation4(4) ) seems to be insignificantly different from its theoretical value of one. Finally, the share of the users of unemployment benefits in total unemployment has a significant negative impact on the matching process, while time trend significantly positively affects matching process.Footnote11
Still, more interesting results occur if we look at the disaggregated functions, i.e. different submarkets based on similar groups of occupations. In all the cases (submarkets) results are largely similar to those for the aggregate function. The differences mainly lie in the size of the elasticity coefficients (in the market for white-collar occupations there is somewhat smaller weight on the unemployment) or the coefficient for the time trend (slightly higher for white-collar occupations or slightly lower for blue-collar occupations). The share of the users of unemployment benefits among unemployed negatively affects the matching process in both disaggregated markets.
However, the main difference lies in the coefficient for the mismatch indicator. For instance, it seems that in the market for white-collar occupations, the occupational mismatch index significantly positivelyFootnote12 affects the process of matching, while in the market for blue-collar occupations this index has a negative (insignificant) impact. This finding suggests that perhaps due to the specific distribution of both unemployment and vacancies over different submarkets and due to the size of each particular submarket, the mismatch index proves to be insignificant (and positive) on the aggregate level while it has different (opposite) effects in the submarkets for white- and blue-collar occupations. This result supports the logic behind the estimation of the disaggregated matching functions in this article. Evidently, the labour market for white-collar and blue-collar occupations operates on different postulates.Footnote13
As previously mentioned, there has been a recession in Croatia since the second half of 2008 which has caused a huge rise in unemployment. In order to see whether the crisis may have caused some of the (unusual) results in our estimations, we estimate the (restricted) models again for two different sub-periods: 2004–2007 and 2008–2011 (Table ). Although the sample is now much smaller we can observe some differences between the two sub-periods. For instance, time trend is significant (and positive) for the blue-collar occupations as well as for the aggregate market only in the second sub-period (2008–2011) while for the white-collar occupational submarket the opposite is true. The coefficient for the incorporated mismatch index is not significant in any of the presented cases, although it suggests a positive impact on the matching process while the share of the users of unemployment benefits in total unemployment has a significant (negative) effect only in the labour market for white-collars. In addition, in almost all of the estimations the coefficient for the unemployment (α) is smaller than in the original model specification (Table ).
Table 3. Estimation results for the restricted estimation – crisis effect.
Additionally, by using the estimated parameters from equation Equation3(3) we can determine how much of total unemployment can be attributed to occupational mismatch. The mismatch index (equation Equation4
(4) ) for the aggregate function is calculated with the estimated coefficients of elasticity from the TSNLS IV restricted estimation for February 2004–December 2011 period (column 2 in Table ) and is illustrated in Figure (left-hand side).
Figure 1. Share of total unemployment attributed to occupational mismatch (left) and unemployment attributed to occupational mismatch as a percentage of the labour force (right).
Notes: mm – mismatch index.
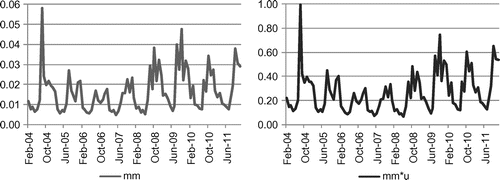
The figure clearly shows that occupational mismatch explains only 1% to 6% of total unemployment,Footnote14 with significant variability (seasonality) of the index. This result, together with regression estimations in Table , suggests that occupational mismatch is not very important for the high and persistent (aggregate) unemployment in Croatia. The right-hand side of Figure shows unemployment attributed to occupational mismatch as a percentage of the labour force, which is calculated as the mismatch indicator, mm, multiplied by the unemployment rate, u. It is observable that the two indicators (relative and absolute) follow a similar trend, which implies that in the period when the unemployment rate was high, the mismatch index was also high, and vice versa. What’s more, this pattern suggests that the mismatch indicator is counter-cyclical, i.e. it increases during recessions and decreases during booms. But again, it needs to be pointed out that occupational mismatch is not very important for the overall unemployment in Croatia. According to these results, if structural balance were achieved, i.e. if there were no mismatch between unemployment and vacancies in the labour market, the unemployment rate would fall by roughly 0.2 to 0.8 percentage points.
As far as the mismatch indicator, mm, for each of the submarkets is concerned, there is again great (monthly) variability in the indicator in every submarket, together with significant difference across two different submarkets. In the case of white-collar workers the indicator explains between 2% and 20% of total white-collar unemployment, but only up to 1% for blue-collar occupations. Once again, the results show remarkable difference between the functioning of the labour market for white- and blue-collar occupations in Croatia. Unfortunately, data on the size of the workforce by occupations do not exist so it was impossible to check how much of the unemployment as a percentage of the labour force is attributed to occupational mismatch for each of the occupational groups (submarkets).
It needs to be pointed out that there are several possible shortcomings of this study. First of all, the number of vacancies used in the analysis is not the total number of open positions in a given month. If the extent of non-posting of vacancies were uniform across submarkets, our mismatch index would be unaffected (Dur, Citation1999). However, it is not very likely that this holds in reality.
Another problem concerns the variables used as instruments in the TSNLS estimation. Namely, this article uses same variables as instruments for all the submarkets (occupational groups) as for the aggregate market. However, variables that proved to be good instruments for the aggregate market may not be as good for the analysed submarkets. Thus, the obtained results should be taken with caution. Furthermore, since endogenous variables (especially unemployment) exhibit huge persistency throughout the observed period lagged endogenous variables perhaps would not serve as sufficient instruments. That is why we checked whether endogenous variables transformed by within-transformation or first-differenced endogenous variables as instruments would provide better results; however, the outcome shows rather similar results as when the lagged endogenous variables served as instruments (see Table in Appendix).
Furthermore, since it has already been shown that there exists a regional mismatch in the Croatian labour market (Obadić, Citation2006a, Citation2006b), the model could be controlled for (possible) regional mismatch.Footnote15 This is done by estimating the model using the data for occupational distribution of unemployment and vacancies on a NUTS2 level in CroatiaFootnote16 but both fixed-effects panel estimation as well as regular NLS estimation with regional dummies models show that the regional effects are not significant (see Table in Appendix). Additionally, the original model (Dur, Citation1999) assumes the function exhibits constant returns to scale. Nevertheless, this is not true in all cases in this article (especially in the case of blue-collar occupations). That is why some of the results might be biased (for instance, the calculation of the portion of total unemployment due to occupational mismatch in the market for blue-collars).
Finally, the choice of nine broad occupational groups can give rise to doubt if we consider two questions: (1) is it detailed enough to provide important information on the (in)existence of structural unemployment in the Croatian labour market; and (2) is the use of occupation categories a good proxy for skills? Even though not quite clear from the presented analysis, the answer to both of these questions is yes. As explained earlier, this is the best grouping of occupations available from the CES statistics, while the question of the use of occupations as a proxy for skills is justifiable on the grounds that more sophisticated occupations usually imply higher educational achievement (see Table ).
5. Conclusions
This article studies the importance of structural unemployment in the Croatian labour market through estimation of the matching function that incorporates the effect of occupational mismatch on the flow of filled vacancies. According to the obtained results it appears that occupational mismatch does not have significant impact on the aggregate flow of filled vacancies, i.e. on the matching process in the overall labour market. However, when the labour market is examined through its submarkets, i.e. similar occupational groups, occupational mismatch seems to have an influence on the matching process in these (sub)markets. Namely, occupational mismatch (significantly) positively affects the matching process in the market for white-collars, while it has negative (insignificant) impact in the (sub)market for blue-collar occupations. In addition, for both the overall labour market as well as for each of the submarkets (occupational groups) the share of the users of unemployment benefits in total unemployment has a negative impact on the matching process, while time trend positively affects the matching process which indicates that Croatia is experiencing greater matching efficiency in the labour market over time. Moreover, in most of the cases the hypothesis of constant returns to scale cannot be rejected.
Nonetheless, the fraction of total (aggregate) unemployment that can be attributed to occupational mismatch is estimated to be between 1% and 6%, depending on the time period. This number is pretty low to be able to explain the high and persistent unemployment in Croatia. The fraction of unemployment attributed to mismatch in different submarkets varies greatly; from 2% to 20% in the white-collar submarket and only up to 1% for blue-collar occupations. If there were no (occupational) mismatch in the Croatian labour market, the unemployment rate would fall by roughly 0.2 to 0.8 percentage points. Hence, the general conclusion is that occupational mismatch has some impact on the matching process in (occupational) submarkets, while its effect on the level of overall unemployment is not very important.
What do these findings tell us with respect to policy implications? Taking the obtained results from this article, together with some previous findings (Obadić, Citation2005, Citation2006a, Citation2006b), it seems that mismatch between supply and demand in the labour market is not a predominant factor in explaining unemployment in Croatia. This is especially true in the time of economic crisis, when deficient demand serves as a primary factor in explaining high and persistent unemployment. However, this does not mean that structural unemployment is not an issue, since the existing works present just ‘the tip of the iceberg’ in this area. Still, in order to propose concrete policy measures, further research concerning mismatch in the Croatian labour market is needed.
Notes
1. It has been argued that Croatia went through a somewhat different path in this process in comparison to other CEE economies due to the existence of some form of market economy already in the 1980s as well as due to the impact of civil war in the early 1990s. For details, see Bićanić and Babić (Citation2008).
2. Fahr and Sunde (Citation2004) argue that the results on the aggregate level are of little help when trying to target certain labour market interventions to certain groups of workers or firms in order to maximise their impact.
3. A comprehensive overview of the literature regarding skills mismatch is given in Quintini (Citation2011).
4. See, for instance, Barlevy (Citation2011), Estevao and Tsounta (Citation2011), Cotti and Drewianka (Citation2012), or Sahin, Song, Topa, and Violante (Citation2012).
5. I.e. they represent the flow (as opposed to stock) variable.
6. Based on International Standard Classification of Occupations (ISCO). Military occupations are left out of the analysis since in some of the periods there were no registered unemployed or vacancies in this group. Perhaps a more detailed categorisation of occupations would bring more information. However, due to its specific construction, the next categorisation provides too detailed grouping of occupations which means that many of these groups do not have any open vacancies or even any unemployed in many of the months studied in the article and thus it could not be used in the empirical estimation.
7. Legislators, senior officials and managers; skilled agricultural and fishery workers; and plant and machine operators and assemblers, with shares in total employment and vacancies of less than 10%.
8. For more details, refer to Appendix.
9. For more details, see Dur (Citation1999).
10. In Dur (Citation1999), instruments used in the model were, besides lagged endogenous variables, the logs of capacity utilisation, the tax wedge, consumer minus producer prices, the size of the working-age population and the capital stock. Unfortunately, data for most of these variables are not available in Croatia, especially on a monthly basis.
Details on the ‘appropriateness’ (Hausman and Sargan tests) of the used instruments are available in Appendix.
11. Šergo et al. (Citation2009) show that the improvement of the matching efficiency in Croatia has been rising since the war and the de-industrialisation shocks in the 1990s which indicates that Croatia is experiencing greater matching efficiency in the labour market over time.
12. Remember that in the empirical equation (equation Equation5(5) ) the coefficient for the mismatch index is expressed as (1 − ξ).
13. We also estimated the unrestricted model. Those estimations suggest that the mismatch index does not have a (statistically) significant impact on the process of matching. However, for both white- and blue-collar submarkets the results suggest a negative impact of occupational mismatch on the process of matching. Additionally, in most of the cases the null hypothesis about constant returns to scale (α + β = 1) cannot be rejected. Results are available upon request.
14. Obadić (Citation2006a, Citation2006b) calculated the ‘implicit’ regional mismatch indicator using arbitrary coefficients of elasticities (both α and β were set to 0.50) and she obtained values for the regional mismatch indicator ranging from 2% to 4% in the period 1993–2002.
15. For instance, Jurajda and Terrell (Citation2009) explain how within-country regional variation in inherited human capital in four transition economies (Czech Republic, Hungary, Bulgaria and Ukraine) accounts for the bulk of regional variation in unemployment.
16. The analysis is done on a NUTS2 instead of a NUTS3 level since there were no data for specific occupations in many months in the latter, and thus the estimation (that uses logarithms) could not have been conducted.
References
- Barcena-Martín, E., Santiago Budría, S., & Moro-Egido, A. I. (2012). Skill mismatches and wages among European university graduates. Applied Economics Letters, 19, 1471–1475.10.1080/13504851.2011.633886
- Barlevy, G. (2011). Evaluating the role of labor market mismatch in rising unemployment. Economic Perspectives, 35, 82–96.
- Bartlett, W. (2012). Structural unemployment in the Western Balkans: Challenges for skills anticipation and matching policies. European Planning Studies. Retrieved from http://www.tandfonline.com/doi/abs/10.1080/09654313.2012.722933
- Bićanić, I., & Babić, Z. (2008). Survey of the croatian labour market. In D. Boršič & A. Kavlker (Eds.), Labour market characteristics in selected economies (pp. 51–69). Maribor: Schwarz.
- Boeri, T. (2000). Optimal speed of transition 10 years after. (Discussion Paper No. 2384). Centre for Economic Policy Research (CEPR).
- Botrić, V. (2011). Structural unemployment and its determinants in Southeast Europe. Ekonomska misao i praksa, 20, 81–100.
- Brixiova, Z., Li, W., & Yousef, T. (2009). Skill shortages and labour market outcomes in Central Europe. Economic Systems, 33, 45–59.10.1016/j.ecosys.2008.07.002
- Croatian National Bank. (2010). Bulletin No. 160 – Quarterly report. Zagreb: Croatian National Bank.
- Cotti, C. D., Drewianka, S. (2012). Labor market inefficiency during the current business cycle: Evidence from industry beveridge curves. (Working Paper). University of Wisconsin-Milwaukee.
- Dur, R. A. J. (1999). Mismatch between unemployment and vacancies in the Dutch labour market. Applied Economics, 31, 237–244.10.1080/000368499324462
- Estevao, M., Tsounta, E. (2011). Has the great recession raised U.S. structural unemployment? (Working Paper No. WP/11/105). IMF.
- Fahr, R., & Sunde, U. (2001). Disaggregate matching functions (Discussion Paper No. 335). IZA.
- Fahr, R., & Sunde, U. (2002). Estimations of occupational and regional matching efficiencies using stochastic production frontier models (Discussion Paper No. 552). IZA.
- Fahr, R., & Sunde, U. (2004). Occupational job creation: Patterns and implications. Oxford Economic Papers, 56, 407–435.10.1093/oep/gpf059
- Farčnik, D., & Domadenik, P. (2012). Has the Bologna reform enhanced the employability of graduates? Early evidence from Slovenia. International Journal of Manpower, 33, 51–75.10.1108/01437721211212529
- Greene, W. H. (2008). Econometric analysis (6th ed.). Upper Saddle River, NJ: Prentice Hall.
- Hujer, R., Blien, U., Caliendo, M., & Zeiss, C. (2002). Macroeconometric evaluation of active labour market policies in germany – A dynamic panel approach using regional data (Discussion Paper No. 616). IZA.
- Jackman, R., & Roper, S. (1987). Structural unemployment. Oxford Bulletin of Economics and Statistics, 49, 9–36.
- Jeong, B., Kejak, M., & Vinogradov, V. (2008). Changing composition of human capital: The Czech Republic, Hungary and Poland. Economics of Transition, 16, 247–271.10.1111/j.1468-0351.2008.00320.x
- Jurajda, S., & Terrell, K. (2009). Regional unemployment and human capital in transition economies. Economics of Transition, 17, 241–274.10.1111/ecot.2009.17.issue-2
- Kogan, I., & Unt, M. (2005). Transition from school to work in transition economies. European Societies, 7, 219–253.10.1080/14616690500083428
- Kucel, A., Vilalta-Bufi, M., & Robert, P. (2011). Graduate labor mismatch in Central and Eastern Europe (Working Paper No. 259). Universitat de Barcelona: Espai de Recerca en Economia.
- Lamo, A., & Messina, J. (2010). Formal education, mismatch and wages after transition: Assessing the impact of unobserved heterogeneity using matching estimators. Economics of Education Review, 29, 1086–1099.10.1016/j.econedurev.2010.06.002
- Matković, T. (2011). Obrasci tranzicije iz obrazovnog sustava u svijet rada u Hrvatskoj [Patterns of transition from education to the world of work in Croatia]. (Doctoral dissertation). Zagreb: Pravni fakultet Sveučilišta u Zagrebu.
- Obadić, A. (2005). Dezagregirana analiza tržišta rada Hrvatske prema stručnoj spremi i djelatnostima. [Disagregated analysis of Croatian labour market according to qualification level and activities]. Ekonomija, 11, 588–620.
- Obadić, A. (2006a). Theoretical and empirical framework of measuring mismatch on a labour market. Zbornik Ekonomskog fakulteta u Rijeci [Proceedings of Rijeka Faculty of Economics], 24, 55–80.
- Obadić, A. (2006b). Influence of regional mismatch on the employment process in selected transition countries. Ekonomski pregled, 57, 3–30.
- Quintini, G. (2011). Over-qualified or under-skilled: A review of existing literature (Working Paper No. 121). OECD Social, Employment and Migration.
- Roberts, K. (1998). School-to-work transitions in former communist countries. Journal of Education and Work, 11, 221–238.10.1080/1363908980110301
- Sahin, A., Song, J., Topa, G., & Violante, G. L. (2012). Mismatch unemployment ( NBER Working Paper No. 18265).
- Sattinger, M. (1993). Assignment models of the distribution of earnings. Journal of Economic Literature, 31, 831–880.
- Schioppa, F. P. (Ed.). (1991). Mismatch and labour mobility. Cambridge: Cambridge University Press.
- Šergo, Z., Poropat, A., & Gržinić, J. (2009). Croatia’s beveridge curve and estimation of natural rate of unemployment. Economic Research-Ekonomska Istraživanja, 22, 29–47.
- Shah, C., & Burke, G. (2001). Occupational replacement demand in Australia. International Journal of Manpower, 22, 648–663.10.1108/EUM0000000006235
- Tomić, I., (in press), “Regional matching (In)efficiency on the Croatian labour market.” Acta Oeconomica, 64.
- Turvey, R. (1977). Structural change and structural unemployment. International Labour Review, 116, 209–215.
Appendix 1:
More details about derivations of equations 3, 4 and 5
Perfect structural balance
Matching function, M (·), is by assumption convex, liner homogeneous function, so it can be written in a following form: , where f’ > 0, f’’ < 0 and index i stands for occupation in this case.
If there is no structural unemployment, the existing number of unemployed, given the pattern of vacancies, should maximise aggregate hires, i.e. ; s.t.
= U = const.\Vi − given. First order condition gives:
= const. which provides the definition of the structural balance where the ratio of unemployment to vacancies is equalised across submarkets (Jackman & Roper, Citation1987).
Mismatch indicator
It needs to be pointed out that the result from equation Equation4(4) holds only in the case of α + β = 1, i.e. if the matching function exhibits constant returns to scale. Namely, in perfect structural balance (S) it holds that
as well as that
, which also gives the following result:
. In deriving the equation Equation4
(4) , all the preceding assumptions are equally important.
By substituting the appropriate expressions for MS and M into MS = M we get: . Next, dividing both sides by
and by
(and k) we obtain:
. Replacing
by
leads us to
after which one gets the relationship between perfect structural balance unemployment (US) and actual unemployment (U), but only if the assumption that α + β = 1, i.e. β = 1 - α holds.
In case the assumption about constant returns to scale does not hold the relationship between perfect structural balance unemployment (US) and actual unemployment (U) is: . However, if we stick to the assumption from the original model(α + β = 1) the difference between actual unemployment and perfect structural balance unemployment is equal to equation Equation4
(4) .
Empirical counterpart of equation Equation3
(3)
Evidently, the equation Equation5(5) is slightly more flexible than the exact counterpart of equation Equation3
(3) . It would look like:
.
However, if we want to follow the procedure from the original paper (Dur, Citation1999), where the author stresses that he does not initially enforce constant returns to scale nor a unitary coefficient for the mismatch index, we will stick to equation Equation5(5) in our estimation too.
Appendix 2:
Instruments
In this part we show some details about the instrumental variable estimation, i.e. choosing the ‘appropriate’ instruments and evaluating their ‘appropriateness’.
Correlation matrix
Table shows correlation coefficients between endogenous variables (unemployed, vacancies and the share of the users of the unemployment benefits in total unemployment) and potential instruments.
Table A.1. Correlation coefficients between endogenous variables and potential instruments.
As is evident from the table variables that are highly (negatively) correlated with the unemployment variables are indices for the state of the economy in different areas: construction, industrial production, retail trade or the official Zagreb Stock Exchange share index. In addition, the share of the average net in the average gross wage is also correlated with the unemployment variables. In this case, we chose the index of construction works as an instrument for the unemployment variables being that in most of the observed period (2004–2011) construction sector was the ‘driving force’ of the Croatian economy. Moreover, from Table one can observe that this index is not correlated with the matching variable. In the case of vacancies – none of the observable variables is highly correlated with the number of vacancies on a monthly basis (apart from the number of opened vacancies for seasonal employment). Still, there are some variables that are pretty correlated with (aggregate) vacancies and not with the number of hirings (like the index of construction works or the share of net in gross wage). For the share of the users of the unemployment benefits in total unemployment the most correlated variable is the spread between interest rates on short-term loans for enterprises and interest rates on foreign currency deposits for enterprises. This variable is not correlated with the number of hirings/matchings, and it is only slightly correlated with the number of vacancies, and could serve as a good instrument.
Hausman test
This test usually evaluates the significance of an estimator versus an alternative estimator. In this case, we test the potential endogeneity of regressors by comparing LS and IV (TSLS) estimator. The null hypothesis says that the potentially endogenous regressors (u, v and unben) are actually exogenous, i.e. both the LS and IV estimators are consistent (Greene, Citation2008). In this case we use the so-called variable addition test as explained in Greene (Citation2008). In the first step we regress all three variables – u, v and unben – onto the all exogenous regressors (including all the instruments) and save the (estimated) residuals from each of the three regressions. The endogenous regressor is exogenous in the case if these residuals are not correlated with residuals from the original specification (regression).
Since we do not have information about the residuals from the original specification, in the second step we regress the dependent variable from the model (m) that should be correlated with the residuals from the original specification onto the all the variables from the original model plus the residuals from the first step. If the coefficient for the residuals in this second step is significant, we have endogenous regressors in the original specification and must proceed with IV (TSLS) estimation. Table shows estimated coefficients for residuals in the second step. In addition, F-statistics is also shown. As is evident from the tables, in some cases the coefficients for residuals are significant while in others (especially for vacancies) are not.
Table A.2. Hausman specification test.
Test of over-identifying restrictions
Test of over-identifying restrictions is often called Sargan or Hansen test. It is based on the assumption that if the instruments are really exogenous then the residuals (from TSLS IV estimation) should be uncorrelated with the exogenous variables (Table ). The over-identifying restrictions test statistic can be calculated as N*R2 (the number of observations multiplied by the coefficient of determination) from the LS regression of the residuals onto the set of exogenous variables (including instruments). This statistic will be asymptotically chi-squared under the null hypothesis that the error term is uncorrelated with the instruments, i.e. that all the instruments are exogenous. Test statistic has q, or in our case 3(6), degrees of freedom (number of instruments – number of endogenous regressors). Table shows values of the test statistic (N*R2) for each of the models estimated before. One can observe that in most of the cases the null hypothesis cannot be rejected.
Table A.3. Test of over-identifying restrictions (Sargan test).
Appendix 3:
Robustness check
This part presents some of the mentioned alternative calculations for the model presented in the main text. This is not robustness check as understood usually in the literature; it is more of a ‘normative robustness check’ where some alternatives to the existing calculations as well as some new calculations are presented.