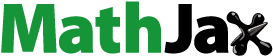
Abstract
In this paper we investigate whether consumer inflation expectations in the E.U. Member States (M.S.) were more forward-looking after the onset of the financial crisis (October 2008–2016) and after the most turbulent times (2013–2016). We compare the results with pre-crisis forward-lookingness (2002–September 2008). Our examination covers the euro area and M.S. with national currencies. We study the properties of expectations, i.e., expectational errors, and macroeconomic efficiency of expectations, as well as estimating the hybrid specification of expectations. We examine panel data. We found that the properties of expectations changed after the crisis sparked off and in the low inflation and deflation environment. We also discovered that formation patterns of expectations within the euro area subsample and non-euro area M.S. sample vary. As far as we know, so far no comparison of expectations properties, especially forward-lookingness among M.S. with and without common monetary policy, has been presented in the literature nor were panel data examined for the post-crisis period. Hence, the paper contributes to the literature on the properties of expectations in the E.U.
1. Introduction
The aim of this paper is to juxtapose properties of consumers’ expectations, especially their forward-lookingness (F.L.), in the European Union (E.U.) economies and compare them over the pre-crisis, post-crisis eruption and post-crisis periods. We use the survey-based proxies of inflation expectations. An important issue discussed in our study is the sample coverage and division. We cover ten euro area Member States (M.S.) that introduced the euro currency in 2002 and eight non-euro area M.S. that have not yet adopted the common currency. With such a choice it is possible to discuss the difference in expectations properties among our subsamples. The E.U. economies operate under common provisions regarding central bank priorities, independence of policymakers and policy transparency. Irrespective of the established general frameworks of monetary policy, national solutions might differ at the strategical and operational level, including with respect to individualised responses to economic performance. With comparable frameworks in all M.S. we can conduct a panel data examination instead of an individual country analysis which is most commonly applied in such examinations.
In addition to the comparison of the euro area vs. non-euro area M.S., we take up a very topical issue, namely the impact that the onset of the financial crisis exerted on the formation of consumers’ inflation expectations. Although there had been studies carried out on the post-crisis evolution of expectation formation, they were not many of them, especially when discussions centre on consumers. Moreover, they relied on a much shorter time sample than ours: here, the whole sample covers the time from 2002 to 2016. It starts with the euro introduction in the euro area M.S. We view the 2002–September 2008 span as a pre-crisis period, October 2008–2016 as a post-crisis eruption period and January 2013–2016 as a post-crisis period. Having made the distinction between the ‘post-crisis incidence’ and ‘post-crisis’ period, we noticed perseverance of the crisis impact on expectations. To the best of our knowledge, post-crisis results have not yet been delivered.
We expect to find differences in the properties of expectations for our subsamples. Our assumptions on the existence of such differences are rooted in monetary policy-related factors (monetary policy frameworks stability, their orientation on the formation of expectations and forward-looking communication of policy actions) and previous inflation experience of our subsamples. Since the results of the previous examinations on the degree of F.L. are inconclusive, we consider this research to be explanatory in nature – leastwise in the part offering a comparison of the euro area and non-euro area M.S. and the very recent post-crisis results.
The paper by Łyziak and Mackiewicz-Łyziak (Citation2014) is closest to ours in terms of methodology. Thus, we consider F.L. not only through a hybrid specification of expectations – we do not discuss the sole relation of backward- and forward-looking components of expectations – but also via forecast errors and macroeconomic efficiency of expectations. The entire analysis can be interpreted from the perspective of a policy-maker’s ability to align expectations to its actions. Minor errors of expectations may indicate that expectations are more convergent with actual policy actions and outcomes (not necessarily to inflation target). When backward-looking information on potential expectations drivers is omitted, there is more room for consumers’ focus on central bank’s communication. Lastly, a more important rational component in the formation of expectations may indicate better perception of the shock and more convergent expectations in terms of policy actions.
The innovative element of our paper is related to the sample and its division into the euro area and non-euro M.S. and the research question arising from such division. We address the issue of the possible differences in the properties of expectations in the euro area M.S. and non-euro area M.S. Thus, our research complements the perspective of transition vs. advanced economies. Another aspect which seems to be natural contribution of our examination is the time span that covers more non-turbulent years than previous papers on the issue of pre- and post-crisis development of expectations.
2. Literature background
Since the neoclassical revolution in macroeconomics rationality of expectations has been the baseline assumption for monetary transmission models. Most of the New Keynesian frameworks embrace this notion. However, rationality of expectations does not mirror the actual behaviour of economic agents: the hypothesis of rational expectations was rejected on the empirical basis (as in the case of the euro area, i.a., by Dias, Duarte, and Rua (Citation2010), for the United Kingdom (U.K.) by Mitchell and Weale (Citation2007), for Czechia and Hungary by Kokoszczyński, Łyziak, and Stanisławska (Citation2010), for Poland by Łyziak (Citation2013), for Sweden by Dräger (Citation2015), and finally for Croatia by Erjavec, Lolić, and Sorić (Citation2015)).
Once rationality of expectations has been rejected, room is made for assessment of the properties of expectations, including a forward-looking component in their formation. Regardless of the extensive research on the rationality of expectations, only a small portion tackles the F.L. analysis. The results of F.L. examination refer mostly to the hybrid specification of expectations where expectations are related to the forward-looking and backward-looking component. The degree of F.L. varies substantially across the countries and changes over time. Gerberding (Citation2001) assessed the average level of F.L. among professional forecasters at 0.32 for Germany and 0.47 for Italy and France (0.13). The degree of anticipation of future inflation in the European monetary union reached 0.40. The consumers in Germany kept the high degree of F.L. and – surprisingly – the consumers in France outperformed professionals in terms of the formation of forward-looking expectations. Consumers’ expectations in Italy and the euro area were entirely backward-looking.
The F.L. of financial markets experts was studied by Heinemann and Ullrich (Citation2006) in the context of the monetary policy change from Bundesbank to the European Central Bank (E.C.B.). The authors estimated hybrid specifications of expectations for the period 1992–2004 and for the subsamples 1992–1998 and 1999–2004. The degree of F.L. in the whole sample was 0.26, whereas it rose from 0.13 (prior to the E.C.B. launch) to 0.18 (from 1999). The change of the monetary regime did not affect the formation of expectations or the degree of their F.L. much.
A comprehensive study of expectations F.L. was presented in Łyziak (Citation2009). It covered 27 E.U. M.S. and the time span 2002–2007. The average weight of forward-looking factors in consumers’ expectations was below 10%. The highest fraction of F.L. of consumers’ expectations was in Italy, Netherlands and the U.K. In 11 countries expectations were only backward-looking. The results varied substantially across the countries, but also over time.
The latest crisis encouraged researchers to focus on expectation F.L. once again. Łyziak and Mackiewicz-Łyziak (Citation2014) estimated hybrid specifications of consumers’ expectations in the case of advanced economies and economies in transition being part of the E.U. In seven economies consumers were solely backward-looking. The most F.L. consumers came from Estonia, France and Netherlands. In addition to the country-by-country analysis, the authors provided the panel data specification. The full sample degree of F.L. for all countries reached 0.12. It was substantially lower prior to the crisis (0.02) and higher after its outburst (0.15). The consumers in transition economies were less forward-looking in comparison to the consumers from advanced economies; they also produced less accurate forecasts while ignoring more relevant information. In both group of economies, F.L. was higher after the crisis erupted, but the change for transition economies was spectacular – from 0.03 to 0.15.
The several studies covering the issue of F.L. presented above can be summed up in the following way: (1) examination of F.L. was usually part of a broader study of the characteristics of expectations and was mostly done via sole estimation of a hybrid specification of expectations. (2) Such research was contingent on the data availability as the history of surveys on expectations starts in 1990s, while the methodology of surveys and frequencies change. (3) The proxy of expectations matters: since expectations are not directly observable, the assumptions on their distribution and quantification procedures affect the result. (4) The results vary substantially across the countries and over time: consumers change the way they form their expectations. (5) Hardly any panel data analysis that includes several economies exists.
In this examination, using panel data modelling, we juxtapose properties of expectations in the euro area and non-euro area M.S. examined as time series cross-sectional data, expecting to find them dissimilar. Several reasons are available to support our assumption. The first one is related to a common monetary policy in place (or lack of it) as well as the way its forward-looking actions are communicated. The way the E.C.B. communicates regarding common monetary policy could become a coordinating factor affecting group-specific results of the euro area economies. The E.C.B. offers the same forward-looking communication for the entire euro area. Such communication could align economic agents’ expectations with the future actions taken by the E.C.B. While this, in turn, can be reflected in more forward-looking expectations – assuming that the communication is effective. Such F.L. would be mirrored not only in a substantial component of rationality in the formation of expectations, but also in smaller expectation errors. More accurate forecasts stand for more convergent expectations with the actual policy results. Additionally, when central bank’s communication gains importance, economic agents are more prone to ignoring historical information on the drivers of expectations. The results of this study will indicate the effectiveness of common monetary policy communication in achieving F.L. rather than that of national policies, bearing in mind that the results for both subsamples are generated as cross-country panel examination.
Expectations alignment to policy actions due to policy communication is especially important for short-term expectations (which are covered by our research) as they are more volatile and heterogeneous (Fukač, Citation2010). There is no perfect alignment of expectations with inflation target, which is the most desired situation from the central bank’s perspective. An alternative option is the alignment of the public’s expectations with the central bank’s expectations and its future policy. This could come about by employing forward-looking communication and signalling intentions, including publication of inflation forecasts (or projections). Forecasts produced for the entire monetary policy horizon can align private forecasts with policy outcomes for a shorter horizon than the monetary policy horizon, especially when the target is temporarily missed (Skořepa & Kotlán, Citation2003). The effect of economic transparency – which, is related to data, models and forecasts – is transmitted via information effect and incentive effects of central bank’s communication for expectations. Information effect stems from the picture of the economy painted by central bank’s forecasts, i.e., it is far from being perfect when the inflation target is met throughout the entire forecasts horizon. It projects an image of an economy deeply affected by shocks. Economic agents derive these shocks from forecasts and may adjust their forecasts as a result of this. Thus, the degree of expectations deviating from the actual inflation in the future is lowered (Geraats, Citation2014). The incentive effect refers to an ex ante structural change in economic behaviour due to the different information structure delivered in central bank’s communication. When the public realises the shock, it gives more room for the central bank’s accommodating actions without de-anchoring its own long-run expectations and having short-run expectations more aligned to policy actions (Geraats, Citation2013). In the light of the above-mentioned theoretical mechanisms, we assume that the properties of consumers’ expectations in the euro area may benefit from a common coordinating factor (communication which aligns expectations with actual actions) which is not available for the non-euro area consumers. Thus, group-specific results for the euro area could be different from group-specific results for the economies that do not enjoy common monetary policy announcements. Nonetheless, as no previous research addressing this issue is available as far as we know, we find our examination explanatory.
The existence of the coordination effect of central bank’s transparency on expectations is confirmed in the empirical literature. Ehrmann, Eijffinger, and Fratzscher (Citation2012) found evidence for a sizeable effect of central banks’ economic transparency, including forecast publications, on forecast disagreement of professionals and non-professionals. Their study covered 12 E.U. and non-member states. Also, several papers tackle the issue of whether inflation forecasts made by central banks actually affect inflation expectations of private agents. The analysis, which was based on the V.A.R. model and covered Canada, Japan, Sweden, Switzerland, and the U.K., proved that central banks are able to affect expectations via their inflation forecasts (Hubert, Citation2015a). Parallel interactions were found for the U.S.A. (Hubert, Citation2015b). As evidenced in literature even imprecise inflation forecasts impact expectations (Crowe & Meade, Citation2008; Ehrmann et al., Citation2012).
Our second rationale for searching the difference in the properties of expectations in our subsamples is related to the examination of cross-sectional data: we acknowledge that some central banks of the non-euro area subsample are even more transparent that the E.C.B (the Czech National Bank and the Sveriges Riksbank publish forecasts consistent with policy paths). However, some others are not (National Bank of Bulgaria, National Bank of Romania). In this study we examine expectations derived from surveys that are held nationally. Consumers in the euro area that respond to the survey’s questions enjoy common and forward-looking communication as well as expectations-oriented monetary policy. Consumers in the non-euro area M.S. receive national communication of monetary policy (also F.L. but the degree and quality of communication differs). The extent to which central bankers pay attention to overt stabilisation of inflation expectations differs across the countries in the non-euro area subsample. General frameworks of monetary policy were less stable over time in these countries.
Lastly, our subsamples differ in terms of the history of disinflation. The euro area M.S. have registered lower inflation rates for a longer time. Backward-looking factors are more obvious inflation-drivers when past inflation is relatively high and the central bank’s credibility (i.e., the public assessment of the central banker’s ability to achieve preannounced target) is lower.Footnote1 The examination of individual countries presented at the beginning of this section suggests a lower degree of F.L. in emerging markets which are the majority of the non-euro area subsample.
The summary of our assumption of finding differences across subsamples is the following: We analyse generalised, cross-sectional results for both subsamples. Thus, we get a picture of the properties of expectations analysed commonly for the euro area and a picture of these properties analysed jointly for prospective members of the euro area (except the U.K.). These pictures may differ for monetary policy-related reasons. Except common communication, they cover monetary policy framework stability over time and its focus on expectations. The non-euro subsample is much more unstable and diversified according to these criteria.
As we also address the question of the degree of F.L. before and after the outburst of the financial crisis, the literature describing the impact that the crisis exerted on expectations is discussed here as well. The pre-crisis and post-crisis studies generally confirm that the formation of expectations was changed by the crisis and its consequences. In addition to the study by Łyziak and Mackiewicz-Łyziak (Citation2014) referred to above, the same results can be found in Andreou, Eminidou, and Zachariadis (Citation2016). They demonstrated that the reaction of consumers in the fifteen euro area M.S. was different in terms of their expectations when it comes to monetary policy shocks in the pre- and post-crisis times. The study of the E.C.B. Survey of Professional Forecasters by Dovern and Kenny (Citation2017) proved the existence of a break in the distribution of experts’ forecasts and a higher risk of lower inflation. The majority of studies on the formation of pre-crisis vs. post-crisis expectations corroborate the impact the crisis exerted on expectations. Nonetheless, they do not offer a direct comparison of the impact in the euro area and non-euro area countries.
3. Sample and data
Our sample covers tenFootnote2 euro area M.S.: Austria, Belgium, Finland, France, Greece, Germany, Italy, Netherland, Portugal and Spain, and eight non-euro area M.S.: Bulgaria, Croatia, Czechia, Hungary, Poland, Romania, Sweden and the U.K. The euro area M.S. subsample is more coherent. Except for the monetary policy held by the E.C.B., they are all advanced economies with a relatively long price stability period ahead. The non-euro area M.S. are diversified: they cover economies in transition and two advanced economies, countries which not so long ago experienced high and volatile inflation rates and different monetary policy regimes. Being aware of the diversification of the non-euro M.S. subsample, we conduct our examination twice: for the entire subsample and with the exclusion of Sweden and the U.K. What our subsamples have in common is constitutional regulations regarding central banks and monetary policy provisions within the E.U.
Consumer expectations are examined in qualitative surveys. We use the datasets offered by the European Commission Business and Consumers Surveys which are conducted monthly (i.e., our sample for the euro area covers 10 M.S. over 178 time intervals, while the non-euro area: 8 M.S. over 178 time intervalsFootnote3). The question on expected inflation is: ‘When compared to the past 12 months, how do you expect consumer prices to develop in the next 12 months?’ The answers to choose from included: ‘They will…increase more rapidly, increase at the same rate, increase at a slower rate, stay about the same, fall, don’t know’ (European Commission, Citation2016). We quantify expectations with the canonical Carlson and Parkin (Citation1975) probabilistic approach adjusted for the polychotomous survey as presented by Batchelor and Orr (Citation1988). Carlson and Parkin’s quantification procedure is extensively applied in empirical research (Dräger, Citation2015; Łyziak, Citation2009, Citation2013), thus the details are not given here. Quantification of expectations is a preliminary stage of our examination.
As we would like to test the rationality of expectations and their F.L., we avoid using actual inflation rates as a scaling factor for such quantification. Therefore, the subjectified version of quantification is applied, where the perceived inflation rate is the scaling factor. Perceived inflation is derived from the same survey questions concerning price development over the last 12 months. To quantify consumers’ answers, a scaling variable must be chosen as well. It is constituted by price dynamics, perceived by consumers as a ‘natural’ rate of inflation or a ‘moderate’ rate of inflation, which reflects the permanent or trend rate of price changes and can be approximated by smoothing the actual inflation (Łyziak, Citation2010). In this study, the 36-month’ moving average of inflation rates represents the ‘natural rate of inflation’.
Moreover, we compiled a dataset of the following macroeconomic indicators: broad money, market interest rates, exchange rates, industrial production index, unemployment rate and oil prices. They represent the year-to-year change or the most recent value of some economic variables, and are used to test macroeconomic efficiency of expectations.
4. Methodology
As suggested by Łyziak and Mackiewicz-Łyziak (Citation2014), the analysis of expectation F.L. should not be limited to a mere estimation of the hybrid specification of expectations. Therefore, we examine: (1) mean errors of expectations, (2) macroeconomic efficiency and (3) hybrid specification of expectations. While testing (2) and (3) we apply standard, theory-related formulas (Geberding, 2001; Łyziak, Citation2013).
While analysing (1) we refer to median errors to diminish the impact of outliers on the results. Forecasts accuracy complement the analysis of F.L. as the same degree of F.L. in the formation patterns of expectations might be related to different expectational errors.
Macroeconomic efficiency (2) is described with the following equations:
(1)
(1)
(2)
(2)
where
is expectation error, in which
is expected at time t inflation rate formed 12 months earlier and
is the actual inflation in period t,
is a macroeconomic variable affecting inflation and
is a white noise error. The two equations were estimated for panel data; therefore we took into account the fixed effects model and the random effects model. To choose a specific model, either the fixed or random effects model, we run the Hausman test. With its null hypothesis the preferred model is the random effects model, while the alternative hypothesis points to the fixed effects model. Macroeconomic efficiency does not occur when
This means that consumers do not process available information efficiently while forming their expectations. Informational lag of 2M is also considered: it is a standard assumption in any examination of consumer expectations as the consumers need time to process information and the surveys may precede the publication of latest economic figures. All the time series were tested for their stationarity (with A.D.F. and K.P.S.S. tests). The non-stationary time series were modified before they were taken into account in equations (i.e., first differences of 3M interbank rates, log differences of the euro and dollar exchange rates and log differences of oil prices were used), while the stationary ones were modified in levels.
There are two alternatives of the hybrid specification of expectations (notations analogous to EquationEquations (1)(1)
(1) and Equation(2)
(2)
(2) ):
(3)
(3)
(4)
(4)
EquationEquation (3)(3)
(3) presents a mixture of forward-looking and adaptive expectations (with an added component representing the change of current inflation), whereas EquationEquation (4)
(4)
(4) incorporates the forward-looking and the static approaches. In the two cases, parameter
represents the forward-looking (rational) component of inflation expectations. The higher the
the more forward-looking are consumers’ expectations. When it is not different from zero – expectations are fully backward-looking. Presenting the results, we refer to the equation with better statistical properties, assuming that it is a more suitable reflection of the formation pattern of expectations in the economies that we cover. In this section we focus on the economic meaning of the models, whereas more details on the rearrangement of equations are presented in Appendix 1.
To check the impact of the crisis outburst and the crisis itself on expectation F.L., we run the procedure four times: for the whole sample, the pre-crisis sample, the post-crisis eruption sample and finally the post-crisis sample. Additionally, we test for the change of the degree of F.L. in the following periods: (1) before 2008, (2) after 2008 and (3) after 2013 through estimating a model with interactions. We construct dummy variables taking 1 in the period of interest and 0 otherwise, and allow it to interact with the variable and
Next, we test for the significance of the variables (EquationEquations (3)
(3)
(3) and Equation(4)
(4)
(4) in Appendix 1).
Our data series consist of repeated time-series observations on fixed, cross-sectional units. Such panel data, also named ‘time-series cross-sectional data’ (T.S.C.S.), are likely to be characterised by complex error structures. Estimating the coefficients using the ordinary least squares (O.L.S.) method produces inefficient approximations with biased standard errors. In such case, two estimators are recommended – either the feasible generalised least squares estimator (F.G.L.S.) of Parks (Citation1967) or an alternative estimator, based on O.L.S., but using ‘panel-corrected standard errors’ (P.C.S.E.) of Beck and Katz (Citation1995) – see, for example, Reed and Webb (Citation2010).
In order to choose the proper estimator, we follow the requirements presented in Reed and Ye (Citation2011) as well as Moundigbaye, Rea, and Reed (Citation2017). Reed and Ye present the results of the Monte Carlo study in which they tested the so-called efficiency and coverage of various estimators, inter alia in the case of panels where T > N (our data are characterised by large – or moderate – T and relatively small N; in the case of the whole sample of the euro area members T = 178, while N = 10; in the case of the non-euro M.S.: N = 8 or N = 6, when Sweden and the U.K. are excluded from the sample). The coverage measures the percent of estimated 95% confidence intervals that contain the true value of the coefficient, while the efficiency – the ratio of mean square errors for the respective estimator and O.L.S. The results have been verified and updated by Moundigbaye et al. (Citation2017). The authors suggest that different estimators should be used, depending on their purpose. When the main goal of the research is to test a hypothesis, the author should use the estimator that maximises ‘coverage’, while when the goal is to obtain most accurate estimates – the one that maximises ‘efficiency’. The results of the two studies show that as far as efficiency is concerned, the best estimator is F.G.L.S. (Parks), while in the case of coverage – either P.C.S.E. (Beck and Katz, Citation1995) or O.L.S. with robust errors (heteroscedasticity and cross-sectional dependence). Therefore, when it comes to EquationEquations (3)(3)
(3) and Equation(4)
(4)
(4) , when we want to obtain the best possible estimates of the coefficients, we use the Parks estimator; on the other hand, in EquationEquation (1)
(1)
(1) , where we want to test the significance of various variables
we chose the P.C.S.E. one. For more information about panel data and their estimation we refer the readers to, e.g., Hsiao (Citation2007) or Greene (Citation2008).
Yet another problem appears in the case of EquationEquation (2)(2)
(2) (and in fact also (3)), where lagged dependent variable appears on the right-hand side of the equation. Thus, in the ‘standard’ panel case, the risk of a high Nickel bias appears. However, Kristensen Samii, and Wawro (Citation2011) performed a Monte Carlo study to examine the existence of the Nickel bias as well as other biases in the case of the TSCS of various N and T. They showed that the Nickel bias is already negligible when T > 20. Beck and Katz (Citation2011) present a simulation where they compare three estimators: L.S.D.V., Anderson and Hsiao (Citation1982) and Kiviet (Citation1995) correction. They compare the bias and R.M.S.E., arguing that ‘root mean square error is more important since it incorporates both bias and estimation variability. That is, we might be willing to use a slightly biased estimator that has dramatically smaller sampling variance.’ The authors conclude that the advantage of the Kiviet estimator over L.S.D.V. declines as T gets larger and it is advisable to use L.S.D.V. even for T > 20. Following the advice, we used the Beck–Katz approach in our study.
Our calculations were performed in R, and we used the following packages: plm (Croissant & Millo, Citation2008), (Millo, Citation2017) sandwich (Zeileis, Citation2004) and panelAR (Kashin, Citation2015).
To summarise, when drawing conclusions on the similarities or differences among the euro area and non-euro area MS, we apply panel data estimations. The outcome – based purely on the case-by-case analysis – may be misleading as the estimations for individual states boast different statistical properties. Estimations of expectations’ rationality for a panel of the countries in question can be found in the literature (see Miah, Rahman, & Albinali, Citation2016), but the panel analysis is rarely presented in comparison to the individual country approach.
5. Results and their interpretation
Our analysis commences with the examination of expectational errors ().We refer to median errors and express them in accordance with the formula applied for EquationEquations (1)(1)
(1) and Equation(2)
(2)
(2) . In most cases, mean median errors remain positive, which means that consumers overestimated the future inflation rate. It is not true, however, in the case of the pre-crisis period when inflation rates for all subsamples were, on average, slightly underestimated. The period of low inflation or deflation was registered in the majority of E.U. M.S. during the last sub-period of our analysis. This fact explains why expectation errors had the highest and positive values in this period regardless of the subsample: consumers generally underestimated the drop of inflation. It is visible in the post-crisis eruption period as it covers the time of low inflation or deflation.
Table 1. Expectational errors calculated across countries.
While referring to absolute percentage errors, we capture the lowest errors for the euro area and the highest for the non-euro area M.S., excluding Sweden and the U.K. The differences in accuracy offered by private forecasts in our subsamples are notable. We attribute it to the fact that the euro area subsample is entirely based on developed economies with longer episodes of low inflation and commonly-held monetary policy which did not undergo structural changes during the research period. The level of accuracy of private forecasts was lower in the economies in transition. Except for the obvious interpretation (these economies have different starting point conditions than the developed economies), it should be noticed that their monetary policy was conducted independently and in a less stable way than the E.C.B. policy. It will be commented on in the further sections if this paper.
Finally, regardless of the subsample, median absolute errors reflect the impact of the crisis. They are the lowest in the pre-crisis period and increase substantially in the post-crisis sample. However, as they are the highest for the subsample 2013–2016, we attribute their change not only to the crisis itself but to its longer-run consequences, namely the medium-run low inflation environment.
The next step of our analysis refers to the macroeconomic efficiency tests. They verify whether consumers take into consideration all relevant information available when forming their expectations. The hypothesis of rational expectations assumes that they do. The results of the macroeconomic efficiency tests are shown in . Given that the models based on EquationEquation (2)(2)
(2) had a much higher coefficient of determination, we present only the results of the study referring to them.
Table 2. Macroeconomic efficiency tests.
The macroeconomic efficiency tests suggest that there is difference in acquiring and processing information on the drivers of expectations in our subsamples. The consumers in the euro area process information on inflation drivers less efficiently than the consumers in the non-euro area M.S. The result for broad money is quite surprising, especially for the euro area M.S. where it was ignored: it is the E.C.B. – rather than national central banks – that emphasises monetary factors in its policy. In general, the results of the macroeconomic efficiency tests for both non-euro area subsamples (full one and the one without Sweden and the U.K.) suggest that consumers outside the euro area pay more attention to the drivers of expectations. They take into consideration broad money, exchange rates, unemployment, and oil prices. The fact that in the non-euro area M.S., information on inflation drivers was more properly interpreted in all sub-periods is an argument in favour of the impact of common monetary policy on expectations and their F.L. In the non-euro area subsample consumers (properly) analyse each piece of information, one by one. In the euro area, they are offered the possibility to focus mostly on the policy-maker communication about its future actions without direct analysis of the drivers of expectations. At the same time, the national factors regarding real sphere development which is not directly covered by the E.C.B. mandate (industrial production, unemployment) were interpreted properly more frequently than the common factors (interbank rate, exchange rate and broad money). This is how the problem of dual sourcing of information (national and euro area level) could be solved: the consumers in the euro area find national information more relevant and appropriate. Nonetheless, if their expectations are more accurate than the expectations of the non-euro area consumers, they need to find alternative sources of information about future inflation realisation, and we attribute it to the E.C.B. forward-looking communication.
When the financial crisis erupted, consumers’ interest in acquiring and processing information on inflation drivers when forming their expectations remains the same: the amount of information which was efficiently processed did not increase significantly.
The hybrid specification of expectations delivers results of the F.L. degree in our sample (). We estimated EquationEquations (3)(3)
(3) and Equation(4)
(4)
(4) for our subsamples, but as the properties of EquationEquation (3)
(3)
(3) and their goodness-of-fit were much better we present and interpret the results for EquationEquation (3)
(3)
(3) . The adaptive specification of the backward-looking expectations’ component was much more relevant. More details on estimation results are presented in Appendix 2.
Table 3. Forward-looking component of consumers’ expectations.
As we expected, consumers’ F.L. is reduced – the backward-looking component dominates in all cases – and diversified across our samples and over time. The euro area consumers were most forward-looking regardless of the sub-period. Their pre-crisis F.L. was the lowest in comparison to other sub-periods. It increased for the period that starts after the outburst of the global crisis and it was significantly higher (0.11 vs. 0.22) from 2013. Once again, longer-run consequences of the global financial crisis were important for the change of expectations: the low inflation and deflation period accompanied by the extended unconventional monetary policy measures applied by the E.C.B. (the E.C.B. balance sheet expansion started in mid-2012).
A similar situation occurred in the non-euro M.S.: lower pre-crisis F.L. of consumers was accompanied by its higher degree after the outburst of the crisis. It is true for the entire non-euro area sample and for the subsample that excludes Sweden and the U.K. Contrary to the euro area, post-crisis F.L. was lower than the one captured for post-2008. The difference was also statistically significant after 2013. Thus, we can infer that F.L. is greater in post-crisis subsamples in comparison to the pre-crisis period.
Some of the economies included in the non-euro area subsample also registered a period of low inflation or deflation, but its effect on F.L. was less significant than in the case of the euro area. Consumers switched towards greater F.L., which suggests that they find previous formation patterns of expectations inadequate for turbulent times. At the same time, they made less accurate forecasts. Additionally, it should be noticed that consumers in non-euro area M.S. without Sweden and the U.K. were purely backward-looking in the pre-crisis era. Thus, their F.L. skyrocketed when the pre-crisis and post-crisis period were juxtaposed. Most of these economies just introduced new monetary policy frameworks at the beginning of the twenty-first century and some of them still suffered from high and volatile inflation (Romania, Hungary). Moreover, in some of them the monetary policy focus on the formation of expectations was lower as their central banks chose an alternative regime rather than inflation targeting: the National Bank of Bulgaria carries out its monetary policy under the currency board regime which was a stabilisation tool after the financial crisis in 1996–1997. In Croatia, the rationale behind choosing exchange rate-oriented monetary strategy was related to the exchange rate channel prevalence in the monetary transmission mechanism, especially in the highly euroised economy (Benazić & Rami, Citation2016).
Some central banks of the non-euro area economies have less experience in forward-looking communication. Inflation forecasts are hardly important in the policy of the Bulgarian National Bank and the Croatian National Bank. In Poland, they have been published since 2004, and in Romania – since 2005. Even if there is a group of highly transparent central banks in the non-euro area subsample, the impact of differences in transparency can be captured by a group-specific analysis. Central bank’s minutes reflect individualised approaches to forward-looking analyses in their monetary policy decisions. Each of the central banks places different emphasis to the management of expectations. The lack of coordination may be an important factor affecting the results of the cross-country F.L. analysis.
Finally, we can summarise our results in the following way: we captured differences in the properties of expectations among the euro area and non-euro area M.S. The consumers in the euro area produce more accurate forecasts and they use available information about inflation drivers less efficiently. They disregard monetary policy-related factors as broad money and interest rates. The fact that they interpreted information less properly, but still managed to deliver more accurate forecasts may be attributed to the fact that they do not pay attention to particular inflation drivers, but they asses the E.C.B.’s communication about its policy actions and stance. The euro area consumers are also more forward-looking when compared to the non-euro area M.S. consumers. They benefit from longer episodes of low inflation, so the linkage of past inflation and expectation has already been broken, but they also benefit from common monetary policy held within more stable frameworks than the individual policies of the non-euro economies. Contrary to these favourable conditions, consumers in the non-euro area M.S. survived a more turbulent economic situation inherent to the economies undergoing transition, which in this case constitutes the majority of the non-euro sample. Their economies went through structural changes, institutional preparation for the E.U. accession, and a striking modification of their monetary policy. The consumers in these economies are less forward-looking and before the financial crisis erupted they were purely backward-looking according to cross-country examination. Their backward-lookingness might be attributed to the relatively high inflation experience remembered by economic agents. Thus, their forecasts are less accurate even if they try to capture more information relevant to inflation in the forthcoming periods.
Secondly, our aim was to assess the impact that the crisis exerted on expectations. The study captures the modification of expectational errors after the outburst of the financial crisis and from 2013, and then compares them to the pre-crisis sample results. As the results for the October 2008–2016 sub-period and the 2013–2016 sub-period are divergent, we could observe that expectations are also affected by the most turbulent economic situation. In the post-crisis period, consumers are most forward-looking, which may be the remedy for low inflation figures.
As for the results for the non-euro area sample, a caveat needs to be introduced: the sample is much more diversified than the sample of the euro area states. A cross-country examination offered by the panel data analysis does not reveal individual differences. However, we still think it is reasonable to apply the panel analysis: the same scope of variables is to be analysed in the cross-country approach, while the countries included in the sample have some institutional and economic similarities.
The second caveat that needs to be mentioned here refers to the last, relatively short, sub-period. With the sample that we have at our disposal we can carry out an econometric evaluation, but when the sample is extended, the results require revalidation. But then again, the period of extensively low inflation in the E.U. is over. So, the subsample of 2013–2016 will always represent these extraordinary economic circumstances in Europe.
6. Conclusion
The issue of inflation expectations has reappeared in economic discussions after some extraordinary changes that suggest that their properties might be modified to some extent. The financial crisis renewed the discussion. In this paper, we investigate properties of consumers’ expectations in 18 economies composed of the euro area and non-euro area M.S.. The research suggested that differences do exist between the two groups of on the aggregated level. Properties of expectations also evolved over time.
Generally, the forward-looking component in the hybrid specification of expectations was higher in the M.S. that share common monetary policy. Consumers of the euro area produced more accurate forecasts. Consequently, expectations were more convergent with actual economic performance. We attribute this result to the more coherent economic development in these countries over the last decades and to more coherent monetary policy of the E.C.B., including its forward-looking communication. They are the old E.U. M.S., with the lowest and more stable inflation rates.
We cannot clearly tell which portion of differences in expectations formation between our samples can be attributed to the differences in the economic development; nor can we tell which portion of differences stems from the fact that the euro area economies have common monetary policy and communication at their disposal. We acknowledge the importance of the first factor, but we still see some room for the influence of the E.C.B.’s common and coherent communication and its focus on keeping inflation low on greater F.L. of consumers. Moreover, the E.C.B. may simply do better than other central banks when it comes to managing of economic agents’ expectations. It offers the same general framework of monetary policy and long experience in producing and revealing macroeconomic projections.
Once economic environment becomes more turbulent, the economic agents are more prone to news when forming their forecasts and making economic decisions. Moreover, the post-crisis eruption and post-crisis subsamples cover the period of the lowest inflation and deflation episodes that have been recently registered in Europe. Central banks, including the E.C.B., declared that bringing inflation back to the close-to-target level was crucial. They widely applied additional monetary policy measures. Moreover, in the last decade central banks received the additional mandate of macroprudential policy. Their declaration to give price stability preference has not yet been verified in practice as the conflict of interests between the goal of price stability and financial stability has not occurred yet. Under such circumstances, formation of expectations may be more problematic and very remote from the ‘normal times patterns’.
Disclosure statement
No potential conflict of interest was reported by the authors.
Notes
1 Since we have distinguished different sources of divergences of our subsamples, some of them arising from the fact that the non-euro subsample covers two developed economies and very experienced inflation targeters at the same time (Sweden and the U.K.), we examine the non-euro M.S. as well, excluding Sweden and the U.K.
2 If any M.S. is missing from the sample, it is due to its absence from the surveys on expectations. We excluded the euro area new joiners as they do not fit either of the group in our examination.
3 The non-euro M.S. panel is unbalanced, the shortest T equals 128.
References
- Anderson, T. W., & Hsiao, C. (1982). Formulation and estimation of dynamic models using panel data. Journal of Econometrics, 18(1), 47–82. doi:10.1016/0304-4076(82)90095-1
- Andreou, E., Eminidou, S., & Zachariadis, M. (2016). Inflation expectations and monetary policy in Europe (CEPR Discussion Paper, 11306). London: Center for Economic Policy Research.
- Batchelor, R. A., & Orr, A. B. (1988). Inflation expectations revisited. Economica. New Series, 55(219), 317–331. doi:10.2307/2554010
- Beck, N., & Katz, J. (1995). What to do (and not to do) with time-series cross-section data in comparative politics. American Political Science Review, 89(03), 634–647. doi:10.2307/2082979
- Beck, N., & Katz, J. N. (2011). Modeling dynamics in time-series–cross-section political economy data. Annual Review of Political Science, 14, 331–352. doi:10.1146/annurev-polisci-071510-103222
- Benazić, M., & Rami, J. (2016). Monetary policy and unemployment in Croatia. Economic Research–Ekonomska Istraživanja, 29(1), 1038–1049. doi:10.1080/1331677X.2016.1211955
- Carlson, J. A., & Parkin, J. M. (1975). Inflation expectations. Economica, 42(166), 123–138. doi:10.2307/2553588
- Croissant, Y., & Millo, G. (2008). Panel data econometrics in R: The plm package. Journal of Statistical Software, 27(2), 1–43. doi:10.18637/jss.v027.i02
- Crowe, C., & Meade, E. (2008). Central bank independence and transparency: Evolution and effectiveness. European Journal of Political Economy, 24(4), 763–777. doi: doi:10.1016/j.ejpoleco.2008.06.004
- Dias, F., Duarte, C., & Rua, A. (2010). Inflation expectations in the euro area: Are the consumers rational? Review of World Economics, 146(3), 560–591. doi:10.1007/s10290-010-0058-6
- Dovern, J., & Kenny, G. (2017). The long-term distribution of expected inflation in the euro area: What has changed since the great recession? (EBC Working Paper Series, 1999). Frankfurt am Main: European Central Bank.
- Dräger, L. (2015). Inflation perceptions and expectations in Sweden—Are media reports the missing link? Oxford Bulletin of Economics and Statistics, 77(5), 681–700. doi:10.1111/obes.12078
- Ehrmann, M., Eijffinger, S., & Fratzscher, M. (2012). The role of central bank transparency for guiding private sector forecasts. The Scandinavian Journal of Economics, 114(3), 1018–1052. doi:10.1111/j.1467-9442.2012.01706.x
- Erjavec, N., Lolić, I., & Sorić, P. (2015). How (i)rrational are we? A case of Croatian inflation. Croatian Operational Research Review CRORR, 6(1), 241–253. doi:10.17535/crorr.2015.0019
- European Commission (2016). The joint harmonized EU programme of business and consumer surveys. User Guide. Retrieved fromhttp://ec.europa.eu/economy_finance/db_indicators/surveys/documents/bcs_user_guide_en.pdf
- Fukač, M. (2010). Heterogeneous expectations, adaptive learning, and forward-looking monetary policy. In P. Sinklar (ed.), Inflation expectations (pp. 62–75). London: Routledge.
- Geraats, P. M. (2013). Transparency, flexibility, and macroeconomic stabilization. In: P. L., Siklos, & J.-E., Sturm (Eds.), Central Bank communication, decision making, and governance: Issues, challenges, and case studies (pp. 49–82). Cambridge MA: The MIT Press.
- Geraats, P. M. (2014). Monetary policy transparency. In J., Forssbaeck, & L., Oxelheim (Eds.), The Oxford handbook of economic and institutional transparency (pp. 111–152). Oxford: Oxford University Press.
- Gerberding, C. (2001). The information content of survey data on expected price development for monetary policy (Deutsche Bundesbank Discussion Paper, 9). Frankfurt am Main: Deutsche Bundesbank.
- Greene, W. H. (2008). Econometric analysis. Upper Saddle River, N.J: Prentice Hall.
- Heinemann, F., & Ullrich, K. (2006). The impact of EMU on inflation expectations. Open Economies Review, 17(2), 175–195. doi:10.1007/s11079-006-6809-2
- Hsiao, C. (2007). Panel data analysis—Advantages and challenges. Test, 16(1), 1–22. doi:10.1007/s11749-007-0046-x
- Hubert, P. (2015a). Do central bank forecasts influence private agents forecasting performance vs. signals? Journal of Money, Credit and Banking, 47(4), 771–789. doi:10.1111/jmcb.12227
- Hubert, P. (2015b). The influence and policy signalling role of FOMC forecasts. Oxford Bulletin of Economics and Statistics, 75(5), 655–680. doi:10.1111/obes.12093
- Kashin, K. (2015). PanelAR: Estimation of linear AR(1) panel data models with cross-sectional heteroscedasticity and/or correlation. Retrieved fromhttps://cran.r-project.org/web/packages/panelAR/index.html
- Kiviet, J. F. (1995). On bias, inconsistency, and efficiency of various estimators in dynamic panel data models, Journal of Econometrics, 68(1), 53–78. doi:10.1016/0304-4076(94)01643-E
- Kokoszczyński, R., Łyziak, T., & Stanisławska, E. (2010). Consumer inflation expectations: Usefulness of survey-based measures—A cross-country survey. In P. Sinclair (ed.), Inflation expectations (pp. 76–100). Oxford: Routledge.
- Kristensen, I. P., Samii, C., & Wawro, G. J. (2011). On the use of fixed effect estimators for time series cross-section data. Unpublished draft. Retrieved fromhttp://cyrussamii.com/wp-content/uploads/2013/08/kristensen_etal.pdf.
- Łyziak, T. (2009). Measuring consumer inflation expectations in Europe and examining their forward-lookingness (MPRA Paper, 18890). Munich: Personal RePEc Archive.
- Łyziak, T. (2010). Measurement of perceived and expected inflation on the basis of consumers survey data (Irving Fisher Committee on Central Bank Statistic Working Papers, 5). Basel: Bank for International Settlements.
- Łyziak, T. (2013). Formation of inflation expectations by different economic agents. The case of Poland. Eastern European Economics, 51(6), 5–33. doi:10.2753/EEE0012-8775510601
- Łyziak, T., & Mackiewicz-Łyziak, J. (2014). Do consumers in Europe anticipate future inflation? Has it changed since the beginning of the financial crisis? Eastern European Economics, 52(3), 5–32. doi:10.2753/EEE0012-8775520301
- Moundigbaye, M., Rea, W. S., & Reed, R. W. (2017). Which panel data estimator should I use?: A corrigendum and extension. Economics: The Open-Access, Open-Assessment E-Journal, 12(2018-4), 1–31. http://dx.doi.org/10.5018/economics-ejournal.ja.2018-4.
- Miah, F., Rahman, S., & Albinali, K. (2016). Rationality of survey based inflation expectations: A study of 18 emerging economies’ inflation forecasts. Research in International Business and Finance, 36, 158–166. doi: doi:10.1016/j.ribaf.2015.09.029
- Millo, G. (2017). Robust standard error estimators for panel models: A unifying approach. Journal of Statistical Software, 82(3), 1–27. doi:10.18637/jss.v082.i03
- Mitchell, J., & Weale, M. (2007). The rationality and reliability of expectations reported by British households: Micro evidence from the British Household Panel Survey. Deutsche Bundesbank Discussion Paper Series 1: Economic Studies, 19. Frankfurt am Main: Deutsche Bundesbank.
- Parks, R. (1967). Efficient estimation of a system of regression equations when disturbances are both serially and contemporaneously correlated. Journal of the American Statistical Association, 62(318), 500–509. doi:10.2307/2283977
- Reed, R. W., & Ye, H. (2011). Which panel data estimator should I use? Applied Economics, 43(8), 985–1000. doi:10.1080/00036840802600087
- Reed, R. W., & Webb, R. (2010). The PSE estimator is good—Just not as good as you think (Department of Economics and Finance Working Papers in Economics, 10/53). Christchurch: University of Canterbury.
- Skořepa, M., & Kotlán, V. (2003). Assessing future inflation in inflation targeting: Forecasts or simulations. In Monetary policy in changing environment (pp. 147–157). Bank for International Settlements Paper No. 19. Basel: Bank for International Settlements.
- Zeileis, A. (2004). Econometric computing with HC and HAC covariance matrix estimators. Journal of Statistical Software, 11(10), 1–17. http://www.jstatsoft.org/v11/i10/.
Appendix 1
Model specification and estimation
There are two alternatives of the hybrid specification of expectations Equation(3)(1)
(1) and Equation(4)
(2)
(2) :
(3)
(3)
(4)
(4)
We estimate them using panel methods. The equation Equation(4)(2)
(2) is estimated as follows. As the parameter
is present in two expressions, we re-organize the equation:
Thus, the problem is reduced to estimation of the equation of the type:
Model Equation(3)(1)
(1) has been reduced to the following form:
and estimated as:
In the panel notation we have:
where:
.
Appendix 2
Full model estimations
1. Estimates of model Equation(3)(1)
(1) - euro area, whole period
Table
2. Estimates of model Equation(3)(1)
(1) - euro area, pre-crisis
Table
3. Estimates of model Equation(3)(1)
(1) - euro area, post 2008
Table
4. Estimates of model Equation(3)(1)
(1) - euro area, post 2013
Table
5. Estimates of model Equation(3)(1)
(1) – non-euro area, reduced (excluding Sweden and UK), whole period
Table
6. Estimates of model Equation(3)(1)
(1) – non-euro area, reduced (excluding Sweden and UK), pre-crisis
Table
7. Estimates of model Equation(3)(1)
(1) – non-euro area, reduced (excluding Sweden and UK), post 2008
Table
8. Estimates of model Equation(3)(1)
(1) – non-euro area, reduced (excluding Sweden and UK), post 2013
Table
9. Estimates of model Equation(3)(1)
(1) - non-euro area, whole period
Table
10. Estimates of model Equation(3)(1)
(1) – non-euro area, pre-crisis
Table
11. Estimates of model Equation(3)(1)
(1) – non-euro area, post 2008
Table
12. Estimates of model Equation(3)(1)
(1) – non-euro area, post 2013
Table