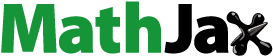
Abstract
The evaluation of sustainable rural tourism potential is a key work in sustainable rural tourism development. Due to the complexity of the rural tourism development situation and the limited cognition of people, most of the assessment problems for sustainable rural tourism potential are highly uncertain, which brings challenges to the characterisation and measurement of evaluation information. Besides, decision-makers (DMs) usually do not exhibit complete rationality in the practical evaluation process. To tackle such problems, this paper proposes a new behaviour multi-attribute group decision-making (MAGDM) method with probabilistic linguistic terms sets (PLTSs) by integrating Wasserstein distance measure into TODIM (an acronym in Portuguese of interactive and multicriteria decision making) method. Firstly, a new Wasserstein-based distance measure with PLTSs is defined, and some properties of the proposed distance are developed. Secondly, based on the correlation coefficient among attributes and standard deviation of each attribute, an attribute weight determination method (called PL-CRITIC method) is proposed. Subsequently, a Wasserstein distance-based probabilistic linguistic TODIM method is developed. Finally, the proposed method is applied to the evaluation of sustainable rural tourism potential, along with sensitivity and comparative analyses, as a means of illustrating the effectiveness and advantages of the new method.
1. Introduction
Rural tourism refers to a type of tourism that takes rural communities as activities and takes rural unique production forms, lifestyles, and idyllic scenery as objects (Lane, Citation1994). In recent years, under the joint promotion of policies and the market, China's rural tourism has developed vigorously, which has greatly promoted the increase in output and income of farmers, diversified agricultural operations, and beautiful and prosperous rural areas (Su, Citation2011). The sustainable development of rural tourism has become an important force in promoting rural revitalisation (Gao & Wu, Citation2017; Griesiene & Georgeta, Citation2017; McAreavey & McDonagh, Citation2011). Nowadays, especially in China, the sustainable development of rural tourism directly affected regional economics and management. For the government, it is very important to identify sustainable rural tourism regions with higher potential and extend their successful experiences to other regions. For the investors, to make investment decisions in rural tourism, they must first determine the potential of rural tourism. As a result, the evaluation of the sustainable rural tourism potential is not only an effective way to analyse its constraints, but also provides some decision-making reference for rural tourism investors. To this end, how to evaluate the potential of sustainable rural tourism has become an important issue that needs to be focussed on in the study of sustainable rural tourism development (Puška et al., Citation2019; Trukhachev, Citation2015). However, due to the complexity of the rural tourism development situation and the limited cognition of people, certain challenges are implied in evaluating the potential of sustainable rural tourism. The main challenges include: (a) how to characterise highly uncertain evaluation information; (b) how to construct reasonable evaluation indicators; and (c) how to establish effective evaluation methods. Since each settlement of rural tourism has distinguished properties than the others with respect to criteria and decision‐makers (DMs) may express the different standpoints regarding them, to some extent, we can declare that the selection of sustainable rural tourism with the highest potential has been generally formulated and addressed as a multi-attribute group decision-making (MAGDM) with highly uncertain information.
In the actual multi-attribute decision-making (MADM), the decision-maker (DM) is more inclined to use linguistic terms to evaluate alternatives (Herrera & Herrera-Viedma, Citation2000). For example, when the DM evaluates sustainable rural tourism potential, he or she is accustomed to expressing personal preference in the form of linguistic terms such as ‘very high’, ‘high’, ‘medium’ and ‘low’ instead of crisp numbers for qualitative indicators related to potentials of environment, economy, society, and management. Since the concept of linguistic variable was first proposed by Zadeh (Citation1975) in 1975, due to the needs of different decision-making environments, complex linguistic expressions in different forms of structure are employed to simulate people’s cognition and judgement (Wang et al., Citation2018; Zhang, Zhu, et al., Citation2017). Among them, hesitant fuzzy linguistic term set (HFLTS) (Rodriguez et al., Citation2012), as a new qualitative decision tool, allows the DM to express his or her preference in several different linguistic terms at the same time, which accords with the uncertainty and hesitation of human perception and judgement (Wu & Xu, Citation2016). In an HFLTS, all possible linguistic terms have equal weight or importance degree. However, different preferences over different linguistic terms may occur in many practical situations. For example, 50% of DMs think that the sustainable potential of the area is ‘high’, 30% sure it is ‘medium’, only 10% believe that it is ‘slightly high’, and others do not express any opinions. In this case, the obtained information for the above evaluation of the sustainable rural tourism potential can be summarised as follows: {(high, 0.5), (slightly high, 0.1), (medium, 0.3)}. To facilitate the fast and efficient processing of this type of linguistic evaluation information provided by multiple DMs or experts in the actual decision-making process, Pang et al. (Citation2016) defined probabilistic linguistic term sets (PLTSs) and then put forward some basic operational laws for PLTSs. The PLTSs-based expression not only enriches the way in which DMs express qualitative evaluation information (Lei et al., Citation2020), but also improves the accuracy of information processing by adding probabilities without loss of any original linguistic information (Xu et al., Citation2019).
To accurately measure the information difference between probabilistic linguistic evaluations is the premise and basis for reasonably comparing two PLTSs and effectively determining weights of attributes with PLTSs. To do this, some scholars have devoted themselves to the research on information measures between two PLTSs. Among them, the research on the distance measures between two PLTSs has occupied an important position due to the simplicity and practicality of distance metrics (Lin et al., Citation2019; Mao et al., Citation2019; Wu & Liao, Citation2019; Zhang, Xu, et al., Citation2016, Citation2017). However, with the deepening of relevant research, several open issues of PLTSs-based distance measures need to be resolved. First, to some extent, adding linguistic terms in the normalising process may affect the distance value, and the initially useful information may be ignored for the added linguistic terms with zero probability. Second, it is difficult for the existing distance measure to satisfy the properties of the triangle inequality, which may lead to the unrealistic situation (e.g., the distance between two different PLTSs can be equal to zero). Therefore, some of the existing distance measures between two PLTSs still need to be further improved, which implies that a new PLTSs-based distance measure for making up the above deficiencies is an imminent need.
With the increasing complexity of decision-making environments and the limitations of human cognition, it is hard for a single DM to consider all relevant aspects or attributes of decision-making (Saaty & Peniwati, Citation2013). MAGDM is that a group of DMs evaluate various alternatives under multiple attributes, which has been widely concerned by researchers in different fields. In recent years, a wealth of research results concerned with PLTSs indicates that it has become a research hotspot in the field of MADM (Liao et al., Citation2020). Especially, probabilistic linguistic MAGDM, combining PLTSs-based expression and collective decision wisdom, has attracted the attention of some scholars. Related research focuses on the operations of PLTSs (Gou & Xu, Citation2016; Li, Liu, & Wei, Citation2020; Mao et al., Citation2019), the comparisons of PLTSs (Bai et al., Citation2017; Feng et al., Citation2019; Pang et al., Citation2016), the weight determination of attributes with PLTSs (Lei et al., Citation2020; Wang, Wang, et al., Citation2019; Wei et al., Citation2019), and the probabilistic linguistic MAGDM approaches (He et al., Citation2019; Lu et al., Citation2019; Wei, Lu, et al., Citation2020).
In order to make a reasonable and practicable decision-making, many efforts to explore decision-making approaches have been made in the past decades (Alinezhad & Khalili, Citation2019). Among them, most of approaches based on the expected utility theory have been developed to analyse and solve diverse decision-making problems. These approaches usually assume that DMs have the behaviour characteristics of ‘complete rationality’. However, it is difficult for DMs to be completely rational in the actual decision-making process. DMs usually have the behaviour characteristics of ‘limited rationality’ when faced with uncertain decision-making situations, where their psychological behaviours have distinct influence on their choices (Li, Liu, Yang, et al., Citation2020; Ma et al., Citation2021). Accordingly, it is necessary to develop some behaviour decision-making methods that consider DMs’ different psychological behaviours by utilising some behavioural decision theories such as prospect theory, cumulative prospect theory, regret theory, disappointment theory, and fairness theory (Liu et al., Citation2020; Zhang, Zhu, et al., Citation2016). Especially, the TODIM (an acronym in Portuguese of interactive and multicriteria decision making) method, derived from the prospect theory (Tversky & Kahneman, Citation1992), has become an effective tool to handle the uncertain multiple attribute decision-making problem based on the modern behaviour decision theories (Gomes & Lima, Citation1992). In essence, it captures the loss and gain under uncertainty from the view of reference point and the DM is more sensitive to the loss (Qin et al., Citation2017). Therefore, compared with the existing behaviour decision methods, the main advantage of the TODIM method is to consider the limited rational behaviour characteristics (Wei et al., Citation2015). That is, the DMs seek the maximisation of the value function in the context of bounded rationality decision rather than the maximisation of utility under the complete rationality decision. In view of the above advantages of the TODIM method, recently, scholars have applied it to different decision fields (Llamazares, Citation2018). For instance, the TODIM method has been used to rank the pollution potential of industries (Soni et al., Citation2016), to assess cleaner production for gold mines (Liang et al., Citation2018), and to rank products with online reviews (Zhang et al., Citation2020). Furthermore, it is also worth noting that the TODIM method has been extended to solve the MADM problems with PLTSs (Wang, Liu, et al., Citation2019; Liu & You, Citation2017; Wang, Liu, et al., Citation2019; Zhang et al., Citation2019). The above results greatly promote the study of behavioural multiple attribute decision making in uncertain environments.
Nevertheless, so far, there exist the following some deficiencies for MAGDM with PLTSs.
The existing distance measures only extend the traditional measure to the PLTSs-based decision environment while failure to fully exploit the characteristics of PLTSs. Moreover, most of them are difficult to satisfy all the axioms of the distance, which may cause the unreasonable results (see Section 5.5.1 for details).
In the traditional PLTSs-based MAGDM, one usually needs to add some artificial linguistic terms to facilitate the processing of probabilistic linguistic information such as comparison operation of PLTSs. However, the adding operation may lead to the loss of original information.
In the existing PLTSs-based TODIM method, the attributes with PLTSs are usually assumed to be independent each other. Nevertheless, correlation of attributes often exists in a real decision environment. Therefore, it is necessary to construct an attribute weight determination method, where the correlation between attributes is taken into consideration.
Based on the assumption that the DMs are completely rational, most of the existing MAGDM methods with probabilistic linguistic information were proposed. However, there are no DMs with complete rationality in practice decision making problems. Therefore, it is necessary to develop a new behaviour decision method for solving MAGDM with PLTSs that considers the psychological behaviour of DMs.
Given the defects of the existing research, this paper aims to propose a new behaviour decision method for solving MAGDM with PLTSs by integrating Wasserstein distance measure into the probabilistic linguistic TODIM, which is applied to the evaluation of sustainable rural tourism potential. The main contributions and innovations of this paper are as follows.
A new Wasserstein-based distance measure between two PLTSs is developed to make up for the shortcomings of the existing distance measure. In addition, our proposed distance measure satisfies all the axioms of the distance especially including the property of the triangle inequality, which is difficult to be involved in the existing distance measures.
Considering both contrast intensity and conflict of attributes which are contained in the decision information of probabilistic linguistic MAGDM problems, we propose an objective determination method of attribute weights (called PL-CRITIC method).
The proposed Wasserstein distance-based extended PL-TODIM method reduces the manual processing of decision information, namely, neither needs to unify PLTS to the same length nor needs to rearrange PLTS in some order, which greatly improves the processing efficiency of complex decision information. Besides, comprehensively considering the interaction between attributes and the psychological behaviours of DMs, we can obtain more reasonable and reliable results by the proposed method than by the existing method.
The effectiveness of the proposed method is demonstrated by a real case on the evaluation of sustainable rural tourism potential. In addition, the advantages of the proposed method are verified by comparative analysis with other MAGDM methods.
The paper is structured as follows. In Section 2, we introduce the basic content of LTS, PLTS, Wasserstein distance and classical TODIM method. In Section 3, we propose a Wasserstein-based distance measure between PLTSs. In Section 4, the Wasserstein distance-based extended PL-TODIM method is proposed to solve the MAGDM problems. Section 5 provides the application of the proposed method to a real case on the evaluation of sustainable rural tourism potential and comparative analysis is also conducted. Conclusion remarks are offered in Section 6.
2. Preliminaries
In this section, we first review some basic concepts of linguistic term set (LTS) and PLTS.
2.1. Basic concepts
Definition 1
(Herrera & Herrera-Viedma, Citation2000). Let be a subscript-symmetric ordinal LTS, where
is a positive integer,
represents the assessment of ‘indifference’ or ‘fair’, and the remaining linguistic terms are symmetrically distributed around
in ascending order of intensity.
satisfies the following conditions:
The set is ordered:
iff
;
The negation operator is defined as:
For example, the LTS with seven granularities could be:
Definition 2
(Gou et al., Citation2017). Let be a subscript-symmetric ordinal LTS. Then, the equivalent membership degree
of the linguistic term
is obtained with the following transformation function
(1)
(1)
Definition 3
(Pang et al., Citation2016). Let be a subscript-symmetric ordinal LTS. The PLTS can be expressed by
(2)
(2)
where
denotes the k-th linguistic term
with the probability
is the number of all different LTS in
and the linguistic terms
are arranged according to the values of
in descending order. Here, the expression
indicates that the DM, due to his/her lack knowledge, cannot provide complete assessment information, resulting in the absence of partial probability information. Therefore, the original information should be normalised. The normalisation method of PLTS is defined in the following definition 4.
Definition 4
(Pang et al., Citation2016). Let be two PLTSs, then two steps to normalising
are shown in below.
Step 1: Given a PLTS with
the normalised PLTS
is defined as
(3)
(3)
where
Step 2: If when
then we can add
linguistic terms to
so that the numbers of linguistic terms in
and
are identical. The added linguistic terms are the smallest one in
and their probabilities are zero.
Definition 5
(Wu et al., Citation2018). Given a PLTS based on
and
be the subscript of linguistic term
Then the expected value function of
is expressed as
(4)
(4)
and the variance value function of
is defined as follows:
(5)
(5)
where
is the expected value of
For two PLTSs and
if
then we can say that
is superior to
denoted as
Similarly, if
then
is inferior to
denoted as
However, for two
and
with
if
then
is superior to
denoted as
if
then
is superior to
denoted as
if
then
is indifferent to
denoted as
2.2. Wasserstein distance
Wasserstein distance (Lipman et al., Citation2013), also known as earth mover's distance (EMD), is a method for measuring histogram difference, which was first introduced into the field of computer vision. Wasserstein distance has also a long history in probability theory and mathematical statistics, and it is a classical method to measure the difference between probability distributions. Its physical and economic significance is to solve the minimum consumption of transportation problem of moving one probability distribution to another. At present, some scholars have extended Wasserstein distance from pure mathematics to other fields such as medical image and biological data analysis (Oh et al., Citation2020; Ran et al., Citation2019).
Definition 6
(Villani, Citation2008). Let be a Polish metric space (a space homeomorphic to a complete metric space that has a countable dense subset), and let
for any two probability measures
on
the Wasserstein distance of order
between
and
is defined by the formula
(6)
(6)
where
denotes the collection of all measures on
with marginals
and
on the first and second factors respectively. The set
is also called the set of all couplings of
and
indicates the expected value of a random variable and the infimum is taken over all joint distributions of the random variables
and
with marginals
and
respectively. To simplify the calculation, in this study we suppose
Remark 1.
Especially, in one dimension, we can suggest some special cases where we can solve the optimal transport problem (i.e., EquationEquation (6)(6)
(6) ) with a finite number of variables (Solomon, Citation2020). For example, given two discrete probability measures
on
it is a linear program solvable using many classic algorithms, such as the simplex or interior. In the technical literature, this setup is referred to as fully discrete optimal transport. To take another example, suppose the probability measures
on
is a superposition of
measures and the other probability measures
is absolutely continuous. It corresponds to transporting from a distribution with mass concentrated at a few points to a distribution with a smoother distribution. In the technical literature, this setup is known as semidiscrete optimal transport. illustrates these two cases, respectively.
2.3. Classical TODIM method
The TODIM approach is a useful behaviour decision technique to solve MAGDM problems based on prospect theory (Tversky & Kahneman, Citation1992), which can capture the psychological behaviour of decision-makers in the process of real decision-making. In the classical TODIM method (Gomes & Lima, Citation1992), the prospect value function is first constructed to measure the dominance degree of each alternative over the others, which reflects the behavioural characteristics of DMs’ reference dependence and loss aversion, and then the alternatives are ranked according to the overall dominance degrees of each alternative.
For the convenience of the subsequent description, let
be the set of
alternatives, and
be the set of
attributes. A decision matrix is denoted by
where
are crisp numbers. Then, the basic steps of classical TODIM method are listed below (Gomes & Lima, Citation1992; Qin et al., Citation2017):
Step 1. Calculate the relative weight of the attributes
to the reference attribute
where
is the weight of the attribute
and
Step 2. Compute the dominance degree of each alternative over the alternative
using the following expression:
where the parameter
indicates the attention factor of the losses. Different choices of
lead to different shapes of the prospect theoretical value function. The range of the parameter values is
>0. If 0<
<1, then the influence of loss will increase; if
>1, then the influence of loss will decrease.
Step 3. Obtain the overall dominance degree of each alternative over each alternative
with respect to attribute
by the following formula
Step 4. Get the overall prospect value of the alternative
by the following expression:
Step 5. Rank all the alternatives by their overall prospect values The higher the value of
the better the alternative
3. Wasserstein-based distance measure between PLTSs
Definition 7.
Let and
be two PLTSs with
(
) on the linguistic evaluation scale
The corresponding distributions are defined as
and
Then, the Wasserstein distance between two PLTSs can be defined as follows
(7)
(7)
where
represents the set of joint distribution
of
and
and
indicates the cost for transporting one unit of mass from
to
EquationEquation (7)
(7)
(7) aims at pursuing the infimum of the expectation of random variable function
Intuitively, if each distribution is viewed as a unit amount of ‘soil’, the PLTSs-based Wasserstein distance is the minimum ‘cost’ of turning one pile soil into the other, which is assumed that the soil cost per unit to be moved must be multiplied by the distance to be moved.
In mathematics, one way to understand the motivation of the Definition 7 is to consider the fully discrete optimal transport problem. That is, for a discrete probability distribution of mass on a space
we wish to transport the mass in such a way that it is transformed into a discrete probability distribution
on the same space
If the distributions are interpreted as different ways of piling up a certain amount of soil over the space
the PLTSs-based Wasserstein distance is the minimum transportation cost of all the moving ways that we turn one pile soil into the other, where the cost is assumed to be amount of dirt moved times the distance by which it is moved.
Given the cost function where
is the equivalent transformation function of linguistic terms in Definition 2, we can obtain the unit transportation cost
between any two linguistic terms
in
and
in
(8)
(8)
Then, the Wasserstein distance between two PLTSs in EquationEquation (7)(7)
(7) can be converted to the following optimisation model (M1):
(M1)
In what follows, some basic properties of the PLTSs-based Wasserstein distance can be obtained.
Property 1. The proposed distance between and
satisfies:
1)
2)
3)
4)
Proof.
The proof of Property 1 is provided in Section A.1 of the Appendix.
From Property 1, we can find that satisfies all the axioms of distance. Therefore, it is really a distance measure, which can accurately measure the information difference of any two PLTSs. Below we use an example to illustrate its simple application.
Example 1.
Suppose that five experts are invited to form a group of experts to evaluate the sustainable development potential of rural tourism in two areas A and B. Under the environment factor indicator, their evaluation information for area A is collected as {medium, slightly high, slightly high. Slightly high, medium} while the one for area B is collected as {medium, medium, very high, medium, medium}. Below we can use the proposed distance formula to give the specific difference between the two evaluation values for area A and area B.
First, according to Definition 1 and Definition 3, the group’s evaluation information in the form of PLTS for area A and area B is expressed by
respectively. Also, based on Definition 2, the equivalent transformation function of linguistic terms is denoted as
For example,
Then, after solving the model M1 that is equivalent to a balanced transportation problem by Microsoft Excel solver or LINGO (Winston & Goldberg, Citation2004), we can easily obtain Wasserstein distance between
and
However, if the distance formulas proposed by Pang et al. (Citation2016) and Wang, Wang, et al. (Citation2019) are utilised, the obtained distances between
and
are both equal to zero, which is contrary to our intuition.
4. Wasserstein distance-based probabilistic linguistic TODIM method
4.1. Problem description
Because there are intrinsic uncertainties related to the reliability of information about the sustainable rural tourism potential of settlements or areas, we use LTSs to express experts’ opinions. Thus, aggregated information from all relevant experts can be described as PLTSs. According to the introduction, it is noted that the problem of evaluating the potential for sustainable rural tourism essentially can be regarded as a MAGDM problem with probabilistic linguistic information in this section. Here, PLTSs will be used as an effective tool to simulate highly uncertain assessment information. The specific formal description of the problem is as follows.
Let be a subscript-symmetric LTS,
be a finite set of m alternatives, and
be a set of n attributes, where
and
represent the benefit attribute and cost attribute sets, respectively. The attribute’s weight vector is denoted as
such that
and
Due to the complexity and uncertainty of the real problems, the information about the attributes weight is completely unknow. The collective evaluation values given by DMs for alternative
with respect to the attribute
is denoted as
(characterised by PLTSs).
is called a probabilistic linguistic decision matrix (PLDM) of the DMs over
alternatives with respect to
attributes.
4.2. PL-CRITIC method for calculating the attribute weights
Attribute weight assignment plays an important role in multi-attribute decision making. For the MAGDM problems where the attribute weights information is completely unknow or incomplete, we first need to determine the attribute weights in advance (Lei et al., Citation2019). In general, the approaches to determining the weights of criteria mainly include three categories (i.e., subjective weight-determining, objective weight-determining, and combinative weight-determining) (Alemi-Ardakani et al., Citation2016). Sometimes, as various reasons (e.g., lack of knowledge and data, and the avoidance of personal judgement biases towards attributes) are involved, the DM may be reluctant to provide subjective judgments about attributes (Fu et al., Citation2018). Therefore, objective weight-determining methods are often favoured by DMs. The classical CRITIC (Criteria Importance Through Intercriteria Correlation) method as an objective weight-determining method, which are employed to extract statistical (unbiased) weights through dispersion analyses of a given decision matrix, was proposed by Diakoulaki et al. (Citation1995). When using CRITIC to excavate the potential information of attributes, the contrast intensity of each attribute and the conflict between the attributes (they are measured by the standard deviation and the correlation coefficient, respectively), are synthetically considered (Peng et al., Citation2019; Wei, Lei, et al., Citation2020).
In this section, motivated by the classical CRITIC, a probabilistic linguistic CRITIC (PL-CRITIC) method for solving objective weights of attributes is proposed firstly. In PL-CRITIC method, the standard deviation of attributes is used to represent the difference between the same attributes in different alternatives. Based on the correlation coefficients between attributes, a quantitative expression that Wasserstein-based distance measure between PLTSs is integrated with is constructed to represent the conflict between attributes with PLTSs. In what follows, based on the above idea, we give the specific steps for determining attribute weights.
First, using EquationEquation (3)(3)
(3) in Definition 4, the normalisation of the PLDM
is denoted as
With the help of comparison rules of PLTSs (see Definition 5), the positive-ideal solution (PIS) for attribute
(
) can be defined as:
(9)
(9)
Meanwhile, the negative-ideal solution (NIS) for attribute (
) can be defined as
(10)
(10)
Then, for attribute we can obtain the Wasserstein distance between PIS and the evaluation value of alternative
(11)
(11)
and the Wasserstein distance between PIS and NIS for attribute
(12)
(12)
Thus, the correlation coefficient between -th and
-th attributes can be calculated as follows:
(13)
(13)
where
Also, the standard deviation of each attribute is determined by the following EquationEquation (14)(14)
(14) .
(14)
(14)
According to the idea of classical CRITIC method, the information contained in each attribute is calculated, as shown in EquationEquation (15)(15)
(15) .
(15)
(15)
where
reflects the overall conflict between the
-th attribute and other attributes.
Based on the above analysis, the larger the value the larger the amount of information transmitted by the corresponding attribute. As a result, the relative importance of the criteria
is greater with the increasing value
The objective weight of the attribute is determined by the following equation.
(16)
(16)
4.3. PL-TODIM method based on Wasserstein distance
In this section, by integrating Wasserstein-based distance measure between PLTSs, PL-CRITIC and PL-TODIM method, a new method (called Wasserstein-based PL-TODIM method) to handle MAGDM problems with probabilistic linguistic information is developed. We summarise its procedure as a flowchart shown in .
Step 1. Identify all the alternatives to be evaluated, and the evaluation attributes. Determine the subscript-symmetric ordinal LTS the alternatives set
and the attributes set
Construct the group PLDM
by aggregating the original individual linguistic decision matrix (LDM) directly, and then determine the equivalent PLDM
where
(17)
(17)
In EquationEquation (17)(17)
(17) ,
represents negative operator, that is
(18)
(18)
where
is the subscript of the LTS
Step 2. Compute the normalised decision matrix according to EquationEquation (3)
(3)
(3) in Definition 4.
Step 3. Determine the PIS and the NIS
of attribute
by EquationEquations (9)
(9)
(9) and (10), respectively. Using EquationEquations (11)
(11)
(11) and Equation(12)
(12)
(12) , obtain the values of
and
respectively.
Step 4. Calculate the weight vector by EquationEquations (13)–(16).
Step 5. Obtain the relative weight of the criterion
to reference point
by
(19)
(19)
where
Step 6. Calculate the dominance degree of alternative over alternative
with respect to attribute
using the following expression:
(20)
(20)
where the comparison between
and
is computed by Definition 5 (See Section 2).
Step 7. Compute the overall dominance degree of alternative over alternative
in the following form
(21)
(21)
Step 8. Get the overall prospect value of alternative
(
) by the following expression
(22)
(22)
Step 9. Rank the alternatives according to the overall prospect value
and select the optimal alternative.
Step 10. End.
Remark 2.
Compared with the decision procedure of the classical TODIM method, the above proposed Wasserstein-based PL-TODIM procedure is characterised by the following three aspects. (a) Simplify the normalised processing of PLDM (see Step 2 in detail). (b) Determine the attribute weights by integrating a new Wasserstein-based distance measure into PL-CRITIC method (see Step 4 in detail). (c) Construct a Wasserstein-based PL-TODIM method to rank the alternatives (see Step 6 in detail).
5. Application to the evaluation of sustainable rural tourism potential
In this section, we further illustrate the practicality of the proposed method by a real case on the evaluation of sustainable rural tourism potential. In addition, sensitivity analysis is carried out on the psychological behaviour parameters of DMs to explore the influence on the decision results. Finally, some comparisons are conducted to verify the effectiveness and advantages of the proposed method.
5.1. Case study
Rural tourism is an important part of rural industrial development in the new period, new background and new conditions, and an important focal point for promoting rural economic development. In recent years, in order to thoroughly implement the strategic plan for rural revitalisation, promote the quality and efficiency of rural tourism, promote the sustainable development of rural tourism, and accelerate the formation of new kinetic energy for agricultural and rural development, China's Ministry of Culture and Tourism, the National Development and Reform Commission and 17 other departments issued the ‘Guiding Opinions on Promoting the Sustainable Development of Rural Tourism’ on November 15, 2018 (http://zwgk.mct.gov.cn/auto255/201812/t20181211_836468.html). Since then, China and its local governments at all levels attach great importance to the sustainable development of rural tourism. According to Ning Zhizhong, chief planner of the Tourism Research and Planning and Design Centre of the Chinese Academy of Sciences, the size of the rural tourism market will continue to expand as China's urbanisation level continues to increase. Approximately 70 percent of China's tourism resources are distributed in the countryside, with huge potential for development. This section is focussed on the selection of sustainable rural tourism with the highest potential from several rural settlements or alternatives, which is convenient to both make the next tourism policy for the governments and provide some investment reference for rural tourism investors.
Currently, there are three rural settlements to be evaluated in the rural areas of Xuancheng District, Anhui Province, China (
Chaji Village, Taohuatan Township, Jing County;
Taoyuan Village, Taiji Cave Scenic Area, Guangde County;
Qianqiu Village, Yuntai Township, Ningguo City), which make up a sample of three alternatives described in .
To assess the sustainable tourist potential of the above three rural settlements, a group of five experts from different backgrounds and professional fields are invited. The five experts were appointed in cooperation with the Government of Xuancheng District. First, we identified the list of potential experts, which was the basis for the selection of the experts. To conduct this study, five experts were appointed who visited the selected areas. In addition, the five experts presented and used all the information on these areas owned by the Government of Xuancheng District. On this basis, the five experts evaluated the sustainable rural tourism potential for three rural settlements of Xuancheng District. The group of five experts need to compare the three settlements or alternatives in order to select the most potential alternative(s) and rank them in terms of their potential strength. Based on the principle of index construction, referring to the literature related to the tourism evaluation index system, the national standard evaluation criteria of tourism resources, and combining the actual situation of rural tourism development in Anhui Province, the potential evaluation indicators from four factor dimensions of environment, economy, society, and management are designed. The four decision attributes or indicators
is detailly explained in the following. Also, suppose that the weight vector of the attributes is unknown completely.
Environment factor (): It mainly includes ecological environment and tourism resources, focussing on (1) the quality of water bodies, space and sound environment; forest cover, waste disposal rate, tourist climate comfort period, the harmony between construction projects and the surrounding landscape; and (2) rural landscape accessibility, rural landscape singularity, rural landscape authenticity, the scale and abundance of rural tourism resources.
Economy factor (). It mainly includes economic benefits and economic structure, focussing on (1) domestic and international tourism revenue, the number of tourists attracted; (2) total tourism revenue as a proportion of GDP, tourism transportation and communication revenue as a proportion of tourism revenue, accommodation revenue as a proportion of tourism revenue, food and beverage revenue as a proportion of tourism revenue, entertainment revenue as a proportion of tourism revenue, and tourism merchandise sales revenue as a proportion of tourism revenue.
Society factor (). It includes three main aspects: social participation, human resources and tourism experience, focussing on (1) the proportion of community residents in the workforce, resident satisfaction; (2) the number of tourism employees, the proportion of tour guides with certificates, the revisit rate; and (3) tourist satisfaction, length of stay per capita and abundance of participation projects.
Management factor (). It mainly consists of integrated management and government support, focussing on (1) institutions and systems, the scientific aspects of strategic planning, tourism service centres; and (2) planning, policy, and regulation development.
In order to scientifically and rationally select the most potential rural settlement, the experts separately evaluate the above three settlements with respect to four attributes based on the linguistic evaluation scale with seven granularities where ‘
very low’, ‘
low’, ‘
slightly low’, ‘
medium’, ‘
slightly high’, ‘
high’, and ‘
very high’. The original information given five experts is shown in . Note that the symbol ‘-‘ in means that the DMs cannot give complete evaluation information due to limited personal knowledge. The administration has recorded the linguistic evaluation information of all experts, for each alternative with respect to each attribute, into a group decision matrix (as shown in ) by the PLTSs. The data unit can also imply the partial information missing, that is, the sum of probabilities of linguistic terms in the PLTS can be less than 1.
Table 1. The LDM provided by the first expert.
Table 2. The LDM provided by the second expert.
Table 3. The LDM provided by the third expert.
Table 4. The LDM provided by the fourth expert.
Table 5. The LDM provided by the fifth expert.
Table 6. The group PLDM.
5.2. The decision steps
Step 1. We can get the group PLDM (as shown in ) by aggregating these five tables directly. For example, the probabilistic linguistic evaluation value of with respect to
is
which indicates one of five DMs selects
two of five DMs selects
and one of five DMs insists
Consider that all the attributes are benefit attribute, we can determine the equivalent PLDM
Step 2. The evaluation information can also imply the partial information missing, that is, the sum of probabilities of linguistic terms in the PLTS can be less than 1. Consequently, by using EquationEquation (3)(3)
(3) in Definition 4, the normalised PLDM is shown in , and obtain the following normalised decision matrix
Table 7. The normalised group PLDM.
Table 8. The PIS and NIS of each attribute and the distance between them.
Table 9. The relative weight
Table 10. Effect of the parameter on the ranking results.
Table 11. Comparison results of the existence distances and the PL-Wasserstein distance.
Table 12. Ranking results of different methods.
Step 3. Using EquationEquations (4)(4)
(4) and Equation(5)
(5)
(5) , obtain the expected values and the variance values of all attributes of PLTEs, which are shown in form of the following two matrices
and
Based on these, using EquationEquations (9)
(9)
(9) and (10), calculate the PIS
and NIS
of attribute
which are shown in .
Based on the EquationEquations (11)(11)
(11) and Equation(12)
(12)
(12) , obtain the values of
and
as follows:
Step 4. Using EquationEquations (13)–(16), we can get attribute weight vector
Step 5. Obtain the relative weight of the criterion
to reference point
by EquationEquation (19)
(19)
(19) shown in .where
Step 6. Calculate the dominance degree of each alternative over each alternative
with respect to attribute
according to EquationEquation (20)
(20)
(20) . For each attribute
the dominance degree matrices
of pairwise alternatives can be obtained by EquationEquation (20)
(20)
(20) as follows (
).
Step 7. The overall dominance degree is calculated according to EquationEquation (21)(21)
(21) , and get the overall dominance degree matrix of pairwise alternatives as follows:
Step 8. Using EquationEquation (22)(22)
(22) , get the global prospect value
of alternative
Step 9. Rank the three alternatives in accordance with the value of and get
Therefore, we select the optimal alternative (Chaji Village, Taohuatan Township, Jing County), which shows that the village is the area of the sustainable rural tourism with highest potential for Xuancheng District.
5.3. Management implications
In view of the above results of the case study, we try to explore the management implications of the evaluation of sustainable rural tourism potential to help managers or decision-makers at a higher level to provide a reference for decision-making. The management implications are summarised as follows. On the one hand, considering Chaji Village has been selected as the best alternative by the comprehensive evaluation of the group, the local government can set the Chaji Village as a model for the sustainable rural tourism development. Other rural settlements are encouraged to learn from its successful experience in sustainable tourism development such as economy and society factors. On the other hand, reasonable and benign evaluation of sustainable rural tourism development potential is conducive to improving the investment environment of rural tourism. To this end, a lot of advance publicity for the rural tourism settlements with higher sustainable potential is needed to attract investors to increase their investment and accelerate the construction of new rural areas.
5.4. Sensitivity analysis
In the proposed Wasserstein-based PL-TODIM method, the attention factor of the losses plays an important role when calculating the dominance degree of each alternative. The value of the parameter reflects the decision-making attitude towards risk. According to experiments, Tversky and Kahneman (Citation1992) point out that the attenuation factor of losses is about 2.25, ranging from 1 to 2.5. Especially when
= 2.25, it is most consistent with the decision-maker’s psychological attitude towards risk. To enhance the universal applicability of the proposed method, we change the values of
from 1 to 2.5 to obtain the prospect values and ranking results of three alternatives. The corresponding results are shown in .
From , we can easily find that the prospect values of alternative and alternative
remain unchanged, with values of 1 and 0, respectively. However, the range of the prospect value of alternative
is very small. Besides, it can be seen from the results that the change of
from 1 to 2.5 has no effect on the ranking results. In other words, in this case, the parameter value of risk preference of decision makers does not affect the optimal selection of alternatives.
5.5. Comparative analysis
5.5.1. Comparisons for distance measures of PLTSs
In view of the shortcomings of the above distance measures between two PLTSs, a novel distance between two PLTSs is defined by employing the idea of classical Wasserstein distance. Compared with different existing distances, the proposed PL-Wasserstein distance has the following evidences summarised in . From the , it is not difficult to find that the proposed distance measure overcome the defects of the existing distance measure in the Refs. Pang et al. (Citation2016); Mao et al. (Citation2019); Wang, Wang, et al. (Citation2019); Zhang, Xu, et al. (Citation2016); Zhang, Xu, et al. (Citation2017); Lin et al. (Citation2019); Zhang et al. (Citation2019).
Remark 3.
Unlike the existing distance measures, Wu et al. (Citation2018) proposed an adjustment rule of PLTSs, aiming at obtaining the adjusted PLTSs with the same probability set, before calculating the distance between two PLTS. The advantage of Wu et al.’s distance measure is no need to add linguistic terms to the smaller one, and it is very effective in dealing with small and medium scale decision-making problems with PLTSs. However, this adjustment of probability information indirectly increases the complexity of information processing for large-scale decision-making problems with PLTSs. Besides, after verification, we found that the results calculated by Wu et al.’s distance measure are consistent with those calculated by the proposed PL-Wasserstein distance when
while the proposed distance measure can be applied to a wider range of situations such as when
Remark 4.
Compared with the distance measure proposed by Wu et al. (Citation2018), the proposed distance measure has the following advantages. Firstly, it neither needs to unify PLTS to the same length nor needs to rearrange PLTS in some order. Secondly, it can fully capture the infimum of difference degree between two PLTSs from an optimisation perspective. In summary, one can find that the difference reflects the profound physical and economic significance due to the involvement of the optimal transport theory.
5.5.2. Comparisons for decision-making approaches
In this section, we further demonstrate the rationality and effectiveness of the developed method by comparing it with four other MAGDM methods including the PL-TODIM method (Liu & You, Citation2017), the extended PL-TOPSIS method (Pang et al., Citation2016), the PL-GLDS method (Wu & Liao, Citation2019), and the traditional HFLTS-based TOPSIS method (Pang et al., Citation2016). The related decision results are shown in .
Obviously, the ranking results obtained by the proposed method in this paper is the same as that obtained by the PL-TODIM method (Liu & You, Citation2017), the extended PL-TOPSIS method (Pang et al., Citation2016) and the PL-GLDS method (Wu & Liao, 2019), which also demonstrates the effectiveness of the proposed method. Even so, there is reason to believe that the proposed method has some desirable advantages over the three other methods as below:
Compared with the PL-TODIM method, the characterises of the proposed method is mainly reflected by the following two aspects. (a) In terms of evaluation information processing, the proposed method avoids loss of information and fully capture the infimum of difference degree between two PLTSs from an optimisation perspective, namely, neither needs to unify PLTS to the same length nor needs to rearrange PLTS in some order. Therefore, the result obtained by the proposed method is more actual and credible. (b) In terms of determining the objective weight of attributes, the attribute weight in Liu and You (Citation2017) is determined by the information entropy method. It does not consider the influence of the correlation between attributes on the decision results. However, correlation of attributes often exists objectively in a real decision environment. Therefore, the attribute weight obtained by the PL-CRITIC method, which considers the contrast intensity and conflict of attributes, is more reasonable.
Compared with the extended PL-TOPSIS method, the proposed method has the following advantages. (a) The proposed method does not need to add artificial linguistic terms or rearrange PLTS in the normalising process, thus simplifying the calculation process of complex decision information. (b) The maximum deviation method is utilised to determine attribute weights in Pang et al. (Citation2016), which cannot reflect the correlation between attributes. Conversely, the attribute weight vector in this paper is obtained by the PL-CRITIC method considering the contrast intensity and conflict of attributes. (c) The proposed method considers the psychological behaviour of DMs. Thus, in terms of simulating a real decision situation, our proposed method is much closer to reality than Pang et al.’s method.
The PL-GLDS method is proposed by Wu and Liao (Citation2019). The main idea of PL-GLDS method is to calculate the dominance flow between two alternatives over each criterion. Under each criterion, the selected alternative has the high gained dominance score as well as the low lost dominance score. For ease of comparison, we assume that the attribute weights are consistent with those of the proposed approach. Thus, the proposed method has the following advantages over the PL-GLDS method proposed by Wu and Liao (Citation2019). (a) The efficiency of information processing and the breadth of problem-solving are both improved (see Remark 3). (b) The Wasserstein-based distance measure with PLTSs imply profound physical and economic significance (see Remark 4). (c) The behaviour characteristics of ‘limited rationality’ of DMs are taken into consideration in the proposed method.
However, the ranking result of the method proposed in this paper is not the same as that of the traditional HFLTSs-based TOPSIS method in Pang et al. (Citation2016). The possible reasons are the following three points: (a) Based on the HFLTSs, the proportions of different assessed values are not reflected, leading to different decision outcomes. (b) The expanded method that makes all HFLTSs have the same number of linguistic terms changes the actual information to some extent. (c) The TOPSIS approach using the traditional HFLTSs does not fully utilise the original linguistic information provided by DMs, and thus may lead to distorted decision results.
6. Conclusions
Evaluation of the potential for sustainable rural tourism is a very important challenging issue including both improving uncertain information processing and simulating DMs’ complex behaviours. PLTSs can be used to represent the highly uncertain preferences of the invited experts when evaluating the potential for sustainable rural tourism. Besides, experts usually have the behaviour characteristics of ‘limited rationality’ in the actual assessment process. Therefore, research on behavioural MAGDM methods based on PLTSs for the evaluation of sustainable rural tourism potential is very meaningful. In this paper, to improve information processing of PLTS (such as measuring differences among PLTSs), a new Wasserstein-based distance measure is firstly defined. After that, we propose the PL-CRITIC method to determine the attribute weight. Subsequently, we develop a new behaviour decision method by integrating Wasserstein distance measure into the PL-TODIM method. At length, a case study is illustrated to verify the practicability and validity of the proposed method. The proposed Wasserstein-based PL-TODIM method has the following characteristics.
Do not need to unify PLTS to the same length and to rearrange PLTS in some order. Thus, not only does it greatly improve the efficiency of processing complex decision information, but it also avoids erroneous decision results that are inconsistent with intuition.
The Wasserstein-based distance measure between PLTSs satisfies all the axioms of the distance especially including the property of the triangle inequality, which is difficult to be involved in the existing distance measures.
The interaction between attributes and the psychological behaviours of DMs is comprehensively considered in the proposed MAGDM method, which ensures that the obtained decision results are more reasonable and reliable.
In the future, there exist good prospects for the further study of the Wasserstein-based behaviour MAGDM method with PLTSs, which can be extended to other decision environments such as dynamic, risky, and consensus evaluation of sustainable rural tourism potential.
Disclosure statement
No potential conflict of interest was reported by the authors.
Additional information
Funding
References
- Alemi-Ardakani, M., Milani, A. S., Yannacopoulos, S., & Shokouhi, G. (2016). On the effect of subjective, objective and combinative weighting in multiple criteria decision making: A case study on impact optimisation of composites. Expert Systems with Applications, 46, 426–438. https://doi.org/10.1016/j.eswa.2015.11.003
- Alinezhad, A., & Khalili, J. (2019). New methods and applications in multiple attribute decision making (MADM). Springer.
- Bai, C., Zhang, R., Qian, L., & Wu, Y. (2017). Comparisons of probabilistic linguistic term sets for multi-criteria decision making. Knowledge-Based Systems, 119, 284–291. https://doi.org/10.1016/j.knosys.2016.12.020
- Diakoulaki, D., Mavrotas, G., & Papayannakis, L. (1995). Determining objective weights in multiple criteria problems: The critic method. Computers & Operations Research, 22(7), 763–770. https://doi.org/10.1016/0305-0548(94)00059-H
- Feng, X., Liu, Q., & Wei, C. (2019). Probabilistic linguistic QUALIFLEX approach with possibility degree comparison. Journal of Intelligent & Fuzzy Systems, 36(1), 719–730. https://doi.org/10.3233/JIFS-172112
- Fu, C., Xu, D. L., & Xue, M. (2018). Determining attribute weights for multiple attribute decision analysis with discriminating power in belief distributions. Knowledge-Based Systems, 143, 127–141. https://doi.org/10.1016/j.knosys.2017.12.009
- Gao, J., & Wu, B. (2017). Revitalising traditional villages through rural tourism: A case study of Yuanjia Village, Shaanxi Province, China. Tourism Management, 63, 223–233. https://doi.org/10.1016/j.tourman.2017.04.003
- Gomes, L. F. A. M., & Lima, M. M. P. P. (1992). TODIM: Basics and application to multicriteria ranking of projects with environmental impacts. Foundations of Computing and Decision Sciences, 16(4), 113–127.
- Gou, X., & Xu, Z. (2016). Novel basic operational laws for linguistic terms, hesitant fuzzy linguistic term sets and probabilistic linguistic term sets. Information Sciences, 372, 407–427. https://doi.org/10.1016/j.ins.2016.08.034
- Gou, X., Xu, Z., & Liao, H. (2017). Multiple criteria decision making based on Bonferroni means with hesitant fuzzy linguistic information. Soft Computing, 21(21), 6515–6529. https://doi.org/10.1007/s00500-016-2211-1
- Griesiene, I., & Georgeta, C. P. (2017). Sustainable tourism development in the rural areas. Transformations in Business & Economics, 16(3), 42.
- He, Y., Lei, F., Wei, G., Wang, R., & Wei, C. (2019). EDAS method for multiple attribute group decision making with probabilistic uncertain linguistic information and its application to green supplier selection. International Journal of Computational Intelligence Systems, 12(2), 1361–1370.
- Herrera, F., & Herrera-Viedma, E. (2000). Linguistic decision analysis: Steps for solving decision problems under linguistic information. Fuzzy Sets and Systems, 115(1), 67–82. https://doi.org/10.1016/S0165-0114(99)00024-X
- Lane, B. (1994). What is rural tourism? Journal of Sustainable Tourism, 2(1–2), 7–21. https://doi.org/10.1080/09669589409510680
- Lei, F., Wei, G., Gao, H., Wu, J., & Wei, C. (2020). TOPSIS method for developing supplier selection with probabilistic linguistic information. International Journal of Fuzzy Systems, 22(3), 749–759. https://doi.org/10.1007/s40815-019-00797-6
- Lei, F., Wei, G., Lu, J., Wu, J., & Wei, C. (2019). GRA method for probabilistic linguistic multiple attribute group decision making with incomplete weight information and its application to waste incineration plants location problem. International Journal of Computational Intelligence Systems, 12(2), 1547–1556.
- Li, P., Liu, J., & Wei, C. (2020). Factor relation analysis for sustainable recycling partner evaluation using probabilistic linguistic DEMATEL. Fuzzy Optimisation and Decision Making, 19(4), 471–497. https://doi.org/10.1007/s10700-020-09326-9
- Li, P., Liu, J., Yang, Y., & Wei, C. (2020). Evaluation of poverty-stricken families in rural areas using a novel case-based reasoning method for probabilistic linguistic term sets. Computers & Industrial Engineering, 147, 106658. https://doi.org/10.1016/j.cie.2020.106658
- Liang, W., Luo, S., & Zhao, G. (2019). Evaluation of cleaner production for gold mines employing a hybrid multi-criteria decision making approach. Sustainability, 11(1), 146. https://doi.org/10.3390/su11010146
- Liao, H., Mi, X., & Xu, Z. (2020). A survey of decision-making methods with probabilistic linguistic information: bibliometrics, preliminaries, methodologies, applications and future directions. Fuzzy Optimisation and Decision Making, 19(1), 81–134. https://doi.org/10.1007/s10700-019-09309-5
- Lin, M., Chen, Z., Liao, H., & Xu, Z. (2019). ELECTRE II method to deal with probabilistic linguistic term sets and its application to edge computing. Nonlinear Dynamics, 96(3), 2125–2143. https://doi.org/10.1007/s11071-019-04910-0
- Lipman, Y., Puente, J., & Daubechies, I. (2013). Conformal Wasserstein distance: II. Computational Aspects and Extensions. Mathematics of Computation, 82(281), 331–381.
- Liu, P., & You, X. (2017). Probabilistic linguistic TODIM approach for multiple attribute decision-making. Granular Computing, 2(4), 333–342. https://doi.org/10.1007/s41066-017-0047-4
- Liu, X., Wang, Z., Zhang, S., & Liu, J. (2020). Probabilistic hesitant fuzzy multiple attribute decision-making based on regret theory for the evaluation of venture capital projects. Economic Research-Ekonomska Istraživanja, 33(1), 672–697. https://doi.org/10.1080/1331677X.2019.1697327
- Llamazares, B. (2018). An analysis of the generalised TODIM method. European Journal of Operational Research, 269(3), 1041–1049. https://doi.org/10.1016/j.ejor.2018.02.054
- Lu, J., Wei, C., Wu, J., & Wei, G. (2019). TOPSIS method for probabilistic linguistic MAGDM with entropy weight and its application to supplier selection of new agricultural machinery products. Entropy, 21(10), 953. https://doi.org/10.3390/e21100953
- Ma, Z., Zhu, J., & Zhang, S. (2021). Probabilistic-based expressions in behavioural multi-attribute decision making considering pre-evaluation. Fuzzy Optimisation and Decision Making, 20(1), 145–173. https://doi.org/10.1007/s10700-020-09335-8
- Mao, X. B., Wu, M., Dong, J. Y., Wan, S. P., & Jin, Z. (2019). A new method for probabilistic linguistic multi-attribute group decision making: Application to the selection of financial technologies. Applied Soft Computing, 77, 155–175. https://doi.org/10.1016/j.asoc.2019.01.009
- McAreavey, R., & McDonagh, J. (2011). Sustainable rural tourism: Lessons for rural development. Sociologia Ruralis, 51(2), 175–194. https://doi.org/10.1111/j.1467-9523.2010.00529.x
- Oh, J. H., Pouryahya, M., Iyer, A., Apte, A. P., Deasy, J. O., & Tannenbaum, A. (2020). A novel kernel Wasserstein distance on Gaussian measures: An application of identifying dental artefacts in head and neck computed tomography. Computers in Biology and Medicine, 120, 103731. https://doi.org/10.1016/j.compbiomed.2020.103731
- Pang, Q., Wang, H., & Xu, Z. (2016). Probabilistic linguistic term sets in multi-attribute group decision making. Information Sciences, 369, 128–143. https://doi.org/10.1016/j.ins.2016.06.021
- Peng, X., Zhang, X., & Luo, Z. (2019). Pythagorean fuzzy MCDM method based on CoCoSo and CRITIC with score function for 5G industry evaluation. Artificial Intelligence Review, 53, 3813–3847. https://doi.org/10.1007/s10462-019-09780-x
- Puška, A., Stojanović, I., & Maksimović, A. (2019). Evaluation of sustainable rural tourism potential in Brcko district of Bosnia and Herzegovina using multi-criteria analysis. Operational Research in Engineering Sciences: Theory and Applications, 2(2), 40–54.
- Qin, J., Liu, X., & Pedrycz, W. (2017). An extended TODIM multi-criteria group decision making method for green supplier selection in interval type-2 fuzzy environment. European Journal of Operational Research, 258(2), 626–638. https://doi.org/10.1016/j.ejor.2016.09.059
- Ran, M., Hu, J., Chen, Y., Chen, H., Sun, H., Zhou, J., & Zhang, Y. (2019). Denoising of 3D magnetic resonance images using a residual encoder-decoder Wasserstein generative adversarial network. Medical Image Analysis, 55, 165–180. https://doi.org/10.1016/j.media.2019.05.001
- Rodriguez, R. M., Martinez, L., & Herrera, F. (2012). Hesitant fuzzy linguistic term sets for decision making. IEEE Transactions on Fuzzy Systems, 20(1), 109–119. https://doi.org/10.1109/TFUZZ.2011.2170076
- Saaty, T. L., & Peniwati, K. (2013). Group decision making: Drawing out and reconciling differences. RWS Publications.
- Solomon, J. (2020). Optimal transport on discrete domains. Proceedings of Symposia in Applied Mathematics, 76, 103–142. https://doi.org/10.1090/psapm/076/00673
- Soni, N., Christian, R. A., & Jariwala, N. (2016). Pollution potential ranking of industries using classical TODIM method. Journal of Environmental Protection, 07(11), 1645–1656. https://doi.org/10.4236/jep.2016.711134
- Su, B. (2011). Rural tourism in China. Tourism Management, 32(6), 1438–1441. https://doi.org/10.1016/j.tourman.2010.12.005
- Trukhachev, A. (2015). Methodology for evaluating the rural tourism potentials: A tool to ensure sustainable development of rural settlements. Sustainability, 7(3), 3052–3070. https://doi.org/10.3390/su7033052
- Tversky, A., & Kahneman, D. (1992). Advances in prospect theory: Cumulative representation of uncertainty. Journal of Risk and Uncertainty, 5(4), 297–323. https://doi.org/10.1007/BF00122574
- Villani, C. (2008). Optimal transport: Old and new. Springer Science & Business Media, 338, 105–108.
- Wang, H., Xu, Z., & Zeng, X. J. (2018). Modelling complex linguistic expressions in qualitative decision making: An overview. Knowledge-Based Systems, 144, 174–187. https://doi.org/10.1016/j.knosys.2017.12.030
- Wang, W., Liu, X., Qin, J., & Liu, S. (2019). An extended generalised TODIM for risk evaluation and prioritisation of failure modes considering risk indicators interaction. IISE Transactions, 51(11), 1236–1250. https://doi.org/10.1080/24725854.2018.1539889
- Wang, X., Wang, J., & Zhang, H. (2019). Distance-based multicriteria group decision-making approach with probabilistic linguistic term sets. Expert Systems, 36(2), e12352. https://doi.org/10.1111/exsy.12352
- Wei, C., Ren, Z., & Rodríguez, R. M. (2015). A hesitant fuzzy linguistic TODIM method based on a score function. International Journal of Computational Intelligence Systems, 8(4), 701–712. https://doi.org/10.1080/18756891.2015.1046329
- Wei, G., Lei, F., Lin, R., Wang, R., Wei, Y., Wu, J., & Wei, C. (2020). Algorithms for probabilistic uncertain linguistic multiple attribute group decision making based on the GRA and CRITIC method: Application to location planning of electric vehicle charging stations. Economic Research-Ekonomska Istraživanja, 33(1), 828–846. https://doi.org/10.1080/1331677X.2020.1734851
- Wei, G., Lu, J., Wei, C., & Wu, J. (2020). Probabilistic linguistic GRA method for multiple attribute group decision making. Journal of Intelligent & Fuzzy Systems, 38(4), 4721–4732. https://doi.org/10.3233/JIFS-191416
- Wei, G., Wei, C., Wu, J., & Wang, H. (2019). Supplier selection of medical consumption products with a probabilistic linguistic MABAC method. International Journal of Environmental Research and Public Health, 16(24), 5082. https://doi.org/10.3390/ijerph16245082
- Winston, W. L., & Goldberg, J. B. (2004). Operations research: applications and algorithms (Vol. 3). Thomson/Brooks/Cole.
- Wu, X., & Liao, H. (2019). A consensus-based probabilistic linguistic gained and lost dominance score method. European Journal of Operational Research, 272(3), 1017–1027. https://doi.org/10.1016/j.ejor.2018.07.044
- Wu, X., Liao, H., Xu, Z., Hafezalkotob, A., & Herrera, F. (2018). Probabilistic linguistic MULTIMOORA: A multicriteria decision making method based on the probabilistic linguistic expectation function and the improved Borda rule. IEEE Transactions on Fuzzy Systems, 26(6), 3688–3702. https://doi.org/10.1109/TFUZZ.2018.2843330
- Wu, Z., & Xu, J. (2016). Possibility distribution-based approach for MAGDM with hesitant fuzzy linguistic information. IEEE Transactions on Cybernetics, 46(3), 694–705. https://doi.org/10.1109/TCYB.2015.2413894
- Xu, Z., He, Y., & Wang, X. (2019). An overview of probabilistic-based expressions for qualitative decision-making: Techniques, comparisons and developments. International Journal of Machine Learning and Cybernetics, 10(6), 1513–1528. https://doi.org/10.1007/s13042-018-0830-9
- Zadeh, L. A. (1975). The concept of a linguistic variable and its application to approximate reasoning—I. Information Sciences, 8(3), 199–249. https://doi.org/10.1016/0020-0255(75)90036-5
- Zhang, D., Li, Y., & Wu, C. (2020). An extended TODIM method to rank products with online reviews under intuitionistic fuzzy environment. Journal of the Operational Research Society, 71(2), 322–334. https://doi.org/10.1080/01605682.2018.1545519
- Zhang, S., Zhu, J., Liu, X., & Chen, Y. (2016). Regret theory-based group decision-making with multidimensional preference and incomplete weight information. Information Fusion, 31, 1–13. https://doi.org/10.1016/j.inffus.2015.12.001
- Zhang, S., Zhu, J., Liu, X., Chen, Y., & Ma, Z. (2017). Adaptive consensus model with multiplicative linguistic preferences based on fuzzy information granulation. Applied Soft Computing, 60, 30–47. https://doi.org/10.1016/j.asoc.2017.06.028
- Zhang, Y., Xu, Z., & Liao, H. (2017). A consensus process for group decision making with probabilistic linguistic preference relations. Information Sciences, 414, 260–275. https://doi.org/10.1016/j.ins.2017.06.006
- Zhang, Y., Xu, Z., & Liao, H. (2019). Water security evaluation based on the TODIM method with probabilistic linguistic term sets. Soft Computing, 23(15), 6215–6230. https://doi.org/10.1007/s00500-018-3276-9
- Zhang, Y., Xu, Z., Wang, H., & Liao, H. (2016). Consistency-based risk assessment with probabilistic linguistic preference relation. Applied Soft Computing, 49, 817–833.
Appendix
A.1. The proof of Property 1
Proof.
3) When if and only if
then
and
that is
which completes the proof.