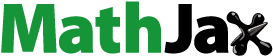
Abstract
In recent years, emergent public health events happen from time to time, which puts forward new requirements for the establishment of a perfect medical emergency system. It is a new direction to evaluate the effectiveness of medical emergency systems from the perspective of multi-attribute group decision making (MAGDM) issues. In such article, we tend to resolve the MAGDM issues under single-valued neutrosophic sets (SVNSs) with TODIM method based on cumulative prospect theory (CPT). And the single-valued neutrosophic TODIM method based on CPT (CPT-SVN-TODIM) for MAGDM issues are developed. This new method not only inherits advantages of classical TODIM method, but also has further improvement in some aspects. For example, we set up the entropy to calculate attribute weights for ensuring the more objective decision-making process. Furthermore, it is also an extension of MAGDM method to utilize single-valued neutrosophic numbers (SVNNs) to depict decision makers’ ideas. In addition, we introduce the application of CPT-SVN-TODIM method in the assessment of medical emergency management. And finally, the reliability of CPT-SVN-TODIM method is confirmed by comparing with some other methods.
1. Introduction
The indistinct or indeterminate thing pervades the real world. In 1965, Zadeh (Citation1965) created fuzzy sets which takes advantage of membership functions to present imprecise phenomena. After that, a variety of fuzzy sets become progressively more, such as intuitionistic fuzzy sets (Atanassov, Citation1986; Zhang, Gao, et al., Citation2021; Zhao, Wei, Chen, et al., Citation2021), bipolar fuzzy sets (Wen-Ran, Citation1994; Zhao, Wei, Guo, et al., Citation2021), neutrosophic sets (Wang et al., Citation2010), Pythagorean fuzzy sets (He et al., Citation2021; Yager, Citation2014; Zhao, Wei, Wei, et al., Citation2021), and picture fuzzy sets (Cuong, Citation2014). The MADM or MAGDM refers to the decision-making issues of choosing the best alternative or alternative-ranking when considering multiple attributes (Wei, Wei, et al., Citation2021; Zanon et al., Citation2021; Zhao, Li, et al., Citation2021). In traditional MADM or MAGDM, the attribute value is expressed with crisp number (Agrebi & Abed, Citation2021; Guo et al., Citation2021; Huang et al., Citation2021). At present using fuzzy numbers to study MADM or MAGDM problems has been extended to many fields (Lei et al., Citation2021; Tehreem et al., Citation2021; Verma, Citation2021).
The basic concept of neutrosophic set (NS) was built by Smarandache (Citation2002) in 2002. Wang et al. (Citation2010) built the single-valued neutrosophic set (SVNS) for dealing with the difficulty of NS in practical application. Huang (Citation2016) proposed distance formula & similarity formula of SVNSs. Ji et al. (Citation2018) defined frank operations of SVNSs and presented Frank BM (SVNFBM) operator under SVNSs. Wu et al. (Citation2018) investigated the entropy & similarity under SVNSs. Peng et al. (Citation2019) also put forward some power Shapley Choquet average under SVNSs.
In order to study the issue of MADM/MAGDM in-depth, lots of methods were created, such as TODIM method (Gomes et al., Citation2009; Long et al., Citation2020), WASPAS method (Davoudabadi et al., Citation2020; Dorfeshan & Mousavi, Citation2020), MABAC method, Taxonomy method (Jurkowska, Citation2014), TOPSIS method (Xu, Ke, et al., Citation2020) and so on. The design idea of TODIM method is derived from the different attitudes of decision makers towards profit and loss, which makes the method have good applications. Moreover, many scholars combined TODIM method with different fuzzy sets. For example, Xu et al. (Citation2017) developed TODIM method with SVNS information. Liang et al. (Citation2019) built TODIM method under proportional hesitant fuzzy linguistic setting. Liu et al. (Citation2019) focused on fermatean fuzzy linguistic information. Lin et al. (Citation2020) established new TODIM method under hesitant fuzzy linguistic setting. Ji et al. (Citation2020) selected the dual hesitant Pythagorean fuzzy setting to investigate TODIM method. Lu et al. (Citation2020) utilized triangular fuzzy number to express the uncertain information. Sun et al. (Citation2019) proposed a new SVNS distance and utilized it in establishing extended TODIM model and ELECTRE III model. Xu et al. (Citation2019) constructed SVNS TODIM method as the tool for dealing with the decision making in venture capital. Long et al. (Citation2020) also created new TODIM with SVNS and the determination method of weights. Xu, Wei, et al. (Citation2020) chose to improve TODIM method and PROMETHEE method under single-valued neutrosophic environment.
Tian et al. (Citation2019) built TODIM method based on CPT (CPT-TODIM). This method uses the concept of weight function to improve the traditional TODIM. In the traditional TODIM method, relative weights are used to deal with attribute weights, while CPT-TODIM takes advantage of weight functions to express the influence of decision-makers’ different attitudes towards gains and losses on attribute weights. To a certain extent, increasing the risk weighting moderately is conducive to the enterprise’s risk avoidance and conforms to the enterprise’s decision-making requirements. Therefore, in my opinion, CPT-TODIM method has obvious advantages in dealing with multi-attribute decision problems. However, there are few studies using this method and few studies evaluating medical emergency response systems based on this method. Therefore, this paper aims to build the single-valued neutrosophic TODIM based on CPT (CPT-SVN-TODIM) method and discuss its application to evaluation of medical emergency system.
The structure of such paper is given as follows. In the section 2, we introduce the definition of NSs and SVNSs. In addition, we also introduce the CPT-TODIM method. In the section 3, we establish CPT-SVN-TODIM method and demonstrate its calculative procedure including determining attribute weights. In the section 4, we apply this CPT-SVN-TODIM method to evaluation of the medical emergency system. And through the fifth part of the comparative analysis concludes that the CPT-SVN-TODIM method proposed in such paper is with effectiveness.
2. Preliminary knowledge
In this section, we introduce the basic knowledge about SVNSs and the CPT-TODIM method.
2.1. NSS and SVNSs
Definition 1
(Smarandache, Citation2002). A NS which consists of truth-membership
indeterminacy-membership
and falsity-membership
can be expressed as follows in a fix set
(1)
(1)
where
are lying in
and
Neutrosophic set brings in a new function named as indeterminacy-membership function, but it is hard to apply in practice. Hence, the SVNS is exploited.
Definition 2
(Wang et al., Citation2010). A SVNS in a fix set
can be expressed as the following form
(2)
(2)
where truth-membership
indeterminacy-membership
and falsity-membership
all belong to
and satisfy
For convenience, we usually use single-valued neutrosophic number (SVNN) in calculating. Moreover, the score and the accuracy function are created to describe relative precision.
Definition 3
(Zhang et al, Citation2014). The score function of SVNN is
(3)
(3)
Definition 4
(Zhang et al., Citation2014). The accuracy function of SVNN is
(4)
(4)
Definition 5
(Zhang et al., Citation2014). Suppose two SVNNs and
means
if
when
then
and when
then
Definition 6
(Wang et al., Citation2010). Suppose two SVNNs and
then the basic operations are given:
Definition 7
(Sahin & Kucuk, Citation2014). Let and
be two SVNNs respectively, the Hamming distance between two given SVNNs is defined by EquationEq. (5)
(5)
(5) .
(5)
(5)
Definition 8
(Zhang et al., Citation2014). If there is a collection of SVNNs (
) and the weighting vector of
(
) is
where
and
then the single-valued neutrosophic weighted averaging (SVNWA) operator is:
(6)
(6)
Definition 9
(Zhang et al., Citation2014). If there is a group of SVNNs (
) and the weighting of
(
) is
where
and
then the single-valued neutrosophic weighted geometric (SVNWG) operator is:
(7)
(7)
2.2. CPT-TODIM method
In this topic, we introduce the CPT-TODIM method (Tian et al., Citation2019). There are two collections including the set of alternatives and the set of attributes
The vector of attribute weights is
(
and
). At the same time, establish a decision matrix
in which represents the value of alternative
(
) under attribute
(
).
Step 1. Compute the modified weights
(
) based on EquationEq. (8)
(8)
(8) and EquationEq. (9)
(9)
(9) , where
and
as parameters are used to express the curvature of weighting function.
Step 2. Acquire the comprehensive predominance
(
) by taking advantage of EquationEq. (10)
(10)
(10) .
where
(11)
(11)
and
and
are the parameters.
Step 3. Calculate the overall predominance
(
) by applying EquationEq. (12)
(12)
(12) .
Step 4. According to the overall predominance
(
), rank all alternatives and the most optimal alternative with the biggest value of overall predominance.
3. Single-valued neutrosophic TODIM method for MAGDM based on CPT
Based on the above TODIM method and SVNSs, we create the CPT-SVN-TODIM method which is expounded in this section for resolving the issue of MAGDM. There are three sets of information: the set of alternatives the set of attributes
and the set of decision makers
About the decision maker
expresses the evaluation of the alternative
about the attribute
Gathering the assessment of decision maker
for every alternative in every attribute, we can get single-valued neutrosophic decision matrix
where
as well as
respectively indicate truth-membership, indeterminacy-membership and falsity-membership and satisfy
and
(
). Furthermore, the weighting values of DMs is
(
and
).
First of all, keep unification of attributes with different characters by using the EquationEq. (13)(13)
(13) and make up the standardized single-valued neutrosophic decision matrix
(
).
(13)
(13)
The foundation of the follow-up work is to integrate all decision matrices from different decision makers into one group decision matrix (
). The EquationEq. (14)
(14)
(14) can help us to finish it.
(14)
(14)
Attribute weights is a prerequisite for guaranteeing more impersonal consequence. Therefore, we select the single-valued neutrosophic entropy (Wu et al., Citation2018) to analyze the information of group decision matrix (
) and achieve the initial weighting vector of attributes
(
and
) which is figured out by EquationEqs. (15)–(17).
(15)
(15)
(16)
(16)
(17)
(17)
The weighting function (18) and EquationEq. (19)(19)
(19) are taking advantage of disposing the initial weighting vector of attributes
to obtain the modified weights
(
).
(18)
(18)
(19)
(19)
Then, based on the modified weights and the distance equation (EquationEq. (20)(20)
(20) ), we have ability to calculate the relative predominance
of alternative
compared with
underneath the attribute
(20)
(20)
(21)
(21)
where
and
are the parameters. And the relative predominance
can be gathered in the relative predominance matrix
just as:
(22)
(22)
The overall predominance matrix (
) is adding all relative predominance matrices together.
(23)
(23)
Except for the diagonal elements, each element of overall predominance matrix is computing by EquationEq. (24)
(24)
(24) .
(24)
(24)
Finally, the standard overall predominance (
) of the alternative
over all others is determined according to EquationEq. (25)
(25)
(25) .
(25)
(25)
The standard overall predominance value of the optimal alternative is equivalent to 1.
To sum up, the CPT-SVN-TODIM method includes the following steps:
Step 1. Build the single-valued neutrosophic decision matrix
Step 2. Take advantage of the EquationEq. (13)
(13)
(13) to ensure the unification of all of attributes.
Step 3. Integrate all single-valued neutrosophic decision matrices into group decision matrix
with respect to EquationEq. (14)
(14)
(14) .
Step 4. Acquire the modified weights
on the basis of EquationEqs. (15)–(19).
Step 5. Figure out the relative predominance
according to EquationEqs. (20)
(20)
(20) and Equation(21)
(21)
(21) .
Step 6. Determine the overall predominance
in line with EquationEq. (24)
(24)
(24) .
Step 7. Calculate the standard overall predominance
by using EquationEq. (25)
(25)
(25) .
Step 8. Obtain the order of alternatives by means of sorting the standard overall predominance
in descending order.
4. Numerical instance
Earthquake, in view of its great destructive power, huge difficulty in forecasting and the consequent influence upon social order, needs us to be fully aware of the importance of medical aid in disaster rescue. With the development of society, human beings have put forward higher and higher demands on the need and ability to provide health security. Especially when life is threatened, they are eager to receive timely and efficient emergency assistance. Therefore, it is of great practical significance to reduce disasters and improve the efficiency of medical rescue at the beginning of the new century. In order to testify this new CPT-SVN-TODIM method, we apply this new proposed method to the assessment of medical emergency management. Now there are five regions’ medical emergency systems (
) awaiting evaluation. Five experts
(
) are invited to analyze six aspects of these systems. Additionally, the weighting vector of experts is
And then the six attributes respectively are: (1)
is the diagnostic testing capability; (2)
is the awareness of risk information; (3)
is the capability to process different sources of information; (4)
is the immunity from interference in analyzing information; (5)
is the capability of precision positioning; (6)
is the heterogeneous team coordination ability. Each expert’s assessment is shown in the .
Table 1. Decision matrix given by the expert
Table 2. Decision matrix given by the expert
Table 3. Decision matrix given by the expert
Table 4. Decision matrix given by the expert
Table 5. Decision matrix given by the expert
Based on the above information, by using EquationEqs. (13)(13)
(13) and Equation(15)
(15)
(15) , the single-valued neutrosophic group decision matrix
is obtained successfully, which is demonstrated in .
Table 6. Group decision matrix
Because the weight information is completely unknown, we use the entropy weight method, EquationEqs. (15)–(17), analyzing the information of group decision matrix and working out the original attribute weights
Then EquationEqs. (18)
(18)
(18) and Equation(19)
(19)
(19) are used to compute the modified weights
as listed in . (
based on the experiment of Tversky & Kahneman (Citation1992))
Table 7. The modified weights
Table 8. The modified weights
Table 9. The modified weights
Table 10. The modified weights
Table 11. The modified weights
Suppose
and
(Tversky & Kahneman, Citation1992), according to distances shown in , as well as the modified weights, we can acquire the relative predominance matrix
(
) under different attributes for each of the two alternatives.
Table 12. Distance between each of the two alternatives.
The overall predominance matrix is adding all relative predominance matrices together.
Finally, obtain the standard overall predominance (
) by utilizing EquationEq. (25)
(25)
(25) and the outcomes are
Therefore, the ordering of alternatives is
and the alternative
is the most excellent one.
It is obvious that the value of parameters just as can make a change in the above calculative outcome. And there is no doubt that we need to select the perfect parameters according to the problem we study. The responsibility of this paper isn’t to analyze the parameters but to establish a brilliant single-valued neutrosophic MAGDM model.
5. Comparative analysis
It is necessary to bring in other methods for verifying this new proposed CPT-SVN-TODIM method. In this part, we select some methods including SVNWA operator (Zhang et al., Citation2014), SVNWG operator (Zhang et al., Citation2014), TODIM method (Xu et al., Citation2017), TOPSIS approach (Nancy & Garg, Citation2019; Selvachandran et al., Citation2018), Single valued neutrosophic cross-entropy (Ye, Citation2014) and MABAC method (Peng & Dai, Citation2018) to compare with the new method in this paper.
From , we can come to the same conclusion that the alternative is the greatest, although there are subtle differences. However, the superiority of CPT-SVN-TODIM method, in describing decision maker’s psychological states about risk, reflects distinctiveness which keeps practicability of this new CPT-SVN-TODIM method. In addition, CPT-SVN-TODIM method also takes a more scientific approach to solving attribute weights for preventing subjective assumptions from adversely affecting the outcome. Hence, the above evidences suggest that the new proposed CPT-SVN-TODIM method is reliable and valid.
Table 13. The sequence from different methods.
6. Conclusions
The MAGDM issue is a very important in practical management decision-making all the time. With the continuous development of society, more and more situations can be classified as MAGDM. In such article, we tend to resolve the MAGDM issues with SVNSs and CPT-TODIM method and put forward the CPT-SVN-TODIM method. This new method not only inherits some advantages of classical TODIM method, but also has further improvement in some aspects. For example, we set up the entropy to calculate attribute weights for ensuring the more objective decision-making process. Furthermore, it is also an extension of MAGDM method to utilize SVNN to express decision makers’ ideas. In addition, we introduce the application of CPT-SVN-TODIM method in the assessment of medical emergency management. Finally, the reliability of CPT-SVN-TODIM algorithm is checked by comparing with other existed methods. In the future, we shall continue to explore the application of this method in some other different fields (Fan et al., Citation2021; Lu et al., Citation2021; Wei, Wu, et al., Citation2021)and make continuous improvement to build more scientific and reasonable new methods to solve MAGDM issues (Jin et al., Citation2021; Kumar et al., Citation2021; Xu et al., Citation2021; Zhao, Wei, Guo, et al., Citation2021).
Disclosure statement
No potential conflict of interest was reported by the authors.
References
- Agrebi, M., & Abed, M. (2021). Decision-making from multiple uncertain experts: Case of distribution center location selection. Soft Computing, 25(6), 4525–4544. https://doi.org/10.1007/s00500-020-05461-y
- Atanassov, K. T. (1986). Intuitionistic fuzzy sets. Fuzzy Sets and Systems, 20(1), 87–96. https://doi.org/10.1016/S0165-0114(86)80034-3
- Cuong, B. C. (2014). Picture fuzzy sets. Journal of Computer Science and Cybernetics, 30, 409–420.
- Davoudabadi, R., Mousavi, S. M., & Mohagheghi, V. (2020). A new last aggregation method of multi-attributes group decision making based on concepts of TODIM, WASPAS and TOPSIS under interval-valued intuitionistic fuzzy uncertainty. Knowledge and Information Systems, 62(4), 1371–1391. https://doi.org/10.1007/s10115-019-01390-x
- Dorfeshan, Y., & Mousavi, S. M. (2020). A novel interval type-2 fuzzy decision model based on two new versions of relative preference relation-based MABAC and WASPAS methods (with an application in aircraft maintenance planning). Neural Computing and Applications, 32(8), 3367–3385. https://doi.org/10.1007/s00521-019-04184-y
- Fan, J. P., Yan, F., & Wu, M. Q. (2021). GLDS method for multiple attribute group decision making under 2-Tuple linguistic neutrosophic environment. Journal of Intelligent & Fuzzy Systems, 40(6), 11523–11538. https://doi.org/10.3233/JIFS-202748
- Gomes, L., Gomes, C. F., & Rangel, L. (2009). A comparative analysis with THOR and TODIM: Rental evaluation in Volta Redonda. Revista Tecnologia, 30, 7–11.
- Guo, F., Hu, M. C., Duffy, V. G., Shao, H., & Ren, Z. G. (2021). Kansei evaluation for group of users: A data-driven approach using dominance-based rough sets. Advanced Engineering Informatics, 47, 101241. https://doi.org/10.1016/j.aei.2020.101241
- He, T., Wei, G., Wu, J., & Wei, C. (2021). QUALIFLEX method for evaluating human factors in construction project management with Pythagorean 2-tuple linguistic information. Journal of Intelligent & Fuzzy Systems, 40(3), 4039–4050. https://doi.org/10.3233/JIFS-200379
- Huang, H. L. (2016). New distance measure of single-valued neutrosophic sets and its application. International Journal of Intelligent Systems, 31(10), 1021–1032. https://doi.org/10.1002/int.21815
- Huang, Y., Lin, R., & Chen, X. (2021). An enhancement EDAS method based on prospect theory. Technological and Economic Development of Economy, 27(5), 1019–1038. https://doi.org/10.3846/tede.2021.15038
- Ji, P., Wang, J. Q., & Zhang, H. Y. (2018). Frank prioritized Bonferroni mean operator with single-valued neutrosophic sets and its application in selecting third-party logistics providers. Neural Computing and Applications, 30(3), 799–823. https://doi.org/10.1007/s00521-016-2660-6
- Ji, X. N., Yu, L. X., & Fu, J. P. (2020). Evaluating personal default risk in P2P lending platform: Based on dual hesitant pythagorean fuzzy TODIM approach. Mathematics, 8(1), 8.
- Jin, X. W., Ji, Y., & Qu, S. J. (2021). Minimum cost strategic weight assignment for multiple attribute decision-making problem using robust optimization approach. Computational & Applied Mathematics, 40, 193.
- Jurkowska, B. (2014). The Federal States of Germany—Analysis and measurement of development using taxonomic methods. Oeconomia Copernicana, 5(3), 49–73. https://doi.org/10.12775/OeC.2014.019
- Kumar, J., Cannbron-Mellott, M. J., Tencer, T., Will, O., Mackie, D. S., & Beusterien, K. (2021). Patient and neurologist preferences in the United States for relapsing-remitting multiple sclerosis treatments: Findings from a discrete choice experiment. Patient Preference and Adherence, 15, 1515–1527.
- Lei, F., Wei, G., & Chen, X. (2021). Model-based evaluation for online shopping platform with probabilistic double hierarchy linguistic CODAS method. International Journal of Intelligent Systems, 36(9), 5339–5358. https://doi.org/10.1002/int.22514
- Liang, Y. Y., Tu, Y., Ju, Y. B., & Shen, W. J. (2019). A multi-granularity proportional hesitant fuzzy linguistic TODIM method and its application to emergency decision making. International Journal of Disaster Risk Reduction, 36, 101081. https://doi.org/10.1016/j.ijdrr.2019.101081
- Lin, M. W., Wang, H. B., & Xu, Z. H. (2020). TODIM-based multi-criteria decision-making method with hesitant fuzzy linguistic term sets. Artificial Intelligence Review, 53(5), 3647–3671. https://doi.org/10.1007/s10462-019-09774-9
- Liu, D. H., Liu, Y. Y., & Wang, L. Z. (2019). Distance measure for Fermatean fuzzy linguistic term sets based on linguistic scale function: An illustration of the TODIM and TOPSIS methods. International Journal of Intelligent Systems, 34(11), 2807–2834. https://doi.org/10.1002/int.22162
- Long, X. L., Liu, L. Z., Xiao, C., Cheng, P. F., & Fu, C. X. (2020). Restoration Methods Selection for Wood Components of Chinese Ancient Architectures Based on TODIM with Single-Valued Neutrosophic Sets. Mathematical Problems in Engineering, 2020, 1–14. https://doi.org/10.1155/2020/5049360
- Lu, J., Zhang, S., Wu, J., & Wei, Y. (2021). COPRAS method for multiple attribute group decision making under picture fuzzy environment and their application to green supplier selection. Technological and Economic Development of Economy, 27(2), 369–385. https://doi.org/10.3846/tede.2021.14211
- Lu, Z. M., Gao, Y., & Zhao, W. H. (2020). A TODIM-based approach for environmental impact assessment of pumped hydro energy storage plant. Journal of Cleaner Production, 248(3), 119265.
- Nancy, & Garg, H. (2019). A novel divergence measure and its based TOPSIS method for multi criteria decision-making under single-valued neutrosophic environment. Journal of Intelligent & Fuzzy Systems, 36, 101–115.
- Peng, J. J., Tian, C., Zhang, Z. Q., Song, H. Y., & Wang, J. Q. (2019). Single-valued neutrosophic power shapley choquet average operators and their applications to multi-criteria decision-making. Mathematics, 7(11), 1081.
- Peng, X. D., & Dai, J. G. (2018). Approaches to single-valued neutrosophic MADM based on MABAC, TOPSIS and new similarity measure with score function. Neural Computing and Applications, 29(10), 939–954. https://doi.org/10.1007/s00521-016-2607-y
- Sahin, R., & Kucuk, A. (2014). On similarity and entropy of neutrosophic soft sets. Journal of Intelligent & Fuzzy Systems, 27, 2417–2430.
- Selvachandran, G., Quek, S. G., Smarandache, F., & Broumi, S. (2018). An extended technique for order preference by similarity to an ideal solution (TOPSIS) with maximizing deviation method based on integrated weight measure for single-valued neutrosophic sets. Symmetry, 10(7), 236. https://doi.org/10.3390/sym10070236
- Smarandache, F. (2002). A unifying field in logics: Neutrosophic logic. Multiple-Valued Logic, 8(3), 297–384.
- Sun, R. X., Hu, J. H., & Chen, X. H. (2019). Novel single-valued neutrosophic decision-making approaches based on prospect theory and their applications in physician selection. Soft Computing, 23(1), 211–225. https://doi.org/10.1007/s00500-017-2949-0
- Tehreem, Hussain, A., Lee, J. R., Khan, M. S. A., & Shin, D. Y. (2021). Analysis of social networks by using pythagorean cubic fuzzy Einstein weighted geometric aggregation operators. Journal of Mathematics, 2021, 5516869. https://doi.org/10.1155/2021/5516869
- Tian, X. L., Xu, Z. S., & Gu, J. (2019). An extended TODIM based on cumulative prospect theory and its application in venture capital. Informatica, 30(2), 413–429. https://doi.org/10.15388/Informatica.2019.212
- Tversky, A., & Kahneman, D. (1992). Advances in prospect theory: Cumulative representation of uncertainty. Journal of Risk and Uncertainty, 5(4), 297–323. https://doi.org/10.1007/BF00122574
- Verma, R. (2021). Fuzzy MABAC method based on new exponential fuzzy information measures. Soft Computing, 25(14), 9575–9589. https://doi.org/10.1007/s00500-021-05739-9
- Wang, H., Smarandache, F., Zhang, Y. Q., & Sunderraman, R. (2010). Single valued neutrosophic sets. Multispace and Multistructure, 2010, 410–413.
- Wei, G., Wei, C., & Guo, Y. (2021). EDAS method for probabilistic linguistic multiple attribute group decision making and their application to green supplier selection. Soft Computing, 25(14), 9045–9053. https://doi.org/10.1007/s00500-021-05842-x
- Wei, G., Wu, J., Guo, Y., Wang, J., & Wei, C. (2021). An extended COPRAS model for multiple attribute group decision making based on single-valued neutrosophic 2-tuple linguistic environment. Technological and Economic Development of Economy, 27(2), 353–368. https://doi.org/10.3846/tede.2021.14057
- Wen-Ran, Z. (1994). Bipolar fuzzy sets and relations: A computational framework for cognitive modeling and multiagent decision analysis. In NAFIPS/IFIS/NASA '94. Proceedings of the First International Joint Conference of the North American Fuzzy Information Processing Society Biannual Conference. The Industrial Fuzzy Control and Intellige (pp. 305–309).
- Wu, H. B., Yuan, Y., Wei, L. J., & Pei, L. D. (2018). On entropy, similarity measure and cross-entropy of single-valued neutrosophic sets and their application in multi-attribute decision making. Soft Computing, 22(22), 7367–7376. https://doi.org/10.1007/s00500-018-3073-5
- Xu, C. B., Ke, Y. M., Li, Y. B., Chu, H., & Wu, Y. N. (2020). Data-driven configuration optimization of an off-grid wind/PV/hydrogen system based on modified NSGA-II and CRITIC-TOPSIS. Energy Conversion and Management, 215, 112892. https://doi.org/10.1016/j.enconman.2020.112892
- Xu, D. S., Hong, Y. R., & Xiang, K. L. (2019). A method of determining multi-attribute weights based on single-valued neutrosophic numbers and its application in TODIM. Symmetry-Basel, 11, 12.
- Xu, D. S., Wei, C., & Wei, G. W. (2017). TODIM method for single-valued neutrosophic multiple attribute decision making. Information, 8(4), 125. https://doi.org/10.3390/info8040125
- Xu, D. S., Wei, X. L., Ding, H., & Bin, H. Q. (2020). A new method based on PROMETHEE and TODIM for multi-attribute decision-making with single-valued neutrosophic sets. Mathematics, 8(10), 1816. https://doi.org/10.3390/math8101816
- Xu, W. H., Shang, X. P., & Wang, J. (2021). Multiple attribute group decision-making based on cubic linguistic Pythagorean fuzzy sets and power Hamy mean. Complex & Intelligent Systems, 7(3), 1673–1693. https://doi.org/10.1007/s40747-020-00255-z
- Yager, R. R. (2014). Pythagorean membership grades in multicriteria decision making. IEEE Transactions on Fuzzy Systems, 22(4), 958–965. https://doi.org/10.1109/TFUZZ.2013.2278989
- Ye, J. (2014). Single valued neutrosophic cross-entropy for multicriteria decision making problems. Applied Mathematical Modelling, 38(3), 1170–1175. https://doi.org/10.1016/j.apm.2013.07.020
- Zadeh, L. A. (1965). Fuzzy sets. Information and Control, 8(3), 338–356. https://doi.org/10.1016/S0019-9958(65)90241-X
- Zanon, L. G., Marcelloni, F., Gerolamo, M. C., & Carpinetti, L. C. R. (2021). Exploring the relations between supply chain performance and organizational culture: A fuzzy grey group decision model. International Journal of Production Economics, 233, 108023. https://doi.org/10.1016/j.ijpe.2020.108023
- Zhang, H. Y., Wang, J. Q., & Chen, X. H. (2014). Interval neutrosophic sets and their application in multicriteria decision making problems. TheScientificWorldJournal, 2014, 645953. https://doi.org/10.1155/2014/645953
- Zhang, S., Gao, H., Wei, G., & Chen, X. (2021). Grey relational analysis method based on cumulative prospect theory for intuitionistic fuzzy multi-attribute group decision making. Journal of Intelligent & Fuzzy Systems, 41(2), 3783–3795. https://doi.org/10.3233/JIFS-211461
- Zhao, M., Li, L. Y., & Xu, Z. H. (2021). Study on hotel selection method based on integrating online ratings and reviews from multi-websites. Information Sciences, 572, 460–481. https://doi.org/10.1016/j.ins.2021.05.042
- Zhao, M., Wei, G., Chen, X., & Wei, Y. (2021). Intuitionistic fuzzy MABAC method based on cumulative prospect theory for multiple attribute group decision making. International Journal of Intelligent Systems, 36(11), 6337–6359. https://doi.org/10.1002/int.22552
- Zhao, M., Wei, G., Guo, Y., & Chen, X. (2021). CPT-TODIM method for interval-valued bipolar fuzzy multiple attribute group decision making and application to industrial control security service provider selection. Technological and Economic Development of Economy, 27(5), 1186–1206. https://doi.org/10.3846/tede.2021.15044
- Zhao, M., Wei, G., Wei, C., & Wu, J. (2021). Pythagorean fuzzy TODIM method based on the cumulative prospect theory for MAGDM and its application on risk assessment of science and technology projects. International Journal of Fuzzy Systems, 23(4), 1027–1041. https://doi.org/10.1007/s40815-020-00986-8