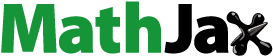
ABSTRACT
In the kingdom of Saudi Arabia almost 60% of the construction projects were delayed in the recent decade. Contractor selection is the critical decision for the success of any construction project. The typical method of contractor selection in Saudi Arabian projects is generally based on prioritizing the lowest bid price, irrespective of experience, quality, and other factors without investigating in-depth the pre-qualification of contractors. This often leads to disputes and project delays. In this regard, the main goal of the presented research work is the development of a framework that can help decision-makers in both government and private sector to investigate the pre-qualification, and then to select the most suitable contractors for the proposed projects. The presented framework was developed based on a detailed literature review, market analysis, information collection from stakeholders and decision-makers along with collaboration with industry-academia experts. Forty criterion-based selection framework was developed in the presented research work with each criterion possessing a relative weightage developed in comparison with ones obtained using smart techniques. The main categories of the selection criteria are project value, financial capability, reputation, management structure, technical capability and performance, organizational culture, along with safety and environmental health. Through detailed survey and analysis, the main criterion for contractor selection was identified as quality assurance and control with the least important being the Current/Past Joint Venture(s). The presented framework model was validated using a case study where three construction contractors were evaluated using Multi-Attribute Utility Theory Score (MAUT). The framework was deemed reliable and valuable for contractor selection in construction projects for adding the increased selection criterion and insight into the selection evaluation.
1. Introduction
The global construction industry is expected to grow to an estimated USD 8 trillion in 2030, which will increase to USD 17.5 trillion by 2030. A growth of 85%, with an annual rate of 3.9% is reached. The Middle East and Africa are set to represent 6% of the global construction market in 2030 (Paul Citation2015). In 2016, the Kingdom of Saudi Arabia (KSA) launched the Saudi “Vision 2030,” a strategic plan that sets out the new economic direction for KSA aimed to raise efficiency and performance while also reducing financial waste, non-transparency, and corruption in all sectors of the economy (Beale and Company Citation2016). The construction sector in Saudi Arabia is second in contributing to the gross domestic product, only surpassed by the petroleum sector (SCA Citation2018). The construction industry in Saudi Arabia grew at a rate of 6.5% annually from 2014 to 2016 and is predicted to grow at a rate of 7.5% annually from 2016 to 2020. The amount of investment in the construction industry in Saudi Arabia is estimated at USD 84 billion in 2016 (Jeddah Chamber, Construction and real-estate sector, Technical report, Citation2016). Saudi Arabia’s construction sector plays a key role in supporting and developing the kingdom’s infrastructure and other sectors (AlMutairi, Saud, Augest Citation2017).
The selection of the most suitable contractor for a proposed construction project poses a challenge to private and government entities. Since construction projects vary from small scale projects to large megaprojects, the selection of a professional, knowledgeable contractor team plays a key role in securing the success of the
project. A well-designed project can suffer delays and disruptions owing to a lack of a suitable construction contractor. These disruptions and problems often cause financial disputes and project delays, and they could lead to substandard work and instability in a project. In this regards the selection of a suitable contractor is of prime importance for any project. Traditionally, contractors for any proposed construction project are selected using the lowest bid method. Contractors who are short of work or projects may bid more aggressively in the hope that they might win a project (Gary et. al. Citation1995). In case the project is awarded to the lowest bidding contractors; they need to cut their expenses in new ways so that they can complete the project without losing money (Zedan et. al. Citation1998). This can lead to poor quality of work and reduced performance of the project. For large construction projects, the most essential factor to consider when selecting the most appropriate contractor is to minimize the chances of a project implementation catastrophe in case a contractor fails to successfully execute the project. A reliable set of standards is thus required to be sure that each bidder’s professional capacity and quoted price can be realistically evaluated so that the most competent and cost-effective contractor will be selected to perform the construction of the proposed project (Halil Citation2007; Zavadskas, Peldschus, and Kaklauskas Citation1994; Sodangi, Salman, and Saleem Citation2018; Chee, Gary, and Patricia Citation2010; Saleem et al. Citation2019; Saleem and Hosoda Citation2021; Daniel et al. Citation2020). The Ministry of Municipal and Rural Affairs spent more than SR 12.5 billion on projects in KSA cities and provinces from 2014 to 2015. The percentage of delay on these projects averaged over 40% as shown in (Contracts and Projects, Technical report, 2015/2016, AlBogamy, Scott, and Dawood Citation2012). Several causes were identified as causing the delay of the projects with the main factors circulating around the contractor’s performance.
Figure 1. Delayed and completed projects in KSA (Contracts and Projects, Technical report, 2015/2016).
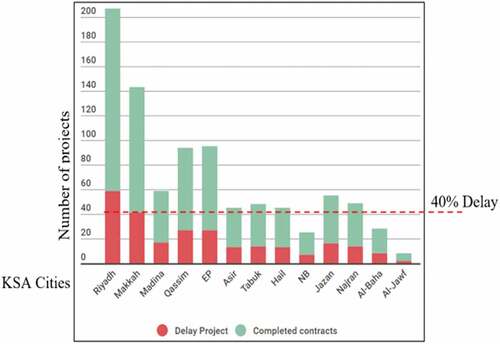
According to the process of contractor selection, the Saudi government has been highly dependent on the low bid delivery method for contractor selection, with the classification of contractors made according to prequalified methods as carried out by the Saudi Ministry of Municipal and Rural Affairs (AlMutairi Citation2017). Classification plays a key role in contractor selection. The Classification Agency has been responsible
for rating and classifying contractors with special rules and specific criteria and supervises the issuing of classification certificates. This certificate is a primary requisite for Saudi or foreign contractors to participate in government or non-government tenders (Tasneef, 2015). The criteria of contractor evaluation are based on the Ministry of Municipality of Rural Affairs requirements as detailed below (CSA, Citation2017);
2. Technical evaluation
Workforce or available manpower
Construction equipment
Current projects (ongoing)
Evaluation of the technical staff
Client satisfaction
3. Financial evaluation
Company capital/ working capital
Financial statements.
The pre-qualification method is commonly adopted to identify reliable contractors and it is a process in which contractors are compared based on key criteria. These criteria include financial stability, similar experience, and performance/client satisfaction. The pre-qualification process includes screening contractors using the criteria selected by the individual owners. This method is preferred by clients to minimize the risk of failure (Danial et. al. Citation2020; Chee, Gary, and Patricia Citation2010) as shown in . Researchers in the past have investigated the contractor selection criterion and have categorized them in technical, financial, management and health and safety aspects of evaluation (Othman, Citation2012, Aladdin et. al. Citation2019). Chinang calculated weighted values for technical ability, management capability, financial stability, reputation, and health and safety performance, while (Alshamrani Citation2012; Edumndas et. al. Citation2015; Zedan et. al. Citation1997; Turskis Citation2008), employed multi-attribute decision-making tools for ranking the criteria based on importance index. Abu Nemeh (Citation2012) and Al-Harbi (Citation2001) showed that three out of sixteen criteria constitute about 50% of the impact on the final decision of construction contractor selection. These criteria were (A) contractor submitted complete bid document (B) contractor classification and (C)bid price. Aprna et. al. (Citation2018) proposed a process to evaluate contractors using three new selection factors in addition to the bid price such as time quotes, warranty period, and past performance of past. The study affirmed that the contractor’s selection played a huge role in projects and their success/failure. Somboonpisan et. al. (Citation2021) developed a sustainable bidding model to measure the weight of potential environmental impacts utilizing the amount of CO2 emission generated from construction equipment in fleet operations for high-way projects. Chen et al. (Citation2020) introduced a multi-attribute group decision-making approach for the management and manipulation of bidder selection. The model consists of two-stage logic scoring of preference applying ELECTRE III approach by using fuzzy and nonelinear optimization technique while considering the reasoning logic pattern in human cognition process. Chen et al. (Citation2021) also proposed integrated objective and subjective approach to compute criterion weights via applying of an ELECTRE III-based method that incorporates HFLTS possibility distributions, which enables descision makers in addressing the uncertainty, imprecision, indetermination, and embedded in evaluation of alternative-criterion decisions in the bidding process. Liu, Zhang, and Qi (Citation2021) developed a tripartite game model between the management department, the business experts, and the technical experts is constructed for grouped multiattribute reserve auctions. the factors that affect the equilibrium behavior of each player and the static equilibrium strategies are analyzed by evolutionary game theory. Khoso et al. (Citation2021) developed alternative system of dealing with the embedded group decision under a remote group decision environment by integrating Exploratory Factor Analysis and MACBETH. It was found that an embedded remote group decision making system could serve as an alternative system of group decision making which has plentiful perks in group decision applications.
Figure 2. Pre-qualification diagram (Chee, Gary, and Patricia Citation2010).
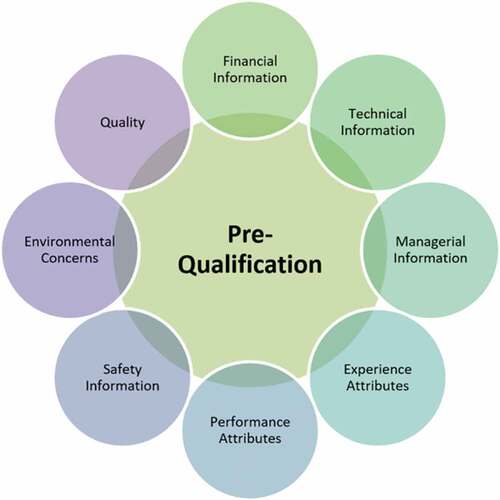
In light of the above-presented discussion, it is evident that in the past research work presented there is a distinct lack of research addressing the criteria for contractor selection in the Saudi Arabian. The contractor evaluation process and selection of bidders require critical knowledge and experience for a client to have confidence that a selected contractor will be able to successfully execute a project. Since, KSA is a growing economy with massive investment in the construction sector, hence any development in this avenue will hold immense value for the decision-makers of the country.
4. Problem statement
Recently, the percentage of delays on government projects in the Kingdom of Saudi Arabia reached 60% (Ismail, Citation2013). One of the fundamental causes identified during the literature review process has been the contractor selection process (Chinang et. al. 2017, Othman 2012, Aladdin et. al. Citation2019). Since the Kingdom of Saudi Arabia is experiencing rapid growth in infrastructure development under Vision 2030, hence, an advanced contractor selection approach is much needed to meet the growing challenges. Furthermore, the development proposed for KSA can also be adopted for other developing countries for contractor selection purposes. In light of the above-presented discussion, the main goal of the presented research work is to develop a framework that will assist both the government and private sectors to evaluate the pre-qualification of the nominated contractors and then choose the most suitable one for construction projects in KSA. The presented research work consisted of three phases: (A) developing the input, which consisted of a selection of criteria based on multiple resources (B) processing, which encompassed data collection and analysis of the developed framework process and (C) evaluating the output with a testing validation to then make the final contractor selection.
5. Methodology
presents the 21-criterion studied in the presented research work. These criteria were selected based on the detailed literature review. These 21 factors remain common in Saudi Arabia and other countries. However, Saudi Arabia nowadays experiences rapid expansion and exponential growth in terms of construction projects and development, hence, it was required to go for further details depth of it (total of 40 factors) that gathered from experts in construction industry. However, this framework allows for modifications of selection criteria and further input from different stakeholders. This research methodology for investigating the presented criterion was divided into three main stages. The stages were named Input, Process, and output. The current section details the methodology adopted for the presented research investigation.
Table 1. Summary of the researchers’ 21 contractor selection criteria.
The first stage was the “Input”, which covered the literature review required to determine the criteria with which to select a construction contractor. The criteria were selected from the literature survey, data/feedbackfrom the Contractor Classification Agency, and expert’s opinion communicated via a survey conducted in the academic and construction field. The second stage titled the “Process”, addressed the information evaluation, ranking, and data processing methodology of the research team. At this stage gaps in information and data collected were identified and criterion was selected to fill these gaps using smart techniques. Furthermore, analysis of all the collected information and the development of the conclusion and recommendations are also presented for the reader’s interest. At the “Processing” stage, once all the information and data were assembled from the “Input” phase, a questionnaire, and interviews were conducted with a government official, private-sector decision-makers, and academia working in the construction sector of KSA. The questionnaire was designed to gather the information needed to complete the data previously collected, along with the expert’s feedback that would be employed for the pilot study to identify a contractor to select for work on a project. In the end, the total highest score was selected as an indicator of the most suitable contractor. The last stage, “Output,” was where the results were compiled from the “Process” phase and analyzed with respect to the criteria. After these results were analyzed, the information has been verified as complete, the result with the final contractor selection is presented as shown in .
5.1. Pilot study & criterion development
To develop an effective questionnaire, the research team decided on conducting a pilot study. The aim of the pilot study was conducted gauge the level of understanding, verification, and enhancement of the proposed questionnaire. 7% of the distributed questionnaire were categorized as a pilot study. The rationale of choosing 7% as a pilot study was to aim in the middle of 5–10% value of the distributed questionnaire. Hence, the percentage studied in the pilot study has a rational background. Based on the feedback of the pilot study, several modifications were incorporated into the final version of the questionnaire with 40 criteria were revised and confirmed through consultations with the experts as presented in . A total of 266 questionnaires were distributed via interviews and through an online survey (using QuestionPro). presented the combination of the criterion used for the investigation. It also shows that 21 criteria were gathered from literature and previous similar works. 5 criteria were introduced by experts who are acting as project and general managers, directors of construction, maintenance, and operation support services in governmental sectors, and contract and procurement managers. The last 14 criteria are proposed by academics who hold Ph.D. in construction and management with field experience and acting as project management advisors and consultants in governmental projects. The criteria were divided into seven major classes, and each class consisted of sub-criterions. These classes were: 1. Project Value, 2. Financial Capability, 3. Reputation, 4. Technical Capability and Performance, 5. Management Ability, 6. Organization’s Culture, and 7. Environmental Safety and Health. Each of the 40-criterion listed in were classified in the above-mentioned 07 classes. Furthermore, for in-depth investigation and analysis, each of the 40 criteria were also further sub-divided into sub-criterions. The aim of the team for this step was to use each description for clarifying to the questionnaire readers and also to use the results for in-depth analysis. presents the details of each criterion, its classification, and sub-criterions. The classification and sub-classes were developed based on a detailed literature review and by consultation with academic and construction experts. The survey results of 266 participants were analyzed using the strategy outlined in . The results obtained from the users were normalized and relative weightage was calculated. The Multi-Attribute Utility Theory Score (MAUT) was then applied to identify the effectiveness and efficiencies of the proposed framework. presents the enlarged methodology adopted for the presented research work. Once the framework was developed using the participant’s feedback, three contractors were selected for validating the proposed framework. The proceeding section details the methodology adopted by the research team for data analysis and using smart techniques to fill in the gaps.
Table 2. Questionnaire survey for the weighting of the criteria on a 1–10 scale.
Table 3. The final selected criteria, incorporating input from construction field experts.
5.2. Data collection and analysis
This section details the data analysis and feedback received from the survey participants. A total of 266 survey questionnaires were distributed to members of the construction industry ranging from governmental ministries to private sectors employees and academia. The research team aimed to develop a wide view of opinions related to the contractor selection criterion prevailing in the KSA construction industry. presents the results of the percentage of the participants who responded to the questionnaire survey. It can be seen that 38% of the questionnaires were returned with completed expert’s responses, which represents 100 responses in total. 166 incomplete questionnaires were received during the survey timeline. These were removed from the data collection, and another 22 were excluded by using the correlation statistics (≤ 0.1). The remaining 78 approved responses are used for detailed analysis in the presented research study.
Out of the 78 approved reliable responses, three participants resided outside the Kingdom of Saudi Arabia. Furthermore, the questionnaires collected from the structured interviews had 60 responses. presents the variation of the received questionnaire participants. From the results, it is evident that the respondents included a range of members from the construction industry. This is a healthy sign from the standpoint of reliability and value of the data analysis. The results indicate that questionnaire respondent consisted of 29 Project Managers (PMs), 5 Area Contract Managers (ACMs), 5 Construction Managers (CMs), 14 ProjectEngineers (PEs), 9 Engineers (ENGs), 5 Resident Engineers (REs), and 11 Architects (ARCHs). Moreover, the age of some of the respondents (PMs, ACMs, and CMs) was between 36–60 years old with 15 to 30 years of experience, while the age of other respondents (ENGs, REs and ARCHs) was between 30–40 years old with 8 to 12 years of experience. The reach team also reached out to at least 100 participants in the field to fill in the questionnaire as shown in . details the sector division of the survey respondents. From the figure, it is evident that the survey results comprised of a reasonable mix of responses from the sectors. This intern adds value to the analysis of the presented data. The questionnaire results indicate that the respondents were employed by the government (17), by semi-governmental agencies (38), and in the private sector (23). presents the response criteria analysis of the survey results from semi-government, government, and private sector participants.
Table 4. The responses criteria analysis.
Table 5. Normalization of the criteria.
This study requires the following matrix
6. Normalization
presents the normalization of the criteria, where each column for each entry in the column was also divided using the column sum to yield all the normalized scores and the sums for each of the columns is equal to 1. These were further used to calculate the weights of each criterion. present the normalized percentage of criteria. These results assisted the research team in identifying the most important and least important parameters related to
contractor selection in the construction industry of KSA. Weightings are the equivalent of having several similar items with the same value involved in the average. The weight is calculated based on one of the highest expert’s weights. The total weight for each main category shall be equal to 1. The procedure to calculate the weighted average is as detailed below;
Where EW is the expert weight and C is a criterion. The similarity between the weighted average and the average of the criterion represents 80%. The relative weights for the criteria were computed for every respondent, followed by mean, median, and the standard deviations calculations accordingly. The relative weight shows the Median, Average, and Standard Deviation for the forty criteria. presents the result related to the Standard Deviation (STDV) for the main construction contractor selection criteria categories. Upon completion of quality assurance checking of the received results. The data were analyzed to develop the framework. The proceeding section details the testing and validation of the proposed framework for contractor selection in construction projects of KSA.
7. Analysis results
78 responses for construction contractor selection criteria passed the statistical tests and could thus be utilized in this evaluation. The relative weights of the pre-qualification and selection criteria were calculated for every respondent, along with the mean, median, mode, and standard deviation. The relative weights of the pre-qualification and selection criteria were then calculated based on the mean/average. Afterward, the average relative weights were calculated. Finally, the Eigen Vector weights were calculated by using the geometric mean calculated for each criterion using the equation. The geometric mean and Eigen Vector weight for each of the 40 criteria are listed in . Using the EV weights, the results of the seven main criteria categories were displayed as a percentage of their perceived importance. The percentage represented the importance of the category with regards to others involved in the analysis. These totals were 8% for the project value, 15% for financial capability, 15% for reputation, 18% for technical capability and performance, 20% for management ability, 11% for the organization’s culture, and 13% for environmental safety and health.
Table 6. Geometric and EV Weight Calculation.
Detailed analysis was conducted to analyze the distribution of weights in each sub-category. From the analysis, it was evident that the project value category criteria were divided almost evenly: 35% for the lists of current projects, 35% for lists of completed projects, and 30% for the total awarded projects. The financial capability category criteria were divided into the following percentages: 17% for the company’s capital & working capital, 19% for its financial statement, 16% for the credit facility, 17% for credit history, 16% for insurance coverage, and 15% for performance bonds.
The reputation category criteria were fairly evenly distributed in terms of their percentages: 18% for the Ministry of Municipal and rural affairs’ classification certificate for “Building”, 15% for the Saudi contractors’ authority membership, 18% for past performance, i.e., failure and success record, 16% for claims and contractual disputes, 18% for overall project satisfaction (past projects, clients, consultants, suppliers), and 15% for the reputation of sub-contractors & long lead item suppliers.
The technical capability and performance category criteria were divided almost evenly in terms of their importance: 14% for certifications/official documents, 16% for previous experience, 14% for construction plant and equipment, 15% for manpower/workforce resources/outsourced workforce, 15% for the experience of the technical staff, 14% for technical advantages (know-how), and 12% for the logistical plans.
The management ability and performance category criteria were almost evenly distributed, with the least-important criterion (head office & sub-office locations) at 9% only 5% behind the three most important criteria: quality assurance & quality control plan, management expertise, and management staff availability, which each had 14% of the total importance. The other categories were close behind, with 13% for the planning & scheduling department, 13% for staff qualification/certified 12% for management tools & technology, and 11% for continuous project monitoring and time-lapse updates.
The organization’s culture category criteria were divided in their importance as follows: 21% for the estimating department, 20% for awareness of the local working culture, 21% for familiarity with the regulating authority/ties, 22% for knowledge of the local environmental conditions, and 16% for current /past joint ventures.
The environmental safety and health category criteria were almost evenly divided: 19% for incident records, 22% for the company safety policy, 19% for an OSHA/HSE certificate, 19% for medical insurance, and 21% for employment and training.
The expert’s judgments were calculated, verified, and the consistency of all the judgments was deemed as satisfactory. The relative weights for each construction contractor pre-qualification and selection criteria were analyzed with indicating the EV weight and ranking for all 40 criteria, grouped by their category. The weights used to determine the ranking were calculated by multiplying the relative weights for each sub-criterion and the corresponding upper element. Afterward, these criteria were ranked in descending order based on the priority EV weight or importance of each criterion as presented in . The percentage of the difference between the first (most important) criteria and the last (least important) one was 63%. The proceeding section details the procedure adopted for the validation of the proposed framework.
Table 7. Ranked important criteria.
8. Framework test and validation
The objective of the proposed framework is to assist the decision-makers in selecting the most suitable candidate for the contractor position needed to execute the project. presents the contractor selection process and assessment flowchart. The selection of the contractor in the presented proposed framework primarily depends on the type of contract and project delivery method. For instance, the framework is suitable for two types of contracts such as negotiated contracts and competitive contracts as shown in . The negotiated contract and delivery method consist of construction and design-construction contracts while the competitive approach includes design-bid-construction and A + B contracts. The selection of the most suitable contractor in the negotiated approach is primarily governed by the contractor evaluation against the selection criteria while no bidding is needed to complete the process. In such a case the most suitable contractor is selected based on the highest obtained score according to the contractor’s performance against the 40 prequalification criteria. On the other hand, the selection of the best contractor for the competitive contracts will be conducted based on both prequalifications that have the 40 selection criteria and bid price as shown in . The contractors’ performance will be first examined against the 40 prequalification criteria. A limited number of contractors with a high obtained score will only be selected to continue for the next stage which includes the bidding and contract price assessment. The most suitable contractor will be then selected based on the highest overall obtained score achieved at both stages i.e. prequalification and bid price assessment. In light of the presented research work, the team decided to validate the proposed framework using a case study. The objective of the proposed case study was to test the proposed framework under real-world application conditions. indicates the steps involved in the validation of the proposed framework for optimized contractor selection for construction projects in KSA. In light of this objective three contractors were selected to test the validity of the presented framework.
These contractors were selected based on the criterion that all three were planning to submit a bid on a major construction project in the eastern region of KSA. Furthermore, these three contractors were among the top-tier contractors in the kingdom. Hence, their selection had a logical background.
To evaluate the developed contractor assessment framework, interviews were conducted with the selected construction contractors. Meetings were arranged with these three contractors A, B, and C, who were assisted by the Royal Commission in Al-Jubail Industrial City (the government agency requesting bids) and classified by the Saudi Classification agency. The three contractors were preparing to bid on the residential projects in Al-Jubail industrial city. The criteria for pre-qualification and selection as proposed in the presented research work were applied to each contractor. After receiving the feedback from all three construction contractors, the analysis was applied to all of their responses. presents the ranked criterion after the analysis of results obtained from the three contractors. Each contractor was evaluated using the 40 criteria developed using the proposed framework. presents the utility curve obtained from the analysis of the presented results. The weight of the utility score for each criterion was calculated from the formula of the utility curve. For example: At criterion 2 the formula used is y = 0.068x – 0.2204 = (0.068*16)-0.2204 = 0.867 = weight for the utility score for criterion 2. shows the utility score for the three contractors. The total score value for each criterion is then calculated using the formula as detailed below.
Table 8. The preference of pre-qualification and selection criteria 2.
Table 9. The contractor score.
After applying the Utility Score calculation for each of the three contractors, the framework indicated the percentage of the suitability scores for each contractor. The total scores were 73 for contractor A, 75 for contractor B, and 78 for contractor C. The contractor obtaining the highest percentage was, therefore, contractor C as shown in . In summary, it can be stated that the contractor who obtains a high percentage, as well as a competitive bidding price, will be the most
qualified to be awarded the project. The most-preferred alternative is the one with the highest score. Government ministries and organizations, as well as the private sector companies, can adjust the criteria based on their requirements, and these requirements will also vary according to project type. Hence, the proposed framework provides in-depth insight into the contractor pre-qualification and selection process and evades the delays associated with the lowest bid selection criterion.
9. Conclusion
The construction industry is massive and complex. Traditionally the lowest bid selection criterion has been prevalent in the construction industry of the kingdom of Saudi Arabia. However, owing to massive delays cost overrun, and poor quality of the finished project. It is necessary to develop an in-depth detailed framework that can take into consideration all possible aspects of a contract’s performance to select the most suitable candidate for the construction of a proposed project. In this regard, the contribution of this study was developing of comprehensive pre-qualification and selection framework for construction projects contractors in KSA. A total of 40 qualification criteria that govern the assessment and selection process were identified in this study based on data gathered from literature, academics, and construction experts. Hence, the developed framework will assist government and buildings owners to firstly identify the qualification of the competitive contractors and then to select the most appropriate one. The following conclusion can be drawn from the presented research work;
The selection process is quite inappropriate, as it considers price as the main criterion for awarding projects. One major benefit of the proposed framework is that it will enhance the process of evaluating the pre-qualification of contractors, awarding the contracts, and restricting the participation of unqualified contractors.
The developed framework is based on the dimensional weighting method and a correlation process, thereby enhancing the selection procedure with an evaluation of forty pertinent criteria in addition to the bidding price. This framework can be adapted by any ministry or large private sector company, as it utilizes the pre-qualification criteria that impact the selection of construction contractors.
Forty criteria were determined from this study, rather than the twenty-one obtained from previous studies.
The presented forty criteria are important standards for choosing contractors, as these are the criteria with the highest weights and thus have the greatest impact on a project’s success.
Government ministries and large construction companies can adjust some of the criteria based on a project’s unique requirements, economic and social status.
To improve the selection for construction contractors in Saudi Arabia, the following avenues are suggested:
The forty criteria for choosing a suitable construction contractor for a project could be adjusted based on the project type and its requirements. This will also be appropriate as it will allow clients to provide their requirements to the framework which could then give them a list of the contractors that will best serve their purpose from different angles. This customizing possibility will thus offer the flexibility to evaluate more contractors based on different criteria.
Training courses to improve the capabilities of bid evaluators will prove valuable, improving and standardizing the assessment process.
An online database of registered construction contractors should be assembled and made available on a government website so private-sector owners and ministries can reach out to quality certified professionals of the industry.
It should be noted that the developed framework is limited to the number of experts and respondents who participated in the different development process stages. It is also limited in terms of location for the construction projects in Saudi Arabia. Additionally, the ranking, weighting, of the prequalification criteria was limited to the applied eigenvector technique. The validation of the framework is limited to several investigated contractors. Moreover, it is limited to the applied analysis technique (Multi-Attribute Utility Theory).
Disclosure statement
No potential conflict of interest was reported by the author(s).
References
- Abu Nemeh, M. 2012. Multi-criteria decision making model for the selection of a construction contractor in Saudi Arabia.s[Master Thesis]. King Fadh University of Petroleum andsMinerals; 2012. 1–186.
- Al-Harbi, K. 2001 Application of the AHP in project Management, International Journal of Project Management. 19: 19–27. . : .
- Al-Reshaid, K., and N. Kartam, ”Design–build Pre-Qualification and Tendering Approach for Public Projects,” Department of Civil Engineering, College of Engineering, Kuwait University Construction Program, Major Projects, Administration, Kuwait University, 17 November 2004.
- Aladdin, A., B. H. Samer, B. Barkokebas, B. Ahmed, and H. Mohamed. 2019. “A BIM-based Automation of Design and Drafting for Manufacturing of Wood Panels for Modular Residential Buildings.” International Journal of Construction Management 19 (3): 187–205. doi:10.1080/15623599.2017.1411458.
- AlBogamy, A., D. Scott, and N. Dawood. 2012. “Addressing Construction Delays in the Kingdom of Saudi Arabia.” International Proceedings of Economics Development & Research, 45, pp. 148.
- AlMutairi, S. 2017. “Assessment and Develop the Saudi’s Contractors Classification System.” Ph.D. Dissertation, Arizona State University.
- Alshamrani, O. 2012. “Evaluation of School Buildings Using Sustainability Measures and Life- Cycle Costing Technique.” PhD Dissertation, Concordia University.
- Aparna, S., S. Senaratne, and Y. G. Sandanayake. 2018. “Methodology to Extract Underlying Basic Assumptions of a Public Sector Construction Project Culture: An Exploratory Case Study.” International Journal of Construction Management 18 (6): 467–481. doi:10.1080/15623599.2017.1338130.
- Awal, S. M. 2010. Contractor Selection in Ghana,” Department of Building Technology. February. Ghana: Kwame Nkrumah University Of Science And Technology In Partial Fulfilment.
- Beale and Company. 2016. “The Future of Saudi Arabia: Opportunities and Challenges in Infrastructure Projects and Beyond.” [Online]. August. Accessed 05 September 2018. http://www.beale-law.com
- Bubshait, A., and K. AL-Gobail, and Member, ASCE. 1966. “Contractor Prequalification in Saudi Arabia.” March.
- Chee, H. W., D. H. Gary, and A. C. Patricia. 2010. “Lowest Price or Value? Investigation of UK construction clients’ tender selection process,” United Kingdom: Construction Management and Economics.
- Chen, Z.-S., X. Zhang, W. Pedrycz, X.-J. Wang, and M. J. Skibniewski. 2020. “Bid Evaluation in Civil Construction under Uncertainty: A Two-Stage LSP-ELECTRE III-Based Approach.” Engineering Applications of Artificial Intelligence 94 (September 2020): 103835. doi:10.1016/j.engappai.2020.103835.
- Chen, Z.-S., X. Zhang, R. M. Rodríguez, W. Pedrycz, and L. Martínez. 2021. “Expertise-based Bid Evaluation for construction-contractor Selection with Generalized Comparative Linguistic ELECTRE III.” Automation in Construction 125: 103578. doi:10.1016/j.autcon.2021.103578.
- Cheng, E., and H. Li. 2004. Contractor Selection Using the Analytic Network. Kowloon, Hong Kong: Department of Building and Real Estate, The Hong Kong Polytechnic University, Hunghom. January 09.
- Contractors classification agency. 2017. [Online]. Accessed 11 September 2018. https://contractors.momra.gov.sa/Classification_Fields.aspx
- Daniel, K., B. Nathan, F. Nicholas, and C. Nicholas. 2020. “Productivity Enablers and Inhibiting Health and Wellbeing Practices of South Australian Construction site-based Workers: A Qualitative Study.” International Journal of Construction Management 20 (8): 882–899. doi:10.1080/15623599.2018.1496538.
- Edmundas, K. Z. 2015. Selecting a Contractor by Using a Novel Method forMultiple Attribute Analysis: Weighted Aggregated Sum Product Assessment with Grey Values (WASPAS-G). Romania: Vilnius Gediminas Technical University.
- El-Sawalhi, N., et al. 2007. “.” In BuHu (Built a Human Environmental Research Institute), School of Construction and Property ManagementEl-Sawalhi, N., Eaton, D., & Rustom, R. (2007). Contractor pre-qualification model: State-of-the-art. International Journal of Project Management, 25(5), 465–474. doi:10.1016/J.IJPROMAN.2006.11.011
- Enshassi, A. E. 2014. Contractors’ Selection Criteria: Opinions Of Palestinian Construction Professionals. Gaza City, Gaza Strip - Palestinian: Department of Civil Engineering, IUG, P O Box 223. February.
- Halil, F. 2007. “Contractor’s Perception of the Use of Statistical Approach in the Tender Evaluation at the Public Works Department, Malaysia.” American Journal of Applied Sciences 4 (12): 1084–1089. doi:10.3844/ajassp.2007.1084.1089.
- Hatush, Z., and M. Skitmore. 1998. “Contractor Selection Using Multiciteria Utility Theory: An Additive Model.” Building and Environment 33(2–3), 105–15. .
- Holt, G. D., P. O. Olomolaiye, and F. C. H. 1995. “Applying multi-attribute Analysis to Contractor Selection Decisions.” European Journal of Purchasing and Supply Management 1: 139–148 .
- Ismail, H., ”Project Managmant, ” Planning Engineer, 06 November 2013. [Online]. Available: https://planningengineer.net/delays-construction-projects-ksa-2/. [Accessed 06 November 2013].
- Jeddah Chamber. 2016. “Construction and real-estate Sector, Technical Report.” [Online]. March. Accessed 05 September 2018. https://www.jcci.org.sa/
- Khoso, A. R., A. M. Yusof, Z.-S. Chen, X.-J. Wang, M. J. Skibniewski, and N. A. Memon. 2021. “Embedded Remote Group Environment through Modification in MACBETH – An Application of Contractor’s Selection in Construction.” Journal of Civil Engineering and Management 27 (8): 595–616. doi:10.3846/jcem.2021.15763.
- Liu, X., Z. Zhang, and W. Qi. 2021. “The Strategy Analysis of Grouped Bid Evaluation in Reverse Auction: A Tripartite Evolutionary Game Perspective.” IEEESystemsJournal. doi:10.1109/JSYST.2021.3066370.
- Paul, N. 2015. A Global Forcast for the Construction Industry 2030. 21 Broadwall, London SE1 9PL United Kingdom: Global Construction Perspectives and Oxford Economics Broadwall House.
- Plebankiewicz, E. Construction Contractor Prequalification From Polish Clients’ Perspective. Kraków, Poland:Institute of Building and Transport Management, Cracow University of Technology, Warszawska. Vol. 24. 31–155 .12 June 2009.
- Saleem, M., and A. Hosoda. 2021. “Development and Testing of Glow-in-the-Dark Concrete Based Raised Pavement Marker for Improved Traffic Safety.” Journal of Civil Engineering and Management 27 (5): 278–287. doi:10.3846/jcem.2021.14902.
- Saleem, M., I. B. Nawaf, S. D. O. Alshamrani, and B. Abdulaziz. 2019. “Fundamental Investigation of Solid Waste Generation and Disposal Behavior in Higher Education Institute of Kingdom of Saudi Arabia.” Indoor and Built Environment 28 (7): 927–937. doi:10.1177/1420326X18804853.
- SCA. 2018. “Statistical Report of Contracting Sector, Statistical Report.” [Online]. https://www.muqawil.org/public/publications/22382cf1827f13e0c8d180b742f29aaeec1a8524.pdf
- Sodangi, M., A. F. Salman, and M. Saleem. 2018. “Building Information Modeling: Awareness across the Subcontracting Sector of Saudi Arabian Construction Industry.” Arabian Journal of Science and Engineering 43 (4): 1807–1816. doi:10.1007/s13369-017-2756-z.
- Somboonpisan, J., and L. Charinee. 2021. “Environmental Weight for Bid Evaluation to Promote Sustainability in Highway Construction Projects.” Journal of Construction Engineering and Management 147 (4, April): 202. doi:10.1061/(ASCE)CO.1943-7862.0002005.
- “Specialize in the Issuance of a Certificate Classification of Saudi and Foreign Contractors and Engineering Consulting Offices.” 2015. [ Online]. Accessed 10 September 2018. http://www.tasneef.com.sa/en/services/4/Classification-Certificate/
- Turskis, Z. 2008.“MULTI-ATTRIBUTE CONTRACTORS RANKING METHOD BY APPLYING ORDERING OF FEASIBLE ALTERNATIVES OFsSOLUTIONS IN TERMS OF PREFERABILITY TECHNIQUE.“ In Technological and economic development Baltic Journal on Sustainability 14 (2): 224–239. doi:10.3846/1392-8619.2008.14.224-239.
- Watt, D. J., B. Kayis, and K. Willey. 2008. Identifying Key Factors in the Evaluation of Tenders for Projects and Services. Sydney, NSW 2052, Australia: University of New South Wales, Faculty of Engineering. February 29.
- Zavadskas, E., F. Peldschus, and A. Kaklauskas. 1994. Multiple Criteria Evaluation of Projects in Construction. Technika: Vilnius.
- Zedan, H., and S. Martin. 1997. “Evaluating Contractor Prequalification Data: Selection Criteria and Project Success Factors.” Construction Management and Economics 15 (2): 129–147. doi:10.1080/01446199700000002.
- Zedan, H., and S. Martin. 1998. “Contractor Selection Using Multiciteria Utility Theory: An Additive Model.” Building and Environment 33 (2–3): 105–115.