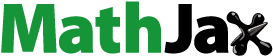
ABSTRACT
In this study, we estimate the effect of workplace automation on the probability of unionization, examining different worker groups in the manufacturing sector in Norway. The results show that automation increases the probability of unionization for the most exposed workers. This group of workers is the most negatively affected in terms of employment and wages, suggesting that the increase in unionization rates is a response to job and wage uncertainty arising from automation.
I. Introduction
In this study, we analyse the effect of a firm’s automation decision on individual-level unionization in the manufacturing sector in Norway and whether this varies between worker groups. We employ a stacked difference-in-differences model where treatment is a spike in the import of automation technologies aaat the firm-level.Footnote1
We analyse four worker groups, split by their tasks in production, to investigate the effects of automation on labour market outcomes and unionization rates. Our study relates to the growing literature on the effects of automation on firm- and individual-level labour market outcomes from automation (see e.g. Gihleb et al. (Citation2022), and Hassel et al., (Citation2022)). Studies that look at the relationship between automation and unionization include Haapanala et al. (Citation2022) who find no significant correlation between occupation task routineness and union membership, and Golin and Rauh (Citation2022) who find that automation risk increases self-reported intention to join a union. Dauth et al. (Citation2021) study how local labour markets adapt to industrial robots in Germany and find that robot exposure is associated with displacement effects, which are concentrated in local labour markets with weaker unions.
The merits of our paper are that we use linked firm-employee register data that identifies automating firms. We study this issue in Norway, which is a well-regulated labour market with high union membership. Although these unions are ‘open shop’, which means that wage agreements cover all employees in firms covered by tariff agreements, union members have access to free legal advice in cases of redundancies and dismissals, implying that membership becomes more attractive if job uncertainty increases.
II. Empirical approach
We follow Bessen et al., (Citation2023) paper and employ a difference-in-differences event study model, limiting treatment and control groups to automating firms. We identify the group of treatment firms by year c, in which they have a spike in their automation investments. Individuals registered as employees in these firms one year before the spike are then followed in the event window from three years before to four years after {−3, … ,4}. The control group consists of workers in firms observed in the same cohort (observation years) but with spikes in automation in years c + 5, or later. The data for each cohort is then stacked to provide balanced panel data in event time.
We estimate variants of
where Yijt is the outcome for individual i, working in firm j at time t and τ is the event time (−3, … , 4). Treat equals 1 for firms that have a spike in their automation imports at τ = 0. It indicates event-time indicators, with τ = −1 as the reference category. The key parameters are βτ, which identify the relative changes in the outcome variable relative to the pre-treatment period τ = −1 for individuals in automating firms versus those in firms that automated later. t denotes calendar year fixed effects, and
cohort by individual fixed effects. Standard errors are clustered at the firm level and at the firm where the treatment (spike) occurred.
We exploit only variation in the timing of treatment, to increase the comparability of treat and control workers, and we limit our sample to those who have worked at least one year prior to an automation spike, to avoid anticipation effects. To further improve the comparability between the treatment and control groups, we use coarsened exact matching (Iacus et al., Citation2008) to match treatment and control on union membership, earnings, firm size, age, and education in the pre-treatment period.Footnote2 Given these features of the empirical design, the βτ-coefficients can be interpreted causally.
III. Data and variables
We exploit matched employer – employee register data for the period 2000–2018. The starting point is all workers between the ages of 20 and 60 in the manufacturing industry in a firm that had an automation spike at time c, c + 5 or later. These individuals are then followed before and after the event, irrespective of whether they continue to work in the same firm. The key dependent variables are individual information on union membership, taken from individual tax registers, employment, and annual labour market earnings.
Our event time analysis is based on the relative time to an automation event, defined as the year when automation costs relative to total operating costs (excluding automation) are three times the average value for a given firm.Footnote3 We are interested in how automation affects the probability of union membership across different types of workers.
We identify automatizing costs based on the Norwegian Trade Statistics Register, which records all cross-border goods purchased by firms. For each year, we have the value of imports disaggregated by products. Trade flows are reported according to the 8-digit Harmonised System (HS) combined nomenclature, see Appendix B for more detailed information on the spike approach on import codes used.
shows the number and share of workers in automating firms by occupation task categories: machine operator, assembler/trade worker, office worker, and engineer/scientist. We have chosen these categorizations based on the potential interactions they might have with new automation technologies. We expect office workers to not work directly with the new technology, while engineers and scientists might have a supporting role in programming and maintaining the new machines. Machine operators work directly with the new machines, and the extent to which they are complementary depends on the relevance of their existing skills and the possibility of receiving additional training to operate the new technology. Of the four occupational categories, assemblers/trade workers are most likely to be substituted by the new automate technologies. These are workers with manual repetitive tasks, often involved directly in production.
Table 1. Occupation of workers in automated firms.
IV. Results
A central mechanism that links automation and union membership is the perceived or actual threat to jobs. Therefore, to motivate the subsequent analyses, we investigate how automation affects the employment shares and annual earnings of workers in our occupation categories.
Results from our firm-level analysis of occupational category shares show a statistically significant and substantial decrease (17%) in the employment share of assemblers in firms following the adoption of automation technologies (Figure C1 and Table C1). However, the shares of workers in the other three occupation categories have remained steady or increased following automation.Footnote4 Turning to annual earnings, the effects are negative across almost all groups (except machine operators); however, we only find a statistically significant and sizable negative effect for assemblers, with an average reduction of 4% (Figure and Table C2).Footnote5 The negative effects on wages and employment shares could decrease perceived job security, strengthening the incentive to unionize. It is also possible that existing union representatives increase their efforts to recruit new members as a response.
We now turn to the main analyses. presents the stacked difference-in-differences estimates from Equationequation (1)(1)
(1) , where the outcome variable is an indicator of whether an individual is a member of a union, from three years before until four years after automation. We find that unionization increases among assemblers, office workers and engineers after automation, but not machine workers. However, this effect is only statistically significant for assemblers, with automation increasing the probability of unionization by about two percentage points when aggregating the pre-and post-periods (). The average union rate in the pre-period is 0.79, which implies a relative increase of approximately 2.4%. This result is in line with our predictions that assemblers are the closest substitutes for automation tasks. The effects for machine operators and engineers are likely to be insignificant because this worker group is a compliment to the new machines, as many tasks within these occupations are necessary for running and maintaining them. Office workers do not work directly in production and, therefore, are not expected to be affected.Footnote6 The results are not significantly affected by including linear region-specific time trends (Table A5).
Figure 1. Automation and probability of being in a union.
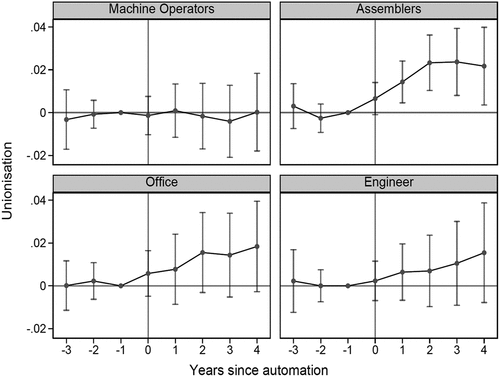
Table 2. Average yearly effect of automation on unionization by occupation category.
V. Conclusion
We apply a stacked difference-in-differences event-study approach to analyse whether increased job insecurity following the introduction of new technology increases the willingness of different categories of workers to unionize. We document negative earnings and employment effects for assemblers, an occupation group that is more exposed to automation at the workplace. Consistent with the negative labour market trajectories, we show that they are significantly more likely to unionize after the automation shock. We find no statistically significant effects for the other categories of workers (machine operators, office workers and engineers).
Our results suggest that workers who are most likely to be affected by automation have an increased chance of guarding against its negative consequences by joining a union. Further research from other countries would also be of interest, to identify the role of institutions for the relationship between automation and unionization.
Supplemental Material
Download MS Word (92.9 KB)Disclosure statement
No potential conflict of interest was reported by the author(s).
Supplementary material
Supplemental data for this article can be accessed online at https://doi.org/10.1080/13504851.2024.2363272.
Additional information
Funding
Notes
1 This does not include automation technologies purchased in Norway or develop inhouse.
2 Strata with only control or treated units are discarded. In our case, 35% of observations are dropped (37% from the control group and 29% from the treatment group). Individuals are weighted by CEM weights. Since we drop treated units, the estimates are local treatment effects.
3 This approach is based on Bessen et al. (Citation2023) approach. Appendix A provides more detail on how this is calculated.
4 Details of the labour market analysis are provided in Appendix C.
5 We use the estimation approach in Equationequation (1)(1)
(1) with log of annual wages as the left-hand side variable.
6 We find similar results for both younger and older workers. 82% of workers in our sample are male and the results is significant only for male workers. See Appendix Tables A3 and A4.
References
- Bessen, J., M. Goos, A. Salomons, and W. van den Berge. 2023. “What Happens to Workers at Firms That Automate?” The Review of Economics and Statistics: 1–45. https://doi.org/10.1162/rest_a_01284.
- Dauth, W., S. Findeisen, J. Suedekum, and N. Woessner. 2021. “The Adjustment of Labor Markets to Robots.” Journal of the European Economic Association 19 (6): 3104–3153. https://doi.org/10.1093/jeea/jvab012.
- Gihleb, R., O. Giuntella, L. Stella, and T. Wang. 2022. “Industrial Robots, workers’ Safety, and Health.” Labour Economics 78:102205. https://doi.org/10.1016/j.labeco.2022.102205.
- Golin, M., and C. Rauh. 2022. “The Impact of Fear of Automation.” Janeway Institute Working Papers 2229, Faculty of Economics, University of Cambridge.
- Haapanala, H., M. Ive, and P. Zachary. 2022. “Robots and Unions: The Moderating Effect of Organised Labour on Technological Unemployment.” IZA Discussion Papers 15080, Institute of Labor Economics.
- Hassel, A., D. Özkiziltan, and K. Weil. 2022. “Labor Market Effects of Automation: A Scoping Review.” Working Paper. https://digitalage.berlin/wp-content/uploads/2022/10/hasseletal_2022_labormarketeffectsofautomation_v2.pdf.
- Iacus, S. M., G. King, and G. Porro. 2008. “Matching for Causal Inference without Balance Checking.” SSRN Electronic Journal. https://doi.org/10.2139/ssrn.1152391.