ABSTRACT
Interrupted education of adolescent mothers remains a major concern, but limited evidence-based programming exists to support postpartum schooling of this group. This study aimed to better understand the factors that render some adolescent mothers vulnerable to school non-enrollment, and how to reduce these risks. Data from 1,046 adolescent and young mothers (10–24 years) from rural and urban communities in South Africa’s Eastern Cape was obtained through a questionnaire containing validated and study-specific measures relating to sociodemographic characteristics, schooling, relationships, violence exposure, and health. Using latent class analysis, we explored emerging latent groups and their relationship to mothers’ enrollment in school, college, or tertiary education. The analyses revealed three distinct groups of mothers: The ‘most disadvantaged’ subgroup (39%) experienced multiple risks, including food insecurity, living in informal housing, lacking positive relationships with their caregiver, and the highest distance to school; The ‘disadvantaged’ subgroup (44%) did not experience food insecurity whilst reporting better caregiver relationships and lower distance to school. However, this group still showed high probabilities of residing in informal housing and in communities that expose them to violence; A ‘least disadvantaged’ subgroup (17%) was largely unaffected by economic and community risks and experienced good caregiver relationships. Compared to the most disadvantaged mothers, the least disadvantaged mothers showed the highest probability of being enrolled in education (65% versus 45%). Adolescent motherhood can impede continued enrollment in education and individual-centered interventions alone might not be sufficient to mitigate the risks for non-enrollment. Services and provisions that address severe poverty and improve family relationships might provide an opportunity to positively influence schooling among adolescent mothers.
Introduction
Globally, each year 18 million children are born to adolescent mothers, most of which reside in sub-Saharan Africa (Blum & Gates, Citation2015; United Nations, Citation2020). Adolescent childbearing is associated with multiple adverse health and socioeconomic disadvantages for women and their children (Amongin et al., Citation2021; Branson et al., Citation2015; Fall et al., Citation2015; Robert et al., Citation2022), and accounts for the majority of maternal deaths of women aged 15–49 years in low- and middle-income countries (Neal et al., Citation2016). Adolescent pregnancy has also been identified as both a cause and consequence of early school dropout; even adolescent mothers with promising educational trajectories face substantial barriers to completing their education once the child is born (Dupéré et al., Citation2015; Groves et al., Citation2021; Morrell et al., Citation2012; Stoner et al., Citation2019). Given that education is a social determinant of health (Freudenberg & Ruglis, Citation2007), it is critical to find ways of promoting the continued school enrollment of adolescent mothers as a way to strengthen health and social outcomes for them and their children (Maslowsky et al., Citation2021).
In South Africa, numerous studies have shown that early motherhood leads to high rates of school dropout and grade delay in the immediate years after birth (e.g., Grant & Hallman, Citation2008; Madhavan & Thomas, Citation2005; Marteleto et al., Citation2008; Stoner et al., Citation2019; Timæus & Moultrie, Citation2015). Fewer studies have investigated young mothers’ postpartum return to school (Grant & Hallman, Citation2008; Jochim et al., Citation2020), but highlight that only between 30% and 65% of mothers manage to resume their education, and even fewer might manage to graduate (Ardington et al., Citation2015; Kakal, Citation2015). The strong variability of school enrolment within the group of South African adolescent mothers, some of whom continue to catch up in education until the early 20s, warrants explorations into the variables associated with their enrolment status. Oftentimes, young mothers’ negative outcomes, including incomplete education, are perceived as a continuation of individual-level problem behaviors, such as delinquency, and a longer history of poor school performance (e.g., Grant & Hallman, Citation2008; Oxford et al., Citation2006, Citation2005). However, as a heterogeneous group, adolescent mothers will not only differ on individual-level behaviors, and an assessment of the role of economic, family, and community factors for adolescent mothers’ schooling remains essential, given their pivotal role for both adolescent motherhood and school dropout (Blum et al., Citation2014; Blum & Gates, Citation2015; Chung et al., Citation2018; Gunawardena et al., Citation2019; Imamura et al., Citation2007; Kassa et al., Citation2018; Mmari & Blum, Citation2009; Pradhan et al., Citation2015; Rumberger & Lim, Citation2008).
To date, very few studies have examined the associations between enrollment in education and non-individual level factors among adolescent mothers. This is despite ample evidence from low- and middle-income countries, including South Africa, indicating that school dropout and grade failure are associated with economic deprivation (Boyes et al., Citation2017; Fleisch et al., Citation2012; Flisher et al., Citation2010; Orkin et al., Citation2014; Watkins et al., Citation2014), violence exposure (Herrero Romero et al., Citation2021), and other school-access variables, such as the distance from school (Singh & Mukherjee, Citation2018). In addition, family-level variables, such as supportive parenting, has shown to buffer educational risks among children aged 7 to 13 (Sherr et al., Citation2016) and might protect adolescents against school delay in violence-affected environments (Herrero Romero et al., Citation2018). Even though evaluated interventions for adolescent mothers’ are scarce, a promising pilot intervention – using group sessions with mentor mothers – showed improved rates of enrollment and attendance (Shazi et al., Citation2018). However, retaining adolescent mothers in the study was reported to be difficult due to practical childcare and transport challenges, suggesting that, without an understanding of how combinations of economic, social, and structural variables affect adolescent mothers, we might partly stifle the development of policies with a programmatic focus beyond individual-level behaviors to support them.
Conceptual framework and research questions
The study is situated at the nexus of stress process models, which would consider adolescent motherhood as an event that can cause coping and adjustment problems, and resilience frameworks that explain how individuals react to the stressor (Dupéré et al., Citation2015). In terms of school enrollment and education, resilience is often understood to reflect individual resources that might enable driven and motivated mothers to remain in school (Masten, Citation2011). However, recent work of adolescent mothers in South Africa showed the importance of social, institutional, and structural resources for adolescent mothers’ schooling, potentially via affecting resilience levels (Groves et al., Citation2021; Van Breda & Theron, Citation2018). The present study aimed to explore how family- and household-, school-, and community-level factors affect enrollment in education among 1,046 adolescent mothers living in rural and urban South Africa by answering the following research questions: (1) Which clusters of variables can be identified among adolescent mothers? And (2) Is subgroup membership related to adolescent mothers’ enrollment in school, college, or tertiary education?
Methods and materials
Participants, procedures, and ethics
Data for this study was collected as part of the HEY BABY (Helping Empower Youth Brought up in Adversity with their Babies and Young Children) cohort study of adolescent and young mothers and their children residing in the Eastern Cape province of South Africa (N = 1,046). Adolescent mothers aged 10 to 24 were recruited from urban and rural locations of two health districts in the Eastern Cape, South Africa between 2017 and 2019. All participants had become mothers for the first time before the age of 20. Our inclusion criteria therefore align with the World Health Organization definition of ‘adolescence’ (World Health Organization, Citation1998) and defines adolescent motherhood as the occurrence of a birth among girls and young women at the age of 19 or below. The period of time since the mothers gave birth varied among participants; mothers were able to complete the interviews from 3 months postpartum, but several years had passed since birth for other participants (The mean age of participants’ oldest children was 1.72 years [SD 1.51]). Informed consent was sought from adolescents who were above the age of 18 and assent was provided by underage participants in addition to consent from their adult caregiver. All consent forms and questionnaires were available in English and isiXhosa.
Participants were recruited in a six-pronged parallel strategy, developed with local experts and an advisory group of adolescent mothers, which included the following channels: All known health facilities within the district (n = 73), secondary schools (randomly selected; n = 43), maternity obstetric units (n = 9), referrals by service providers and social workers, neighbouring adolescents of participants (to reduce stigma) and, referrals by adolescent mothers themselves. Piloting of data collection tools was undertaken with adolescent mothers (n = 9) and adolescents living with HIV (n = 25).
Assisted by trained interviewers, adolescents completed two complementary questionnaires, each taking about 60 minutes. Ethical clearance was provided by the Universities of Oxford (R48876/RE001; R48876/RE002) and Cape Town (HREC REF: 226/2017) and by the provincial government departments of Health, Basic Education, and Social Development.
The final analyses were based on 1,029 participants. Sixteen participants were excluded because they were older than 19 when they became mothers for the first time, and one participant was excluded due to missing data on key variables. After these exclusions, missing data were less than 5% on all variables and thus no imputation was conducted.
Measuring tools
Educational enrollment assessed if the participant was enrolled in primary school, secondary school, university, college, or further education and training (0: not enrolled; 1: enrolled) at the time of participating in the study, which was at least 3 months postpartum.
Social- family- and household-level factors
Food insecurity was determined by one item from the National Food Consumption Survey (Labadarios et al., Citation2005) to assess insufficient food for more than 2 days in the past week. Informal housing was assessed by one item from the South African census (Statistics South Africa, Citation2001). Co-residency with a caregiver was assessed by one self-report item. Three measures were used to assess the relationship between the adolescent and their parent or caregiver: Two subscales from the Alabama Parenting Questionnaire (Elgar et al., Citation2007) were used to measure positive parenting/caregiving (range 0–24) and parental/caregiver monitoring (range 0–40). The quality of communication between the adolescent and their caregiver was assessed using five items from the Child-Parent Communication Apprehension Scale (Lucchetti et al., Citation2002) which assesses how comfortable the adolescent is to speak to their caregiver about their problems or worries (range 0–20). Physical and emotional abuse at home was assessed using 12 items from the UNICEF Measures for national-level monitoring of orphans and other vulnerable children (Snider & Dawes, Citation2006). Past-year exposure to physical abuse by a caregiver was assessed by indicating having experienced any of the following: Being hit with an item or physically hurt through slapping, punching, hitting, or pulling hair. Past-year exposure to emotional abuse by a caregiver was assessed by having experienced any insults, threats, being kicked out of the house, or withholding a meal as punishment. The continued relationship with the child’s father was assessed by one item asking the mother if the father is still in a relationship with father of her child.
School-level factors
Distance from school was assessed with one item that was recoded to reflect if participants travelled longer than the sample mean to get to school (0: ≤ 30 min; 1: >30 min.).
Community-level factors
Community violence exposure in the previous year was assessed with the Child Exposure to Community Violence Checklist (Martinez & Richters, Citation1993), adapted to the four most common community traumas for children in South Africa, coded as victimization by any of the following: Assault, robbery, and witnessing of stabbings and/or shootings. Daycare/Crèche use was captured by asking participants if their oldest child attends childcare services.
Covariates
Were maternal age at the time of the study and the adolescent mothers’ age at birth, child age, multiparity, being the primary caregiver of the child, rural residency, having repeated a grade preceding the pregnancy. The analyses also controlled for maternal HIV status which was ascertained through self-report and verified through clinical records.
Statistical analysis
All analyses were completed in three steps using Stata 15.1 and Mplus 7.0. In step 1, frequencies for all outcomes, and covariates were examined for the whole sample and disaggregated by enrollment status. In step 2, Latent Class Analysis (LCA) was used to explore distinct patterns of exposure to social-, family- and household-, school-, and community-level variables in the sample. To compare enrollment in education across profiles, a one-step ‘distal-as-indicator’ model was applied (Lanza et al., Citation2013). We followed the recommended approach of simultaneously modelling the latent class variable and the associations between the latent profiles and school enrollment (Lanza et al., Citation2013; see, ). With a view to identifying factors associated with individual profile membership, probabilistic predictors (maternal age and age at birth, child age, multiparity, rural residency, HIV status, having repeated a grade preceding the pregnancy, and being the primary caregiver) included in the model via the post-hoc AUXILIARY option in MPlus. We used full information maximum likelihood to account for missing data.
Figure 1. Final one-step ‘distal as indicator’ model. Rectangles represent observed variables and ovals represent latent variables. The eleven indicators are presented at the top, covariates on the left-hand side, and the outcome of enrolment in school, college, or tertiary education on the right.
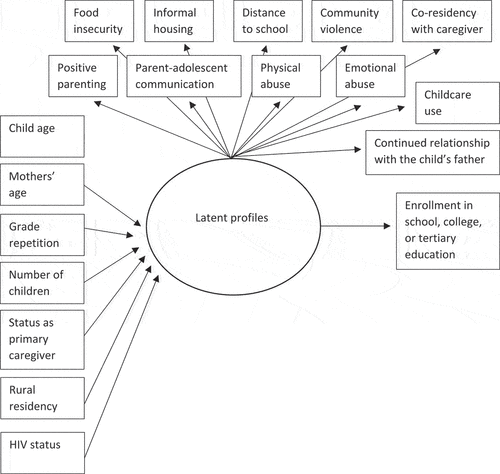
The number of latent classes was determined iteratively. After conducting a two-profile LCA, successive LCAs estimating three to five profiles were carried out in order to fully test for multiple homogenous groups (Nylund et al., Citation2007). The decision on the final number of classes was based on the interpretability of the results, meaningfulness of classes, and five indices to estimate relative model fit. First, the Akaike Information Criterion (AIC) and Bayesian Information Criterion (BIC) were examined, where lower values indicate a better model, although sometimes minimum values are not reached (Masyn, Citation2013; Nylund et al., Citation2007). Second, the adjusted Lo-Mendell-Rubin Likelihood Ratio Test (ALMR- LR) of goodness of fit which points at the ‘best’ model with the smallest number of classes that is not significantly improved by the addition of another class (Masyn, Citation2013). Third, the bootstrap likelihood test that uses the bootstrapped samples. Lastly, classification quality was assessed using relative entropy, with higher values being superior (Masyn, Citation2013).
Results
displays the characteristics of the sample. The mean age of participants was 18 years (SD = 1.8) and they became mothers, on average, just over the age of 16 years (SD = 1.5). The sample showed relatively high levels of socioeconomic disadvantage and large variability in terms of enrollment in education. About a quarter of the participants indicated not having enough food in the house in the previous week (22.9%), 21.8% lived in informal housing, about a third of participants lived with HIV (28.6%), and over 20% reporting having experienced any community violence in the previous year.
Table 1. Summary of sample characteristics disaggregated by school enrolment.
About 54% of adolescent mothers were enrolled in secondary school, college, or tertiary education at the time of the interview and 54.4% had already been grade delayed preceding the motherhood. The vast majority of participants (91.3%) continued to reside with their caregiver. The mean score for participants’ experiences of positive parenting by their parent or caregiver was 17.22 (SD 5.28), exposure to caregiver monitoring was 32.13 (SD 8.90), and reported caregiver-adolescent communication was 12.71 (SD 3.76). Under half of participant reported a continued relationship with the father of their oldest child (43.7%) and about a quarter of participants reported access to childcare (24.2%).
LCA model
The results of the LCAs with 2–5 latent classes are displayed in . The LCA showed that there were three heterogeneous profiles of adolescents – differentiated according to their exposure to social-, family- and household-, school-, and community-level factors. In terms of information and classification criteria, models with greater number of profiles showed smaller values for AIC and BIC, and higher values of entropy. Upon inspection of the interpretability of the models, the solution with three latent classes was meaningful and offers the greatest parsimony.
Table 2. Statistical criteria and profile sizes for LCA estimating 2–5 latent profiles (n = 1,029).
shows the difference in estimated probabilities and means of all variables across the three profiles. Adolescents in latent class I can be considered to belong to the ‘Most disadvantaged’ group of adolescent mothers (n = 402, 39%), of whom 49% experienced food insecurity and 31% lived in informal housing. Participants in this group showed high probabilities to continued living with their caregiver (89%), but experienced the lowest mean scores of positive parenting (M = 12.34) and parent-adolescent communication (M = 10.33). The probabilities for physical abuse and emotional abuse experiences in the home were 4% and 8%, respectively, and about 14% had experienced community violence in the past year. This group showed the highest proportion of mothers travelling longer than 30 minutes to get to school (33%). 41% indicated a continued relationship with the father of the child and 24% indicated using formal childcare services. The probability to be enrolled in school, college, or tertiary education was lowest in this group, indicating 45%.
Table 3. Probabilities and mean scores for social-, family and household-, school-, and community-level factors, respectively, by profile membership.
Adolescents in latent class II can be considered belonging to the ‘Disadvantaged’ group of mothers (n = 457, 44%), of which only 8% experienced food insecurity, but 23% resided in informal housing. Participants in this group also showed high probabilities to continued living with their caregiver (90%) and received the highest mean scores of positive parenting (M = 21.62). Compared to the ‘most disadvantaged’ group, this group also indicated slightly higher scores for parent-adolescent communication (M = 12.45), but slightly lower scores for caregiver monitoring (M = 30.41). The probabilities for physical abuse and emotional abuse were 3% and 11%, respectively, and reported the highest proportion of exposure to community violence in the previous year (38%). There were 20% of mothers who travelled longer than 30 minutes to get to school. Compared to other groups, participants showed similar levels of a continued relationship with the child’s father (46%), but higher use for daycare services (32%). Compared to the ‘most disadvantage’ group, the probability to be enrolled in school, college, or tertiary education was 11 percentage points higher.
Adolescents in latent class III can be considered belonging to the ‘Least disadvantaged’ group of adolescent mothers (n = 170, 17%), of which 5% experienced food insecurity and 2% resided in informal housing. Participants in this group showed higher probabilities to continued living with their caregiver than the other two groups (98%), and reported the highest mean scores for parent-adolescent communication (M = 18.47) and caregiver monitoring (M = 37.07), whilst showing a mean score of 16.46 for receiving positive parenting. The probabilities for physical abuse and for emotional abuse, respectively, was 0% for this group, as was their exposure to community violence. Compared to the other groups, participants showed similar levels of a continued relationship with the child’s father (43%), but lower use for daycare services (17%). There were 30% of mothers who travelled longer than 30 minutes to get to school. The probability to be enrolled in school, college, or tertiary education was highest in this group (65%).
Discussion
Adolescent mothers experience multiple obstacles to their pursuit of education. Despite the overall high level of economic and social disadvantage in the sample, our results support that adolescent mothers are a diverse group (Hamburg, Citation1986). Applying a person-centered approach, we identified three subgroups of mothers based on their different exposure to economic- and social- and community-variables. Adolescent mothers affected by severe levels of poverty who also have a poorer relationship with their parents or caregivers – both independently associated with early motherhood – and live further away from school show lower educational enrollment (45%) than mothers living in better familial and socio-economic circumstances (65%). Therefore, the present study provides novel evidence on modifiable economic and parenting variables that could be addressed through services and interventions aiming to reduce risk pathways to educational non-enrollment for adolescent mothers.
Latent classes and school enrollment
The overall probability of being in education was about 50% for over four-fifths of mothers in the present sample (i.e., Class I and Class II combined). These enrolment rates are somewhat higher than expected, given that school enrollment in South Africa sharply declines after the compulsory years (grade 9, age 15; e.g., Timæus et al., Citation2013) and the average age at pregnancy of our sample exceeded 15 years. Since about 40%-50% of all children successfully complete secondary school (Spaull, Citation2019; Van Broekhuizen & Spaull, Citation2017), we anticipated that about half of participants had discontinued their education prematurely, even in the absence of an early pregnancy. We also expected significant rates of pre-pregnancy school withdrawal (Stoner et al., Citation2019), which was the case for about 5% of participants, and high rates of grade delay that leads to a greater risk to discontinue school over time (Gubbels et al., Citation2019). About 10% of non-enrolled participants had completed their secondary education, either before or after the pregnancy, but the vast majority (93%) did not participate in any further education. This highlights that some mothers who are not currently enrolled in further education, were able to reach the important milestone of obtaining their matriculation.
Our results showed that certain key predictors of adolescent motherhood – poverty and poor relationships with the caregiver – may continue to affect adolescent mothers’ lives once their child is born and could contribute towards some of the outcomes, including education, observed in this group. We observed a staggered relationship between the identified subgroups of adolescent mothers and school enrollment: First, the more adolescent mothers were affected by poverty, the less likely they were to be enrolled in school, college, or tertiary education. Even though participants were likely affected by multiple disadvantages associated with heightened levels of poverty, such as poor quality of education, our results indicate differences between extreme forms of poverty that affect the household (i.e., hunger) and community-level poverty, showing an 11% difference in enrolment between the ‘most disadvantaged’ and the ‘disadvantaged’ group. Further, many participants had entered late adolescents or young adulthood, or might be perceived as adults due to their parent status (Swartz et al., Citation2018). However, receiving good parenting might remain important for these maturing adolescents, even though practices should evolve towards joint decision-making and monitoring of activities without exerting control over them (United Nations Children’s Fund, Citation2021). In our study, adolescent mothers with the highest levels of parental monitoring, good adolescent-caregiver communication, and no experience of abuse in the home showed the highest probability of being enrolled in school. Further research should investigate the direction of our findings and examine to what extent they reflect a consistent use of key core parenting components that strengthened parental relationship, and facilitated good schooling, over time (Branje, Citation2018). Our findings also partly align with research showing that structural variables, such as the distance to school, can affect enrolment (De Kadt et al., Citation2014; Filmer et al., Citation2007). However, we identified the same proportion of learners travelling high distances across different levels of disadvantages and school enrolment, suggesting that greater distances might interfere with schooling without the ability to cover transport costs (Filmer, Citation2007; Statistica, Citation2022). Unexpectedly, daycare use was highest in the two disadvantaged groups. It is possible that poorer communities show a higher density of unregulated childcare providers (Hughes et al., Citation2021) which are cheaper, but offer poor quality services (e.g., Madiba et al., Citation2019), but future research should explore these differences, and the potential role of familial childcare support further.
The strength of this is study is the large sample of adolescent mothers who, due to the deployed recruitment channels, were both engaged and not engaged in services. This is also the first set of analyses on adolescent mothers in South Africa which moves beyond single associations with school enrollment or participation in education and, therefore, allowed to explore patterns of disadvantage. This study also has several limitations. First, our measures of poverty can only be interpreted as an indicator of greatest disadvantage in an already disadvantaged setting, due to the recruitment from largely low socioeconomic communities. Exposure to economic disadvantages is likely compounded with other risks (including low parental education and attending low-resourced schools) that are associated with school dropout which may have further contributed to an accumulated educational risk over time. Whilst we attempted to account for the impact of these variables by controlling for impaired school progression preceding the pregnancy, it would have been useful to assess other school-level variables that could affect enrollment, such as violence exposure from fellow students or teachers. Second, we only examined three dimensions of adolescent mother-parent/ caregiver relationship, but did not assess other caregiver behaviors which also influence early pregnancy and schooling. For instance, parents’ intention to instill confidence, build self-esteem, or promote self-efficacy may be relevant caregiver-factors for further study. Third, we re-coded variables measuring violence exposure into binary variables to capture any violence experiences, even though this compromised our ability to examine the variability provided by the original scales. Fourth, the cross-sectional nature of this study precludes an understanding of causality between subgroup membership and educational enrollment which should be examined through future longitudinal research. Fifth, the generalizability of our results and the emerged latent classes will need to be tested by future studies. In addition, future studies will benefit from investigating the variables in our analyses in addition to other country- and context-specific variables which affect adolescent pregnancies, motherhood and schooling in sub-Saharan Africa, such as child marriage.
Conclusion
This study presents a meaningful shift away from interrogating adolescent and young mothers’ problem behaviors as predictors of poor educational outcomes. We showed that negative school outcomes among adolescent mothers, which are often attributed to personality ‘flaws’, such as lack of interest and low success in school, are also linked to economic and social variables that may render some mothers more vulnerable to disrupted schooling than others. Our findings suggest that leveraging services and interventions that alleviate severe poverty and strengthen family-relationships (either separately or combined) likely reduce the risk for school non-enrollment, but future research is needed to examine what type of evidence-based programs are most likely to be effective in the South African context. Future research will be able to build on these findings to further disentangle co-occurring risk and protective factors for enrolment in education within adolescent mothers. This may improve our knowledge of which mothers most require targeted educational support and elucidate how dropout prevention interventions, or combination interventions, can be best tailored to support school enrolment and further education for the most vulnerable subgroups of adolescent mothers.
Data Sharing and Accessibility
Prospective users, policymakers/government agencies/researchers (internal/external) will be required to contact the study team to discuss and plan the use of data. Research data will be available on request subject to participant consent and having completed all necessary documentation. All data requests should be sent to the Principal Investigator.
Acknowledgments
The authors are grateful to the young mothers and their families, the data collection team, and partner organizations who supported the research process. We are also grateful to Anna Carlqvist, William Rudgard, and Lakshmi Neelakantan, for engaging with the conceptual thinking surrounding this research and valuable discussions and comments on the manuscript.
Disclosure statement
No potential conflict of interest was reported by the author(s).
Additional information
Funding
References
- Amongin, D., Kågesten, A., Tunçalp, Ö., Nakimuli, A., Nakafeero, M., Atuyambe, L., Hanson, C., & Benova, L. (2021). Later life outcomes of women by adolescent birth history: Analysis of the 2016 Uganda demographic and health survey. BMJ Open, 11(2), e041545. http://dx.doi.org/10.1136/bmjopen-2020-041545
- Ardington, C., Menendez, A., & Mutevedzi, T. (2015). Early childbearing, human capital attainment, and mortality risk: Evidence from a longitudinal demographic surveillance area in rural KwaZulu-Natal, South Africa. Economic Development and Cultural Change, 63(2), 281–317. https://doi.org/10.1086/678983
- Blum, R. W., Astone, N. M., Decker, M. R., & Mouli, V. C. (2014). A conceptual framework for early adolescence: A platform for research. International Journal of Adolescent Medicine and Health, 26(3), 321–331. https://doi.org/10.1515/ijamh-2013-0327
- Blum, R. W., & Gates, W. H. (2015). Girlhood, not motherhood: Preventing adolescent pregnancy. New York: United Nations Population Fund (UNFPA). https://www.unfpa.org/sites/default/files/pub-pdf/Girlhood_not_motherhood_final_web.pdf
- Boyes, M. E., Berg, V., & Cluver, L. D. (2017). Poverty moderates the association between gender and school dropout in South African adolescents. Vulnerable Children and Youth Studies, 12(3), 195–206. https://doi.org/10.1080/17450128.2017.1308613
- Branje, S. (2018). Development of parent–adolescent relationships: Conflict interactions as a mechanism of change. Child Development Perspectives, 12(3), 171–176. https://doi.org/10.1111/cdep.12278
- Branson, N., Ardington, C., & Leibbrandt, M. (2015). Health outcomes for children born to teen mothers in Cape Town, South Africa. Economic Development and Cultural Change, 63(3), 589–616. https://doi.org/10.1086/679737
- Chung, H. W., Kim, E. M., & Lee, J. E. (2018). Comprehensive understanding of risk and protective factors related to adolescent pregnancy in low-and middle-income countries: A systematic review. Journal of Adolescence, 69(1), 180–188. https://doi.org/10.1016/j.adolescence.2018.10.007
- De Kadt, J., Norris, S. A., Fleisch, B., Richter, L., & Alvanides, S. (2014). Children’s daily travel to school in Johannesburg-Soweto, South Africa: Geography and school choice in the birth to twenty cohort study. Children’s Geographies, 12(2), 170–188. https://doi.org/10.1080/14733285.2013.812304
- Dupéré, V., Leventhal, T., Dion, E., Crosnoe, R., Archambault, I., & Janosz, M. (2015). Stressors and turning points in high school and dropout: A stress process, life course framework. Review of Educational Research, 85(4), 591–629. https://doi.org/10.3102/0034654314559845
- Elgar, F. J., Waschbusch, D. A., Dadds, M. R., & Sigvaldason, N. (2007). Development and validation of a short form of the Alabama parenting questionnaire. Journal of Child and Family Studies, 16(2), 243–259. https://doi.org/10.1007/s10826-006-9082-5
- Fall, C. H., Sachdev, H. S., Osmond, C., Restrepo-Mendez, M. C., Victora, C., Martorell, R., Stein, A. D., Sinha, S., Tandon, N., Adair, L., Bas, I., Norris, S., & Richter, L. M. (2015). Association between maternal age at childbirth and child and adult outcomes in the offspring: A prospective study in five low-income and middle- income countries (COHORTS collaboration). The Lancet Global Health, 3(7), e366–e377 . https://doi.org/10.1016/S2214-109X(15)00038-8
- Filmer, D. (2007). If you build it, will they come? School availability and school enrolment in 21 poor countries. The Journal of Development Studies, 43(5), 901–928. https://doi.org/10.1080/00220380701384588
- Fleisch, B., Shindler, J., & Perry, H. (2012). Who is out of school? Evidence from the statistics South Africa community survey. International Journal of Educational Development, 32(4), 529–536. https://doi.org/10.1016/j.ijedudev.2010.05.002
- Flisher, A. J., Townsend, L., Chikobvu, P., Lombard, C. F., & King, G. (2010). Substance use and psychosocial predictors of high school dropout in Cape Town, South Africa. Journal of Research on Adolescence, 20(1), 237–255. https://doi.org/10.1111/j.1532-7795.2009.00634.x
- Freudenberg, N., & Ruglis, J. (2007). Reframing school dropout as a public health issue. Preventing Chronic Disease, 4(4), A107. http://www.cdc.gov/pcd/issues/2007/oct/07_0063.htm
- Grant, M. J., & Hallman, K. K. (2008). Pregnancy‐related school dropout and prior school performance in KwaZulu‐Natal, South Africa. Studies in Family Planning, 39(4), 369–382. https://doi.org/10.1111/j.1728-4465.2008.00181.x
- Groves, A. K., Gebrekristos, L. T., McNaughton Reyes, L., Moodley, D., Raziano, V., & Maman, S. (2021). A mixed-methods study of resilience and return to school among adolescent mothers in South Africa. Global Public Health, 9, 1–14. https://doi.org/10.1080/17441692.2021.1970208
- Gubbels, J., van der Put, C. E., & Assink, M. (2019). Risk factors for school absenteeism and dropout: A meta-analytic review. Journal of Youth and Adolescence, 48(9), 1637–1667. https://doi.org/10.1007/s10964-019-01072-5
- Gunawardena, N., Fantaye, A. W., & Yaya, S. (2019). Predictors of pregnancy among young people in sub-Saharan Africa: A systematic review and narrative synthesis. BMJ Global Health, 4(3), e001499. https://doi.org/10.1136/bmjgh-2019-001499
- Hamburg, B. A. (1986). Subsets of adolescent mothers: Developmental, biomedical, and psychosocial issues. In J. B. Lancaster & J. B. Lancaster (Eds.), School-age pregnancy and parenthood (1st ed., pp. 115–147). Transaction Publishers.
- Herrero Romero, R. H., Hall, J., Cluver, L., & Meinck, F. (2018). Can supportive parenting protect against school delay amongst violence-exposed adolescents in South Africa? Child Abuse & Neglect, 78(1), 31–45. https://doi.org/10.1016/j.chiabu.2017.09.025
- Herrero Romero, R., Hall, J., Cluver, L., Meinck, F., & Hinde, E. (2021). How does exposure to violence affect school delay and academic motivation for adolescents living in socioeconomically disadvantaged communities in South Africa? Journal of Interpersonal Violence, 36(7–8), NP3661–NP3694. https://doi.org/10.1177/0886260518779597
- Hughes, R. C., Kitsao-Wekulo, P., Muendo, R., Bhopal, S. S., Kimani-Murage, E., Hill, Z., & Kirkwood, B. R. (2021). Who actually cares for children in slums? Why we need to think, and do, more about paid childcare in urbanizing sub-Saharan Africa. Philosophical Transactions of the Royal Society B, 376(1827), 20200430. https://doi.org/10.1098/rstb.2020.0430
- Imamura, M., Tucker, J., Hannaford, P., Da Silva, M. O., Astin, M., Wyness, L., Bloemenkamp, K. W. M., Jahn, A., Karro, H., Olsen, J., & Temmerman, M. (2007). Factors associated with teenage pregnancy in the European Union countries: A systematic review. European Journal of Public Health, 17(6), 630–636. https://doi.org/10.1093/eurpub/ckm014
- Jochim, J., Groves, A. K., & Cluver, L. D. (2020). When do adolescent mothers return to school? Timing across rural and urban South Africa. South African Medical Journal, 110(9), 850–854. https://doi.org/10.7196/SAMJ.2020.v110i9.14664
- Kakal, T. A. (2015). A tale of two sisters: Investigating the socio-economic outcomes of teen childbearing in South Africa (ISS Working Paper Series/General Series, 604). National Bureau of Economic Research. https://ideas.repec.org/p/ems/euriss/77611.html
- Kassa, G. M., Arowojolu, A. O., Odukogbe, A. A., & Yalew, A. W. (2018). Prevalence and determinants of adolescent pregnancy in Africa: A systematic review and meta-analysis. Reproductive Health, 15(1), 1–17. https://doi.org/10.1186/s12978-018-0640-2
- Labadarios, D., Steyn, N. P., Maunder, E., Macintryre, U., Gericke, G., Swart, R., Huskisson, J. H., Dannhauser, A., Vorster, H. H., Nesmvuni, A. E., & Nel, J. H. (2005). The national food consumption survey (NFCS): South Africa, 1999. Public Health Nutrition, 8(5), 533–543. https://doi.org/10.1079/phn2005816
- Lanza, S. T., Tan, X., & Bray, B. C. (2013). Latent class analysis with distal outcomes: A flexible model-based approach. Structural Equation Modeling: A Multidisciplinary Journal, 20(1), 1–26. https://doi.org/10.1080/10705511.2013.742377
- Lucchetti, A. E., Powers, W. G., & Love, D. E. (2002). The empirical development of the child parent communication apprehension scale for use with young adults. The Journal of Family Communication, 2(3), 109–131. https://doi.org/10.1207/S15327698JFC0203_1
- Madhavan, S., & Thomas, K. J. (2005). Childbearing and schooling: New evidence from South Africa. Comparative Education Review, 49(4), 452–467. https://doi.org/10.1086/432770
- Madiba, S., Chelule, P. K., & Mokgatle, M. M. (2019). Attending informal preschools and daycare centers is a risk factor for underweight, stunting and wasting in children under the age of five years in underprivileged communities in South Africa. International Journal of Environmental Research and Public Health, 16(14), 2589. https://doi.org/10.3390/ijerph16142589
- Marteleto, L., Lam, D., & Ranchhod, V. (2008). Sexual behavior, pregnancy, and schooling among young people in urban South Africa. Studies in Family Planning, 39(4), 351–368. https://doi.org/10.1111/j.1728-4465.2008.00180.x
- Martinez, P., & Richters, J. E. (1993). The NIMH community violence project: II. Children’s distress symptoms associated with violence exposure. Psychiatry, 56(1), 22–35. https://doi.org/10.1080/00332747.1993.11024618
- Maslowsky, J., Hendrick, C. E., & Stritzel, H. (2021). Mechanisms linking teenage mothers’ educational attainment with self-reported health at age 50. BMC Women’s Health, 21(1), 1–9. https://doi.org/10.1186/s12905-020-01150-y
- Masten, A. S. (2011). Resilience in children threatened by extreme adversity: Frameworks for research, practice, and translational synergy. Development and Psychopathology, 23(2), 493–506. https://doi.org/10.1017/S0954579411000198
- Masyn, K. E. (2013). Latent class analysis and finite mixture modeling. In T. D. Little (Ed.), The Oxford Handbook of Quantitative Methods (2nd ed, 551-611). Oxford University Press. https://doi.org/10.1093/oxfordhb/9780199934898.013.0025
- Mmari, K., & Blum, R. W. (2009). Risk and protective factors that affect adolescent reproductive health in developing countries: A structured literature review. Global Public Health, 4(4), 350–366. https://doi.org/10.1080/17441690701664418
- Morrell, R., Bhana, D., & Shefer, T. (2012). Books and babies: Pregnancy and young parents in schools. HSRC Press.
- Neal, S., Mahendra, S., Bose, K., Camacho, A. V., Mathai, M., Nove, A., Santana, F., & Matthews, Z. (2016). The causes of maternal mortality in adolescents in low and middle income countries: A systematic review of the literature. BMC Pregnancy and Childbirth, 16(1), 1–18. https://doi.org/10.1186/s12884-016-1120-8
- Nylund, K. L., Asparouhov, T., & Muthén, B. O. (2007). Deciding on the number of classes in latent class analysis and growth mixture modeling: A Monte Carlo simulation study. Structural Equation Modeling: A Multidisciplinary Journal, 14(4), 535–569. https://doi.org/10.1080/10705510701575396
- Orkin, M., Boyes, M. E., Cluver, L. D., & Zhang, Y. (2014). Pathways to poor educational outcomes for HIV/AIDS-affected youth in South Africa. AIDS Care, 26(3), 343–350. https://doi.org/10.1080/09540121.2013.824533
- Oxford, M. L., Gilchrist, L. D., Lohr, M. J., Gillmore, M. R., Morrison, D. M., & Spieker, S. J. (2005). Life course heterogeneity in the transition from adolescence to adulthood among adolescent mothers. Journal of Research on Adolescence, 15(4), 479–504. https://doi.org/10.1111/j.1532-7795.2005.00108.x
- Oxford, M. L., Gilchrist, L. D., Gillmore, M. R., & Lohr, M. J. (2006). Predicting variation in the life course of adolescent mothers as they enter adulthood. Journal of Adolescent Health, 39(1), 20–26. https://doi.org/10.1016/j.jadohealth.2005.08.001
- Pradhan, R., Wynter, K., & Fisher, J. (2015). Factors associated with pregnancy among adolescents in low-income and lower middle-income countries: A systematic review. Journal of Epidemiology and Community Health, 69(9), 918–924. https://doi.org/10.1136/jech-2014-205128
- Roberts, K. J., Smith, C., Cluver, L., Toska, E., Zhou, S., Boyes, M., & Sherr, L. (2022). Adolescent motherhood and HIV in South Africa: Examining prevalence of common mental disorder. AIDS and Behavior. https://doi.org/10.1007/s10461-021-03474-8
- Rumberger, R. W., & Lim, S. A. (2008). Why students drop out of school: A review of 25 years of research (California Dropout Research Project #15). University of California. http://www.cdrp.ucsb.edu/pubs_reports.htm
- Shazi, Z., Groves, A. K., Bruns, L., Gebrekristos, L. T., Moletsane, R., Maman, S., & Moodley, D. (October, 2018). Describing the feasibility and acceptability of an intervention designed to increase adolescent mothers return to school postpartum. International Workshop on HIV and Adolescence, Cape Town, South Africa.
- Sherr, L., Hensels, I. S., Skeen, S., Tomlinson, M., Roberts, K. J., & Macedo, A. (2016). Exposure to violence predicts poor educational outcomes in young children in South Africa and Malawi. International Health, 8(1), 36–43. https://doi.org/10.1093/inthealth/ihv070
- Singh, R., & Mukherjee, P. (2018). Push out, pull out, or opting out? Reasons cited by adolescents for discontinuing education in four low-and middle-income countries. In J. E. Lansford & P. Banati Eds., Handbook of adolescent development research and its impact on global policy (1st ed., pp. 239–259). Oxford University Press. https://doi.org/10.1093/oso/9780190847128.003.0012
- Snider, L, & Dawes, A. (2006). Psychosocial vulnerability and resilience measures for national-level monitoring of orphans and other vulnerable children: Recommendations for revision of the UNICEF psychological indicator. Cape Town: UNICEF. https://bettercarenetwork.org/sites/default/files/Psychosocial%20Vulnerability%20and%20Resilience%20Measures%20for%20National-Level%20Monitoring%20of%20Orphans%20and%20Other%20Vulnerable%20Children.pdf
- Spaull, N. (2019, January 19). Priorities for educational reform (Background Note for Minister of Finance. Nic Spaull. (Retrieved 19/January/2019). https://nicspaull.com/2019/01/
- Statistica (2022). Distribution of modes of transport used to get to school in South Africa in 2019. https://www.statista.com/statistics/1116057/distribution-of-transportation-used-to-get-to-school-in-south-africa/
- Statistics South Africa. (2001). South African Census 2001: Household Questionnaire.
- Stoner, M. C., Rucinski, K. B., Edwards, J. K., Selin, A., Hughes, J. P., Wang, J., Agyei, Y., Gomez-Olive, F. X., MacPhail, C., Kahn, K., & Pettifor, A. (2019). The relationship between school dropout and pregnancy among adolescent girls and young women in South Africa: A HPTN 068 analysis. Health Education & Behavior, 46(4), 559–568. https://doi.org/10.1177/1090198119831755
- Swartz, C., Colvin, Harrison, C., & Harrison, A. (2018). The problem or the solution? Early fertility and parenthood in the transition to adulthood in Khayelitsha, South Africa. Reproductive Health Matters, 26(54), 145–154. https://doi.org/10.1080/09688080.2018.1537417
- Timæus, I. M., Simelane, S., & Letsoalo, T. (2013). Poverty, race, and children’s progress at school in South Africa. The Journal of Development Studies, 49(2), 270–284. https://doi.org/10.1080/00220388.2012.693168
- Timæus, I. M., & Moultrie, T. A. (2015). Teenage childbearing and educational attainment in South Africa. Studies in Family Planning, 46(2), 143–160. https://doi.org/10.1111/j.1728-4465.2015.00021.x
- United Nations (2020). World Fertility 2019 - Early and Later Childbearing among Adolescent Women. https://www.un.org/en/development/desa/population/publications/pdf/fertility/World_Fertility_2019.pdf
- United Nations Children’s Fund (2021). Parenting of adolescents. Programming Guidance for Parenting of Adolescents. https://www.unicef.org/documents/parenting-adolescents-programming-guidance
- van Breda, A. D., & Theron, L. C. (2018). A critical review of South African child and youth resilience studies, 2009–2017. Children and Youth Services Review, 91(1), 237–247. https://doi.org/10.1016/j.childyouth.2018.06.022
- Van Broekhuizen, H., & Spaull, N. (2017). The ‘Martha Effect’: The compounding female advantage in South African higher education. Stellenbosch: Stellenbosch Economic Working Papers.
- Watkins, J. A., Sello, O. M., Cluver, L., Kaplan, L., & Boyes, M. (2014). ‘At School I Got Myself a Certificate’: HIV/AIDS orphanhood and secondary education: A qualitative study of risk and protective factors. Global Social Welfare, 1(3), 111–121. https://doi.org/10.1007/s40609-014-0022-9
- World Health Organization (1998). The second decade: Improving adolescent health and development. https://apps.who.int/iris/handle/10665/64320