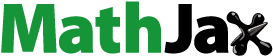
Abstract
Infectious diseases are a major threat to Asian shrimp aquaculture, as they proliferate at system level rather than only the individual level. We assess the impact of various forms of cooperation among Vietnamese farmers on yield risks caused by white spot disease and acute hepatopancreatic necrosis disease. Using a stochastic simulation model, we simulate shrimp farming yield risks based on input from two expert workshops. The results provide a relative comparison of expected yield losses caused by both diseases comparing a baseline scenario (no cooperation) and three scenarios with varying degrees of synchronization and information sharing across farms. Results show lower expected yield losses in all three cooperation scenarios in comparison with the farm-based scenario, highlighting the value of synchronization and information sharing practices to mitigate yield losses. We discuss the potential this has to reduce systemic risks in aquaculture, thereby potentially incentivizing the reintroduction of risk-sharing mechanisms.
Introduction
Infectious diseases remain a major problem for shrimp aquaculture, particularly in Asia, where approximately 60% of losses are caused by viral pathogens and 20% by bacterial pathogens (FAO, Citation2020). White spot disease (WSD) and acute hepatopancreatic necrosis disease (AHPND) continue to be the most serious diseases (Thitamadee et al., Citation2016) causing significant losses on Asian—including Vietnamese—shrimp aquafarms (Asche et al., Citation2020). In Vietnam, about 80% and 95% of areas affected by WSD and AHPND respectively are in open production systems.
The Mekong Delta is one of the leading shrimp cultivation regions in Vietnam, both with regard to size and production volume. In 2018, the total farmed area in this region was around 720,000 ha with an average production of 745,000 tons per year (MARD, Citation2019). The average size of farms in the region is 1.13 ha, 0.95 ha, 1.93 ha, and 4.31 ha for intensive, semi-intensive, improved extensive, and integrated mangrove shrimp systems, respectively (Lien et al., Citation2021). Annual yields across these production systems range between 6,191 kg/ha/year for intensive systems to 299 kg/ha/year for integrated mangrove systems (Joffre & Bosma, Citation2009). Like most other countries in Southeast Asia, Littopenaeus vannamei and Panaeus monodon are cultivated—with L. vannamei the more intensively produced species with up to three crops per year, while P. monodon are produced in up to two crops per year. The shrimp farming sector is dominated by small-holder farmers (80%) who contribute more than 80% of the national production volume (GSO, Citation2020). Despite shrimp farming being a major livelihood strategy in the Mekong Delta, various economic risks are present that undermine the stainability small-holder livelihoods (Ha et al., Citation2013).
Small-scale shrimp farmers in the Mekong Delta face various systemic production risks when it comes to infectious disease, i.e. risks that proliferate at system level rather than only at the individual farm level, as pathogens can easily spread in open systems with outdoor earthen ponds (Bush et al., Citation2010; Corsin et al., Citation2005) or with shared water sources (Hoa et al., Citation2011). In 2015, disease outbreaks of WSD and AHPND in the Mekong Delta led to losses of US$ 55.58 million and US$ 97.96 million, respectively (Hien et al., Citation2016). The systemic nature of the risk impedes certain risk-sharing solutions, such as insurance schemes or supply and risk pooling agreements across supply chains (Meuwissen et al., Citation2019).
Disease prevention and mitigation has been mainly focused on farm-based practices to improve farm performance and adoption of technological solutions. Examples include standardized protocols for pond preparation and water treatment (Pongthanapanich et al., Citation2019; Tang et al., Citation2020). Farmers control water quality through, for e.g., biosecurity measures, bio-floc, minimization of water exchange, and instruments to monitor feeding patterns (Dey et al., Citation2020; Thitamadee et al., Citation2016).
However, whether such farm-based approaches are sufficient to address disease risk, which is highly dependent on systemic factors, can be questioned. First, as argued by Bottema et al. (Citation2019) and Bush et al. (Citation2019), these farm-based practices do not provide incentives for farmers in a given area to cooperate. Second, though technical solutions can enhance individual farm performance (Crab et al., Citation2012; N. T. K. Duy et al., Citation2015; Tendencia et al., Citation2012), they are costly and therefore hard to implement for the majority of small-scale farmers (Hasan et al., Citation2020; Ulhaq et al., Citation2022). In addition, while aquaculture farmers are assigned to groups or cooperatives to follow local regulations (Ha et al., Citation2013, Joffre et al., Citation2019), farmers may not know each other or do not hold the same perception of production risks, which results in a lack of incentives for collaboration (Bottema et al., Citation2019). As such, these groups do not benefit from collaborative measures designed to mitigate water quality and disease incidence such as the synchronization of farming practices (e.g. water discharge, harvesting, and stocking) and the sharing of information on farm activities. Lien et al. (Citation2021) found that shrimp farmers cooperate and share more information with neighboring farmers who experience the same production risks, namely, disease, stemming from a shared common water source, or with their peers whom they trust. They concluded that this so-called “socio-spatial connectivity” is crucial to collectively shape risk behavior. We therefore hypothesize that losses due to contagious diseases in shrimp farming become less systemic if the motivation for “beyond farm cooperation and information sharing” can be deployed to incentivize joint activities stimulating risk prevention and mitigation.
The objective of this paper is to assess how various forms of cooperation among farmers affect yield risks caused by two infectious shrimp diseases, i.e. WSD and AHPND. The analysis focuses on open systems as they are most frequently used by small-scale farmers in Vietnam. As data on the effect of cooperation on yield risks is lacking, expert elicitation is used to parameterize a stochastic simulation model, comparable to Meuwissen et al. (Citation2003), who used expert elicitation to model the risk of swine epidemics and inform the design of epidemic disease insurance.
The study is outlined as follows: the “WSD and AHPND in shrimp farming in Vietnam” section describes the epidemiological background of WSD and AHPND in shrimp farming in Vietnam. The “Materials and methods” section presents the materials and methods used in this study, including: the description of three different cooperation scenarios, the workshops employed for expert elicitation, and the set-up of the stochastic simulation model bringing the various expert estimations together into yield losses for a hypothetical area of 1,000 shrimp farms. Findings of the workshops and the stochastic simulation model are listed in the “Results” section, followed by a discussion and conclusion in the “Discussion” and “Conclusion” section respectively.
WSD and AHPND in shrimp farming in Vietnam
WSD and AHPND are caused by a white spot syndrome virus and strains of Vibrio parahaemolyticus that contain a unique virulence plasmid respectively (OIE, Citation2021). The mass mortality of these two shrimp diseases has been explored in a variety of studies (Dey et al., Citation2020; Tang et al., Citation2020; Thitamadee et al., Citation2016; Tuyen et al., Citation2014). The virus causing WSD may lead to 100% mortality of shrimp cultures in as few as 3 to 10 days (Dey et al., Citation2020; OIE, Citation2021). In contrast, mortality caused by AHPND occurs slower and reaches about 70% to 100% within 30 to 45 days after stocking in shrimp cultivation ponds with post larvae (PL) (OIE, Citation2021; Thitamadee et al., Citation2016). Although the mortality rate of these diseases is high, exact information about their prevalence is limited (Kelly & Renukdas, Citation2020). Nevertheless, persistent occurrence of the pathogens causing WSD and AHPND in shrimp ponds and common water sources was confirmed by various authors including Hong To et al. (Citation2020), Hoa et al. (Citation2011) and Anh et al. (Citation2010).
WSD and AHPND can be transmitted vertically from brood stooks and horizontally via contaminated water through cannibalism of sick and dead shrimp in a pond (Tang et al., Citation2020; Thitamadee et al., Citation2016) or through vectors such as crabs or birds (Sánchez-Paz, Citation2010; Tuyen et al., Citation2014). However, disease occurrence depends on many factors such as farm site characteristics, culture period, water management, stocking density, feed and medical inputs, biosecurity measures and a range of environmental factors including water temperature, pH and salinity (Boonyawiwat et al., Citation2017, Citation2018; Hasan et al., Citation2020; Millard et al., Citation2021; Tendencia et al., Citation2011).
To manage the risks related to WSD and AHPND, shrimp farmers usually adopt farm-based risk prevention strategies during the preparation and cultivation phase. These focus on: adequate preparation and disinfection of the shrimp pond, implementation of biosecurity measures, use of good quality PL from reputable hatcheries, control of water quality, adherence to a seasonal crop calendar, and detailed monitoring of shrimp health (Corsin et al., Citation2001, Citation2005; Dey et al., Citation2020; Flegel, Citation2019; Hasan et al., Citation2020; Millard et al., Citation2021; Tang et al., Citation2020; Tendencia et al., Citation2011; Thitamadee et al., Citation2016). At present, farmers have very limited opportunities to mitigate the consequences after a WSD outbreak or a late diagnosis of AHPND (Dey et al., Citation2020; Tang et al., Citation2020).
Materials and methods
The overall methodological approach of this paper is based on a stochastic simulation model of shrimp farming yield risks that is calibrated on data from two expert elicitation workshops. A base farm-based scenario is compared to three cooperation scenarios, that will be introduced first in the next section. The subsequent sections will then develop the yield risk estimation, detail the expert workshops and finally develop the stochastic simulation model and its sensitivity analysis.
A farm-based scenario and three cooperation scenarios
The farm practices as described in “WSD and AHPND in shrimp farming in Vietnam” section are the basis for the “Farm-based scenario.” This base scenario reflects a situation without explicit cooperation activities and includes only individual farm practices ().
Table 1. Farm practices in farm-based scenario and additional practices in three cooperation scenarios.
The cooperation scenarios reflected in , i.e., “Synchronization,” “Information sharing” and “Combination,” are not yet implemented formally in Vietnam—although practices of these scenarios have already been discussed in literature. For instance, as suggested by Pongthanapanich et al. (Citation2019), frequently sharing information among social relations and synchronizing risk management at the regional level can reduce the spread of infectious disease to other areas. Along the same line, there are many studies on shrimp farming in Vietnam that found that information sharing within socio-spatially connected networks has a positive impact on the adoption disease control measures (Joffre et al., Citation2020; Lien et al., Citation2021). As argued by Bottema et al. (Citation2019), farmers have a greater incentive to adopt these cooperation practices with farmers who either experience similar risks or who are close in their social relations. As such, we design three cooperation scenarios based on two main practices: “Synchronization” and “Information sharing.”
Conceptualizing yield risks
Yield loss is our main indicator of yield risk (Harwood, Citation1999). Yield losses were estimated by the difference between the yield potential and the yield that materializes when a disease occurs, multiplied with the shrimp selling price per kg at farm gate:
(1)
(1)
Yieldpotential is defined as the yield which could potentially be achieved in the absence of disease. It is derived from the literature (Yieldreference) corrected for prevalence and mortality rates, also obtained from literature (Prevalencereference and Mortalityreference respectively). Note that due to the lack of information on yield loss for specific diseases, these reference yields and rates are defined in general terms:
(2)
(2)
The yield impact of a specific disease is determined by its prevalence and mortality rate, in our case specific for WSD and AHPND:
(3)
(3)
We make use of these equations to estimate the yield losses per crop per farm. Prevalence and mortality rates vary per scenario (see below).
Expert elicitation workshops on estimation of prevalence and mortality rate in cooperation scenarios
Two separate workshops were organized in May 2022 via 2-h online meetings. The first workshop with five international experts (three professors, one certification expert, one industry specialist) was presented and discussed in English and the second with nine experts from Vietnam (four associated professors, one senior researcher, one certification expert, three local government staff) was conducted in Vietnamese. The consulted experts have knowledge and experience about disease transmission and landscape aquaculture management and included local and global aquaculture specialists, aquaculture researchers in universities and research institutes, and local government staff. Separate workshops were organized to avoid language barriers that could hamper the discussion or lead to misunderstandings.
The objectives of the workshops were to (1) present the three cooperation scenarios, i.e. “Synchronization,” “Information sharing,” and “Combination” and (2) critically evaluate and assess the prevalence and mortality rate of WSD and AHPND in each scenario.
At the beginning of the workshop, the context of the risk analysis was presented and discussed, such as the specificities of shrimp farming in the Mekong Delta, the actual disease situation, and current risk management strategies. In addition, the characterization of the farm-based and cooperation scenarios was presented and discussed. was used to specify the type of strategies in each scenario. The next phase of the workshop focused on the parameterization of the prevalence and mortality rates. For the “Farm-based” scenario, we used values from literature and shared these with the experts, i.e. the prevalence and mortality rates of WSD ranges from 40% to 71% and from 80% to 100%, respectively (according to Desrina et al. [2022] and OIE [Citation2021]), while for AHPND these range from 52%to 87% (Nguyen et al., Citation2021) and from 40% to100%, respectively (according to Boonyawiwat et al. [Citation2018] and OIE [Citation2021]). Next, we used individual online spreadsheets to elicit experts’ individual assessments of the prevalence and mortality rates for each disease in each cooperation scenario. To account for uncertainty, experts were always asked to indicate three values: the most likely, the minimum and the maximum value.
The final step of the workshop was a plenary session aimed at reaching group consensus for each parameter. To do so, we first calculated the average values of all experts’ individual assessments for all parameters. Second, a discussion was initiated among the experts to reach consensus about the average values. Following Kaner (Citation2014) we used an agreement level of at least 80% to acceptably reflect consensus. and in the Appendix present the results of the expert parameter elicitation in the two workshops.
Stochastic simulation model and model variables
We use a stochastic simulation modeling approach which generates distributions of model outputs based on the variability of input parameters that are calibrated to realistic values (e.g. see Clark et al., Citation2010; Ngoc et al., Citation2016). For the stochastic simulation model, we start with the yield loss per crop at one farm, and then scale this up to annual yield losses of a hypothetical area with 1,000 farms. At farm level, the number of crops cultivated per year is assumed to range from one to three with different probability values. In the model, the losses are simulated independently for each crop per year. The model input variables along with their units of measurement are listed in .
Table 2. Overview of input data in the farm-based scenario and the different cooperation scenarios.
The stochastic simulation model was created in Microsoft Excel with the add-in @Risk version 8.2 (Palisade, Citation2020). The Monte Carlo sampling method was used to sample values from input distributions with 10,000 iterations, which was considered sufficient to produce a stable output distribution. The model is based on several assumptions. First, we assume that the disease risk control practices in the different scenarios are taken up adequately by all 1,000 farmers. This is based on our understanding of the research area that when farmers cooperate in (many but) small social networks., e.g. around their common water source, there is more intrinsic motivation for so-called beyond farm cooperation. Second, we assume that, despite the size of the area, the price per kg shrimp is not affected by the level of disease. Experience from e.g. the African swine fever outbreaks in China show that prices are only affected in case of major disease outbreaks (Mason-D’Croz et al., Citation2020). Third, we did not estimate disease virulence as a separate variable and assume it to be part of mortality. While virulence is an important factor to explain disease consequences and losses (Dey et al., Citation2020; Kelly & Renukdas, Citation2020) its role is more or less the same across open production systems with variation of stocking density, which are the focus of this risk analysis. When modeling intensive systems with high levels of biosecurity in place, we expect virulence to play a more relevant role (Hoa et al., Citation2011).
Model parameterization and sensitivity analysis
In order to parametrize the model, data were collected from two sources: a literature review and expert elicitation (as clarified in ). The Yieldreference is the yield mentioned in the literature, which is usually affected by the normal disease prevalence rate (the so-called prevalence ratereference) ranging from 10% to 20% per crop at farm level (Phong et al., Citation2021) and the mortality ratereference which ranges from 10% to 30% per crop at farm level. This is in line with the average survival rate of 70% to 90% as reported by Ngoc et al. (Citation2021). The number of crops per year is one, two or three with a probability of 0.35, 0.59, and 0.06, respectively, based on D. T. Duy et al. (Citation2021). The values of minimum, most likely and maximum shrimp prices at farm gate are 30, 130 and 190 VND per kg respectively, according to a study of Le et al. (Citation2022). To estimate the impact of WSD and AHPND in the “Farm-based” scenario, we combined information from multiple sources. For WSD, parameters are based on Desrina et al. (Citation2022) for the disease prevalence rate and on OIE (Citation2021) and Thitamadee et al. (Citation2016) for the mortality rate. For AHPND information was retrieved from Nguyen et al. (Citation2021) for prevalence and from Boonyawiwat et al. (Citation2018) and OIE (Citation2021) for mortality. Exact values are shown in .
The impact of uncertainty in the input variables in the various scenarios was assessed using the built-in sensitivity analysis of @Risk 8.2. The sensitivity analysis was carried out using Spearman rank correlation coefficients as the relationship between some inputs and the outputs of the area yield losses were nonlinear.
Results
Impact of cooperation strategies on WSD losses
The cumulative distribution functions of expected yield losses are shown in with results based on the workshop conducted in English on the left and the one conducted in Vietnamese on the right. Corresponding loss numbers are shown in in the Appendix. Our results demonstrate that losses in the cooperation scenarios are expected to be consistently lower than in the baseline model, i.e. the “Farm-based” loss curve is consistently positioned more to the right, demonstrating higher losses. Looking into the cooperation scenarios in more detail, we find that experts participating in the workshop conducted in English expect synchronization of practices to be the most effective (curve is positioned most to the left), while the experts in the second workshop attribute the highest merit to the scenario in which synchronization was combined with information sharing. Experts in the workshop in English indicated that in the case of WSD there is very little time for information sharing, which likely explains their priority for “Synchronization.” The rapid spread of WSD was also mentioned by Dey et al. (Citation2020) and Desrina et al. (Citation2022).
Impact of cooperation strategies on AHPND losses
The cumulative distribution functions of simulated expected yield losses for AHPND are shown in . Averages, standard deviation and 5% and 95% percentiles are in in the Appendix. In line with the results for WSD, cooperation scenarios outperform the scenario in which disease management in purely based on farm-level practices. With regard to the cooperation scenarios, “Synchronization” outperforms “Information sharing” and experts in both workshops agreed that for AHPND, a scenario in which synchronization of practices is combined with information sharing would be most effective (the “Combination” curve is most to the left).
Sensitivity analysis
The input variables with the largest effect on annual yield losses are reported in and in the Appendix. The most influential variables in all three cooperation scenarios are the number of crops per year and the actual yield (Yielddisease) with a correlation coefficient greater than 0.6 and 0.3, respectively. This is a sensible result as the number of crops scales actual yield multiplicatively, which in itself is a major source of variation. Three other model inputs which are found to be influential are: shrimp price, disease prevalence rate and mortality rate.
Discussion
The results of the stochastic simulation modeling show that cooperation scenarios are promising to reduce the systemic risk of WSD and APHND in open production aquaculture systems. This builds on work by Bottema et al. (Citation2019) and Lien et al. (Citation2021) who argue that the socio-spatial connectivity between farmers empowers their collaboration, thereby leading to better synchronization of risk mitigation practices and information sharing. The importance of social interactions within farmer groups to explain behavior and change of practices was also discussed by Spielman et al. (Citation2011). Their results suggest that “bottom-up” strategies are more effective than “top-down” approaches, such as described by Bush et al. (Citation2019) to manage the systemic nature of disease risk. As such, we argue that the cooperation scenarios in this paper provide a useful guide for bottom-up strategies, especially in areas with a relatively strong socio-spatial connectivity.
Although experts’ estimations did not entirely converge across scenarios, their overall appreciation for the cooperation scenarios was evident. Moreover, the remarks in the plenary discussions yielded useful additional insights. For instance, all experts agreed that virulence was not explicitly addressed in the current assessment but that the type of practices as included in the cooperation scenarios in the long run also positively affects the virulence, thereby further reducing the systemic nature of the disease risk in open production systems. In addition, experts expected that the practices also have a positive effect on overall yield levels. This might be a further incentive for farmers to implement such practices.
Even though yield variability is recognized as a major driver of Vietnamese aquaculture revenue volatility (Petersen & Schilizzi, Citation2010), future research could explore further the potential impact of prevention of WSD and AHPND outbreaks on price developments and price volatility in the longer run.
Conclusion
The stochastic simulation model developed provides a relative comparison of the yield losses caused by two shrimp diseases, WSD and AHPND, between a farm-based scenario that involves no cooperation and three different cooperation scenarios. The results are scaled to a hypothetical area of 1,000 shrimp farms in the Mekong Delta in Vietnam. Due to the lack of information on the effects of cooperation beyond the farm, we make use of expert elicitation to assess the two main parameters of our stochastic simulation model: the prevalence rate and mortality rate for the two different diseases. Our results show that experts indicate that all of the three cooperation scenarios would result in lower yield losses upon WSD and AHPND outbreaks in comparison with the farm-based scenario. More fundamentally, these results illustrate the promise of cooperation practices fueled by small social networks where so-called socio-spatial connectivity drives intrinsic motivation for joint risk management to combat contagious diseases.
The observed effects of the cooperation scenarios from our stochastic simulation model in mitigating the systemic risk in aquaculture industry are still at the early stage of development and need further research. However, they provide a basis for thinking about the future configuration of cooperation beyond the farm level. The outcomes of our model might also be used to convince farmers about the potential financial benefits of collaborative management. In addition, the cooperation scenarios and outcomes of the risk analysis can inspire private actors, such as banks, insurance companies, wholesalers and processors, to design risk-sharing solutions with Vietnamese small-holders, thereby taking away downside risk and empowering sustainable development.
Author contributions
Ho Hong Lien: Conceptualization, Investigation, Methodology, Data collection and analysis, Writing—original draft, Writing—review and editing. Yann de Mey: Conceptualization, Investigation, Data collection and analysis, Writing—review and editing. Dang Kieu Nhan: Conceptualization, Methodology, Data collection, Investigation. Simon Bush: Conceptualization, Investigation, Methodology, Writing—review and editing. Miranda Meuwissen: Conceptualization, Investigation, Data collection and analysis, Writing—review and editing.
Acknowledgements
We would like to thank all experts who participated in the workshops.
Disclosure statement
No potential conflict of interest was reported by the author(s).
Additional information
Funding
References
- Anh, P. T., Kroeze, C., Bush, S. R., & Mol, A. P. J. (2010). Water pollution by intensive brackish shrimp farming in south-east Vietnam: Causes and options for control. Agricultural Water Management, 97(6), 872–882. https://doi.org/10.1016/j.agwat.2010.01.018
- Asche, F., Anderson, J. L., Botta, R., Kumar, G., Abrahamsen, E. B., Nguyen, L. T., & Valderrama, D. (2020). The economics of shrimp disease. Journal of Invertebrate Pathology, 186, 107397. https://doi.org/10.1016/j.jip.2020.107397
- Boonyawiwat, V., Nga, N., & Bondadreantaso, M. G. (2018). Risk factors associated with acute hepatopancreatic necrosis disease (AHPND) outbreak in the Mekong Delta, Viet Nam. Asian Fisheries Science S, 31, 226–241.
- Boonyawiwat, V., Patanasatienkul, T., Kasornchandra, J., Poolkhet, C., Yaemkasem, S., Hammell, L., & Davidson, J. (2017). Impact of farm management on expression of early mortality syndrome/acute hepatopancreatic necrosis disease (EMS/AHPND) on penaeid shrimp farms in Thailand. Journal of Fish Diseases, 40(5), 649–659. https://doi.org/10.1111/jfd.12545
- Bottema, M. J. M., Bush, S. R., & Oosterveer, P. (2019). Moving beyond the shrimp farm: Spaces of shared environmental risk? The Geographical Journal, 185(2), 168–179. https://doi.org/10.1111/geoj.12280
- Bush, S. R., Oosterveer, P., Bottema, M., Meuwissen, M., de Mey, Y., Chamsai, S., Lien, H. H., & Chadag, M. (2019). Inclusive environmental performance through ‘beyond-farm’ aquaculture governance. Current Opinion in Environmental Sustainability, 41, 49–55. https://doi.org/10.1016/j.cosust.2019.09.013
- Bush, S. R., van Zwieten, P. A., Visser, L., van Dijk, H., Bosma, R., de Boer, W. F., & Verdegem, M. (2010). Scenarios for resilient shrimp aquaculture in tropical coastal areas. Ecology and Society, 15(2), 15. https://doi.org/10.5751/ES-03331-150215
- Clark, J. L., Weldon, R. N., Adams, C. M., & Wirth, F. F. (2010). Risk assessment of a shrimp aquaculture investment in Florida. Aquaculture Economics & Management, 14(4), 332–357. https://doi.org/10.1080/13657305.2010.526023
- Corsin, F., Turnbull, J. F., Hao, N. V., Mohan, C. V., Phi, T. T., Phuoc, L. H., Tinh, N. T. N., & Morgan, K. L. (2001). Risk factors associated with white spot syndrome virus infection in a Vietnamese rice-shrimp farming system. Diseases of Aquatic Organisms, 47(1), 1–12. https://doi.org/10.3354/dao047001
- Corsin, F., Turnbull, J. F., Mohan, C., Hao, N., & Morgan, K. (2005). Pond-level risk factors for white spot disease outbreaks. Diseases in Asian Aquaculture, V, 75–92.
- Crab, R., Defoirdt, T., Bossier, P., & Verstraete, W. (2012). Biofloc technology in aquaculture: Beneficial effects and future challenges. Aquaculture, 356-357, 351–356. https://doi.org/10.1016/j.aquaculture.2012.04.046
- Desrina, Prayitno, S. B., Verdegem, M. C. J., Verreth, J. A. J., & Vlak, J. M. (2022). White spot syndrome virus host range and impact on transmission. Reviews in Aquaculture, 14 (4), 1843–1860. https://doi.org/10.1111/raq.12676
- Dey, B. K., Dugassa, G. H., Hinzano, S. M., & Bossier, P. (2020). Causative agent, diagnosis and management of white spot disease in shrimp: A review. Reviews in Aquaculture, 12(2), 822–865. https://doi.org/10.1111/raq.12352
- Duy, D. T., Trung, T. Q., Lan, T. H. P., Berg, H., & Thi Da, C. (2021). Assessment of the impacts of social capital on the profit of shrimp farming production in the Mekong Delta, Vietnam. Aquaculture Economics & Management, 26(2), 152–170. https://doi.org/10.1080/13657305.2021.1947414
- Duy, N. T. K., Tu, N. D., Son, T. H., & Khanh, L. H. D. (2015). Automated monitoring and control system for shrimp farms based on embedded system and wireless sensor network. In 2015 IEEE International Conference on Electrical, Computer and Communication Technologies (ICECCT), Coimbatore, India (pp. 1–5). IEEE. https://doi.org/10.1109/ICECCT.2015.7226111
- FAO. (2020). Towards sustainability in the shrimp industry. https://www.fao.org/in-action/globefish/market-reports/resource-detail/en/c/1261310/
- Flegel, T. W. (2019). A future vision for disease control in shrimp aquaculture. Journal of the World Aquaculture Society, 50(2), 249–266. https://doi.org/10.1111/jwas.12589
- GSO. (2020). Statistical yearbook of 2020.
- Ha, T. T. P., van Dijk, H., Bosma, R., & Sinh, L. X. (2013). Livelihood capabilities and pathways of shrimp farmers in the Mekong Delta, Vietnam. Aquaculture Economics & Management, 17(1), 1–30. https://doi.org/10.1080/13657305.2013.747224
- Harwood, J. L. (1999). Managing risk in farming: Concepts, research, and analysis. US Department of Agriculture, ERS.
- Hasan, N. A., Haque, M. M., Hinchliffe, S. J., & Guilder, J. (2020). A sequential assessment of WSD risk factors of shrimp farming in Bangladesh: Looking for a sustainable farming system. Aquaculture, 526, 735348. https://doi.org/10.1016/j.aquaculture.2020.735348
- Hien, N. T., Huong, N. T. L., Chuong, V. D., Nga, N. T. V., Quang, P. H., Hang, B. T. V., & Long, N. V. (2016). Status of acute hepatopancreatic necrosis disease (AHPND) and other emerging diseases of penaeid shrimps in Viet Nam. In R. V. Pakingking Jr., E. G. T. de Jesus-Ayson, & B. O. Acosta (Eds.), Addressing Acute Hepatopancreatic Necrosis Disease (AHPND) and Other Transboundary Diseases for Improved Aquatic Animal Health in Southeast Asia: Proceedings of the ASEAN Regional Technical Consultation on EMS/AHPND and Other Transboundary Diseases for Improved Aquatic Animal Health in Southeast Asia, 22–24 February 2016, Makati City, Philippines (pp. 88–95). Aquaculture Department, Southeast Asian Fisheries Development Center.
- Hoa, T. T. T., Zwart, M. P., Phuong, N. T., Vlak, J. M., & de Jong, M. C. M. (2011). Transmission of white spot syndrome virus in improved-extensive and semi-intensive shrimp production systems: A molecular epidemiology study. Aquaculture, 313(1-4), 7–14. https://doi.org/10.1016/j.aquaculture.2011.01.013
- Hong To, T. T., Yanagawa, H., Khanh Thuan, N., Hiep, D. M., Cuong, D. V., Khai, L. T. L., Taniguchi, T., Kubo, R., & Hayashidani, H. (2020). Prevalence of Vibrio parahaemolyticus causing acute hepatopancreatic necrosis disease of shrimp in shrimp, Molluscan shellfish and water samples in the Mekong Delta, Vietnam. Biology, 9(10), 312. https://doi.org/10.3390/biology9100312
- Huong, N. T. L., Chuong, V. D., Nga, N. T. V., Quang, P. H., Hang, B. T. V., & Long, N. V. (2016, February 22–24). Status of acute hepatopancreatic necrosis disease (AHPND) and other emerging diseases of penaeid shrimps in Viet Nam. In Addressing Acute Hepatopancreatic Necrosis Disease (AHPND) and Other Transboundary Diseases for Improved Aquatic Animal Health in Southeast Asia: Proceedings of the ASEAN Regional Technical Consultation on EMS/AHPND and Other Transboundary Diseases for Improved Aquatic Animal Health in Southeast Asia (pp. 88–95). Aquaculture Department, Southeast Asian Fisheries Development Center.
- Joffre, O. M., & Bosma, R. H. (2009). Typology of shrimp farming in Bac Lieu Province, Mekong Delta, using multivariate statistics. Agriculture, Ecosystems & Environment, 132(1-2), 153–159. https://doi.org/10.1016/j.agee.2009.03.010
- Joffre, O. M., De Vries, J. R., Klerkx, L., & Poortvliet, P. M. (2020). Why are cluster farmers adopting more aquaculture technologies and practices? The role of trust and interaction within shrimp farmers’ networks in the Mekong Delta, Vietnam. Aquaculture, 523, 735181. https://doi.org/10.1016/j.aquaculture.2020.735181
- Joffre, O. M., Poortvliet, P. M. & Klerkx, L. (2019). To cluster or not to cluster farmers? Influences on network interactions, risk perceptions, and adoption of aquaculture practices. Agricultural Systems 173: 151–160.
- Kaner, S. (2014). Facilitator’s guide to participatory decision-making. John Wiley & Sons.
- Kelly, A. M., & Renukdas, N. N. (2020). 5 Disease management of aquatic animals. In F. S. B. Kibenge & M. D. Powell (Eds.), Aquaculture health management (pp. 137–161). Academic Press.
- Le, N. T. T., Hestvik, E. B., Armstrong, C. W., & Eide, A. (2022). Determinants of inefficiency in shrimp aquaculture under environmental impacts: Comparing shrimp production systems in the Mekong, Vietnam. Journal of the World Aquaculture Society, 53(5), 963–983. https://doi.org/10.1111/jwas.12874
- Lien, H. H., de Mey, Y., Bush, S. R., & Meuwissen, M. P. M. (2021). A socio-spatial index for risk management in shrimp aquaculture across landscapes. Aquaculture, 531, 735861. https://doi.org/10.1016/j.aquaculture.2020.735861
- MARD. (2019). Statistics. https://www.mard.gov.vn/en/Pages/default.aspx.
- Mason-D’Croz, D., Bogard, J. R., Herrero, M., Robinson, S., Sulser, T. B., Wiebe, K., Willenbockel, D., & Godfray, H. C. J. (2020). Modelling the global economic consequences of a major African swine fever outbreak in China. Nature Food, 1(4), 221–228. https://doi.org/10.1038/s43016-020-0057-2
- Meuwissen, M. P., Feindt, P. H., Spiegel, A., Termeer, C. J., Mathijs, E., de Mey, Y., Finger, R., Balmann, A., Wauters, E., Urquhart, J., & Vigani, M. (2019). A framework to assess the resilience of farming systems. Agricultural Systems, 176, 102656.
- Meuwissen, M. P. M., Van Asseldonk, M. A. P. M., & Huirne, R. B. M. (2003). Alternative risk financing instruments for swine epidemics. Agricultural Systems, 75(2-3), 305–322. https://doi.org/10.1016/S0308-521X(02)00072-0
- Millard, R. S., Ellis, R. P., Bateman, K. S., Bickley, L. K., Tyler, C. R., van Aerle, R., & Santos, E. M. (2021). How do abiotic environmental conditions influence shrimp susceptibility to disease? A critical analysis focussed on White Spot Disease. Journal of Invertebrate Pathology, 186, 107369. https://doi.org/10.1016/j.jip.2020.107369
- Ngoc, P. T. A., Le, V., Pham, T. T., Pham, H. C., Le, T. C., & Oude Lansink, A. (2021). Technical and scale efficiency of intensive white-leg shrimp (Litopenaeus vannamei) farming in Vietnam: A data envelopment analysis. Aquaculture Economics & Management, 27(1), 50–65. https://doi.org/10.1080/13657305.2021.2003483
- Ngoc, P. T. A., Meuwissen, M. P., Cong Tru, L., Bosma, R. H., Verreth, J., & Lansink, A. O. (2016). Economic feasibility of recirculating aquaculture systems in pangasius farming. Aquaculture Economics & Management, 20(2), 185–200. https://doi.org/10.1080/13657305.2016.1156190
- Nguyen, H. T., Van, T. N., Ngoc, T. T., Boonyawiwat, V., Rukkwamsuk, T., & Yawongsa, A. (2021). Risk factors associated with acute hepatopancreatic necrosis disease at shrimp farm level in Bac Lieu Province, Vietnam. Veterinary World, 14(4), 1050–1058. https://doi.org/10.14202/vetworld.2021.1050-1058
- OIE. (2021). Animal diseases. https://www.oie.int/en/what-we-do/animal-health-and-welfare/animal-diseases
- Palisade. (2020). @Risk, version 8.2. Palisade Corporation.
- Petersen, E., & Schilizzi, S. (2010). The impact of price and yield risk on the bioeconomics of reservoir aquaculture in Northern Vietnam. Aquaculture Economics & Management, 14(3), 185–201. https://doi.org/10.1080/13657305.2010.503466
- Phong, T. N., Thang, V. T., & Hoai, N. T. (2021). What motivates farmers to accept good aquaculture practices in development policy? Results from choice experiment surveys with small-scale shrimp farmers in Vietnam. Economic Analysis and Policy, 72, 454–469. https://doi.org/10.1016/j.eap.2021.09.015
- Pongthanapanich, T., Nguyen, K. A. T., & Jolly, C. M. (2019). Risk management practices of small intensive shrimp farmers in the Mekong Delta of Viet Nam. FAO Fisheries and Aquaculture Circular, C1194, I-20.
- Sánchez-Paz, A. (2010). White spot syndrome virus: An overview on an emergent concern. Veterinary Research, 41(6), 43. https://doi.org/10.1051/vetres/2010015
- Spielman, D. J., Davis, K., Negash, M., & Ayele, G. (2011). Rural innovation systems and networks: Findings from a study of Ethiopian smallholders. Agriculture and Human Values, 28(2), 195–212. https://doi.org/10.1007/s10460-010-9273-y
- Tang, K. F. J., Bondad-Reantaso, M. G., Arthur, J. R., MacKinnon, B., Hao, B., Alday-Sanz, V., Liang, Y., & Dong, X. (2020). Shrimp acute hepatopancreatic necrosis disease strategies manual. FAO Fisheries and Aquaculture Circular, C1190, 1–65.
- Tendencia, E. A., Bosma, R. H., Primavera, J. H., & Verreth, J. A. J. (2012). Effect of different mangrove-to-pond area ratios on influent water quality and WSSV occurrence in Penaeus monodon semi-intensive farms using the greenwater culture technique. Aquaculture, 362-363, 72–79. https://doi.org/10.1016/j.aquaculture.2012.07.020
- Tendencia, E. A., Bosma, R. H., & Verreth, J. A. J. (2011). White spot syndrome virus (WSSV) risk factors associated with shrimp farming practices in polyculture and monoculture farms in the Philippines. Aquaculture, 311(1-4), 87–93. https://doi.org/10.1016/j.aquaculture.2010.11.039
- Thitamadee, S., Prachumwat, A., Srisala, J., Jaroenlak, P., Salachan, P. V., Sritunyalucksana, K., Flegel, T. W., & Itsathitphaisarn, O. (2016). Review of current disease threats for cultivated penaeid shrimp in Asia. Aquaculture, 452, 69–87. https://doi.org/10.1016/j.aquaculture.2015.10.028
- Tuyen, N. X., Verreth, J., Vlak, J. M., & de Jong, M. C. M. (2014). Horizontal transmission dynamics of White spot syndrome virus by cohabitation trials in juvenile Penaeus monodon and P. vannamei. Preventive Veterinary Medicine, 117(1), 286–294. https://doi.org/10.1016/j.prevetmed.2014.08.007
- Ulhaq, I., Pham, N. T. A., Le, V., Pham, H.-C., & Le, T. C. (2022). Factors influencing intention to adopt ICT among intensive shrimp farmers. Aquaculture, 547, 737407. https://doi.org/10.1016/j.aquaculture.2021.737407
Appendices
Appendix B:
Additional tables
Table B1. Annual WSD expected yield losses in the farm-based scenario and three cooperation scenarios for a hypothetical area of 1,000 shrimp farms (in 1,000,000 VND).
Table B2. Annual AHPND expected yield losses in the farm-based scenario and three cooperation scenarios for a hypothetical area of 1,000 shrimp farms (in 1,000,000 VND).