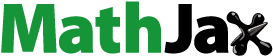
ABSTRACT
Lately, Open Data (OD) has been promoted by governments around the world as a resource to accelerate innovation within entrepreneurial ventures . However,it remains unclear to what extent OD drives innovative entrepreneurship. This paper sheds light on this open question by providing novel empirical evidence on the relationship between OD publishing and (digital) entrepreneurship at the country-level. We draw upon a longitudinal dataset comprising 90 countries observed over the period 2013–2016. We find a significant and positive association between OD publishing and entrepreneurship at the country level. The results also show that OD publishing and entrepreneurship is strong in countries with high institutional quality. We argue that publishing OD is not sufficient to improve innovative entrepreneurship alone, so states need to move beyond a focus on OD initiatives and promotion, to focus on a broader set of policy initiatives that promote good governance.
1. Introduction
Data has been highlighted as ‘the new oil’ to fuel innovation for the digital economy (Matsakis Citation2019; Parkins Citation2017; Dance, LaForgia, and Confessore Citation2018). Open Data (OD) has been promoted by governments as a resource to accelerate innovation in the digital economy by driving the creation of new products and processes within entrepreneurial ventures (Janssen et al. Citation2012; Hughes-Cromwick and Coronado Citation2019). OD refers to information that has been collected by an organisation (usually a public administration) which owns the IPR, but which is published online for other organisations and entrepreneurs to use in the development of new products and services and/or the formulation of new business models (Open Data Institute Citation2015). What is especially notable is how OD has to be made available at no cost, and which can be used by any organisation (Open Data Institute Citation2015). Advocates of the Open Data Movement claim that it reduces costs (as it is free), and enables entrepreneurs to retain more monetary value from new innovations, while providing access to previously unavailable data for experimentation in new prototypes (Lee, Almirall, and Wareham Citation2014; BIS Citation2014; Magalhaes and Roseira Citation2017). Advocates also claim that OD has the potential to advance entrepreneurial activities through innovation (Huijboom and Van den Broek Citation2011). OD may provide the information needed for the identification of new business opportunities, strategic planning and the evaluation of investment projects (Bonina Citation2013), in addition to being a core input to innovations (Lee, Almirall, and Wareham Citation2014; Hughes-Cromwick and Coronado Citation2019). However, these innovation benefits are still far from being fully understood and realised, and it remains unclear to what extent OD drives the formation of new enterprises with high innovation potential. (Lee, Almirall, and Wareham Citation2014; Almirall Citation2015; Huber, Wainwright, and Rentocchini Citation2020). For example, studies have examined government publication of OD, at the expense of investigating how entrepreneurs are able to use OD in their innovation activities. Furthermore, in common with studies of open innovation, research is often firm-centric (Bogers et al. Citation2017) with only anecdotal insight into OD use through sectoral analyses or case studies (Janssen et al. 2015; Corrales-Garay, Ortiz-de-Urbina-Criado, and Mora-Valentín Citation2019), with cross-comparative analysis at the country-level particularly lacking.
This contributes to our paper’s problem formulation. On the one hand, governments have become more involved in open innovation (Gershman, Roud, and Wolfgang Citation2019), particularly through OD publication to enhance transparency (Bates Citation2012), but to also provide OD to help entrepreneurs develop new products and services (Hughes-Cromwick and Coronado Citation2019). On the other hand, while research has highlighted OD use by entrepreneurs, there is much less evidence available on the wider effects of OD publication and how it facilitates the creation of innovative ventures (Jannsen et al. 2015). Although OD is ‘free’ to users, its publication is not without cost, owing to the resources needed to publish data, update it, respond to user queries, training, and in some cases lost revenue from making data freely available (Huber, Wainwright, and Rentocchini Citation2020). As such, with any government intervention to support entrepreneurship, the efficacy of policies needs to be assessed, to determine how well the support works owing to differences in institutions and contexts (Huijboom and Van den Broek Citation2011; Lundvall Citation2007; Choi and Park Citation2018). For instance, entrepreneurs in a country with a low-level of institutional quality may find the use of OD for innovation more difficult, with limited successful outcomes. Subsequently, this leads us to pose two questions: First, what is the association between the adoption of OD and innovative entrepreneurial activity at the country-level? Second, does the quality of institutions moderate the relationship between OD and the creation of innovative firms at the country-level?
This paper aims to shed light on these questions by providing novel empirical evidence on the relationship between OD publishing and entrepreneurial outcomes at country-level. We draw upon a longitudinal dataset comprising 90 countries observed over the period 2013–2016. Our results provide novel support for the argument that OD is beneficial for entrepreneurship and innovation. Overall, there is a significant and positive association between OD publishing and country-level entrepreneurship. The results also show that OD publishing and entrepreneurship is strong in countries with high institutional quality. We argue that unless a country has quality institutions, publishing OD alone does not positively affect entrepreneurship for the digital economy. Publishing OD is not sufficient to improve entrepreneurship and innovation alone, so state institutions need to move beyond a focus on OD promotion, to focus on a broader set of policy initiatives that promote good governance.
We contribute to an established stream of the literature in innovation and entrepreneurship studies, which highlights the contribution of new firms and small and medium enterprises (SMEs) to innovation and technological change (for a survey of the relevant literature please refer to Acs and Audretsch Citation2005). Since the seminal work of Schumpeter (Citation1929), we know that entrepreneurs historically introduce radical innovations, which subsequently transform existing technologies (Audretsch Citation2004) and spur economic growth via a process of creative destruction (Aghion and Howitt Citation1992). We contribute to a rich empirical literature on the antecedents of innovative entrepreneurship (see Block, Fisch, and Van Praag Citation2017 for a recent survey of the literature) by (i) showing the direct contribution of data published openly by governments worldwide to entrepreneurial activities; (ii) how the OD ‘resource’ can be effectively exploited if and only if the quality of institutions in the country is high (Gittelman Citation2006).
This paper is structured as follows. Section 2 provides a review of the extant literature and the main research questions. Section 3 discusses the data and methodology, and Section 4 the results. Section 5 concludes the paper.
2. Literature review and research questions
2.1. Open data in the digital economy
Researchers have long recognised the role of entrepreneurs in innovation and economic growth within national economies (Baumol Citation2002; Wolff and Pett Citation2006; Acs, Autio, and Szerb Citation2014; David, Aparicio, and Audretsch Citation2019). More recently, researchers have come to view data-driven start-ups as having the potential to disrupt existing markets and to create new economic value (Bogers et al. Citation2017; Dodgson, Gann, and Salter Citation2006; Huber Citation2013; Whelan et al. Citation2010). Data has been viewed as the ‘raw material’ for the digital economy, with scholars becoming interested in topics such as ‘big data’ (Chen and Zhang Citation2014) and ‘linked data’ (Wood Citation2010). One particular phenomenon within the field of the digital economy, which so far remains understudied is the concept of open data (OD) (Huber, Wainwright, and Rentocchini Citation2020).
OD is published by public and private sector organisations online and in machine-readable format, but is unique in that it is licenced for everybody to use and republish without financial costs (Open Data Institute Citation2015). For entrepreneurs in the digital economy, OD offers substantial potential, by avoiding the costs of acquiring proprietary data, and using it to create new value-added applications and services, overcoming resource constraints (Chan Citation2013; Janssen, Citation2012; Lee, Almirall, and Wareham Citation2014; Eftekhari and Bogers Citation2015; Nagaraj Citation2020). Furthermore, the publication of data by government agencies offers entrepreneurs new opportunities, through the release of data that was not previously available (Magalhaes and Roseira Citation2017). This has led to the emergence of OD ecosystems, where OD is published by government institutions and consumed by non-government actors such as corporations, universities, citizens and entrepreneurs, who may refine or re-share the OD and/or publish their own non-government OD, releasing it back into the ecosystem (Kitsios, Kamariotou, and Grigoroudis Citation2021; Heimstädt, Saunderson, and Heath Citation2014; Welle Donker and van Loenen Citation2017). These collaborative ecosystems, move beyond the simple sharing of data and can lead to the co-creation of new apps and services and emerged as part of national and sectoral OD Movements.
It is difficult to determine the exact beginning of the first Open Data Movement, but in 2009 the US government launched the Memorandum on Transparency and Open Government to make more government data public (Lee, Almirall, and Wareham Citation2014). European and emerging economy governments have also begun to publish OD, partly to enhance transparency (Bates Citation2012), but more recently to also provide data-driven opportunities for entrepreneurs in the digital economy (Lindman and Nyman Citation2014; Corrales-Garay, Ortiz-de-Urbina-Criado, and Mora-Valentín Citation2019). OD covers sectors such as transportation, location-based services, property, education, health and meteorological activities.
While there are a range of case studies concerning the successful use of OD by entrepreneurs, it remains unclear to what extent OD systematically leads to positive entrepreneurial outcomes (Lee, Almirall, and Wareham Citation2014; Almirall Citation2015; Huber, Wainwright, and Rentocchini Citation2020). For example, Corrales-Garay, Ortiz-de-Urbina-Criado, and Mora-Valentín (Citation2019) argue that OD can add $900 billion to the global economy, while Tinholt (Citation2013) calculated that the annual economic aggregate impact from apps based on OD across the EU17 is €140 billion.
While these headline statistics make for positive reading, the current body of research is underdeveloped, with a notable absence of wider systematic study, or deeper understanding into the mechanisms that lead to positive entrepreneurial outcomes. For instance, many earlier papers from the OD literature are conceptual McDermott Citation2010) or examine the design of OD ecosystems (Zuiderwijk, Janssen, and Davis Citation2014). In addition, the literature has been largely exploratory (Janssen et al. Citation2012), with research often focussing on qualitative methods or case study approaches, at the sectoral or Valentín Citation2019). In contrast, there are a limited number of quantitative studies relating to entrepreneurship, with few notable exceptions (Lakomaa and Kallberg Citation2013; Jones and Tonetti Citation2020; Nguyen and Paczos Citation2020; World Bank Group Citation2021). Similarly, there is a particular dearth of research on the cross-comparative aspects of OD, with the exception of consultancy and practitioner reports, such as the above, which are mostly descriptive (e.g. Tinholt Citation2013). While research on the public sector has often focussed on the risks, advantages and barriers of OD publication, it has often overlooked relationships with user communities and entrepreneurs (Huber, Wainwright, and Rentocchini Citation2020). There are many benefits advanced by proponents of OD to justify its publication, including a boost to economic growth, job creation, innovation and the development of transparency, but Huijboom and Van den Broek (Citation2011) argue that evidence of the precise effects are often lacking.
As such, we argue that a cross-comparative, macro-level and quantitative study will make a novel contribution to research on OD, particularly on how OD affects entrepreneurship, and how this relationship is moderated by country-level institutions.
2.2. Mechanisms linking OD to entrepreneurial activity
2.2.1. The association between OD and entrepreneurship
Digital entrepreneurship studies broadly seek to examine how digitisation transforms the venture creation process (Le Dinh, Vu, and Ayayi Citation2018) and how new entrepreneurial opportunities are created (Sahut, Iandoli, and Teulon Citation2021; Kraus et al. Citation2019). Within this context, the potential for data’s productive transformation on entrepreneurial pursuits has become an increased focus of attention (Obschonka and Audretsch Citation2020; Chhabra, Hassan, and Shamim Citation2021). A specific type of data is OD, which has been recognised for its positive effect on business and product development. For example, Magalhaes and Roseira (Citation2017) highlight how OD assists businesses in undertaking market analysis, process optimisation, product and service development, and R&D. More specifically, OD has been shown to be particularly important in supporting new firm creation and product development (Tinholt Citation2013; Huijboom and Van den Broek Citation2011; Huber, Wainwright, and Rentocchini Citation2020). There are several mechanisms that enable OD to support new firm creation. First, OD facilitates the creation of novel products and services that are reliant on OD (Chan Citation2013). OD consists of diverse and often large datasets that were not previously available, particularly sources of government OD (Magalhaes and Roseira Citation2017; Nagaraj Citation2020). Combining external OD with internal linked-data sets, can also unlock value through innovation (Hughes-Cromwick and Coronado Citation2019). Second, digitally focussed start-ups are reliant on data for their innovation. The costs of proprietary data can be high, which can create a barrier to new firm creation (Berends et al. Citation2020). The availability of OD can remove cost barriers to resource constrained firms, supporting firm formation. Third, a related mechanism centres on price reduction. The use of freely available OD can reduce costs for start-up services. Easily discoverable OD that can be accessed for free, where the data is harmonised, reduces administrative burdens and time costs, for new ventures improving efficiency (Estermann Citation2014; Berends et al. Citation2020). App pricing may not be financially viable, or desirable to consumers, if using expensive proprietary data, as this may increase costs of products to end users. The use of OD can reduce costs, making new digital apps and services financially viable. Fourth, access to OD can increase experimentation capacity for new ventures, enhancing innovation. As OD is freely available, resource constrained, new ventures are able to develop different prototypes of new services at low cost, without having to invest in proprietary data, which may not yield results (Huber, Wainwright, and Rentocchini Citation2020). This enables new ventures to experiment widely using OD in the pursuit of new products and service development, while also potentially reducing nascent entrepreneurs’ fear of failure (c.f Wennberg, Pathak, and Autio Citation2013).
Despite the potential positive effect that OD has on new ventures, its usefulness can vary in different dimensions. OD that is out of date, unreliable, and with availability constraints, can undermine its usefulness in innovation. In the following, we put forward three main mechanisms through which OD are expected to contribute to new firm formation at the country level: timeliness, source reliability and future availability.
2.2.2. OD timeliness
Timeliness links OD to entrepreneurship, the importance of which has been highlighted by researchers and practitioners, where timeliness refers to the how frequently OD is updated. OD can be published as a one-off static source of data as one snapshot in time, or it may be constantly undated in real-time through application programme interfaces (APIs) (Huber, Wainwright, and Rentocchini Citation2020).
OD that is newer and updated frequently has the potential to increase entrepreneurial opportunities (c.f. Tinholt Citation2013). First, if OD is published at a higher frequency, there is a larger volume of data. An abundance of OD can arguably contribute to the capture of opportunity recognition, as more OD can present more opportunities (Berends et al. Citation2020). Second, OD that is updated more frequently is likely to be more accurate providing a richer source of OD, with wider potential for wider uses. Tinholt (Citation2013) notes that granular data and depth is important, in addition to updating it regularly, an issue that 22% of countries overlook. Accurate and granular OD increases the potential of opportunity recognition and successful entrepreneurial orientation (cf. Davidsson Citation2015) due to potential for a wider range of applications.
One example frequently highlighted in the literature is that of transport APIs, where real-time OD is made available by transport providers to third-party entrepreneurs, who create transport and navigation apps that use OD (Lindman and Nyman Citation2014). While static timetable OD could be useful, richer OD available in real-time can account for delays, enabling users to make better decisions on transport routes.
2.2.3. OD source reliability
Source reliability of OD affects the ability of entrepreneurs to exploit data in new applications and services. While policy-makers may have previously focussed on the volume of OD published, scholars have highlighted the importance of information quality and in turn, the reliability of the data source (Lindman and Nyman Citation2014). This reliability is contingent on good governance of the OD publishing institutions (Huijboom and Van den Broek Citation2011)
In supporting entrepreneurs to capture opportunities using OD, better quality data provides opportunities to undertake more complex and niche tasks, with limited OD data functionality impeding commercialisation (Tinholt Citation2013). Poor quality OD cannot be used as widely, or there is limited information about the source quality and collection methods (Janssen, Charalabidis, and Zuiderwijk Citation2012). As such, OD following international standards and licencing can be used more easily by entrepreneurs and is more likely to be incorporated into new apps and services (Berends et al. Citation2020).
One particular example that relies upon data quality has involved the publication of geospatial OD (Nagaraj Citation2020). Location-based mobile apps are reliant on accurate data to assist users in navigation tasks. If OD geospatial data is inaccurate, it’s unusable by entrepreneurs, who would instead have to purchase more expensive proprietary data. In this event, an app or service may not be cost effective. Alternatively, entrepreneurs may seek to experiment with new apps and services using OD (Janssen, Charalabidis, and Zuiderwijk Citation2012; Huber, Wainwright, and Rentocchini Citation2020). If accurate data is not available, entrepreneurs may not experiment with a new service as they may not be able to afford proprietary data, or may discount a service as being unviable if the OD is inaccurate.
2.2.4. OD future availability
Future availability is a mechanism that links OD and entrepreneurship. OD needs to be sustained and entrepreneurs need to be confident that sources of OD will be available in the future (Lindman and Nyman Citation2014). As noted above, there can be variations in the frequency of OD publication, but OD sources can also be removed, or left unsupported.
If an entrepreneur is to develop an app or service around a source of OD, they need to be sure that the data will be available in the future (Berends et al. Citation2020). Even though OD is free, its exploitation requires the investment of resources. In the scenario where an OD source may not be available in the future, the entrepreneur may not develop the new app or service as it may fail if OD is withdrawn, so new products and services are not created or opportunities captured (Huber, Wainwright, and Rentocchini Citation2020). Concern over the future availability of data may be real, or perceived.
One particular example that relies on future OD availability concerns real-estate applications. Many apps enable citizens to view the availability of and quality of local amenities and services. OD on health and education quality are often available (Janssen, Charalabidis, and Zuiderwijk Citation2012). Many of these apps collate OD on local services. If these sources of OD were discontinued, a central feature of home search functionality would be lost.
Overall, having discussed potential mechanisms, there is a lack of systematic research on whether OD contributes to new firm formation. This leads us to highlight the first hypothesis we would like to test:
H1: There is a positive association between publishing OD and entrepreneurial activity at the country-level.
2.3. The role of institutions on the relationship between OD and entrepreneurship
Country-level institutions may influence how OD publication affects entrepreneurial activity. The role of the state goes beyond simply publishing OD as an available asset, as they also affect the context within which entrepreneurial processes and outcomes occur (Huijboom and Van den Broek Citation2011; Lindman and Nyman Citation2014). For example, country-level institutions that protect intellectual property can help entrepreneurs to develop viable business models when using OD, or provide education, training and discovery support to assist entrepreneurs in innovating with OD (Corrales-Garay, Ortiz-de-Urbina-Criado, and Mora-Valentín Citation2019). Furthermore, countries with better governance are more likely to have government units that liaise with users and entrepreneurs, to make sure that the three main OD characteristics (timeliness, source reliability and future availability) are adequately taken care of.
Recent work has highlighted how studies of entrepreneurship have long overlooked the role of country-level institutions on entrepreneurial activity (Acs, Autio, and Szerb Citation2014; Autio et al. Citation2014). Scholars have argued that studies have previously examined entrepreneurship at the individual cognitive-level (Shane and Venkataraman Citation2000) at the expense of the effect of country-level institutions,Footnote1 or have acknowledged the influence of context (Aldrich and Fiol Citation1994; Welter Citation2011), but have still focussed on the individual-level creating a gap in the literature (Sorensen Citation2007; Zahra and Wright Citation2011). This underplays the role of how institutions regulate choices and behaviour (Davidsson Citation2006).
A dedicated research stream has examined how entrepreneurial activity is influenced by the institutional context (Estrin, Korosteleva, and Mickiewicz Citation2013). Baumol (Citation1990) identified how institutions create the structure of incentives that determine the choice of entry into entrepreneurship, while North (Citation1990) argues that incentives for value adding behaviour depend on the quality of institutions. Furthermore, country-level institutional characteristics regulate resource allocation systems, which in turn determines individual opportunity pursuit (Acs, Autio, and Szerb Citation2014).
While the notion that institutions are the ‘rules of the game’ (North Citation1990) is widely utilised by scholars, there are a range of theoretical approaches. The role of institutional context has been conceptualised in various spatial scales. While we recognise the role of regional entrepreneurial ecosystems (Acs et al., Citation2017), this particular paper is concerned with the role of country-level institutions. In this paper, we understand institutional quality as country-level characteristics of governance levels regarding the implementation of rules supporting contractual relationships and market exchanges (Dau and Cuervo-Cazurra Citation2014). This is centred on the topics of broad property rights and corruption (Woodruff Citation2006), and high institutional quality means that the rule of law is applied and misbehaviour inhibited, where countries with the highest institutional quality have the ‘best’ institutions (Sobel Citation2008).
As entrepreneurial behaviour is about mobilising and coordinating resources – such as OD – there is less insight into how variations in context – for example, quality of institutions – determine how easily resources can be mobilised and opportunities recognised, and in turn how this influences entrepreneurial activity (Autio et al. Citation2014). As entrepreneurial activity is shaped by country-level institutions, due to specific institutional configurations (Boettke and Coyne Citation2003; Sobel Citation2008; Estrin, Korosteleva, and Mickiewicz Citation2013), differences in institutional quality will be likely to have an effect on (i) the quality and quantity of OD publishing and (ii) on the likelihood that OD as a raw material can be exploited by entrepreneurs, the latter being the focus of the paper.
Regarding (i), scholars have emphasised how good governance and leadership results in the publication of a greater volume of OD, but which is also of a better quality (Janssen et al. 2015; Lindman and Nyman Citation2014). Leadership and a responsibility for the publication of OD have been important for creating the infrastructure to publish quality OD (Berends et al. Citation2020). If leadership and good governance is absent, then it does not become a priority, leading to less data being published, which cannot then be used to create novel services and applications (Lindman and Nyman Citation2014). Studies have noted that in order to increase the publication of OD, formal policies are needed to steer government units into publishing OD (Huijboom and Van den Broek (Citation2011). The ease of extraction of OD for external use is affected by institutional quality, where the development of clear OD standards, metadata, contextual information and licencing is important in enabling entrepreneurs to acquire OD (Dawes and Helbig Citation2010; Lindman and Nyman Citation2014). While there is already some literature on (i), the focus of this paper is on the unexplored question (ii), the potential effect of institutional quality on the entrepreneurial exploitation of existing OD. Thus, the focus of our paper is on the important question of whether institutional quality moderates the relationship between OD and entrepreneurship at the country-level. The following mechanisms may be underlying.
First, institutional quality may affect how OD affects opportunity recognition as low quality institutions undermine the institutional trust needed for entrepreneurship (Anokhin and Schulze Citation2009; Hey and Trefethen Citation2005). A lack of trust in OD due to a low perception of the publishing quality institutions at the country-level may lead potential entrepreneurs to overlook opportunities. For instance, entrepreneurs may believe that OD published in a low quality institutional environment are not timely and reliable (c.f. Janssen et al. 2015). Also, a lack of trust in the public sector’s commitment to delivering a continuous stream of OD in the future may lead to lower levels of entrepreneurial activity, as future availability is important for returns on investment. Furthermore, these perceptions may lead to overestimating the risks; that is, even if entrepreneurs see an opportunity, they may reject and not pursue it (Cacciotti et al. Citation2016). These dimensions may be based on a low perception of trust in OD quality, even if the quality may be high, as nascent entrepreneurship often relies on subjective perceptions (Arenius and Minniti Citation2005).
Second, the dysfunctional effects of low quality of institutions on training and skill development may lead to lack of entrepreneurial abilities (c.f. Huber, Wainwright, and Rentocchini Citation2020). That is, those dysfunctional effects may mean that there are fewer entrepreneurs with the ability to utilise publicly available OD for innovation as low quality institutions reduce the required capabilities in the digital economy (e.g. data science skills, for an overview of required skills see Open Data Institute Citation2019).
Third, high quality institutions can facilitate the development of good relationships between government publishers of OD and the user community, for example through supported innovation contests (Juell-Skielse et al. Citation2014). This relationship improves and enhances the ability of entrepreneurs to realise the value of OD and to recognise opportunities that can be captured (c.f Zurada and Karwowski Citation2011). Countries with better governance and quality institutions appear to be more transparent and open to engaging with external innovation (c.f. Janssen et al. 2015). Transparent procedures and rules supporting contractual relationships and market exchanges make a development of a constructive relationship between OD publishers and OD users more likely.
Fourth, trust in the future availability of OD. Trust in the rule of law including IP may affect entrepreneurial aspirations, by reducing a fear of failure (Cacciotti et al. Citation2016). For instance, corruption-related costs and risks may be a disincentive to invest in entrepreneurial growth (Dutta and Sobel Citation2016).
In light of the above theoretical arguments, our study attempts to investigate a second hypothesis:
H2: The quality of institutions moderates the relationship between OD and entrepreneurship at the country-level.
3. Data description and method
3.1. Data sources
The empirical analysis is based on a dataset that has been obtained by combining six different sources of country-level data: 1) The Global Entrepreneurship and Development Institute (GEDI) index; 2) The Open Data Barometer (ODB) score; 3) The Worldwide Governance Indicators (WGI) database; 4) The Global Competitiveness (GC) report; 5) The Economic Freedom (EF) index and 6) The Global Innovation (GI) index. Following the results from recent meta-studies on the reuse of data for scientific research, we take advantage of several data sources and combine them in a novel way to address our main research question (Faniel, Kriesberg, and Yakel Citation2016; Pasquetto, Borgman, and Wofford Citation2019).
The combination of these datasets ended-up with a panel data structure, comprising of 90 countries over the period 2013–2016. The restrictive time frame is due to the recent development of the OD Movement and its formalisation in terms of data collection, release and comparison as an indicator at the country-level.
3.2. Measures and methods
3.2.1. Dependent variables and estimation method
As discussed in Section 1, we examine the relationship between OD and entrepreneurship at the country-level. Therefore, we estimate the following econometric model:
where is a series of year dummies;
is a vector of country-specific control variables;
indicates the country-specific unobserved heterogeneity term and
is the usual error term. As we expect the strength of the relationship between open data and entrepreneurship to be affected by the institutional quality of the country, in a second specification we add the interaction between open data and institutional quality (
).Footnote2
We adopt different specifications of our panel data models: pooled cross-sectional approach, random effects, fixed effect, between effects and within/between estimators. First, we assume the unobserved heterogeneity term to be zero and estimate the models as pooled cross-sectional ones, while adjusting for standard errors given the longitudinal dimension of the data. Although a useful starting point, the pooled model fails to check for unobserved time-invariant factors, such as entrepreneurial ability at the country level. For this reason, we apply both standard random- and fixed-effects panel estimators. In a similar vein, we adopt the between estimator suggested by Hauk and Wacziarg (Citation2009), which has been shown to be more robust for measurement errors than other panel data estimators. Finally, we apply a hybrid approach (Schunck Citation2013; Trevis Certo, Withers, and Semadeni Citation2017), which combines the advantages of both within and between estimators. The intuition behind this approach is that each explanatory variable is decomposed into within and between components and the model is run as a random-effects panel estimator. Using this approach allows us to disentangle the effect of OD on entrepreneurship in relation to: i) how it changes between countries (e.g. a group of countries characterised by higher levels of OD publishing are more entrepreneurial than countries belonging to a different group) and ii) within countries over time (e.g. a country can becomes more entrepreneurial by introducing or strengthening open data publishing).
Our dependent variable is the Global Entrepreneurship Index (GEI) developed by the Global Entrepreneurship and Development Institute (GEDI). GEI is an indicator ranging between 0 and 100 which measures the quality and the scale of the entrepreneurial process in more than 120 countries worldwide. It is a measure of entrepreneurial determinants at national-level, based on three sub-indexes labelled as attitudes, abilities, and aspirations (Acs, Autio, and Szerb Citation2014). Overall, these sub-indexes are composed of fourteen pillars.Footnote3 Entrepreneurial attitudes refer to the identification of new opportunities, networking and risk acceptance. Entrepreneurial abilities are related to personal attributes, the capacity to adopt and implement technology and the development of strategies in order to be competitive in the market. Entrepreneurial aspirations refer to the innovation and quality of product development, attraction of risk capital and globalisation. GEI index bears several advantages compared to other country-level indicators of entrepreneurship, such as the Global Entrepreneurship Monitor (GEM). First, GEI index is available for a high number of countries (137 countries compared to 50 countries for GEM). Second, GEI index has often been used in combination with institutional and survey data to analyse: contextual features of entrepreneurship (Acs, Autio, and Szerb Citation2014); the association with economic growth (Acs Citation2010); and the role that governments play in fostering entrepreneurship (Saberi and Hamdan Citation2019). Third, the GEI index has been extensively used to provide policy and managerial recommendations (Szerb, Aidis, and Acs Citation2013; Acs, Autio, and Szerb Citation2014; Komlósi et al. Citation2015). Finally, this is a measure of entrepreneurship which refers to self-employment with high potential, particularly in the digital domain (Acs et al., Citation2017). Notably, the number of new ventures matters less (e.g. low value added services) compared to standard measures of entrepreneurship, while high value-added driven by product and process innovation, internationalisation, and high growth are comparatively more important in the chosen measure. The choice of this measure is also relevant given the expectations about the contribution of open government data publication to digital entrepreneurship, rather than to survival self-employment (Gindling and Newhouse Citation2014).Footnote4
3.2.2. Explanatory variables
We measure OD publication at the country-level by relying on the Open Data Barometer score, developed by the World Wide Web foundation.Footnote5 The Open Data Barometer score comprises three types of data collection: i) a peer reviewed expert survey containing a range of questions about OD contexts, policy, implementation and impacts and a detailed assessment of 15 different data types for each country (data availability, format, licence, timeliness and discoverability); ii) a government self-assessment through a simplified version of the survey above and iii) secondary data complementing primary data collection (for the readiness component only) with information retrieved from official data of the World Economic Forum, World Bank, United Nations e-Government Survey and Freedom House. We preferred this source of data compared to others, such as the Global Open Data Index (Open Knowledge Foundation Citation2019), as this is adopts a methodological approach to data collection that makes country data comparable through time across different survey waves, while other OD indicators have a narrow scope in terms of the publication of national government data and a methodology which has changed several times.Footnote6 The indicator comprises three main components: OD readiness, OD implementation, and OD impact. The component of readiness measures how qualified are government designing and adopting OD initiatives related to government actions, civil rights, business, and entrepreneurship. The implementation component measures not only the level of government data published, but also the degree of accessibility, openness and timeliness. Finally, the impact indicator quantifies whether the data released by governments have a practical benefit to society in economic, social and political terms (Open Data Barometer Citation2017). For our purpose, we rely on the implementation component of the Open Data Barometer score. The implementation component comes from a peer reviewed expert survey with detailed assessment of 15 different data types (see Table A1 in the supplementary material for a full list and description of the datasets). The assessment of each data type is carried out by a given expert assigned to a country. The expert provides a specific assessment based on a list of 10 questions.Footnote7 The adoption of the implementation component avoids problems of measurement error or spurious association between our open data measure and the entrepreneurship index as the readiness and impact components of the Open Data Barometer score are closely related to, for example, the impact of open data on the economy or its role for the creation of new businesses.
On the contrary, the implementation component of the open data index measures the extent to which government data is open, accessible and timely by scoring along these dimensions fifteen different types of datasets, thus reflecting a wide range of government functions. Our main indicator is a single score ranging between 0 and 100 for each country (OD score), where a higher level of the score implies a higher level of publishing of OD in the given country.
To measure institutional quality (Inst Qual) at the country level we follow a consolidated literature and rely on the World Bank’s Worldwide Governance Indicators (WGI) (Kaufmann, Kraay, and Mastruzzi Citation2010). These indicators monitor the process of government selections and transitions, the capacity to develop and implement reliable policies and the strength of institutions through the composition of six pillars: voice and accountability, political stability and absence of violence, government effectiveness, regulatory quality, rule of law, and control of corruption. We focus here on the control of corruption indicator, which captures ‘the extent to which public power is exercised for private gain, including both petty and grand forms of corruption, as well as “capture” of the state by elites and private interests’ (pag 223, Kaufmann, Kraay, and Mastruzzi Citation2011). Conceptually, we expect corruption to be a relevant proxy for institutional quality based on the recent literatures in public economics and public management, which argue for an important role to be played by open government data in favouring transparency, which is directly connected to corruption control (see for example Nguyen and Paczos Citation2020). From an empirical point of view, we decided to focus on a single indicator proxying for institutional quality because all WGI indicators are highly correlated (Glaeser et al. Citation2004; Tebaldi and Elmslie Citation2013), thus pointing to a high degree of overlap with these measures (the correlation coefficients range between 0.7 and 0.9).Footnote8 Furthermore, among the studies adopting WGI indicators to proxy for institutional quality, control of corruption is widely used (Pérez-Villar and Seric Citation2015; Aparicio, Urbano, and Audretsch Citation2016; Chowdhury, Audretsch, and Belitski Citation2019).
3.2.3. Control variables
We control for several factors which can affect the entrepreneurial level of a country. We proxy for the innovation activity of a country by relying on the number of patent applications, as patents are considered by the literature as one important determinant of new firm formation (Somaya and Teece Citation2021; Choi and Phan Citation2006). Innovation is the number of patents filed under the Patent Cooperation Treaty (PCT) procedure per million people. Access to the internet is a measure of the total used capacity of international Internet bandwidth, in kilobytes per second (kb/s) divided by the number of users.Footnote9 We also consider the percentage of corporate taxation in a given country (Corporate tax) as an increase or reduction as it may encourage or inhibit the inclusion or exclusion on entrepreneurial activities (De Mooij and Nicodème Citation2006). Labour market rigidity is an index weighting legal and regulatory frameworks such as minimum wages, legality mandated notice period, obstacles to hiring additional workers or rigidity of hours on a given economy (Fuentelsaz et al. Citation2015). Ease new business and ease credit are two measures related to the regulatory environment that directly affect private entrepreneurial endeavours. The former quantifies the level of bureaucracy in terms of the number of procedures, time, cost and paid-in minimum capital requirement for a small- to medium-size limited liability company to start up and formally operate in a country. The latter measures two main financial components for a company in an economy: access to finance and the effectiveness of collateral and bankruptcy laws in facilitating lending (World Bank Group Citation2019). Finally, we control for two standard measures which have been found to be related to the entrepreneurial level of a country (Russell, Atchison, and Brooks Citation2008): GDP per capita in 2010 PPP (Income) and the percentage of population enrolment in tertiary education (Tertiary edu). provides a description of variables with name, data source and period of reference.
Table 1. Description of variables.
4. Results
4.1. Descriptive results
and report the descriptive statistics and correlations for all the variables used in the analysis. As it is usual in analysis where the unit of observation is the country, in some cases correlation among variables is high. We have conducted an in-depth inspection of such correlations to examine whether multicollinearity represents a significant problem in our dataset. We implemented a full range of diagnostic methods found in the statistical literature. First, we calculated mean variance inflation factors. Variance inflation factors range between 1.29 and 4.87 with a mean variance inflation factor of 2.31, all well below the threshold value of 5 (Menard Citation1995, Pag. 66). Second, condition index for the three specifications outlined above ranges between 1 and 20: all values below the threshold of 30 (Hair et al. Citation1998 220). Third, the Theil R2 multicollinearity effect equals 0.01 which is well below the value indicating multicollinearity, i.e. 1 (Theil Citation1971).
Table 2. Descriptive statistics.
Table 3. Correlation table.
shows a graphical representation of the two-way relationship between the level of OD publication and the entrepreneurial score at the country-level. The upward sloping shape of the line points to a positive relationship between the two measures: countries with a high-level of OD are also countries characterised by a high-level of entrepreneurship activity. Even more interestingly, the scatter diagram in illustrates the extent to which the relationship between OD publication and entrepreneurship changes relative to the level of institutional quality (quartiles of the institutional quality distribution). Although the lines are upward sloping for all four quartiles of institutional quality (low, medium-low, medium-high and high institutional quality), the lines gets steeper for high levels of institutional quality (particularly for the third and fourth quartiles) thus pointing to a greater role of OD for entrepreneurship in countries characterised by high institutional quality. The overall pattern from this first descriptive exercise shows a positive association between OD and entrepreneurship and an even greater role played by OD for countries characterised by high institutional quality.
4.2. Econometric results
We investigate how the extent of OD affects the level of entrepreneurship at the country-level building upon the different estimation models introduced in section 3.2. The main results are reported in . The first four columns present different panel data estimators (pooled, random effects, fixed effects and between effects respectively). Column 5 presents the results of the hybrid approach suggested by Schunck (Citation2013), which combines the advantages of both within and between estimators and contributes to disentangling the two effects. As expected, both income per capita and the level of education affect the level of entrepreneurship at the country level: in fact both Income and Tertiary edu are positive and significant at standard confidence levels (p < 0.01) in four specifications out of five.
Table 4. The relationship between open data and entrepreneurship.
Concerning OD, we observe a positive and significant association between OD and entrepreneurship using ordinary least squares, random effects and between effects estimators. As it was the case for the control variables, the effect is not significant for the fixed effect specification. The above result seems to suggest that the positive relationship between OD and entrepreneurship is mainly due to differences in OD publishing between countries (i.e. a group of countries characterised by higher levels of OD publishing are more entrepreneurial than countries belonging to a different group) rather than within countries over time. This interpretation is confirmed by the results reported in column 5 where, via the hybrid approach, we find that it is indeed the between component of OD that shows a positive and significant association with entrepreneurship (β = 0.824, p < 0.05). Nevertheless, we find a positive (β = 0.396) and weak (p < 0.1) association between the within component of OD and entrepreneurship. The effect is also non-negligible from an economic point of view: an interquartile change in the value of OD leads to an increase of the entrepreneurship score of ten percentile points. The picture emerging from the previous results appears more nuanced when we introduce interaction terms to capture the interplay between OD and country institutional quality in driving entrepreneurship. reports our second specification, which adds to the former model the interaction between OD and institutional quality (OD score X inst qual). A positive sign would mean that the two constructs reciprocally reinforce; a negative sign would point to a substitution effect. Columns 1–5 of report a positive and significant coefficient of Open Data X Inst Qual for all the different estimation strategies implemented. This result indicates a reciprocally reinforcing effect between OD and institutional quality for the level of entrepreneurship of a country. Interestingly, and contrary to the case of the direct effect, this effect is positive and significant (p < 0.05) when country-level fixed effects are included (column 3 of ) thus pointing to an effect that is both between and within countries (column 5 of ). We provide a graphical representation of this result and plot the predicted values of the entrepreneurship score against Open Data for different values of Institutional Quality (respectively 10th, 25th, 50th, 75th and 90th percentiles) in . We do so relying on the fixed effect model (Column 3 of ), but results are not different if other models are used. When all of the other variables are at their mean values, the marginal effect of Open Data is negative at very low levels of institutional quality (10th percentile); however, the marginal effects turn positive and are increasing for higher values of Institutional Quality (from the 25th percentile onward). Overall, this graph provides support for a complementarity between Open Data and Institutional Quality in relation to the entrepreneurial potential of a country.
Figure 3. The effect of open data on entrepreneurship for different percentiles of institutional quality.
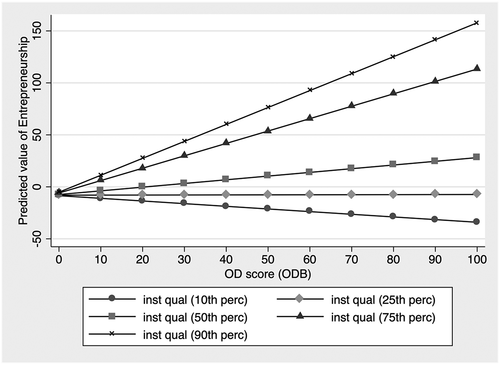
Table 5. The interplay between open data and institutional quality for entrepreneurship.
5. Robustness checks
5.1. Endogeneity
A major concern with the estimated model is that there may be a potential endogeneity problem, i.e. there may be unobserved covariates simultaneously correlated with OD publishing and our measure of entrepreneurship that may be biasing our coefficients. For example, it might be the case that the demand for OD is higher in countries with a more digitally literate population. This would imply a higher ability to start ventures in the era of the digital economy. Under an ideal setting, we would have based our identification strategy following policy changes and/or quasi-natural experiments. Unfortunately, we are unable to distinguish between first and later OD publishers. Major OD regulations were in fact implemented before our period of observation. In addition, OD regulations have never seen a sharp implementation, but rather a slow or diluted adoption through time.Footnote10
We instead take into account endogeneity by instrumenting for our main independent variable (OD score) by resorting to an additional source of data: the World Values Survey which offers a wide range of country-specific cultural data and has been extensively used in cross-cultural and economics of education research.Footnote11 We employ the longitudinal dataset comprising six different survey waves (1981–1984, 1989–1993, 1994–1998, 1999–2004, 2005–2009 and 2010–2014) and compute averages of our variables of interest for the overall period (1981–2014).Footnote12 We exploit this data source and look at the presence of cultural factors which are likely to explain the higher publishing of OD at the country-level but not the level of entrepreneurship, thus providing a credible exclusion restriction for our estimation strategy. More precisely, we define Open Mindedness as a dummy variable taking value 1 if a respondent has indicated at least one of the following options: (i) imagination; (ii) tolerance and respect for other people while answering to the question ‘Here is a list of qualities that children can be encouraged to learn at home. Which, if any, do you consider to be especially important?’. Similarly, we defined the variable Voice as one of the sub-indexes comprising the post-materialist index developed by Welzel (Citation2013).Footnote13 Voice measures the respondents’ priorities for freedom of speech and for people having a voice and a say in how things are done in their society. To measure these priorities, three answers to a question asking which should be country priorities are used: (i) giving people more say in important government decisions; (ii) protecting freedom of speech; (iii) seeing that people have more say about how things are done at their jobs and in their communities. We expect a higher level of open-mindedness and willingness to speak up in a country to influence the likelihood that citizens will ask for a higher government transparency, which in turn can spur government to meet this request via publishing OD. As information on these last variables is not available for all countries, the sample reduces to 203 observations (compared to the 273 originally available).
We adopt a standard two-stage least square IV estimator, although adopting two-step efficient generalised method of moments or limited–information maximum likelihood estimators (Hayashi Citation2000; Baum, Schaffer, and Stillman Citation2007) do not affect our results.Footnote14 We are also careful to run a battery of appropriate statistical tests for our model. First, in the first stages we report various statistics that measure the relevance of the excluded exogenous variables (R2, Adjusted R2, Partial R2 and robust F-statistic). Second, we check whether endogeneity is really an issue in our case by running an endogeneity test robust to heteroscedasticity (Wooldridge Citation1995). Finally, we test the validity of the chosen instrumental variables via a Sargan-Hansen test of overidentifying restrictions.
and present the results for first-stages and final IV estimates respectively. We provide estimates for two just-identified models (columns 1 and 2 of ; columns 1–4 of ) and the over-identified one (column 3 of and columns 5–6 of ).
Table 6. Instrumental variable regressions: first stages.
Table 7. Instrumental variable regressions: the relationship between open data and entrepreneurship.
First of all, it must be noted that our main explanatory variables are affected by a problem of endogeneity. The endogeneity test rejects the null hypothesis of exogenous variables at standard significance levels (columns 1, 3 and 5 of ). Quite reassuringly, the chosen instruments are correlated with the endogenous regressors to a good extent. This is apparent from the results of the first stage equations (). Both Open Mindedness and Voice are positively and significantly related to Open data both separately and together. Overall, the results from the instrumental variable regressions confirm the main results obtained in and . Indeed, columns 1, 3 and 5 in show positive and significant coefficients of the OD score. Similarly, columns 2, 4 and 6 confirm the positive moderating effect of institutional quality in the relationship between open data and entsrepreneurship by showing positive and significant coefficients.
To further control for the robustness of our results to a problem of weak instruments, we implement a Least Absolute Shrinkage and Selection Operator (LASSO) regression approach (Belloni et al. Citation2012). Notably, we use this approach to check whether our chosen instruments (Open mindedness and Voice) appear among a large number of IV candidates (Hain and Jurowetzki Citation2019). We start with a large set of potential instruments (eleven) which also include our two instruments, all generated using questions from the World Values Survey.Footnote15 We implement three different popular approaches to select the instruments: i) post-double selection approach (Belloni, Chernozhukov, and Hansen Citation2014); ii) lasso-double orthogonalisation and iii) post-lasso double orthogonalisation (Chernozhukov, Hansen, and Spindler Citation2015). Results are reported in Table B1 (in the electronic supplementary material). Column 1 shows the results of the first stage regression. Reassuringly, both of our preferred instrumental variables (Open mindedness and Voice) are present in the final list following the LASSO selection procedure. More importantly, the results from the three different LASSO approaches yield comparable results for the effect of OD score on the entrepreneurship index at the country level (Columns 2, 3 and 4).
5.2. Other robustness checks
We conduct a further set of robustness checks pertaining to a number of issues which may affect our estimates.
A first set of robustness checks pertains to a possible measurement error in our key regressor (Open Data score). We test the robustness of our results to alternative definitions of OD. First, we use the Open Data barometer score comprising all the three components (OD readiness, OD implementation and OD impact) and not only OD implementation (as explained in Section 3.2.2). Second, we run our set of estimates using an alternative measure of OD: the Global Open Data Index (GODI). This indicator adopts a different methodology compared to the Open Data Barometer by evaluating the level of open data at the country level by scoring the datasets made available by governments and public institutions. Finally, we consider whether the overlap of OD and institutional quality induces a measurement error in our estimates. Among the others, the Open Data Barometer collects information about citizens and civil society by asking questions such as ‘To what extent is there a robust legal or regulatory framework for protection of personal data in the country?’ and about policies enacted by public administrations (‘To what extent is there a well-defined OD policy and/or strategy in the country?’). Therefore, the OD score partially overlaps with our institutional quality measure, which captures different aspects of the quality of country-level governance. The overlap is considerable, as shown by the pairwise correlation between the two measures in our sample (around 0.8). We control for this potential issue of measurement error by regressing our measure of OD on institutional quality and predicting the residuals. These residuals represent the variance in the OD score that is not explained by the country’s institutional quality. We then use these residuals as the new measure of OD (Open data – residuals). Tables B2, B3 and B4 in the electronic supplementary material report the main results for the three different definitions of OD. Reassuringly, the new measures of OD are still positive and significant and confirm results obtained in and .
In the second set of robustness checks we control whether our estimates are driven by the choice of control of corruption from WGI indicators as the main proxy for institutional quality. First, we rerun our estimates by using all the other WGI indicators of institutional quality. As mentioned in Section 3.2.2, all of the WGI indicators are highly correlated (above 0.9) which means that they are likely to proxy for the same theoretical construct. This descriptive evidence is further corroborated by the results of our robustness check, which confirms our main results.Footnote16 Second, instead of relying on a single indicator we take into consideration all of the six WGI indicators by taking the average as done in previous works (Knack and Keefer Citation1995; Al-Marhubi Citation2005; Bergh, Mirkina, and Nilsson Citation2014). Our results are corroborated by this further robustness check (see Table B5 in the electronic supplementary material). Finally, we rely on a different data source: the Institutional Profile Database (IPD). IPD comprises 127 different variables covering a wide range of country institutional and environmental aspects. We reduced the number of variables by performing a one factor principal components analysis on all variables within each of the four categories in IPD: A) political institutions B) markets for goods and services; C) capital market; D) labour market and social relations. This yields one principal component for each of the four sectors of IPD, which as single item accounts for the largest part of the variance within each category (between 40 and 45%). We then include the predicted scores one at the time (given the high correlation) in our estimates. Unfortunately, due to the availability of the data only for one year (2016), we are able to run only cross-sectional regressions. Interestingly, the only factor confirming our results is political institutions. Results for these further robustness checks are displayed in Tables B6, B7, B8 and B9 of the electronic supplementary material.Footnote17
6. Discussion and outlook
Our paper focused on OD, an asset presented as the raw material for entrepreneurship in the digital age. (Tinholt Citation2013; Magalhaes and Roseira Citation2017; Bonina Citation2013; Hughes-Cromwick and Coronado Citation2019). This has led policy-makers to view OD publication as a way of fuelling entrepreneurship in their digital economies (Huijboom and Van den Broek Citation2011; Cabinet Office Citation2012). This paper presents novel evidence to test this widely held assumption in a more systematic fashion. Based on a comparative and longitudinal analysis at country-level, a first contribution of the paper is to demonstrate an association between OD and entrepreneurship: there is a positive and significant relationship between the extent of publishing of OD and the entrepreneurial level of a country. The positive association between OD and entrepreneurship comes from differences across countries rather than from differences within countries through time, i.e. there are a group of countries which do particularly well in realising the entrepreneurial benefits of OD.
However, our results highlight that mere publishing of OD is not sufficient for entrepreneurial outcomes. A second contribution of this paper is to highlight the important role of country-level institutions for enabling OD-related entrepreneurial activity (Acs, Desai, and Hessels Citation2008; Audretsch, Grilo, and Thurik Citation2007). The results of our study show that within the context of OD, publishing OD is not sufficient for its entrepreneurial exploitation. We show that the quality of country-level institutions, understood as the ‘rules of the game’ regarding the implementation of rules supporting contractual relationships and market exchanges, positively moderate the relationship between published OD and country-level entrepreneurship. The relationship between OD publishing and entrepreneurship is particularly strong in countries with high institutional quality. This stems from an effect which is both across countries and within countries through time. Overall, our results show that unless a country has quality institutions, publishing OD does not positively affect entrepreneurship.
To our knowledge, our work is the first to provide large scale evidence on the positive role of OD at the country-level. For this reason, it is probably too early to draw detailed implications from our results. Nevertheless, we trust that a number of general and specific provisions can be set forward which can inform the current debate on the value and governance of data (Savona Citation2019).
The digital entrepreneurship sector is not expected to automatically exploit the data provided, but benefiting from initiatives such as OD require a wider appreciation of traditional governance dimensions, specifically those regarding the implementation of rules supporting contractual relationships and market exchanges. The results support the importance of broader policy initiatives to develop good governance (Gupta and Abed Citation2002).
Furthermore, the results of our study strongly support the argument that OD is beneficial to entrepreneurship, and given that most OD is currently published by public sector organisations, public policy could facilitate more publishing of OD by private sector organisations. Assuring protection of privacy, policy makers could enforce antitrust measures in the management of personal data to put individuals in control of whether they want to share data for the public good (see e.g. DECODE Citation2019; Savona Citation2019).
Whilst our findings show robust results with a range of robustness checks, they are affected by some limitations. First, the time span of our analysis is shorter than other data sets. This is due to the short longitudinal information available for open data publication. Unfortunately, the Open Data Barometer in its current form ran between 2013 and 2016 only, so it is currently impossible for us to extend the analysis and incorporate a longer period. Second, there are the usual limitations regarding the underlying causal mechanisms in the absence of an experimental design. Also, since empirical indicators of institutional quality are highly correlated, it is difficult to disentangle the exact role of specific sub-components of institutions (Woodruff Citation2006). Finally, future research could look beyond entrepreneurial entry, to growth, in order to examine the impact of OD and institutions on supporting larger firms (Bowen and De Clercq Citation2008). Despite the above-mentioned drawbacks, we would like to stress that our work is one of the first attempts at empirically estimating the correlation between open government data publishing efforts and new firm creation We trust that this is but a first step in the right direction to better gauge the contribution of OD to society at large.
Supplemental Material
Download MS Word (442.7 KB)Acknowledgement
Previous versions of this paper have been presented at the following conferences and seminar series: EMAEE 2019, SPRU - University of Sussex, 3rd-5th June 2019, Falmer, UK; GSSI social science seminar series, L’ Aquila, Italy, 19th May 2020; Seminar series of the department of political science and management, Universitetet i Agder, Norway, 16th September 2020. We are grateful to the discussants and participants at these events. We also thank anonymous reviewers and editors for their helpful suggestions. Francesco Rentocchini is currently an employee of the European Commission. The views expressed are purely his personal views and may not in any circumstances be regarded as stating an official position of the European Commission. The usual caveats apply.
Disclosure statement
No potential conflict of interest was reported by the author(s).
Supplementary material
Supplemental data for this article can be accessed online at https://doi.org/10.1080/13662716.2022.2109455
Notes
1 Earlier exceptions focussed on high tech clusters like Silicon Valley or Route 28 at the regional level (Acs, Autio, and Szerb Citation2014).
2 While our H2 postulates a moderating role of institutions on the relationship between OD and entrepreneurship, one can also postulate that institutions are not only a moderating factor, but first and foremost a direct determinant of OD. We investigate this issue in Section D of the online supplemental material and show the robustness of our results also under an approach via moderation-mediation analysis (Hayes Citation2013). We would like to thank one of the reviewers for pointing this out.
4 To further corroborate this, we computed the correlation between the GEDI entrepreneurship index and a measure proxying for digital self-employment. The correlation is very high (nearly 0.8). We see this as additional descriptive evidence in favour of the interpretation of the GEDI entrepreneurship as a measure of high value-added self-employment. Additional information about this descriptive evidence in provided in the online supplemental material (Section C).
6 In this respect, please refer to the methodological notes of the different open data barometer data collections between 2013 and 2016 (Open Data Barometer Citation2013, Citation2014, Citation2015, Citation2016).
7 For a list of the questions and examples please refer to Tables A2 and A3 in the supplementary material.
8 Our results are broadly confirmed when we consider other WGI indicators. Results are available from the authors upon request.
9 In case of internet access being linked to OD, omitted variable bias affecting the first can result in a bias of our results. Interestingly, the correlation between internet access and OD publication is not excessively high (0.54 as reported in ). Also, rather than focusing on the adoption of OD by the general public (for which internet access would be extremely relevant), our analysis focuses on the publication of open data by government and its use by entrepreneurs. Following the above points, we think that an omitted variable bias problem referring to internet access is very unlikely.
10 For example, in the UK, a mature open data economy, the government set out principles and guidance for the adoption of open data initiatives in public bodies. This has been optional and the adoption of open data has been uneven within different bodies. For example, open data government licencing was developed in 2010, but this didn’t have to be fully adopted. In 2014 an open data strategy was launched, but this was based on principles, rather than statutory obligations to publish data. As such, public bodies have voluntarily decided what to publish, with large variations between different bodies and what open data they provide. Some bodies work closely with entrepreneurs to facilitate the successful use of open data and others do not. Subsequently, the regulation and policies implementation is messy and uneven in one country.
11 For a full list of publications please check http://www.worldvaluessurvey.org.
12 The dataset is freely available at http://www.worldvaluessurvey.org.
13 For details on how the sub-index is constructed please refer to the online appendix of Welzel’s book.
14 Results are available from the authors upon request.
15 Our starting point was to select all variables from the world values survey database which have a high correlation with our OD score variable (above 0.5). After this, we are left with nine variables: 1) Disbelief Component of Secular Values; 2) Post-Materialist index (4-items); 3) Post-Materialist index (12-items); 4) Future changes: More emphasis on technology; 5) Defiance Component of Secular Values; 6) Equality Component of Emancipative Values; 7) Secular values index; 8) Open mindedness and 9) Voice. A detailed definition of items 1–7 in the list can be found in Welzel (Citation2013), Welzel and Christian (Citation2014)), while items 8 and 9 are the two instrumental variables defined above.
16 We do not report the tables in the main manuscript as this would mean to add five more tables to an already excessively long appendix (one for each of the five remaining WGI indicators) and contribute little to the overall narrative of the paper. Results are available from the authors upon request.
17 For ease of presentation the specifications without interaction effects are not reported. Results are similar to those reported in the text and are available from the authors upon request.
References
- Acs, Z. J. 2010. “Entrepreneurship and Economic Development: The Valley of Backwardness.” Annals of Innovation & Entrepreneurship 1 (1): 5641. doi:10.3402/aie.v1i1.5602.
- Acs, Z. J., and D. B. Audretsch. 2005. Entrepreneurship, Innovation, and Technological Change. Foundations and Trends® in Entrepreneurship 1 (4): 149–195. doi:10.1561/0300000004.
- Acs, Z., E. Autio, and L. Szerb. 2014. “National Systems of Entrepreneurship: Measurement Issues and Policy Implications.” Research Policy 43 (3): 476–494. doi:10.1016/j.respol.2013.08.016.
- Acs, Z. J., S. Desai, and J. Hessels. 2008. “Entrepreneurship, Economic Development and Institutions.” Small Business Economics 31 (3): 219–234. doi:10.1007/s11187-008-9135-9.
- Acs, Z. J., E. Stam, D. B. Audretsch, and A. O’Connor. 2017. “The Lineages of the Entrepreneurial Ecosystem Approach.” Small Business Economics 49 (1): 1–10. doi:10.1007/s11187-017-9864-8.
- Acs, Z., L. Szerb, and E. Autio, Eds. 2017. “The Global Entrepreneurship Index.” In Global Entrepreneurship and Development Index 2016, 19–38. Cham: Springer.
- Aghion, P., and P. Howitt. 1992. “A Model of Growth through Creative Destruction.” Econometrica 60 (2): 323–351. doi:10.2307/2951599.
- Al-Marhubi, F. A. 2005. “Openness and Governance: Evidence across Countries.” Oxford Development Studies 33 (3–4): 453–471. doi:10.1080/13600810500199269.
- Aldrich, H. E., and C. M. Fiol. 1994. “Fools Rush In? The Institutional Context of Industry Creation.” Academy of Management Review 19 (4): 645–670. doi:10.5465/amr.1994.9412190214.
- Almirall, E. 2015. “Open Data Is Not Working – How to Fix It?” http://estevealmirall.com/2015/07/26/open-data-is-not-working-how-to-fix-it
- Anokhin, S., and W. S. Schulze. 2009. “Entrepreneurship, Innovation, and Corruption.” Journal of Business Venturing 24 (5): 465–476. doi:10.1016/j.jbusvent.2008.06.001.
- Aparicio, S., D. Urbano, and D. Audretsch. 2016. “Institutional Factors, Opportunity Entrepreneurship and Economic Growth: Panel Data Evidence.” Technological Forecasting and Social Change 102: 45–61. doi:10.1016/j.techfore.2015.04.006.
- Arenius, P., and M. Minniti. 2005. “Perceptual Variables and Nascent Entrepreneurship.” Small Business Economics 24 (3): 233–247. doi:10.1007/s11187-005-1984-x.
- Audretsch, D. B. 2004. “Sustaining Innovation and Growth: Public Policy Support for Entrepreneurship.” Industry and Innovation 11 (3): 167–191. doi:10.1080/1366271042000265366.
- Audretsch, D. B., I. Grilo, and A. R. Thurik. 2007. “Explaining Entrepreneurship and the Role of Policy: A Framework.” In The Handbook of Research on Entrepreneurship Policy, edited by D. B. Audretsch, I. Grilo, and A. R. Thurik, 1–17. Cheltenham: Edward Elgar Publishing.
- Autio, E., M. Kenney, P. Mustard, D. Siegele, and M. Wright. 2014. “Entrepreneurial Innovation: The Importance of Context.” Research Policy 43 (7): 1097–1108. doi:10.1016/j.respol.2014.01.015.
- Bates, J. 2012. “‘This Is What Modern Deregulation Looks Like’: Co-optation and Contestation in the Shaping of the UK’s Open Government Data Initiative.” The Journal of Community Informatics 8 (2). doi:10.15353/joci.v8i2.3038.
- Baum, C. F., M. E. Schaffer, and S. Stillman. 2007. “Enhanced Routines for Instrumental variables/generalized Method of Moments Estimation and Testing.” The Stata Journal 7 (4): 465–506. doi:10.1177/1536867X0800700402.
- Baumol, W. 1990. “Entrepreneurship: Productive, Unproductive, and Destructive.” Journal of Political Economy 98 (5): 893–921. doi:10.1086/261712.
- Baumol, W. 2002. The Free-Market Innovation Machine: Analyzing the Growth Miracle of Capitalism. Princeton: Princeton University Press.
- Belloni, A., D. Chen, V. Chernozhukov, and C. Hansen. 2012. “Sparse Models and Methods for Optimal Instruments with an Application to Eminent Domain.” Econometrica 80 (6): 2369–2429.
- Belloni, A., V. Chernozhukov, and C. Hansen. 2014. “Inference on Treatment Effects after Selection among high-dimensional Controls.” The Review of Economic Studies 81 (2): 608–650. doi:10.1093/restud/rdt044.
- Berends, J., W. Carrara, W. Engbers, and H. Vollers. 2020. In Re-using Open Data. A Study on Companies Transforming Open Data into Economic and Societal Value. Luxembourg: Publications Office of the European Union.
- Bergh, A., I. Mirkina, and T. Nilsson. 2014. “Globalization and Institutional quality—A Panel Data Analysis.” Oxford Development Studies 42 (3): 365–394. doi:10.1080/13600818.2014.884555.
- BIS. 2014. Open Data Strategy 2014-2016. London: BIS.
- Block, J. H., C. O. Fisch, and M. Van Praag. 2017. “The Schumpeterian Entrepreneur: A Review of the Empirical Evidence on the Antecedents, Behaviour and Consequences of Innovative Entrepreneurship.” Industry and Innovation 24 (1): 61–95. doi:10.1080/13662716.2016.1216397.
- Boettke, P. J., and C. J. Coyne. 2003. “Entrepreneurship and Development: Cause or Consequence?” Advances in Austrian Economics 6: 67–87.
- Bogers, M., A. K. Zobel, A. Afuah, E. Almirall, S. Brunswicker, L. Dahlander, L. Frederiksen, et al. 2017. “The Open Innovation Research Landscape: Established Perspectives and Emerging Themes across Different Levels of Analysis.” Industry and Innovation 24 (1): 8–40. doi:10.1080/13662716.2016.1240068.
- Bonina, C. M. 2013. New Business Models and the Value of Open Data: Definitions, Challenges and Opportunities. NEMODE–3K Small Grants Call. http://www.nemode.ac.uk/wp-content/uploads/2013/11/Bonina-Opendata-Report-FINAL.pdf
- Bowen, H. P., and D. De Clercq. 2008. “Institutional Context and the Allocation of Entrepreneurial Effort.” Journal of International Business Studies 39 (4): 747–767. doi:10.1057/palgrave.jibs.8400343.
- Cabinet Office. 2012. Open Data: Unleashing the Potential. London: The Stationery Office.
- Cacciotti, G., J. C. Hayton, J. R. Mitchell, and A. Giazitzoglu. 2016. “A Reconceptualization of Fear of Failure in Entrepreneurship.” Journal of Business Venturing 31 (3): 302–325. doi:10.1016/j.jbusvent.2016.02.002.
- Certo, S. T., M. C. Withers, and M. Semadeni. 2017. “A Tale of Two Effects: Using Longitudinal Data to Compare Within‐and Between‐firm Effects.” Strategic Management Journal 38 (7): 1536–1556. doi:10.1002/smj.2586.
- Chan, C. M. 2013. “From Open Data to Open Innovation Strategies: Creating e-services Using Open Government Data.” System Sciences (HICSS), 2013 46th Hawaii International Conference on System Sciences, Hawaii, 1890–1899.
- Chattapadhyay, S. 2013. “Towards an Expanded and Integrated Open Government Data Agenda for India.” In Proceedings of the 7th International Conference on Theory and Practice of Electronic Governance (pp. 202–205). Seoul: ACM.
- Chen, C. P., and C. Y. Zhang. 2014. “Data-intensive Applications, Challenges, Techniques and Technologies: A Survey on Big Data.” Information Sciences 275: 314–347. doi:10.1016/j.ins.2014.01.015.
- Chernozhukov, V., C. Hansen, and M. Spindler. 2015. “Post-selection and post-regularization Inference in Linear Models with Many Controls and Instruments.” American Economic Review 105 (5): 486–490. doi:10.1257/aer.p20151022.
- Chhabra, M., R. Hassan, and A. Shamim, eds. 2021. Entrepreneurship and Big Data: The Digital Revolution. Florida, US: CRC Press.
- Choi, H., and S. Park. 2018. “Network Variations at the Intersection of National Capability Orientation and Technological Path Dependence – Patent Citation Network Analysis of the Hydrogen Energy and nano-tech Sectors.” Industry and Innovation 25 (8): 8, 809–831. doi:10.1080/13662716.2017.1358605.
- Choi, Y., and P. Phan. 2006. “The Influences of Economic and Technology Policy on the Dynamics of New Firm Formation.” Small Business Economics 26 (5): 493–503. doi:10.1007/s11187-005-5989-2.
- Chowdhury, F., D. B. Audretsch, and M. Belitski. 2019. “Institutions and Entrepreneurship Quality.” Entrepreneurship Theory and Practice 43 (1): 51–81. doi:10.1177/1042258718780431.
- Corrales-Garay, D., M. Ortiz-de-Urbina-Criado, and E. M. Mora-Valentín. 2019. “Knowledge Areas, Themes and Future Research on Open Data: A co-word Analysis.” Government Information Quarterly 36 (1): 77–87. doi:10.1016/j.giq.2018.10.008.
- Dance, G. J. X., M. LaForgia, and N. Confessore. 2018. “As Facebook Raised a Privacy Wall, It Carved an Opening for Tech Giants.” The New York Times. https://www.nytimes.com/2018/12/18/technology/facebook-privacy.html
- Dau, L., and A. Cuervo-Cazurra. 2014. “To Formalize or Not to Formalize: Entrepreneurship and pro-market Institutions.” Journal of Business Venturing 29 (5): 668–686. doi:10.1016/j.jbusvent.2014.05.002.
- David, U., S. Aparicio, and D. B. Audretsch, Eds. 2019. “The Effect of Entrepreneurial Activity on Economic Growth.” In Institutions, Entrepreneurship, and Economic Performance, 85–106. Cham: Springer.
- Davidsson, P. 2006. “Nascent Entrepreneurship: Empirical Studies and Developments.” Foundations and Trends in Entrepreneurship 2 (1): 1–76.
- Davidsson, P. 2015. “Entrepreneurial Opportunities and the Entrepreneurship Nexus: A re-conceptualization.” Journal of Business Venturing 30 (5): 674–695.
- Dawes, S. S., and N. Helbig (2010, August). Information Strategies for Open Government: Challenges and Prospects for Deriving Public Value from Government Transparency. In International Conference on Electronic Government (pp. 50–60). Springer, Berlin, Heidelberg.
- De Mooij, R. A., and G. Nicodème. 2006. “Corporate Tax Policy, Entrepreneurship and Incorporation in the EU.” https://papers.ssrn.com/abstract=956276
- DECODE. 2019. https://decodeproject.eu/publications/impact-and-economic-sustainability-decode-ecosystem-and-future-development
- Dodgson, M., D. Gann, and A. Salter. 2006. “The Role of Technology in the Shift Towards Open Innovation: The Case of Procter & Gamble.” R&D Management 36 (3): 333–346. doi:10.1111/j.1467-9310.2006.00429.x.
- Dos Santos Brito, K., M. A. da Silva Costa, V. C. Garcia, and S. R. de Lemos Meira. 2014. “Brazilian Government Open Data: Implementation, Challenges, and Potential Opportunities.” In Proceedings of the 15th Annual International Conference on Digital Government Research (pp. 11–16). ACM, Aguascalientes Mexico.
- Dutta, N., and R. Sobel. 2016. “Does Corruption Ever Help Entrepreneurship?” Small Business Economics 47 (1): 179–199. doi:10.1007/s11187-016-9728-7.
- Eftekhari, N., and M. Bogers. 2015. “Open for Entrepreneurship: How Open Innovation Can Foster New Venture Creation.” Creativity and Innovation Management 24 (4): 574–584. doi:10.1111/caim.12136.
- Estermann, B. 2014. “Diffusion of Open Data and Crowdsourcing among Heritage Institutions: Results of a Pilot Survey in Switzerland.” Journal of Theoretical and Applied Electronic Commerce Research 9 (3): 15–31. doi:10.4067/S0718-18762014000300003.
- Estrin, S., J. Korosteleva, and T. Mickiewicz. 2013. “Which Institutions Encourage Entrepreneurial Growth Aspirations?” Journal of Business Venturing 23 (4): 564–580. doi:10.1016/j.jbusvent.2012.05.001.
- Faniel, I. M., A. Kriesberg, and E. Yakel. 2016. “Social Scientists’ Satisfaction with Data Reuse.” Journal of the Association for Information Science and Technology 67 (6): 1404–1416. doi:10.1002/asi.23480.
- Fuentelsaz, L., C. González, J. P. Maícas, and J. Montero. 2015. “How Different Formal Institutions Affect Opportunity and Necessity Entrepreneurship.” BRQ Business Research Quarterly 18 (4): 246–258. doi:10.1016/j.brq.2015.02.001.
- Gershman, M., V. Roud, and T. Wolfgang. 2019. “Open Innovation in Russian state-owned Enterprises.” Industry and Innovation 26 (2): 199–217. doi:10.1080/13662716.2018.1496815.
- Gindling, T. H., and D. Newhouse. 2014. “Self-employment in the Developing World.” World Development 56: 313–331. doi:10.1016/j.worlddev.2013.03.003.
- Gittelman, M. 2006. “National Institutions, public–private Knowledge Flows, and Innovation Performance: A Comparative Study of the Biotechnology Industry in the US and France.” Research Policy 35 (7): 1052–1068. doi:10.1016/j.respol.2006.05.005.
- Glaeser, E. L., R. La Porta, F. Lopez-de-Silanes, and A. Shleifer. 2004. “Do Institutions Cause Growth?” Journal of Economic Growth 9 (3): 271–303. doi:10.1023/B:JOEG.0000038933.16398.ed.
- Gupta, M. S., and M. G. T. Abed. 2002. Governance, Corruption, and Economic Performance. Washington DC: International Monetary Fund.
- Hain, D. S., and R. Jurowetzki. 2019. “The Potentials of Machine Learning and Big Data in Entrepreneurship research–the Liaison of Econometrics and Data Science.” In Handbook of Quantitative Research Methods in Entrepreneurship, edited by George Saridakis, and Marc Cowling, 176–220. Cheltenham, United Kingdom: Edward Elgar Publishing.
- Hair, J. F.,sJr., R. L. Tatham, R. E. Anderson, and W. Black. 1998. Multivariate Data Analysis. 5th ed. Englewood Cliffs, NJ: Prentice-Hall.
- Hauk, W., and R. Wacziarg. 2009. “A Monte Carlo Study of Growth Regressions.” Journal of Economic Growth 14 (2): 103–147. doi:10.1007/s10887-009-9040-3.
- Hayashi, F. 2000. Econometrics. Princeton: Princeton University Press.
- Hayes, A. F. 2013. Introduction to Mediation, Moderation, and Conditional Process Analysis: A Regression-Based Approach. New York, NY: Guilford Press.
- Heimstädt, M., F. Saunderson, and T. Heath. 2014. “From Toddler to Teen: Growth of an Open Data Ecosystem.” JeDEM-eJournal of eDemocracy and Open Government 6 (2): 123–135. doi:10.29379/jedem.v6i2.330.
- Hey, T., and A. E. Trefethen. 2005. “Cyberinfrastructure for e-science.” Science 308 (5723): 817–821. doi:10.1126/science.1110410.
- Huber, F. 2013. “Knowledge-sourcing of R&D Workers in Different Job Positions: Contextualising External Personal Knowledge Networks.” Research Policy 42 (1): 167–179. doi:10.1016/j.respol.2012.05.010.
- Huber, F., T. Wainwright, and F. Rentocchini. 2020. “Open Data for Open Innovation: Managing Absorptive Capacity in SMEs.” R&D Management 50 (1): 31–46. doi:10.1111/radm.12347.
- Hughes-Cromwick, E., and J. Coronado. 2019. “The Value of US Government Data to US Business Decisions.” Journal of Economic Perspectives 33 (1): 131–146. doi:10.1257/jep.33.1.131.
- Huijboom, N., and T. Van den Broek. 2011. “Open Data: An International Comparison of Strategies.” European Journal of ePractice 12 (1): 4–16.
- Janssen, M., Y. Charalabidis, and A. Zuiderwijk. 2012. “Benefits, Adoption Barriers and Myths of Open Data and Open Government.” Information Systems Management 29 (4): 258–268. doi:10.1080/10580530.2012.716740.
- Jones, C., and C. Tonetti. 2020. “Nonrivalry and the Economics of Data.” American Economic Review 110 (9): 2819–2858. doi:10.1257/aer.20191330.
- Juell-Skielse, G., A. Hjalmarsson, E. Juell-Skielse, P. Johannesson, and D. Rudmark. 2014. “Contests as Innovation Intermediaries in Open Data Markets.” Information Polity 19 (3–4): 247–262. doi:10.3233/IP-140346.
- Kaufmann, D., A. Kraay, and M. Mastruzzi. 2010. “The Worldwide Governance Indicators: Methodology and Analytical Issues.” World Bank Policy Research Working Paper No. 5430.
- Kaufmann, D., A. Kraay, and M. Mastruzzi. 2011. “The Worldwide Governance Indicators: Methodology and Analytical Issues.” Hague Journal on the Rule of Law 3 (2): 220–246. doi:10.1017/S1876404511200046.
- Kitsios, F., M. Kamariotou, and E. Grigoroudis. 2021. “Digital Entrepreneurship Services Evolution: Analysis of Quadruple and Quintuple Helix Innovation Models for Open Data Ecosystems.” Sustainability 13 (21): 12183. doi:10.3390/su132112183.
- Knack, S., and P. Keefer. 1995. “Institutions and Economic Performance: Cross‐country Tests Using Alternative Institutional Measures.” Economics & Politics 7 (3): 207–227. doi:10.1111/j.1468-0343.1995.tb00111.x.
- Komlósi, É., L. Szerb, Z. J. Ács, and R. Ortega-Argilés. 2015. “Quality-related Regional Differences in Entrepreneurship Based on the GEDI Methodology: The Case of Hungary.” Acta Oeconomica 65 (3): 455–477. doi:10.1556/032.65.2015.3.6.
- Kraus, S., C. Palmer, N. Kailer, F. L. Kallinger, and J. Spitzer. 2019. “Digital Entrepreneurship: A Research Agenda on New Business Models for the twenty-first Century.” International Journal of Entrepreneurial Behavior & Research 25 (2): 353–375.
- Lakomaa, E., and J. Kallberg. 2013. “Open Data as a Foundation for Innovation: The Enabling Effect of Free Public Sector Information for Entrepreneurs.” IEEE Access 1: 558–563. doi:10.1109/ACCESS.2013.2279164.
- Le Dinh, T., M. C. Vu, and A. Ayayi. 2018. “Towards a Living Lab for Promoting the Digital Entrepreneurship Process.” International Journal of Entrepreneurship 22 (1): 1–17.
- Lee, M. J., E. Almirall, and J. D. Wareham. 2014. “Open Data & Civic Apps: 1st Generation Failures – 2nd Generation Improvements.” ESADE Business School Research Paper, No. 256. https://ssrn.com/abstract=2508358
- Lindman, J., and L. Nyman. 2014. “The Businesses of Open Data and Open Source: Some Key Similarities and Differences.” Technology Innovation Management Review 4 (1): 12–17. doi:10.22215/timreview/757.
- Lundvall, B.-A. 2007. “National Innovation Systems – Analytical Concept and Development Tool.” Industry and Innovation 14 (1): 1, 95–119. doi:10.1080/13662710601130863.
- Magalhaes, G., and C. Roseira. 2017. “Open Government Data and the Private Sector: An Empirical View on Business Models and Value Creation.” Government Information Quarterly. doi:10.1016/j.giq.2017.08.004.
- Matsakis, L. 2019. The Wired Guide To Your Personal Data (And Who Is Using It). San Francisco, US: Wired. https://www.wired.com/story/wired-guide-personal-data-collection
- Mc Dermott, P. 2010. “Building Open Government.” Government Information Quarterly 27: 401–413. doi:10.1016/j.giq.2010.07.002.
- Menard, S. 1995. Applied Logistic Regression Analysis: Sage University Series on Quantitative Applications in the Social Sciences. Thousand Oaks, CA: Sage.
- Nagaraj, A. 2020. “The Private Impact of Public Maps: Landsat Satellite Maps Increased Gold Discoveries and Encouraged Entry.” https://abhishekn.com/files/nagaraj_landsat_2020.pdf
- Nguyen, D., and M. Paczos. 2020. “Measuring the Economic Value of Data and cross-border Data Flows: A Business Perspective.” OECD Digital Economy Papers, No. 297, Paris: OECD Publishing.
- North, D. 1990. Institutions, Institutional Change and Economic Performance. Cambridge: Cambridge University Press.
- Obschonka, M., and D. B. Audretsch. 2020. “Artificial Intelligence and Big Data in Entrepreneurship: A New Era Has Begun.” Small Business Economics 55 (3): 529–539. doi:10.1007/s11187-019-00202-4.
- Open Data Barometer. 2013. “Open Data Barometer: 2013 Researchers Handbook.” June 2013. http://opendatabarometer.org/doc/1stEdition/OpenDataBarometer-ResearchHandbookDownloadVersion.pdf
- Open Data Barometer. 2014. Open Data Barometer. 2nd ed. Geneva, Switzerland: ODB Methodology.
- Open Data Barometer. 2015. “Open Data Barometer 3rd Edition ODB Methodology - v1.0.” Accessed April 28 2015. https://opendatabarometer.org/doc/3rdEdition/ODB-3rdEdition-Methodology.pdf
- Open Data Barometer. 2016. “Open Data Barometer 4th Edition ODB Methodology - v1.0.” Accessed April 28 2016. https://docs.google.com/document/d/1_6FR6nZ6Uj4xr2tMsnhGBjocbxOd4MRcBsXe2OMh1CE/edit#
- Open Data Barometer. 2017. Global Report. 4th ed. World wide web foundation, Geneva, Switzerland. https://opendatabarometer.org/doc/4thEdition/ODB-4thEdition-GlobalReport.pdf
- Open Data Institute. 2015. Open Data Means Business: UK Innovation across Sectors and Regions. London, UK.
- Open Data Institute. 2019. “Open Data Skills Framework.” https://theodi.org/article/open-data-skills-framework
- Open Knowledge Foundation. 2019. “Global Open Data Index Methodology” Accessed July 31 2019. https://index.okfn.org/methodology
- Parkins, D. 2017. “The World’s Most Valuable Resource Is No Longer Oil, but Data.” The Economist. https://www.economist.com/leaders/2017/05/06/the-worlds-most-valuable-resource-is-no-longer-oil-but-data
- Pasquetto, I. V., C. L. Borgman, and M. F. Wofford. 2019. “Uses and Reuses of Scientific Data: The Data Creators’ Advantage.” Harvard Data Science Review 1 (2): 1–34. doi:10.1162/99608f92.fc14bf2d.
- Pérez-Villar, L., and A. Seric. 2015. “Multinationals in Sub-Saharan Africa: Domestic Linkages and Institutional Distance.” International Economics 142: 94–117. doi:10.1016/j.inteco.2014.06.001.
- Russell, R., M. Atchison, and R. Brooks. 2008. “Business Plan Competitions in Tertiary Institutions: Encouraging Entrepreneurship Education.” Journal of Higher Education Policy and Management 30 (2): 123–138. doi:10.1080/13600800801938739.
- Saberi, M., and A. Hamdan. 2019. “The Moderating Role of Governmental Support in the Relationship between Entrepreneurship and Economic Growth: A Study on the GCC Countries.” Journal of Entrepreneurship in Emerging Economies 11 (2): 200–216.
- Sahut, J. M., L. Iandoli, and F. Teulon. 2021. “The Age of Digital Entrepreneurship.” Small Business Economics 56 (3): 1159–1169. doi:10.1007/s11187-019-00260-8.
- Savona, M. 2019. The Value of Data: Towards a Framework to Redistribute It. Falmer, UK: SPRU-Science Policy Research Unit, University of Sussex Business School. No. 2019-21.
- Schumpeter, J. 1929. Review of Economic Theory. NY: Oxford University Press.
- Schunck, R. 2013. “Within and between Estimates in random-effects Models: Advantages and Drawbacks of Correlated Random Effects and Hybrid Models.” Stata Journal 13 (1): 65–76. doi:10.1177/1536867X1301300105.
- Shane, S., and S. Venkataraman. 2000. “The Promise of Entrepreneurship as a Field of Research.” Academy of Management Review 25 (1): 217–226.
- Sobel, R. 2008. “Testing Baumol: Institutional Quality and the Productivity of Entrepreneurship.” Journal of Business Venturing 23 (6): 641–655. doi:10.1016/j.jbusvent.2008.01.004.
- Somaya, D., and D. Teece. 2021. Patents, licensing, and entrepreneurship: Effectuating innovation in multi-invention contexts. In edited by E. Sheshinski, R. J. Strom, and W. J. Baumol. Entrepreneurship, innovation, and the growth mechanism of the free-enterprise economies, US.185–212. Princeton: Princeton University Press.
- Sorensen, J. 2007. “Bureaucracy and Entrepreneurship: Workplace Effects on Entrepreneurial Entry.” Administrative Science Quarterly 52 (3): 387–412. doi:10.2189/asqu.52.3.387.
- Szerb, L., R. Aidis, and Z. J. Acs. 2013. “The Comparison of the Global Entrepreneurship Monitor and the Global Entrepreneurship and Development Index Methodologies.” Foundations and Trends® in Entrepreneurship 9 (1): 1–142. doi:10.1561/0300000046.
- Tebaldi, E., and B. Elmslie. 2013. “Does Institutional Quality Impact Innovation? Evidence from cross-country Patent Grant Data.” Applied Economics 45 (7): 887–900. doi:10.1080/00036846.2011.613777.
- Theil, H. 1971. Principles of Econometrics. New York: John Wiley.
- Tinholt, D. 2013. The Open Data Economy: Unlocking Economic Value by Opening Government and Public Data. Capgemini Consulting.
- Welle Donker, F., and B. van Loenen. 2017. “How to Assess the Success of the Open Data Ecosystem?” International Journal of Digital Earth 10 (3): 284–306. doi:10.1080/17538947.2016.1224938.
- Welter, F. 2011. “Contextualizing Entrepreneurship – Conceptual Challenges and Ways Forward.” Entrepreneurship Theory & Practice 35 (1): 165–184. doi:10.1111/j.1540-6520.2010.00427.x.
- Welzel, C. 2013. Freedom Rising. Cambridge University Press.
- Welzel, C., and Christian. 2014. “Description of Welzel Data for QoG and WVS 1 T 6 Key Aggregates.” doi:10.13140/2.1.2217.3769.
- Wennberg, K., S. Pathak, and E. Autio. 2013. “How Culture Moulds the Effects of self-efficacy and Fear of Failure on Entrepreneurship.” Entrepreneurship & Regional Development 25 (9–10): 756–780. doi:10.1080/08985626.2013.862975.
- Whelan, E., R. Teigland, B. Donnellan, and W. Golden. 2010. “How Internet Technologies Impact Information Flows in R&D: Reconsidering the Technological Gatekeeper.” R&D Management 40 (4): 400–413. doi:10.1111/j.1467-9310.2010.00610.x.
- Wolff, J. A., and T. L. Pett. 2006. “Small‐firm Performance: Modeling the Role of Product and Process Improvements.” Journal of Small Business Management 44 (2): 268–284. doi:10.1111/j.1540-627X.2006.00167.x.
- Wood, D., Ed. 2010. Linking Enterprise Data. Berlin, Germany: Springer Science & Business Media.
- Woodruff, C. 2006. “Measuring Institutions.” International Handbook on the Economics of Corruption 1: 105–127.
- Wooldridge, J. 1995. “Score Diagnostics for Linear Models Estimated by Two Stage Least Squares.” In Advances in Econometrics and Quantitative Economics: Essays in Honor of Professor C, edited by R. Rao, G. S. Maddala, P. C. B. Phillips, and T. N. Srinivasan, 66–87. Oxford: Blackwell.
- World Bank Group. 2019. Doing Business 2019. 16th ed. Washington DC, US: World Bank Group. http://www.doingbusiness.org/en/reports/global-reports/doing-business-2019
- World Bank Group. 2021. The Huge Potential of Open Data for Business Applications. Washington DC, US: World Bank Group. https://elibrary.worldbank.org/doi/full/10.1596/978-1-4648-1600-0_Spotlight3_1
- Zahra, S., and M. Wright. 2011. “Entrepreneurship’s Next Act.” Academy of Management Perspectives 25 (4): 67–83. doi:10.5465/amp.2010.0149.
- Zuiderwijk, A., M. Janssen, and C. Davis. 2014. “Innovation with Open Data: Essential Elements of Open Data Ecosystems.” Information Polity 19 (1–2): 17–33.
- Zurada, J., and W. Karwowski. 2011. “Knowledge Discovery Through Experiential Learning from Business and Other Contemporary Data Sources: A Review and Reappraisal.” Information Systems Management 28 (3): 258–274.