Abstract
Context
Keguan-1 (KG-1) plays a vital role in enhancing the curative effects, improving quality of life, and reducing the development of acute lung injury (ALI).
Objective
To unravel the protective effect and underlying mechanism of KG-1 against ALI.
Materials and methods
C57BL/6J mice were intratracheally instilled with lipopolysaccharide to establish the ALI model. Then, mice in the KG-1 group received a dose of 5.04 g/kg for 12 h. The levels of proinflammatory cytokines, chemokines, and pathological characteristics were determined to explore the effects of KG-1. Next, untargeted metabolomics was used to identify the differential metabolites and involved pathways for KG-1 anti-ALI. Network pharmacology was carried out to predict the putative active components and drug targets of KG-1 anti-ALI.
Results
KG-1 significantly improved the levels of TNF-α (from 2295.92 ± 529.87 pg/mL to 1167.64 ± 318.91 pg/mL), IL-6 (from 4688.80 ± 481.68 pg/mL to 3604.43 ± 382.00 pg/mL), CXCL1 (from 4361.76 ± 505.73 pg/mL to 2981.04 ± 526.18 pg/mL), CXCL2 (from 5034.09 ± 809.28 pg/mL to 2980.30 ± 747.63 pg/mL), and impaired lung histological damage. Untargeted metabolomics revealed that KG-1 significantly regulated 12 different metabolites, which mainly related to lipid, amino acid, and vitamin metabolism. Network pharmacology showed that KG-1 exhibited anti-ALI effects through 17 potentially active components acting on seven putative drug targets to regulate four metabolites.
Discussion and conclusions
This work elucidated the therapeutic effect and underlying mechanism by which KG-1 protects against ALI from the view of the metabolome, thus providing a scientific basis for the usage of KG-1.
Introduction
Since the initial outbreak of coronavirus disease 2019 (COVID-19), the propagation has posed a serious threat to humans. The 2019 novel coronavirus is responsible for COVID-19 (Cohen and Normile Citation2020; Zhu et al. Citation2020), leading to a series of pulmonary infections and systemic infections. Its clinical features include fever, cough, dyspnoea, and respiratory symptoms. Some patients can develop rapidly into severe pneumonia, acute lung injury/acute respiratory distress syndrome (ALI/ARDS), multiorgan failure, and even death (Channappanavar and Perlman Citation2017; Huang et al. Citation2020). Among these features, ALI is a common critical disease with very high mortality, seriously affecting the quality of life in COVID-19 patients (Young et al. Citation2013), and is also an important prognostic factor for COVID-19 patients (Ramanathan et al. Citation2020). ALI is an acute diffuse lung injury mainly caused by an inflammatory cytokine storm, which plays a central role in the pathophysiology during the evolvement of ALI (Channappanavar and Perlman Citation2017). It is characterised by initial inflammatory cell infiltration and neutrophil aggregation in the lung, which exhibits destruction of the alveolar structure, and eventually causes diffuse lung injury (Weinacker and Vaszar Citation2001). In addition to mechanical ventilation, liquid management, extracorporeal membrane oxygenation, and a series of supportive measures, effective drugs are still lacking.
The COVID-19 outbreak is an enormous challenge to seek effective therapeutic remedies. Traditional Chinese medicine (TCM) has played a key role in treating and preventing abrupt contagions, including severe acute respiratory syndrome coronavirus, H1N1, H7N9, and Ebola virus, and has achieved remarkable clinical curative effects. On this basis, our research team (Niu et al. Citation2020) screened 46 active ingredients from candidate TCMs that could act on the novel coronavirus S-protein-binding site of the human angiotensin-converting enzyme 2 protein and formed a new anti-coronavirus hospital prescription, “Keguan-1” (KG-1). It is composed of 7 herbal medicines, containing Forsythia suspensa (Thunb.) Vahl (Oleaceae) (Lianqiao in Chinese), Lonicera japonica Thunb. (Caprifoliaceae) (Jinyinhua in Chinese), Morus alba L. (Moraceae) (Sangye in Chinese), Chrysanthemum morifolium Ramat. (Asteraceae) (Juhua in Chinese), Coix lacryma-jobi L. var. mayuen (Roman.) Stapf (Poaceae) (Yiyiren in Chinese), Fritillaria thunbergii Miq. (Liliaceae) (Zhebeimu in Chinese), and Prunus armeniaca L. var. ansu Maxim (Rosaceae) (Kuxingren in Chinese). Further clinical trials using KG-1 granules are warranted to significantly improve the time to fever resolution and reduce the evolvement of ALI with COVID-19 patients (Wang et al. Citation2020). Nevertheless, the study of the mechanism underlying KG-1 treatment still requires many experiments.
Metabolomics is a branch of systematic biology to improve our understanding of both normal physiology and the pathways of many diseases based on the recognition of small molecular metabolites (Wishart Citation2019). Recently, untargeted metabolomics was applied to explore the molecular mechanisms and scientific connotations by which TCM compound preparations treat diseases, such as Xihuang pill (Li et al. Citation2020), Sijunzi decoction (Li et al. Citation2019), and Sancao granule (Yang et al. Citation2019). These studies suggest the power of untargeted metabolomics in the development and application of TCM. In addition, network pharmacology is a system biology method for rapid prediction of active drugs and potential targets, and its multi-component multi-target is consistent with the "multi-component-multi-target" effect of TCM (Li and Zhang Citation2013). Therefore, the combination of metabolomics and network pharmacology can quickly reveal the relationship among TCM, metabolites and targets, and explain the mechanism of regulating diseases with TCM.
Here, we used the combination strategy of animal experiments, untargeted metabolomics and network pharmacology to elucidate the regulatory effect of KG-1 against ALI. Firstly, the effect of KG-1 against ALI was evaluated in LPS induced ALI mice. Secondly, untargeted metabolomics was used to identify the potential metabolites in the treatment of ALI with KG-1. Thirdly, network pharmacology was carried out to predict and screen the potential effective components and targets related to KG-1 against ALI. Finally, by using untargeted metabolomics and network pharmacology, we conducted the interaction network of differentially expressed metabolites, putative targets and potential effective constituents related to KG-1. This strategy can provide novel insight into the mechanism of KG-1 in ALI.
Materials and methods
Animals, drugs and reagents
Male C57BL/6J mice (SPF grade, 7–8 weeks, 20 ± 2 g) were supplied by SPF (Beijing) Biotechnology Co., Ltd. (License No. SYXK 2019-0010). The animals were adaptively fed for 1 week in a pathogen-free environment, with the temperature of 25 °C ± 0.5 °C, the humidity of 55% ± 5%, and a 12 h dark/light cycle. This research scheme was approved by the Ethics Committee of the Fifth Medical Centre of Chinese PLA. Powder versions of the 7 herbal medicines of KG-1 were provided by the Chinese Medicine Pharmacy of the hospital. Dexamethasone was purchased from Harvey-bio-Co. Ltd. (DXMS, Lot. 19JULI519, Beijing, China). LPS was purchased from Sigma-Aldrich (Lot.057M4013V, St. Louis, MO, USA). Ethyl carbamate was purchased from Sinopharm Chemical Reagent Co. Ltd. (Lot. 20190419, Shanghai, China). Methanol and acetonitrile were purchased from Fisher Chemical (Lot. 178498, Lot. 197148, Pittsburgh, PA, USA). Normal saline was purchased from Shijiazhuang No. 4 Pharmaceutical Co., Ltd. (Lot. 2001173203, Shijiazhuang, Hebei, China). Dimethyl sulfoxide was purchased from Beijing Solarbio Science & Technology Co., Ltd. (DMSO, Lot. 1209M032, Beijing, China). Phosphate buffered saline was purchased from MACGENE (Beijing) Biotechnology Co., Ltd. (PBS, Lot. J2209200, Beijing, China). The mouse tumour necrosis factor-alpha (TNF-α) and interleukin-6 (IL-6) assay kits were obtained from Beijing DAKEWE Biotechnology Co., Ltd. (Lot. 2011-1, Lot. 2008-2, Beijing, China). The mouse c-x-c motif ligand 1 (CXCL1) assay kit and c-x-c motif ligand 2 (CXCL2) assay kit were purchased from Multi Sciences (Lianke) Biotech Co., Ltd. (Lot. A29600535, Lot. A214200634, Hangzhou, Zhejiang, China).
Study design and sample collection
LPS was administered to mice by intratracheal instillation at a concentration of 0.8 mg/mL for ALI (Dagvadorj et al. Citation2015). DXMS was prepared by DMSO and then diluted with normal saline. A total of 32 mice were divided into four groups, including the control group, model group, KG-1 group, and DXMS group. The control group and other groups were anaesthetized and then administered PBS and LPS in a volume of 50 μL. Two hours later, the mice were intraperitoneally injected with DXMS at 2 mg/kg and intragastrically administered a dosage of KG-1 at 5.04 g/kg [equivalent to 2 times the clinical equivalent dose (0.27 g/kg)]. KG-1 was ground and suspended in distilled water containing 0.5% sodium carboxymethyl cellulose for administration to mice. After 12 h treatment, mice were sacrificed. The bronchoalveolar lavage fluid concentrations of inflammatory cytokines and plasma levels of metabolites were identified. Lung samples were fixed and preserved in 10% neutral formalin solution, dehydrated in ethanol and xylene, embedded in paraffin, cut into 5 μm sections, and then stained with haematoxylin and eosin (H&E).
Serum samples preparation and LC-MS conditions
The thawed samples were processed according to our previous study (Zhang et al. Citation2020). Meanwhile, quality control samples (QC) are prepared by mixing 10 µL of each sample. An Agilent ZORBAX 300 SB-C18 (2.1 mm i.d. × 100 mm, 1.8 µm i.d.) was utilised, and its temperature was kept at 30 °C. The mobile phase composed of acetonitrile (containing 0.1% formic acid, A) and water (containing 1% formic acid and 5% acetonitrile, B), with gradient elution as follows: 0–1.0 min, 95% (B); 1.0–9.0 min, 95%–60% (B); 9.0–19.0 min, 60%–10% (B); 19.0–21.0 min, 10%–0% (B); 21.0–25.0 min, 100% (A). Flow rate: 0.30 mL/min; sample injection volume: 4 μL.
Meanwhile, the measurement parameters under both ESI + and ESI- modes were set as follows: electron spray ionisation (ESI), full scan range (50–1200 m/z), capillary voltage of 2500 V (ESI+) and 2200 V (ESI-), cone voltage of 40 V, ion source temperature of 130 °C, desolvation temperature of 350 °C, cone gas flow rate of 50 L/h, desolvation gas flow rate of 800 L/h. The leu-enkephalin and sodium formate solutions were calibrated to the mass number and mass axis, respectively.
Metabolomic analysis
Mass Hunter Profinder software was used to pre-process all raw data, which were then filtered and normalised in MetaboAnalyst 4.0 (https://www.metaboanalyst.ca/). Principal component analysis (PCA) and orthogonal projection to latent structures discrimination analysis (OPLS-DA) were performed in SIMCA-P software (version 14.1, Umetrics AB, Umea, Sweden). Then, we identified the metabolites according to the Mass Hunter PCDL Manager database. MetaboAnalyst software was applied to obtain the fold change (FC) and p value. The variable importance in the projection (VIP) and p (correlation coefficient) (p(corr)) were acquired from SIMCA-P software. We next narrowed down metabolites to potential biomarkers by VIP, p(corr), FC, and p value. Furthermore, the potential biomarkers were imported into Kyoto Encyclopaedia of Genes and Genomes (KEGG; https://www.kegg.jp/) for pathway enrichment analysis.
Network pharmacology analysis
The chemical components of the 7 kinds of herbal medicines in KG-1 and their related targets were obtained from the Traditional Chinese Medicine Systems Pharmacology Database and Analysis Platform (TCMSP, http://lsp.nwu.edu.cn/tcmsp.php). Through oral bioavailability ≥30% and drug-likeness ≥0.18 to find the putative effective constituents for KG-1 anti-ALI. Then, we used MBRole 2.0 database (http://csbg.cnb.csic.es/mbrole2/) to predict the protein targets of candidate biomarkers. The aforementioned targets were converted to UniProt id format. Finally, a “compound – target – metabolite” interaction network visualisation was analysed with Cytoscape software (version 3.8.0; http://cytoscape.org/).
Statistical analysis
All data are expressed as the mean ± standard error of the mean (SEM). Comparisons among the groups were made with one-way analysis of variance test using the SPSS software program (version 25.0; SPSS Inc., Chicago, IL, USA). The p value less than 0.05 was set as a significance level.
Results
Inhibitory effects of KG-1 on LPS-induced ALI
TNF-α and IL-6 are important mediators of the pathophysiological evolution of ALI (Zhang et al. Citation2013; Tang et al. Citation2014). Inhibition of proinflammatory cytokines can effectively reduce the pathological changes in ALI. As shown in , KG-1 at 5.04 g/kg significantly inhibited TNF-α and IL-6 concentrations (). In addition, platelet and neutrophil chemokines contribute to the pathogenesis of ALI (Bhatia et al. Citation2012; Bdeir et al. Citation2017). In this study, we found that KG-1 at 5.04 g/kg could also significantly reduce the CXCL1 and CXCL2 levels (). Lung histopathology showed that the model group exhibited alveolar structure damage and inflammatory cells infiltration (). KG-1 and DXMS treatment significantly impaired the levels of alveolar structure damage and inflammatory cells infiltration. These findings suggest that KG-1 could considerably reduce the development of ALI.
Figure 1. Effects of KG-1 on TNF-α (A), IL-6 (B), CXCL1 (C), CXCL2 (D), and lung histopathological changes (E) in ALI mice. Blue, red, green, violet columns represent the control, model, KG-1, and DXMS group, respectively. **p < 0.01 versus the control group, #p < 0.05, ##p < 0.01 versus the model group. Data are presented as the mean ± SEM (n = 8).
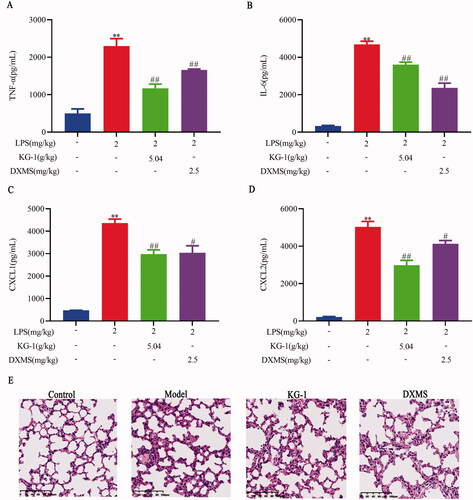
Metabolomics analysis
All QC samples could aggregate under ESI + and ESI- modes, indicating that the analytical system was stable (). Meanwhile, we also observed that there was obvious separation among the control, KG-1 and model groups in the PCA and OPLS-DA models (). This indicates that the inhibitory effect of KG-1 on ALI was a major contributor to the changes in metabolomics profiles. To explore the differential metabolites among the three groups, we first obtained differential variables by comparing the control and KG-1 with model groups. Similarly, a distinct separation was revealed between the control and model groups (), as well as between the KG-1 and model groups (). As shown in the S-polt analysis, we acquired differential variables with VIP >1 and |p (corr)| ≥0.5 between the control and model groups (red dots, ), as well as the KG-1 vs model groups (red dots, ). Meanwhile, according to ANOVA, the variables with FC <0.5 or >2 and p value <0.05 were combined. Furthermore, the Venn diagram of these variables showed that the intersection of two groups was the set of variables related to KG-1 (), which indicates that they can be used as potential biomarkers for KG-1 against ALI.
Figure 2. Metabolome profiling for KG-1 anti- ALI in ESI + model. (A,B) PCA and OPLS-DA plots among the control, model and KG-1 groups. (C,E) OPLS-DA plots in the control group [R2X (cum) = 0.214, R2Y (cum) = 0.997, Q2 (cum) = 0.759] or KG-1 group [R2X (cum) = 0.545, R2Y (cum) = 1, Q2 (cum) = 0.869] in comparison to the model group. (D,F) S-plot analysis in the control group or KG-1 group in comparison to the model group. (G) Venn diagram, the intersection of two groups means was the set of variables related to KG-1 anti-ALI. QC sample (n = 5, yellow circle), the control group (n = 8, green box), the model group (n = 8, blue triangle), the KG-1 group (n = 8, red diamond).
![Figure 2. Metabolome profiling for KG-1 anti- ALI in ESI + model. (A,B) PCA and OPLS-DA plots among the control, model and KG-1 groups. (C,E) OPLS-DA plots in the control group [R2X (cum) = 0.214, R2Y (cum) = 0.997, Q2 (cum) = 0.759] or KG-1 group [R2X (cum) = 0.545, R2Y (cum) = 1, Q2 (cum) = 0.869] in comparison to the model group. (D,F) S-plot analysis in the control group or KG-1 group in comparison to the model group. (G) Venn diagram, the intersection of two groups means was the set of variables related to KG-1 anti-ALI. QC sample (n = 5, yellow circle), the control group (n = 8, green box), the model group (n = 8, blue triangle), the KG-1 group (n = 8, red diamond).](/cms/asset/5dd8d570-8236-4c44-910d-003e6b187545/iphb_a_2040544_f0002_c.jpg)
Figure 3. Metabolome profiling for KG-1 anti- ALI in ESI- model. (A,B) PCA and OPLS-DA plots among the control, model and KG-1 groups. (C,E) OPLS-DA plots in the control group [R2X (cum) = 0.38, R2Y (cum) = 0.986, Q2 (cum) = 0.881] or KG-1 group [R2X (cum) = 0.348, R2Y (cum) = 0.996, Q2 (cum) = 0.926]. (D,F) S-plot analysis in the control group or KG-1 group in comparison to the model group. (G) Venn diagram, the intersection of two groups means was the set of variables related to KG-1 anti-ALI. QC sample (n = 5, yellow circle), control group (n = 8, green box), model group (n = 8, blue triangle), KG-1 group (n = 8, red diamond).
![Figure 3. Metabolome profiling for KG-1 anti- ALI in ESI- model. (A,B) PCA and OPLS-DA plots among the control, model and KG-1 groups. (C,E) OPLS-DA plots in the control group [R2X (cum) = 0.38, R2Y (cum) = 0.986, Q2 (cum) = 0.881] or KG-1 group [R2X (cum) = 0.348, R2Y (cum) = 0.996, Q2 (cum) = 0.926]. (D,F) S-plot analysis in the control group or KG-1 group in comparison to the model group. (G) Venn diagram, the intersection of two groups means was the set of variables related to KG-1 anti-ALI. QC sample (n = 5, yellow circle), control group (n = 8, green box), model group (n = 8, blue triangle), KG-1 group (n = 8, red diamond).](/cms/asset/2d13abbb-c58c-45f0-b704-cbf09e9ad826/iphb_a_2040544_f0003_c.jpg)
Identification of potential biomarkers and involved pathways
Then, by querying the Mass Hunter PCDL Manager database, we identified and screened 12 differential metabolites (). Consequently, a radar chart of these biomarkers is intuitively presented (). Contrasted to the model group, the relative levels of the 10 metabolites decreased after KG-1 treatment, including glucosylceramide (d18:1/22:0), phosphatidylethanolamine (22:4(7Z,10Z,13Z,16Z)/14:1(9Z)), sphingomyelin (d17:1/24:1(15Z)), (indol-3-yl)acetamide, 2-aminophenol, urocanate, 11-dehydrocorticosterone, phosphatidylcholine (22:5(7Z,10Z,13Z,16Z,19Z)/P-16:0), retinoic acid, and phosphatidylinositol (22:6(4Z,7Z,10Z,13Z,16Z,19Z)/16:0). However, l-homophenylalanine and thiamine levels were increased after KG-1 treatment. Meanwhile, involved metabolic pathways were shown in , including glycerophospholipid metabolism, sphingolipid metabolism, thiamine metabolism, retinol metabolism, steroid hormone biosynthesis, histidine metabolism, tryptophan metabolism, and phenylalanine, tyrosine and tryptophan biosynthesis. Simultaneously, we conducted an interaction network of differentially expressed metabolites and related metabolic pathways (). These findings indicate that KG-1 reversibly adjusted these abnormal biomarkers in ALI mice.
Figure 4. Global metabolome outline. (A) Radar chart of 12 metabolites with relative abundance among the control group (blue line), model group (red line) and KG-1 group (green line). (B) Pathway enrichment of metabolites. (C) Network schematic diagram of metabolites and involved pathways. In the KG-1 vs model group, the metabolites in the pink box were increased in the KG-1 group; the metabolites in the blue box were decreased in the KG-1 group; the metabolites without box were not detected. Data are presented as the mean ± SEM (n = 8).
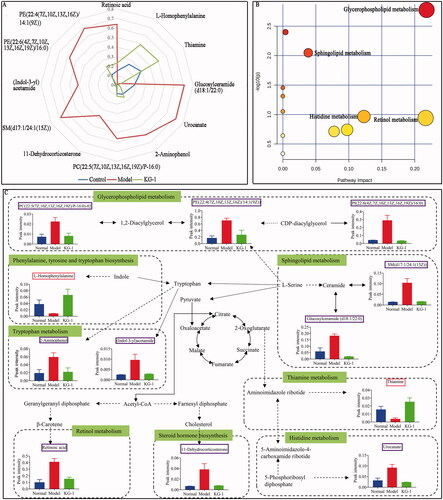
Table 1. The detailed information about 12 metabolites among the control, model and KG-1 groups.
Construction and analysis of “component-target-metabolite” network
To elucidate the protective effect of 7 herbal medicines of KG-1 for the potential metabolites, we integrated chemical components, drug targets, potential metabolites and its acting on targets and conducted a “component-target-metabolite” interaction network, including 93 chemical compounds and their related 150 targets and 6 potential metabolites and their related 558 targets (). As depicted in , 4 candidate metabolites of interactive network, including glucosylceramide (d18:1/22:0) (C01190), retinoic acid (C00777), PC (22:5(7Z,10Z,13Z,16Z,19Z)/P-16:0) (C00157), PE (22:4(7Z,10Z,13Z,16Z)/14:1(9Z)) (C00350), directly acting on the 7 putative drug targets including cytochrome P450 3A4 (CYP3A4, P08684), proteasome subunit beta type-6 (PSB6, P28702), retinoic acid receptor RXR-alpha (RXR-A, P19793), retinoic acid receptor RXR-gamma (RXR-γ, P48443), cytochrome P450 1A2 (CYP1A2, P05177), beta-galactosidase (β-GAL, P16278) and cytosolic phospholipase A2 (cPLA2, P47712).
Figure 5. The “compound-target-potential metabolite” interaction network of KG-1 in the treatment of ALI. (A) Overall interaction outline. (B) For those directly involved metabolites and targets in KG-1. The red triangles represent the putative compounds of KG-1; the sky-blue dots represent KG-1 related to putative targets; the purple dots represent potential metabolites related to putative targets.
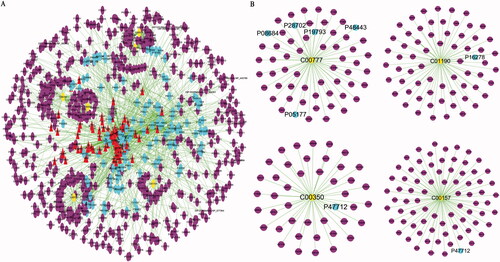
Furthermore, we simplified the “component – target – metabolite” network, as shown in , retinoic acid was co-regulated by Zhebeimu (zhebeiresinol and pelargonidin), Lianqiao (zhebeiresinol, pelargonidin, wogonin, (+)-pinoresinol monomethyl ether and onjixanthone I), Kuxingren (glabridin, machiline, (+)-catechin, oestrone and l-SPD), Sangye (arachidonic acid and tetramethoxyluteolin), Jinyinhua-Lianqiao-Sangye-Juhua (quercetin and kaempferol), Jinyinhua-Sangye (β-carotene), Jinyinhua-Sangye-Yiyiren-Kuxingren (stigmasterol), Lianqiao (hyperforin), acting on five potential targets including RXR-A, CYP1A2, CYP3A4, RXR-γ, PSB6. Tetramethoxyluteolin in Sangye acted on cPLA2 to modulate PC (22:5(7Z, 10Z, 13Z, 16Z, 19Z)/P-16:0) and PE (22:4(7Z, 10Z, 13Z, 16Z)/14:1(9Z)) and β-GAL to modulate glucosylceramide (d18:1/22:0), respectively.
Figure 6. The “compound-target-potential metabolite” simplified interactive network. The navy blue, red, pink, purple, light-green, dark-green, light-yellow triangles represent the putative active compounds of ZBM (Zhebeimu), LQ (Lianqiao), KXR (Kuxingren), SY (Sangye), JLSJ (Jinyinhua-Liaoqiao-Sangye-Juhua), JS (Jinyinhua-Sangye), JSYK (Jinyinhua-Sangye-Yiyiren-Kuxingren), respectively; the yellow dots represent the candidate metabolites; the sky-blue dots represent the shared targets of KG-1 and potential metabolites.
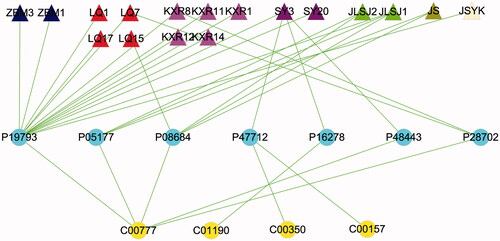
Discussion
KG-1 was synthesised from Sangju Drink, Yinqiao Powder, and Sanren Decoction (Wang et al. Citation2020). KG-1 has played a key role in reducing the generation and progression of ALI/ARDS in patients with COVID-19. To better understand the role of KG-1 anti-ALI, we studied the correlation between the metabolomic profile and phenotypic characteristics during the occurrence and development of ALI. In this study, a total of 12 differential metabolites and 8 metabolic pathways were found, which may be involved in the progression of the disease.
Pulmonary surfactants comprise approximately 90% of lipids (Cao et al. Citation2020), and PC is the most abundant. Changes in surfactant lipids on bacterial LPS have been reported (Deng et al. Citation2017). In addition, phospholipids may be involved in the recruitment of unsaturated fatty acids for inflammatory intermediates (Kalkhof et al. Citation2015; Olsvik et al. Citation2017; Cuykx et al. Citation2018). In this study, significantly higher levels of PC (22:5 (7Z, 10Z, 13Z, 16Z,1 9Z)/P-16:0), PE (22:4(7Z, 10Z, 13Z, 16Z)/14:1(9Z)) and PI (22:6(4Z, 7Z, 10Z, 13Z, 16Z, 19Z)/16:0) were found in the model group relative to the control group, which was consistent with previous literature (Wang et al. Citation2020). l-Serine is the link and bridge between the metabolism of glycerophospholipids and sphingolipids. Sphingolipids are involved in many pulmonary disorders (Navarrete et al. Citation2017). A close relationship between sphingolipid metabolism disorders and TNF-α has been reported (Maceyka and Spiegel Citation2014; Gomez-Muñoz et al. Citation2016). Similarly, the sphingomyelinase-ceramide pathway can activate and promote inflammation and oxidative action (Zhou et al. Citation2019). In light of our results, the levels of glucosylceramide (d18:1/22:0) and SM (d17:1/24:1(15Z)) were increased in LPS-induced ALI. We also observed higher expression of TNF-α, IL-6, CXCL1, and CXCL2 in the model group, compared to the control group. As a result of KG-1 treatment, the levels of the above glycerophospholipid and sphingolipid metabolites were restored to approximately normal levels. These findings indicate that KG-1 plays a significant role in rectifying fluctuations in glycerophospholipid and sphingolipid metabolism in the ALI mouse model.
Moreover, we also observed amino acid metabolism dysfunction in LPS-induced ALI. Tryptophan metabolism is closely associated with inflammation and the immune response (Kimura et al. Citation2012; Hu et al. Citation2020). Tryptophan is converted into several metabolites, such as (indol-3-yl) acetamide and 2-aminophenol, which were significantly increased in the model group compared to the control group. Furthermore, l-histidine is directly transformed into urocanate, which may be key to the occurrence of bacterial pathogenesis (Zhang et al. Citation2014). In this study, the control group showed lower levels of urocanate than the model group. Moreover, the level of l-homophenylalanine, an intermediate metabolite of phenylalanine, tyrosine, and tryptophan biosynthesis, was decreased in the model group than those in the control group. Through KG-1 medication, the (indol-3-yl)acetamide, 2-aminophenol, urocanate, and l-homophenylalanine levels were restored in the KG-1 group.
Retinol metabolism is closely related to vitamin A (Ross and Zolfaghari Citation2004), which promotes the progression and function of the immune system (Medeiros et al. Citation2015). The model group in this study had high levels of retinoic acid rather than the control group, which was consistent with a study observed by Hu et al. (Citation2020). In addition, thiamine is the core metabolite of the thiamine metabolism pathway, which is closely linked to vitamin B. Thiamine (also known as vitamin B1) has an important role in nerve tissue and mentality and was significantly lower in the model group compared to the control group. Similarly, the plasma concentrations of retinoic acid and thiamine were corrected to approximately near-normal levels in the KG-1 group.
To further explicate the modulation mechanism of KG-1 on 12 potential metabolites, we constructed and simplified a complicated "component-target-potential metabolite" interaction network. Seven herb medicines from KG-1 were observed to work together on five targets RXR-A, CYP1A2, CYP3A4, RXR-γ, proteasome subunit β type-6 to regulate retinoic acid. The function of RXR-A is in line with that of retinoic acid R-α, which activates naïve CD4+ T cells (Chambon Citation1996; Taherkhani et al. Citation2018). Furthermore, the differentiation of naïve CD4 + T cells may contribute to reducing the degree of severity of ALI and uncontrolled inflammation (Taherkhani et al. Citation2018). The CYP1A enzymes are protective against hyperoxic lung injury (Callaway et al. Citation2020). Additionally, high CYP1A2 expression has a protective effect on lung adenocarcinoma patients (Gastelum et al. Citation2020). The CYP3A4 is closely implicated in the elicitation of lung cancer (Jia et al. Citation2020). PXR-γ may participate in the pathogenesis of bronchopulmonary dysplasia (Boucherat et al. Citation2007). cPLA2 and β-GAL were the direct-acting targets from Sangye which modulated PC, PE and glucosylceramide, respectively. cPLA2 is a mediator of ALI by sepsis syndrome or acid aspiration (Nagase et al. Citation2000). PLA2 can liberate arachidonic acid from glycerophospholipids and is considered a key enzyme that controls the release of lipid mediator precursors (Lambeau and Lazdunski Citation1999; Fornoni et al. Citation2014). β-GAL is involved in cellular collagen metabolism and may take part in fibrosis in idiopathic interstitial pneumonia (Gessner et al. Citation2002). Meanwhile, β-GAL is known to hydrolyse three glycosphingolipids (Kiuchi et al. Citation1984). Therefore, these above findings may be considered as the putative targets in the occurrence, development and treatment of ALI. Meanwhile, it can explain the underlying mechanism of KG-1 against ALI.
There are some limitations of this research. The specific mechanism by which KG-1 regulates metabolites related to lipid, amino acid, and vitamin metabolism needs to be elucidated. Additionally, these biomarkers and potential targets still need to be validated in humans and investigated in future studies.
Conclusions
Taken together, KG-1 can mainly modulate lipid, amino acid, and vitamin metabolites fluctuations via acting on the putative targets in the treatment of ALI. This paper provides new perspectives and insights for KG-1 against ALI from the combination of untargeted metabolomics and network pharmacology.
Disclosure statement
None of the authors have any conflicts of interest with this work.
Additional information
Funding
References
- Bdeir K, Gollomp K, Stasiak M, Mei J, Papiewska-Pajak I, Zhao G, Worthen GS, Cines DB, Poncz M, Kowalska MA. 2017. Platelet-specific chemokines contribute to the pathogenesis of acute lung injury. Am J Respir Cell Mol Biol. 56(2):261–270.
- Bhatia M, Zemans RL, Jeyaseelan S. 2012. Role of chemokines in the pathogenesis of acute lung injury. Am J Respir Cell Mol Biol. 46(5):566–572.
- Boucherat O, Franco-Montoya ML, Thibault C, Incitti R, Chailley-Heu B, Delacourt C, Bourbon JR. 2007. Gene expression profiling in lung fibroblasts reveals new players in alveolarization. Physiol Genomics. 32(1):128–141.
- Callaway DA, Jiang W, Wang L, Lingappan K, Moorthy B. 2020. Oxygen-mediated lung injury in mice lacking the gene for NRF2: rescue with the cytochrome P4501A-inducer, beta-naphthoflavone (BNF), and differential sex-specific effects. Free Radic Biol Med. 160:208–218.
- Cao Z, Liu JR, Xie X, Zhan SE, Song W, Wu SW, Sun Z, Dong Y, Tang GD, Liu YL, et al. 2020. Lipidomic profiling of amniotic fluid and its application in fetal lung maturity prediction. J Clin Lab Anal. 34(4):e23109.
- Chambon P. 1996. A decade of molecular biology of retinoic acid receptors. FASEB J. 10(9):940–954.
- Channappanavar R, Perlman S. 2017. Pathogenic human coronavirus infections: causes and consequences of cytokine storm and immunopathology. Semin Immunopathol. 39(5):529–539.
- Cohen J, Normile D. 2020. New SARS-like virus in China triggers alarm. Science. 367(6475):234–235.
- Cuykx M, Claes L, Rodrigues RM, Vanhaecke T, Covaci A. 2018. Metabolomics profiling of steatosis progression in HepaRG® cells using sodium valproate. Toxicol Lett. 286:22–30.
- Dagvadorj J, Shimada K, Chen S, Jones HD, Tumurkhuu G, Zhang W, Wawrowsky KA, Crother TR, Arditi M. 2015. Lipopolysaccharide induces alveolar macrophage necrosis via CD14 and the P2X7 receptor leading to interleukin-1α Release. Immunity. 42(4):640–653.
- Deng GM, He H, Chen Z, Ou-Yang LQ, Xiao XQ, Ge JW, Xiang B, Jiang SC, Cheng SW. 2017. Lianqinjiedu decoction attenuates LPS-induced inflammation and acute lung injury in rats via TLR4/NF-κB pathway. Biomed Pharmacother. 96:148–152.
- Fornoni A, Merscher S, Kopp JB. 2014. Lipid biology of the podocyte-new perspectives offer new opportunities. Nat Rev Nephrol. 10(7):379–388.
- Gastelum G, Jiang W, Wang L, Zhou G, Borkar R, Putluri N, Moorthy B. 2020. Polycyclic aromatic hydrocarbon-induced pulmonary carcinogenesis in Cytochrome P450 (CYP)1A1- and 1A2-null mice: roles of CYP1A1 and CYP1A2. Toxicol Sci. 177(2):347–361.
- Gessner C, Wirtz H, Sack U, Winkler J, Stiehl P, Schauer J, Wolff G. 2002. BALF N-Acetylglucosaminidase and beta-galactosidase activities in idiopathic pulmonary fibrosis. Respir Med. 96(9):751–756.
- Gomez-Muñoz A, Presa N, Gomez-Larrauri A, Rivera IG, Trueba M, Ordoñez M. 2016. Control of inflammatory responses by ceramide, sphingosine 1-phosphate and ceramide 1-phosphate. Prog Lipid Res. 61:51–62.
- Hu LQ, Wang YQ, Sun HJ, Xiong Y, Zhong LY, Wu ZF, Yang M. 2020. An untargeted metabolomics approach to investigate the wine-processed mechanism of Scutellariae radix in acute lung injury. J Ethnopharmacol. 253:112665.
- Huang CL, Wang YM, Li XW, Ren LL, Zhao JP, Hu Y, Zhang L, Fan GH, Xu JY, Gu XY, et al. 2020. Clinical features of patients infected with 2019 novel coronavirus in Wuhan, China. Lancet. 395(10223):497–506.
- Jia ZQ, Zhou WR, Zhang GJ, Fu JK, Li DX, Ren L. 2020. CYP3A4 genetic variants are associated with susceptibility of non-small cell lung cancer in a Shaanxi Han population. Genomics. 112(5):3465–3472.
- Kalkhof S, Dautel F, Loguercio S, Baumann S, Trump S, Jungnickel H, Otto W, Rudzok S, Potratz S, Luch A, et al. 2015. Pathway and time-resolved benzo[a]pyrene toxicity on Hepa1c1c7 cells at toxic and subtoxic exposure. J Proteome Res. 14(1):164–182.
- Kimura T, Bier DM, Taylor CL. 2012. Summary of workshop discussions on establishing upper limits for amino acids with specific attention to available data for the essential amino acids leucine and tryptophan. J Nutr. 142(12):2245S–2248S.
- Kiuchi K, Mutoh T, Naoi M. 1984. A fluorometric microassay procedure for monitoring the enzymatic activity of GM1-ganglioside beta-galactosidase by use of high-performance liquid chromatography. Anal Biochem. 140(1):146–151.
- Lambeau G, Lazdunski M. 1999. Receptors for a growing family of secreted phospholipases A2. Trends Pharmacol Sci. 20(4):162–170.
- Li CY, Chen W, Zhang MY, Zhang CE, Cao B, Dong B, Qi SY, Zhang YJ, Fei XF, Li XJ, et al. 2020. Modulatory effects of Xihuang Pill on lung cancer treatment by an integrative approach. Biomed Pharmacother. 130:110533.
- Li CY, Niu M, Wang RL, Zhou XW, Dong B, Qi SY, Chen W, Zhang MY, Shi YF, Li RS, et al. 2019. The modulatory properties of Si Jun Zi Tang enhancing anticancer of gefitinib by an integrating approach. Biomed Pharmacother. 111:1132–1140.
- Li S, Zhang B. 2013. Traditional Chinese medicine network pharmacology: theory, methodology and application. Chin J Nat Med. 11(2):110–120.
- Maceyka M, Spiegel S. 2014. Sphingolipid metabolites in inflammatory disease. Nature. 510(7503):58–67.
- Medeiros SR, Pinheiro-Rosa N, Lemos L, Loli FG, Pereira AG, Santiago AF, Pinter EC, Alves AC, Oliveira JS, Cara DC, et al. 2015. Vitamin A supplementation leads to increases in regulatory CD4 + Foxp3 + LAP + T cells in mice. Nutrition. 31(10):1260–1265.
- Nagase T, Uozumi N, Ishii S, Kume K, Izumi T, Ouchi Y, Shimizu T. 2000. Acute lung injury by sepsis and acid aspiration: a key role for cytosolic phospholipase A2. Nat Immunol. 1(1):42–46.
- Navarrete A, Rupérez FJ, Mendes TO, Pérez-Rial S, Girón-Martínez A, Terrón-Expósito R, Díaz-Gil JJ, Peces-Barba G, Barbas C, García A. 2017. A metabolomic approach shows sphingosine 1-phosphate and lysophospholipids as mediators of the therapeutic effect of liver growth factor in emphysema. J Pharm Biomed Anal. 139:238–246.
- Niu M, Wang RL, Wang ZX, Zhang P, Bai ZF, Jing J, Guo YM, Zhao X, Zhan XY, Zhang ZT, et al. 2020. Rapid establishment of traditional Chinese medicine prevention and treatment of 2019-nCoV based on clinical experience and molecular docking. China J Chin Mater Med. 45:1213–1218.
- Olsvik PA, Skjaerven KH, Søfteland L. 2017. Metabolic signatures of bisphenol A and genistein in Atlantic salmon liver cells. Chemosphere. 189:730–743.
- Ramanathan K, Antognini D, Combes A, Paden M, Zakhary B, Ogino M, MacLaren G, Brodie D, Shekar K. 2020. Planning and provision of ECMO services for severe ARDS during the COVID-19 pandemic and other outbreaks of emerging infectious diseases. Lancet Respir Med. 8(5):518–526.
- Ross AC, Zolfaghari R. 2004. Regulation of hepatic retinol metabolism: perspectives from studies on vitamin A status. J Nutr. 134(1):269S–275S.
- Taherkhani A, Kalantari S, Nafar M. 2018. Prediction of molecular signature, potential biomarkers, and molecular pathways associated with membranous nephropathy based on protein protein interactions. Rev Invest Clin. 70(4):184–191.
- Tang MJ, Tian YH, Li DL, Lv JW, Li Q, Kuang CC, Hu PC, Wang Y, Wang J, Su K, et al. 2014. TNF-α mediated increase of HIF-1α inhibits VASP expression, which reduces alveolar-capillary barrier function during acute lung injury (ALI). PLoS One. 9(7):e102967.
- Wang JB, Wang ZX, Jing J, Zhao P, Dong JH, Zhou YF, Yang G, Niu M, Zhao X, Jiang TJ, et al. 2020. Exploring an integrative therapy for treating COVID-19: a randomized controlled trial. Chin J Integr Med. 26(9):648–655.
- Wang TY, Lin S, Liu R, Li H, Liu ZH, Zhang XN, Xu HR, Li Q, Bi KS. 2020. Metabolomic profile perturbations of serum, lung, bronchoalveolar lavage fluid, spleen and feces in LPS-induced acute lung injury rats based on HPLC-ESI-QTOF-MS. Anal Bioanal Chem. 412(5):1215–1234.
- Weinacker AB, Vaszar LT. 2001. Acute respiratory distress syndrome: physiology and new management strategies. Annu Rev Med. 52:221–237.
- Wishart DS. 2019. Metabolomics for investigating physiological and pathophysiological processes. Physiol Rev. 99(4):1819–1875.
- Yang YX, Li FY, Wei SZ, Liu XY, Wang YY, Liu HH, Wang JB, Li HT, Cai HD, Zhao YL. 2019. Metabolomics profiling in a mouse model reveals protective effect of Sancao granule on Con A-induced liver injury. J Ethnopharmacol. 238:111838.
- Young D, Lamb SE, Shah S, MacKenzie I, Tunnicliffe W, Lall R, Rowan K, Cuthbertson BH. 2013. High-frequency oscillation for acute respiratory distress syndrome. N Engl J Med. 368(9):806–813.
- Zhang H, Neuhöfer P, Song L, Rabe B, Lesina M, Kurkowski MU, Treiber M, Wartmann T, Regnér S, Thorlacius H, et al. 2013. IL-6 trans-signaling promotes pancreatitis-associated lung injury and lethality. J Clin Invest. 123(3):1019–1031.
- Zhang L, Liu XY, Tu C, Li CY, Song D, Zhu JX, Zhou YY, Wang XH, Li RS, Xiao XH, et al. 2020. Components synergy between stilbenes and emodin derivatives contributes to hepatotoxicity induced by Polygonum multiflorum. Xenobiotica. 50(5):515–525.
- Zhang XX, Ritchie SR, Rainey PB. 2014. Urocanate as a potential signaling molecule for bacterial recognition of eukaryotic hosts. Cell Mol Life Sci. 71(4):541–547.
- Zhou XS, Wang RP, Zhang T, Liu F, Zhang W, Wang GL, Gu GR, Han QQ, Xu DM, Yao CL, et al. 2019. Identification of lysophosphatidylcholines and sphingolipids as potential biomarkers for acute aortic dissection via serum metabolomics. Eur J Vasc Endovasc Surg. 57(3):434–441.
- Zhu N, Zhang DY, Wang WL, Li XW, Yang B, Song JD, Zhao X, Huang BY, Shi WF, Lu RJ, et al. 2020. A novel coronavirus from patients with pneumonia in China, 2019. N Engl J Med. 382(8):727–733.