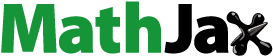
ABSTRACT
Internal crowdsourcing offers new opportunities for ideas to gain legitimacy in idea development as not only managers but also experts are able to provide feedback to improve and subsequently accept ideas for further realisation. Given this far limited knowledge about how ideas gain legitimacy in idea development, this paper focuses on the influence of feedback, a main source of idea legitimacy in internal crowdsourcing. More specifically, this study aims to explore how feedback from different feedback providers (i.e., managers and experts) influences idea selection in internal crowdsourcing idea development. Based on text mining methods including sentiment, topic-model, and expert identification analyses, results reveal that feedback sentiment, feedback diversity and feedback amount play key roles in idea legitimisation. Specifically, these aspects of feedback influence idea acceptance in idea selection through the mediating role of idea revision in idea development and that this relationship is moderated by feedback providers. This study extends previous knowledge about the legitimisation process from the perspective of feedback in idea development by offering both a more comprehensive view, including different feedback aspects, and a more granular measurement of these, through the use of text analysis. Based on insights from the study, practical implications are presented for how to gain legitimacy from feedback for idea selection in internal crowdsourcing.
Introduction
Innovation success greatly depends not only on the quality of created ideas but also on the effectiveness and accuracy of idea selection (Chan et al., Citation2018; Kruft et al., Citation2019; H. Zhu et al., Citation2019). Efficiently and effectively eliminating low-potential ideas can prevent firms from suffering expensive investments and failures (Chan et al., Citation2018). However, idea selection confronts challenges with the wide usage of crowdsourcing for innovation, since there is a large number of ideas generated from diverse crowds and the attention and cognitive capability of managers to identify the value of ideas are limited (Beretta, Citation2019; Özaygen & Balagué, Citation2018). Crowdsourcing, an approach normally utilising an internet-based platform to search for innovative ideas (mainly from external crowds, e.g., customers) (Afuah & Tuccie, Citation2012), has gained increased popularity over time as it has shown itself to support innovation. Inspired by the benefits of crowdsourcing, many firms start to make use of the advantages of employees as innovation sources in the form of internal crowds or communities, since employees possess an in-depth understanding of the organisational context and likely develop more feasible and implementable solutions compared to external crowds (Beretta & Søndergaard, Citation2021; Chen et al., Citation2022; Ruiz & Beretta, Citation2021). This type of firm-internal use of employees is often referred to as internal crowdsourcing, where employees can not only freely submit ideas but also voluntarily comment on others’ ideas, thereby sharing potentially valuable knowledge for innovation (Beretta & Søndergaard, Citation2021; H. Zhu et al., Citation2019). Nevertheless, previous studies have, so far, mostly focused on external crowdsourcing, and the understanding of internal crowdsourcing has so far been limited, especially for what regards idea selection (Beretta & Søndergaard, Citation2021; Chen et al., Citation2020).
An efficient and effective idea selection is a social-political decision-making process highly related to idea legitimacy (Bunduchi, Citation2017; Heusinkveld & Reijers, Citation2009), that is a perception of appropriateness and desirability within a socially constructed system of norms, values, beliefs, and definitions (Suchman, Citation1995; Zimmerman & Zeitz, Citation2002). Generally, ideas can be considered to be legitimate when they are desirable or appropriate within firms’ strategy according to the work of Suchman (Citation1995). However, ideas generated by diverse and distributed employees in internal crowdsourcing are prominently characterised by novelty and uncertainty and generally do not conform to the taken-for-granted patterns of thought and actions (Heusinkveld & Reijers, Citation2009) and can easily be considered to be ‘illegitimate’ (Dougherty & Heller, Citation1994, p. 202). A failure to deal with this lack of legitimacy might lead firms to reject promising ideas or, conversely, to push inadequate ideas too far for political reasons (Bunduchi, Citation2017; Florén & Frishammar, Citation2012; Gutiérrez & Magnusson, Citation2014). Consequently, gaining legitimacy for ideas after idea generation is important for the effective idea selection (Bunduchi, Citation2017; Heusinkveld & Reijers, Citation2009), and then in particular in internal crowdsourcing.
In general, legitimacy is gained from organisational members with formal authority, which in the traditional setting normally refers to individuals holding specific management roles (Dougherty & Heller, Citation1994; Ivory, Citation2013). In internal crowdsourcing, ideas can gain the commitment to align with firms’ strategic interests from not only managers but also experts and peers, since different categories of employees have opportunities to contribute to ideas in the form of online feedback over time through a social-political process of pushing and riding their ideas into good currency. Furthermore, from the point of view of the process of idea legitimisation, internal crowdsourcing enables that idea legitimisation does not only occur in idea generation and idea selection but can also take place in idea development where ideas have a much greater probability of being improved with the help of feedback. Summary in the work of Florén and Frishammar Citation2012, idea legitimisation is mainly reflected by who is involved in idea development and how idea development is conducted. One important aspect of this is arguably feedback, since it offers both social support and knowledge input to managers making decisions in idea selection (Kijkuit & van den Ende, Citation2007). Furthermore, even if ideas have a greater probability of being legitimised with the use of feedback, idea legitimacy could also be increased if feedback providers with formal or informal authority (e.g., managers and experts) become involved in idea development preceding idea selection (Florén & Frishammar, Citation2012). Therefore, understanding how ideas gain legitimacy through feedback from different feedback providers in idea development is a key question in internal crowdsourcing.
Considerable attention has been paid to the constituent elements of legitimacy (Ivory, Citation2013). However, much less effort has been put into understanding the sources of legitimacy and the process as well as the impact of legitimisation (Bunduchi, Citation2017; Suddaby & Greenwood, Citation2005), especially in terms of feedback in internal crowdsourcing idea development. Even if related studies (e.g., Chan et al., Citation2018; Deichmann et al., Citation2021) examine these factors in idea selection, they are mainly focused on the self-legitimisation of ideas in idea generation. To address this identified research gap, this research aims to explore the effects of feedback from different feedback providers (i.e., managers and experts) on idea selection in internal crowdsourcing by answering the research question: How do ideas gain legitimacy for idea selection in internal crowdsourcing idea development?
To address this research question, an empirical study is conducted based on data collected from a Swedish multinational company, with the usage of text mining methods, including sentiment, topic-model and expert-finding analysis in R software. The results reveal that feedback potentially impacts idea selection through the mediating roles of idea development in internal crowdsourcing. Furthermore, it is found that the type of feedback provider plays a role as a moderator on the effects of different feedbacks. The theoretical contribution of this research primarily lies in the development of new insights regarding the legitimisation of ideas by considering different feedback factors in internal crowdsourcing. This is one of the first studies to explore different roles of feedback in conjunction with different feedback providers, thereby providing a more detailed view of the process of ideas gaining legitimacy with feedback in idea development. Moreover, in terms of management contributions, this study provides suggestions on how ideas gain legitimacy from feedback for idea selection in firm-internal crowd-based innovation.
Theoretical background
Idea legitimacy and idea selection
Legitimacy, generally defined as a perception of appropriateness and desirability (Kannan-Narasimhan et al., Citation2014), is rooted in alignment with the prevalent corporate norms, beliefs, and cultural model (Bunduchi, Citation2017). Previous studies argued that ideas lack legitimacy due to their novelty, uncertain and ambiguous. Specifically, key characteristics of new ideas generally do not conform to the taken-for-granted patterns of thought and actions in various ways (Dougherty & Heller, Citation1994; Heusinkveld & Reijers, Citation2009). For instance, the novelty of ideas to some extent shows the violation of existing practices or may be out of sync with the firm’s strategy; thus, new ideas can easily be considered to be ‘illegitimate’ (Bunduchi, Citation2017; Dougherty & Heller, Citation1994, p. 202; Heusinkveld & Reijers, Citation2009). Even though there is a possible technical superiority of ideas, a lack of legitimacy poses a threat to the effective selection of ideas and innovation success as it limits the access to crucial resources for their realisation (Bunduchi, Citation2017). In other words, in idea selection, firms can reject promising ideas or, conversely, push inadequate ideas too far for political reasons because of the failure to deal with the illegitimate (Bunduchi, Citation2017; Florén & Frishammar, Citation2012; Gutiérrez & Magnusson, Citation2014).
In internal crowdsourcing, many of the broad, diverse, and uncertain ideas generated by distributed employees (i.e., managers, experts and peers) tend to lack legitimacy (Bunduchi, Citation2017). When selecting ideas originating from internal crowdsourcing, it is difficult for managers to identify a few valuable ideas in a large pool of ideas for further development and investment when the limited cognitive capability and time of managers are considered (Beretta, Citation2019; Chan et al., Citation2018; Kruft et al., Citation2019). Furthermore, the lack of adequate and concrete information related to ideas would increase the difficulty to pick the ‘right’ one for further investment (Chan et al., Citation2018; Gutiérrez & Magnusson, Citation2014; Kijkuit & Van Den Ende, Citation2007). Therefore, there is a need for ideas to gain and maintain legitimacy to increase the chances of idea selection (Bunduchi, Citation2017; Heusinkveld & Reijers, Citation2009), especially in internal crowdsourcing.
One important opportunity for ideas to gain legitimacy can be found in internal crowdsourcing idea development where employees are able to voluntarily provide feedback that influences idea quality and, consequently, idea selection (Beretta & Søndergaard, Citation2021; Chen et al., Citation2020; H. Zhu et al., Citation2019). In internal crowdsourcing idea development, some ideas are first evaluated based on the knowledge and experience of peers and then improved further according to the provided feedback. When the ideas reach a certain level of development, a formal decision is made via rational means issues in idea selection (Gutiérrez & Magnusson, Citation2014). However, there is little theory on the process by which crowds allocate their attention and contribute on ideas through collective feedback yet manage to gain legitimacy for early-stage ideas. This limited extant knowledge calls for a need for research on idea legitimisation in idea development where ideas can gain support through feedback from different providers to be improved and later selected for further actions and investments.
General factors on idea legitimisation
Ideas can be legitimate when they are perceived as desirable within the organisation’s socially constructed systems (Heusinkveld & Reijers, Citation2009). However, the characteristic of perception indicates that innovative ideas have to also strive for internal validation to be legitimacy (Drori & Honig, Citation2013), i.e., idea itself should not only be seen as desirable in the socio-political dimension but should gain the commitment of contributors to align with their strategic interests after idea generation (Florén & Frishammar, Citation2012; Kannan-Narasimhan, Citation2014). The internal validation of gaining consensus, namely idea legitimisation, highlights the necessity to reinforce the practice of community and to mobilise members around a common vision for ideas since those members are most likely to supply resources (i.e., signals of their feedback) to ideas that appear legitimate (Suchman, Citation1995).
Therefore, except from the factor of the idea itself in idea generation on increasing idea legitimacy, the success of idea legitimisation largely depends on how idea development is conducted and who is involved in idea development before the decision-making in idea selection (Florén & Frishammar, Citation2012). Related factors have been investigated and argued in the previous research about legitimacy-seeking and legitimacy outcomes. For example, Bunduchi (Citation2017) found that idea legitimation can be achieved through legitimacy-seeking behaviours including lobbying through product advertising or strategic communication, seeking external feedback from the external customers or internal audiences and relational building with selected audiences to generate critical mass or to demonstrate alignment with the prevailing model. Kannan-Narasimhan (Citation2014) argued that innovators in the early stage of innovation not only employ managerial attention as a key lever for managing their innovation’s legitimacy but also might have to use the process of gaining resources as an additional pathway to gaining legitimacy.
Based on the previous findings, it can be concluded that factors in idea development revealing the managerial attention, social network, information input, new version of idea description, etc. in idea development especially in form of different feedback potentially influence the idea legitimacy, and then the idea selection, the main outcome of gaining legitimacy (Gutiérrez & Magnusson, Citation2014).
Feedback as legitimacy sources
Feedback given is the main activity in idea development. With potential benefits from the transparent and open data prevalent in internal crowdsourcing, it can be noted that feedback as an information signal affects the manager's ability to select ideas (Beretta, Citation2019; Chan et al., Citation2021). Specifically, different dimensions of feedback such as feedback sentiment, feedback diversity, feedback amount, and feedback providers can be argued as the main aspects to reflect factors on the legitimisation of ideas. From previous research on idea feedback, it can be concluded that feedback from different feedback providers potentially offers three types of support, including political, knowledge, and social support. In this study, we note that political support, which serves as a touchstone for selection decisions on ideas, is related to feedback offered by the managers (Kruft et al., Citation2019). For what regards knowledge support, this is mainly determined by feedback information in terms of its diversity (H. Zhu et al., Citation2019) and feedback providers in terms of their expertise (Chen et al., Citation2020). By contrast, social support is partly dependent on social attention in terms of feedback amount (Di Vincenzo et al., Citation2020) and the emotion represented by feedback sentiment (Beretta, Citation2019). Therefore, we treat different feedback dimensions as the main sources of legitimisation for ideas, and we believe that understanding the influence of different feedback dimensions, including sentiment, diversity, and amount, in terms of political, knowledge, and social support, can provide a more comprehensive view on the process of idea legitimisation for idea selection in internal crowdsourcing.
Development of hypotheses
In order to better understand how ideas gain legitimacy for idea selection in internal crowdsourcing idea development, hypotheses are developed in this section including the direct effect of feedback on idea selection, the mediating role of idea revision and the moderating of feedback providers.
The effects of feedback on idea selection
Feedback sentiment and idea selection
Feedback sentiment, one of the feedback dimensions in terms of its positive and negative sentiment, is related to idea legitimacy with the signals of idea quality and feedback providers’ vision. Previous studies found that feedback framed in terms of positive and negative sentiment influence the manager’s decision-making processes in a different way (see Beretta, Citation2019). In the context of internal crowdsourcing, feedback with highly positive sentiment generally signals the popularity and interest of an idea within the online community (Beretta, Citation2019; Courtney et al., Citation2017) and may represent a recommendation for managers as to whether ideas should be considered for implementation or not (Beretta, Citation2019; DiGangi & Wasko, Citation2009; Jensen et al., Citation2014).
Unlike positive sentiment in feedback, negative sentiment in feedback has different signals and impacts on ideas. First, compared to a positive one with emotional support, feedback with negative sentiment refers to a more critical and informational view on assessing others’ ideas that align with firms’ strategic interests and requirements (Piezunka & Dahlander, Citation2015). This feedback with high negative sentiment, highlighting the limitation of ideas such as illegitimate, is considered highly informative by individuals and tends to be more influential when making decisions (Beretta, Citation2019). Furthermore, negative feedback might underline the risk of ideas and stimulate more scrutiny concerning their acceptance. On this basis, it may be indicated that ideas might be criticised and thus dropped halfway in or changed to a new idea based on negative feedback, something that is likely to result in the decrease in idea acceptance (Beretta, Citation2019). Consequently, the role of feedback sentiment in internal crowdsourcing for ideas can be hypothesised as follows:
H1a:
Feedback with high positive sentiment has a positive effect on the likelihood of idea acceptance in idea selection.
H1b:
Feedback with high negative sentiment has a negative effect on the likelihood of idea acceptance in idea selection.
Feedback amount and idea selection
Two dimensions of feedback information, namely feedback amount and diversity, have attracted the interests of scholars. Specifically, feedback amount, referring to the information amount provided by feedback content, has been argued to be an important factor for the idea acceptance in idea selection (e.g., Chan et al., Citation2021; H. Zhu et al., Citation2019). On the one hand, feedback amount reflects how employees allocate their social attention to certain ideas and is likely to influence managers to consider ideas further or not (Di Vincenzo et al., Citation2020). A related argument is that more extensive feedback provisioning means that ideas have gained more attention and support, and the more support there is for improvement the more the risk for idea rejection in idea selection is reduced (Chan et al., Citation2018). Based on this, we propose the following hypothesis:
H1c:
Feedback amount has a positive effect on the likelihood of idea acceptance in idea selection.
Feedback diversity and idea selection
Feedback diversity refers to the variety of knowledge in the feedback provided. Previous research about the role of feedback diversity has mainly focused on the diversity of feedback providers, namely the diverse knowledge or demographic background of feedback providers (see J. J. Zhu et al., Citation2017). With the development of text analysis technology enabled by the big data era, there is a recent interest on information diversity of feedback content as there are new possibilities to observe the real feedback content, and not only the characteristics of its providers. Higher information diversity then refers to a broader and non-redundant range of knowledge domains presented in the provided feedback and thus assessed by ideators. As more diverse feedback provides more alternative approaches and thereby stimulates divergent thinking of ideators to improve idea quality align with firm’s requirement for idea acceptance (J. J. Zhu et al., Citation2017). Therefore, we hypothesise that:
H1d:
Feedback diversity has a positive effect on the likelihood of idea acceptance in idea selection.
The mediating role of idea revision
Idea revision actions to some extent refer to the efforts of facilitating the knowledge support and learning the valuable knowledge from feedback (McGarrell & Verbeem, Citation2007). McGarrell and Verbeem (Citation2007) have argued the motivational role of feedback on draft revision, while Cho and MacArthur (Citation2010) have investigated the role of revision on the text writing quality. Therefore, we attempt to explore and unpack the influencing mechanisms of feedback by focusing on idea revision after feedback is given and before idea selection takes place, employing the segmentation approach proposed by Rasoolimanesh et al. (Citation2021) to develop a mediator hypothesis.
As mentioned in the above arguments for H1a, H1b, H1c and H1d, it can be indicated that feedback sentiment, feedback diversity, feedback amount and feedback provider all to some extent affect idea acceptance. Taking a more fine-grained view of these relationships, this study proposes that feedback during idea development also influences idea selection indirectly, via idea revision. First, in terms of the roles of feedback sentiment on idea revision, positive feedback may indicate great emotion support, where the motivation of the feedback receiver is stimulated for further revision of ideas (Ogink & Dong, Citation2019). Whereas the negative information indicates more scrutiny of ideas and limited willingness among feedback providers to accept them (Beretta, Citation2019), which could lead to a negative effect on the feedback recipient’s further contribution behaviour to improve ideas (Ogink & Dong, Citation2019). Second, a larger feedback amount generally refers to more concrete hints, and the increased gathered information reduces the uncertainty and increases the understanding of how to improve ideas (Jiang & Wang, Citation2020). Hence, ideators will be more motivated to revise their ideas, since they prefer to accept the less uncertain and more understandable information according to common information process bias (Ogink & Dong, Citation2019). Third, the feedback diversity potentially impacts how ideators make use of knowledge to revise and improve their ideas (Beretta, Citation2019; Chen et al., Citation2020; H. Zhu et al., Citation2019). The more diverse feedback is, the more alternative approaches exist for ideators to revise ideas. Therefore, the impacts of feedback on idea revision can be hypothesised as the following:
H2a:
Feedback with high positive sentiment has a positive effect on idea revision.
H2b:
Feedback with high negative sentiment has a negative effect on idea revision.
H2c:
Feedback amount has a positive effect on idea revision.
H2d:
Feedback diversity has a positive effect on idea revision.
Furthermore, the idea revision efforts motivated by the feedback are likely to improve the quality of idea described in the form of text (J. J. Zhu et al., Citation2017), which has been found to increase idea acceptance in idea selection (Chan et al., Citation2021; Chen et al., Citation2020). This related relationship among feedback, revision and quality has been widely investigated in the learning process (e.g., Cho & MacArthur, Citation2010; McGarrell & Verbeem, Citation2007). Therefore, it can be indicated that feedback affects the probability of idea acceptance in idea selection through the number of efforts put into idea revision in idea development. Following the segmentation approach (see Rasoolimanesh et al., Citation2021), we derive the following hypotheses:
H3a:
Idea revision mediates the relationship between feedback with positive sentiment and idea acceptance.
H3b:
Idea revision mediates the relationship between feedback with negative sentiment and idea acceptance.
H3c:
Idea revision mediates the relationship between feedback amount and idea acceptance.
H3d:
Idea revision mediates the relationship between feedback diversity and idea acceptance.
The moderating roles of feedback providers
The power and status of feedback providers have received substantial attention in previous studies, and it has been stressed that these factors to a certain degree determine feedback recipients’ responses. In particular, it has been found that people perceive feedback from experts and managers differently, as compared to feedback from others (Chan et al., Citation2021; Kruft et al., Citation2019). Experts exert informal power to direct ideators to improve ideas and influence managers to select ideas. The higher expertise that feedback providers have, the more trust ideators will have in the different signals of feedback (i.e., social and knowledge support from feedback amount and feedback sentiment) and the more motivation they will have to make use of feedback to improve their ideas, especially when the feedback contains more negative critical information (Ogink & Dong, Citation2019).
This trust with expertise indicates that feedback with negative sentiment, which has been argued as a demotivation factor for idea improvement, could for example to some extent result in more chances for idea revision in idea development with critical but helpful information input if the feedback comes from an expert. By contrast, when extensive positive feedback is received from an expert, this increases both cognitive and affective support for ideators (Ogink & Dong, Citation2019) in terms of further idea revision. The possible mechanisms behind these patterns would arguably be that more extensive feedback generally refers to more concrete hints on how to improve the idea, which in turn supports its development (Bergendahl & Magnusson, Citation2015; H. Zhu et al., Citation2019). Moreover, ideators would easily be motivated to improve their ideas by extensive feedback. If the extensive positive feedback comes from high-expertise feedback providers (i.e., experts), it would play a more important role than if it comes from individuals with lower expertise (i.e., non-experts) (Brand-Gruwel et al., Citation2005; Larkin et al., Citation1980), as the perceived value of the feedback also stems from the informal knowledge power and the related perceived trust in the information.
Besides the informal power from experts, manager exerting formal power has an impact on the role of feedback as well. Attracting feedback from managers to some extent means the attraction of managerial attention, a positive signal of idea legitimacy (Kannan-Narasimhan, Citation2014). In terms of the effect of the manager on idea revision, we argue that the formal power of the manager has a negative effect on idea revision as it pushes the rational means of idea selection earlier in idea development instead of in idea selection. This change to some extent indicates less space and opportunity for idea revision before the final formal decision-making in idea selection, according to the argument of Gutiérrez and Magnusson Citation2014. For example, positive feedback from a manager is likely to express that the ideas are good enough and there is no need to be changed, or there is information enough to change once instead of changing many times in response according to several repeated short comments from the manager. Thus, it might decrease the positive effects of feedback amount and sentiment in idea revision. Hence,
H4a:
The positive effects of feedback with positive sentiment and feedback amount on idea revision are decreased if the feedback is provided by manager.
H4b:
The positive effects of feedback with positive sentiment and feedback amount on idea revision are increased if the feedback is provided by expert.
H4c:
The negative effects of feedback with negative sentiment on idea revision are increased if the feedback is provided by manager.
H4d:
The negative effects of feedback with negative sentiment on idea revision are decreased if the feedback is provided by expert.
Similarly, the higher expertise feedback providers have, the more trust decision makers have in the received feedback and the more influence the feedback will have on idea selection (Kruft et al., Citation2019). Regarding the effects of cognitive and affective benefits from feedback on the selection of ideas, it has been argued that feedback with higher positive sentiment is more readily accepted if the feedback comes from a high-status provider (Kruft et al., Citation2019). In particular, the acceptance of feedback with high negative sentiment requires that the feedback comes from a high-status provider (Kruft et al., Citation2019) i.e., expect and manager. Specifically, feedback with high negative sentiment will lead managers to become more hesitant to select ideas (Beretta, Citation2019), feedback from someone with higher expertise or formal power would in turn increase the probability that decision-makers perceive that this idea is not complete and/or good enough to be selected.
H5a:
The positive effects of feedback with positive sentiment on idea selection are increased if the feedback is provided by manager.
H5b:
The positive effects of feedback with positive sentiment on idea selection are increased if the feedback is provided by experts.
H5c:
The negative effects of feedback with negative sentiment on idea selection are increased if the feedback is provided by manager.
H5d:
The negative effects of feedback with negative sentiment on idea selection are increased if the feedback is provided by experts.
Based on the above hypotheses, we propose the following research model (see ).
Data collection and measurement
Data collection
Data from an idea management system (IMS) using internal crowdsourcing in a Swedish multinational telecom company was extracted and used to test the proposed hypotheses. Since the IMS was set up in 2008, it has been used to capture and collectively develop ideas globally from employees for process improvement, technological innovation, business innovation, service innovation, etc. In 2014, it was updated with new and increased user and management functionality, as well as new interfaces. The IMS offers opportunities for dispersed and diverse employees to share and learn freely through information search, idea creation, commenting on ideas, etc. Furthermore, ideation is performed using different idea searches to solve different specific problems. The structured search for ideas is managed by voluntary innovation managers who make explicit what type of ideas they are looking for. In early 2016, this IMS had more than 14,000 users, 70000 ideas and around 100,000 comments throughout the global organisation, which brings about both opportunities and challenges for the firms to manage the systems and users to turn ideas into innovations.
In this study, all ideas published within open and collaborative idea searches during 1 year from 30 November 2014, to 1 December 2015, before the data extraction took place in February 2016. This one-year period was selected so that it started with the launch of the updated version of the platform and includes ideas from one full calendar year. The end date for data collection provided a cut off in time earlier than the extracted data on idea development and ideation performance, in order to let all ideas in the selected dataset have the possibility to be selected for further development or not. During this period, 6012 ideas were posted in the IMS. These ideas received 6,348 comments from 2,303 individuals, and 629 of these ideas were eventually accepted for further innovation actions.
Variables and measurements
A detailed description of variables and their measurements is presented in .
Table 1. Variables and measurement.
Dependent variables
Idea acceptance
To test hypotheses regarding idea selection in this study, whether or not the idea was accepted for further consideration was regarded as the main dependent variable in this study, with values of 0 and 1, respectively. A value of 1 is given if the idea has been claimed for interest, action, and/or implementation. Hence, this measure represents if the company has allocated resources to the idea to be further investigated or realised. Contrarily, a value of 0 means that the idea had not been selected for further consideration or investment. The way this dependent variable is operationalised follows studies in the field of crowdsourcing ideas done by Chan et al. (Citation2018) and Chen et al. (Citation2020).
Mediator
Idea revision
Inspired by the work of Chen et al. (Citation2020), idea revision is measured by the number of editions of an idea after the first comment is given.
Independent variables and moderator
Feedback sentiment
Sentiment analysis has been well recognised through Natural Language Processing (NLP) (Nasukawa & Yi, Citation2003). This type of analysis is based on the understanding that certain words reflect specific emotional states and that emotions thus can be captured through the analysis of language used. Most related sentiment analysis packages in, e.g.,, R software are based on polarity, with the counting of positive and negative words. Here, the ‘syuzhet’ package (Jockers, Citation2017) has been used to capture positive- and negative sentiment words in each feedback comment and thereafter the positive- and negative sentiment levels of feedback have been calculated based on the average proportion of positive and negative words in feedback contributed to each idea according to formulas (1) and (2):
P is the number of positive words of feedback to each idea and N is the number of negative words to each idea. PS is the positive sentiment of feedback to each idea, and NS is the negative sentiment of feedback to each idea.
Feedback diversity
Feedback diversity here represents the diverse comments’ content to ideas, measured based on Sharon entropy, something which indicates the diversity and uncertainty degree (Jost, Citation2006; Masisi et al., Citation2008). In order to capture the Sharon entropy of comments, the ‘topicmodels’ package in R based on word frequency has been used (Hornik & Grün, Citation2011). The diversity measured by the entropy based on this method indicates the topic distribution among comments submitted to each idea. The captured examples of topics in this study can be seen in .
Table 2. Examples of topics.
On this basis, the topic entropy of each comment is first calculated by formula (3):
where En(C) is the topical entropy of comment C, denotes the normalised weight on the i:th topic for comment C, and k is the number of topics. With the usage of ‘topicmodels’ package in R, 50 topics were selected according to the work of Hornik and Grün (Citation2011). Thereafter, feedback diversity for each idea was calculated by formula (4):
where En(U) is the comment entropy for idea U, and m is the number of comments contributed to idea U during the selected one-year period.
Feedback amount
Feedback amount refers to the overall attention that an idea has created around itself, measured by the number of comments given to ideas (Chen et al., Citation2020; Di Vincenzo et al., Citation2020; Schemmann et al., Citation2016).
Feedback provider (manager)
Attracting feedback from managers to some extent means the attraction of managerial attention. This managerial attention here is measured by the number of comments provided by managers on an idea.
Feedback provider (expert)
Based on the expert identification theory in online platform, feedback provider in terms of expert is measured by the expertise of feedback providers in this study. In the measurement, three main approaches were used. These were 1) a candidate-based approach with the building of the candidate profile; 2) document-based approaches, and 3) topic modelling. As the topic modelling based on Latent Dirichlet Allocation (LDA) outperformed several profile and document-based approaches (Paul, Citation2016), the measurement of expertise here is based on the LDA-based topic modelling approach with the usage of the ‘topicmodels’ package in R software. After running this package, the topic of the
contribution, including comments and ideas,
as well as their probability distribution
, can be acquired based on the word usage frequency. Here, n is the number of selected topics and m represents the number of observed contributions. On this basis, the expertise of commenters on ideas was calculated based on the topic distribution between their previous contributions (including 21,769 comments and 5,751 ideas). To be more specific, 50 topics were initially selected through the ‘topic model’ package in R. Thereafter, the expertise about the
topic of commenters at comment level could be acquired through formula (5). Here, the setting of topics is based on the finding by Hornik and Grün (Citation2011) when the volume of investigated data is taken into account.
Where , where
denotes the number of previous contributions.
Thereafter, with the output of the topic distribution of the observed 2,314 ideas by ‘topicmodels’, the expertise of commenters at idea level was calculated using Formula (6).
Where denotes the probability distribution of the
topic of an idea.
This calculation is inspired by the recently proposed expert-finding tool proposed by Paul (Citation2016), where the expert was found based on the level of expertise in a specific topic area, and the expertise was calculated based on the topic distribution of individuals’ previous contributions (see formula (5)) in a specific topic area (see formula (6)). Furthermore, the value reflects the overlap degree between the individual’s contributions and the commented ideas. The higher
, the more expert the commenter will be on the commented idea.
Control variables
Idea length
Idea length to some extent can indicate its elaborateness, which might impact the final idea’s success (Chen et al., Citation2020). Here, we add it as a control variable through the same measurement of the number of words in a specific idea description.
Idea sentiment
Sentiment of ideas to some degree reflects the characteristics of creators’ moods, signalling creators’ inferior participation quantity and quality in the future (Coussement et al., Citation2017), and has a potential impact on innovation (O’Leary, Citation2016). It is thus necessary to include it as a control variable. Different from the measurement of feedback sentiment, idea sentiment is measured based on polarity with the counting of positive and negative words. One package, called ‘sentiment’ package in R and proposed by Jurka (Citation2012), can calculate the sentiment based on the value of classified polarity (see Formula (7)). The higher the sentiment value received from the package after running the text of a comment, the more positive this comment is.
Where IS is the sentiment of idea, something that is calculated by the number of positive and negative words, Sum(P) is the total number of positive words in idea description and Sum(N) is the number of negative words in idea description.
Idea relatedness
Idea relatedness is reflected by the similarity in the level of knowledge domain between ideas and their submitted idea boxes (Chen et al., Citation2020). As the case IMS is open for employees to briefly label the knowledge area of ideas with tags (e.g., windows platform, simulator and android) when they submit ideas or create idea boxes, we measured idea relatedness based on the number of identical same tags between an idea and its submitted idea box.
Idea attention
The variable about idea attention in this study refers to whether idea received feedback or not (Chan, et al., Citation2021; Wooten & Ulrich, Citation2017). It is a dummy variable, where Yes means that Idea has received feedback and No means that Idea has not received any feedback.
Feedback timeliness (first comment speed)
The speed of the first comment to some extent reflects the attractiveness of ideas. The attractiveness of an idea can be the result of the novelty and the high quality of the idea, which is related to the idea revision in idea development and idea acceptance in idea selection. The high speed of comment that an idea gains, the high attractiveness of ideas is. It is measured through a countdown of time distance between the time an idea is published and the first comment is created on an idea.
Feedback timeliness (time to feedback)
Feedback timeliness, representing the speed of feedback given, has an impact on the idea acceptance (Chen et al., Citation2020). Thus, feedback timeliness is included as a control variable. The calculation is based on the second unit, measured by the average time distance of feedback given after an idea is submitted (see Formula (2)).
is the average of time to feedback,
denoting the time when the
feedback is given,
represents the number of comments on an idea.
Data analysis and results
Data analysis
In terms of the statistical analysis at the idea level, in order to test the interdependence between multiple variables, a matrix correlation was first calculated. Thereafter, VIF (variance-inflated factors) test was performed to assess whether there was multicollinearity among the tested variables or not. However, since the data analysis is conducted at two stages in terms of idea development and idea selection, the VIF of the two models were tested, respectively. Thirdly, in order to test the effects of independent variables on dependent variables and the interaction effects, two regression models were performed through the statistical software R. The first one is the linear regression for the continuous dependent variable-idea revision, while the second one is a logit model for the binary dependent variable within a large dataset (Aldrich & Nelson, Citation1984). In these two types of models, the interaction effects were tested with the mean-centring variables, because the mean-centring value could help clarify regression coefficients without altering the overall R-square (Iacobucci et al., Citation2016). Finally, in order to test the significance of mediation effects, the Bootstrap test is recommended (X. Zhao et al., Citation2010). By doing so, the ’Mediation’ package in R with the setting of Bootstrap (Tingley et al., Citation2014) was employed to test mediation effects.
Results
Data description
The matrix correlation (see ) was first calculated. This shows that there are several interrelationships between the investigated variables.
Table 3. Matrix correlation of all variables.
Although several correlation coefficients are higher than 0.7, a threshold of high correlation (Dormann et al., Citation2013), we analysed the regression results by adding rather than omitting the potentially relevant collinear variables according to the suggestion of Lindner et al. (Citation2020) in all but extreme cases of collinearity (e.g., 0.8 and above, see Allison, Citation1999, p. 64).
Then, a VIF test with all variables was performed. The results show that the tested regression models are not distorted by this multicollinearity since all VIF values of independent variables are lower than 2, far lower than a threshold level 5 (Cohen et al., Citation2013). Furthermore, the significant coefficient signs of the tested variables are stable along with the minor changes of VIF in different regression models (Vatcheva et al., Citation2016).
Linear regression results for idea development
The influence of the investigated independent variables on the acceptance of ideas is shown in . In , Model 1 is used for all the other models and only includes the control variables. From Model 2 to Model 4, feedback sentiment, feedback diversity, and feedback amount were added, respectively. In model 5 and model 6, the feedback provider as a moderator was added, and the moderation effects were tested.
Table 4. Linear regression results for idea development.
From Model 3 to Model 6, it can be seen that the effects of feedback diversity and feedback amount remain significant, and thus H2c and H2d are supported. For feedback sentiment, Model 5 shows that an increased positive sentiment has a significant positive effect on idea revision, supporting H2a, whereas the effect of negative sentiment is insignificant, and thus not offering support for H2b. In model 6, it is seen that the moderation effects of feedback providers on positive sentiment and feedback amount are statistically significant. These moderation effects of feedback providers are visualised in and .
In , the vertical axis and the horizontal axis show that the positive effects of increased positive sentiment and comment length are decreased if there are more comments provided by managers, supporting H4a. By contrast, shows that the increased length of feedback and expertise of feedback provider along the horizontal axis result in an increased idea revision, thus supporting H4b. However, the insignificant moderation of feedback providers on negative sentiment shows that H4c and H4d are not supported.
Logistic regression results for idea selection
The result about the influence of feedback on the acceptance of ideas in idea selection is shown in . In , Model 1 is used for all the other models and only includes the control variables. From Model 2 to Model 5, independent variables were introduced step by step. The effects of the feedback provider and its moderating effects were tested in Model 6 and Model 7, respectively.
Table 5. Logistic regression results for idea selection.
In , the results about the significant impacts of feedback-negative sentiment and amount on idea selection support H1b, whereas the insignificant role of feedback diversity shows that H1c is not supported. For what concerns the moderation effect of feedback providers in Model 7, the moderation effects on feedback amount and feedback diversity are not included as they were found to lack statistical significance. In order to better understand the role of feedback providers on feedback sentiment, the significant moderation effects of feedback providers are visualised in . It is seen that the influence of feedback sentiment on idea selection is moderated by the expertise of providers but not by the feedback providers being managers where H5a and H5c are not supported. However, the moderation effect of expertise on the positive sentiment of feedback in idea selection is statistically positively significant, whereas the moderation effect of expertise on the influence of negative sentiment of feedback in idea selection is not significant, leading us to conclude that H5b is supported while H5d is not supported.
Mediation test based on bootstrap analysis
In order to test mediation effects, the use of a bootstrap test is recommended (X. Zhao et al., Citation2010). In this study, the ’Mediation’ package in R with bootstrap setting (Tingley et al., Citation2014) was employed to test each mediator separately. The results are shown in .
Table 6. Mediation test results.
shows that the indirect effects of feedback sentiment, amount and diversity on idea acceptance are statistically significant, and H3a, H3b, H3c and H3d are thus supported. Furthermore, the non-significant direct effects of feedback sentiment and diversity show that idea revision completely mediates the effects of feedback sentiment and diversity on idea acceptance. By contrast, the significant direct and indirect shows that the effect of feedback amount on idea acceptance is partly mediated by the idea revision.
Discussion
Feedback from internal crowds clearly holds a potential to inform the idea selection process (Chen et al., Citation2020; Hoornaert et al., Citation2017). The purpose of this study is to explore how feedback from different feedback providers (e.g., managers and experts) influences idea development and idea selection in internal crowdsourcing. Importantly, the empirical study has shown the influence of feedback affecting the idea acceptance in idea selection through the mediating role of idea revision, and this effect is found to be moderated by the feedback providers.
Findings
The first finding concerns the role of feedback in helping managers to get the commitment on ideas to filter out ideas in idea selection regarding H1a, H1b, H1c and H1d. The empirical results about the significant impact of feedback on idea acceptance are consistent with previous findings that the social attention support (Di Vincenzo et al., Citation2020) and social emotion support (Beretta, Citation2019) for the commitment of ideas can be gained from feedback. First, the finding of the positive role of feedback amount is consistent with the argument by Chan et al. (Citation2018), showing that more extensive feedback provisioning means that ideas have gained more attention and support, and the risk for idea rejection in idea selection is reduced. It can be explained that the increased provided social attention in terms of feedback amount refers to the desirable and attractiveness of ideas and increases the willingness of managers to accept this idea. Second, taking a closer look at the content of the feedback, it is found that negative feedback was found to decrease the acceptance of ideas, whereas positive feedback did not reveal a clear overall effect, which is consistent with the work of Beretta (Citation2019) and Piezunka and Dahlander (Citation2015).
The second finding of this study is the identified significant mediating role of idea revision in idea development. Firstly, the observed results show that feedback sentiment, feedback amount and feedback diversity all have a positive effect on idea revision in idea development, supporting H2a, H2b, H2c, and H2d. Secondly, it is seen that the increased idea revision in idea development increases the likelihood of idea acceptance in idea selection. These results are consistent with the opinion that ideators prefer to receive positive, concrete, and diverse feedback to stimulate themselves to make use of feedback to revise their ideas and improve the quality of ideas (Chan et al., Citation2021; Ogink & Dong, Citation2019), something that indirectly increases the likelihood of idea selection.
Third, when the moderation effect of feedback provider is considered, it was found that there is a positive effect of positive feedback in idea selection, but that such an effect is only seen when the feedback provider can be considered an expert. This is consistent with previously presented arguments, namely that managers making decisions tend to trust information provided by experts more than information from other sources (Kruft et al., Citation2019). Thus, feedback can be more useful for ideas to get the commitment for being further accepted if the feedback is from experts (supporting H5b). Furthermore, we also found that feedback given by managers in idea development has a significant effect on idea acceptance. It indicates that this feedback on an overall level provides political support for ideas since it pushes the rational means of idea selection earlier in idea development instead of in idea selection. Regarding the moderation effects of feedback providers on idea revision, the results supporting H4b show that if extensive positive feedback comes from high-expertise feedback providers (i.e., experts), ideators would be more motivated to improve their ideas, which is in line with the related study of Brand-Gruwel et al. (Citation2005). By contrast, the effects of feedback from managers on idea revision are different. The results supporting H4a show that the increased length and positive sentiment of feedback from manager would not increase idea revision. These effects indicate that positive feedback from a manager is likely to express that the ideas are good enough and there is no need to be changed, or there is information enough to change once instead of changing many times in response according to several repeated short comments from the manager.
Finally, what is also seen in the regressions is the finding that the effect of negative feedback is unstable, changing from being positive, at a statistically significant level, to instead being negative, although insignificant (where H1b is not supported). This opens for a discussion of the potential demotivation effects of negative feedback as well as the possible benefits of negative feedback, given its informative characteristics (Zhou, Citation1998). Zhou (Citation1998) initially advocated the use of negative feedback with an informational rather than controlling style, indicating that negative feedback revealing the truth in an informational manner has a better effect on performance than feedback in a controlling style, as the latter might put substantial pressure on the individuals and restrain their creativity. Furthermore, with an open mind to learn from negative feedback and the assumption that negative feedback is in fact showing the reality (Audia & Locke, Citation2004; Zhou, Citation1998), it can be argued that negative feedback sometimes is a good option as well (Erickson et al., Citation2022), but that the motivation of ideators to accept the feedback needs to be taken into account.
Altogether, it can be concluded that gaining idea legitimacy in idea development for idea selection depends on the different social, knowledge, emotional, and political supports gained from different feedback providers (i.e., manager and expert). Furthermore, attending to the diverse effects of feedback sentiment, amount, and diversity on the efforts of idea revision is important, as the resulting efforts in terms of idea quality improvement (Chan et al., Citation2021) lead to the improved idea legitimacy and an increased probability of idea acceptance in idea selection (Chen et al., Citation2020).
Theoretical implications
This study entails several theoretical implications. Firstly, this study contributes to the literature about legitimacy and crowdsourcing ideas by investigating the different roles of different feedback in internal crowdsourcing idea development and providing a new perspective on gaining legitimacy. Specifically, most of the previous attention has been paid to the constituent elements of legitimacy (Ivory, Citation2013). However, much less effort has been put into understanding the sources of legitimacy and the process as well as the impacts of legitimisation (Bunduchi, Citation2017; Suddaby & Greenwood, Citation2005). Even if related studies (e.g., Chan et al., Citation2018; Deichmann et al., Citation2021) examine these factors in online idea selection, they are mainly focused on the self-legitimisation of ideas in idea generation. Feedback in idea development, as one of the legitimacy sources, has been shown to affect the process of legitimisation in terms of idea revision and legitimacy outcomes in terms of idea survival in this study.
Secondly, this study contributes to feedback theory in different ways, by using several theories including motivation theory, signalling theory, power theory, and legitimacy to explain the roles of different feedback. Although Kluger and DeNisi (Citation1996) proposed that there are mixed effects of feedback on performance, we do not have a clear view of these effects in terms of different feedback dimensions. In this study, we discussed how ideators get motivated/demotivated by different feedback in terms of sentiment and amount with the perceived competence and self-determination. We also contribute to the application of signalling theory on feedback to explain how feedback in terms of diversity, length, sentiment, and amount provides knowledge, emotion, and social support for ideators to improve ideas and managers to select ideas. Furthermore, the discussion on the roles of different feedback providers in terms of manager and expert provides a clarity on how formal and informal power influence the trust and usage of feedback to get a commitment to ideas during idea legitimisation. Moreover, we provide a detailed view of how feedback impacts the different processes of idea activity by investigating the mediating role of idea revision. To the best of my knowledge, it is one of the first studies to integrate the view of both idea development and idea selection on the impacts of feedback on both idea revision and idea selection.
Thirdly, this study mainly contributes to the research on the role of feedback content based on text mining analysis, especially in terms of feedback diversity. More specifically, this study separately investigates the diversity of feedback content and the role of different feedback providers, providing a more detailed view of the role of feedback than in previous studies by H. Zhu et al. (Citation2019). Considering the diversity of feedback providers, this study focuses on the expertise of feedback providers, which contributes to existing text mining research on expert identification in crowdsourcing by exploring the practical application of their proposed techniques as well. The empirical results do not only corroborate the usefulness of previously proposed techniques (see Paul, Citation2016) but also extend the research on expert identification in crowdsourcing from the exclusively technical area to also include the management domain.
Management implications
This study also provides implications to improve idea legitimacy and the chance of idea survival, through managing feedback content as well as feedback providers in internal crowdsourcing. First of all, the results show that ideas gain legitimacy not only from the idea quality itself but also from the feedback process in idea development. Thus, the idea development process should be highlighted and encouraged with the support of feedback and different contributors, where information technology such as crowdsourcing could be applied inside firms. Secondly, considering the different effects of feedback on the motivation of idea revision for ideators, managers could be careful about these effects and take motivation approaches as much as possible. For example, when the idea is promising but gets negative feedback and little amount of feedback, there might be a need to motivate ideators to improve ideas. Furthermore, the significance of feedback from managers suggests that appropriate attention to ideas in idea development is necessary for ideas to be improved. Moreover, considering that managers trust more the feedback provided by experts, and the positive feedback from experts has a positive significance on both idea revision and idea acceptance, this study suggests that managers can encourage the experts to contribute ideas and ideators make efforts to attract experts to improve their ideas’ legitimacy.
For the feedback information side, it is indicated on the one hand that a critical thinking style needs to be promoted for commenting and that simple positive feedback like ‘Good idea’ is hardly useful and needs to be more specific. Even if feedback provider thinks that an idea is really good, some reasonable objective reasons for this expression should be given within the feedback content. Moreover, as the encouraged and concrete feedback pushes ideators to pay more critical efforts to improve ideas and attracts managers’ attention on ideas, it can be suggested that firms should place emphasis on the importance of engagement motivation as well as focused areas of contributors. On the other hand, as the negative feedback proposed by high expertise is likely to kill ideas, bad ideas would be efficiently stopped by experts in order to save further efforts and costs. Nevertheless, some good ideas might be killed prematurely, so it is indicated that the objective reasons for why an idea is not regarded as valuable should be given to increase the opportunities for reciprocal discussion and learning leading to further idea development.
Limitation and future research
Although this work contains a range of new findings and implications, it still has some limitations. First of all, it would be desirable to have more complete information to better define the success of ideas or idea quality, which is also a hot issue in the idea evaluation process. On the one hand, in the informal evaluation process, idea development, how ideas attract feedback is largely based on the value of ideas. The unclear idea quality might result in a concern of endogeneity which should be tested in the future study with more supported data. On the other hand, in terms of the dependent variable in this study, the real value of idea acceptance is unclear, given the uncertainty of idea evaluation and implementation. This could be reflected in the incorrect acceptance of ideas that later turn out to be failures, but also in lost opportunities as ‘… people often reject creative ideas, even when espousing creativity as a desired goal’ (Mueller et al., Citation2012, p. 13), resulting in the risk of missing out valuable radical and disruptive ideas. Consequently, the criteria used for evaluating idea quality need to be further investigated in research on idea evaluation in order to reflect the uncertainties of attracting feedback and idea selection process. In terms of idea implementation, uncertainty is more pronounced when the long-term value is taken into account. In future studies, it would be desirable to have more complete information to define the success of ideas or idea quality from a long-term perspective.
Besides this, another limitation is that basic individual information like age, nationality and gender is not considered due to limited access to this type of data. This limitation restrains the validation test of measures such as feedback diversity related to the background of the feedback provider. Future study will consider this data access to support the validation of measurement and statistical analysis. Finally, the generalisability of this research design is limited because the data is extracted from only one specific company. Further studies should extend the data collection to allow for better comparisons of the investigation results across industries and firms.
Author statement
Qian Chen: Methodology, Software, Data curation, Writing – Original draft and Editing. Mats Magnusson: Supervision, Conceptualisation, Reviewing and Editing. Jennie Björk: Co-supervision, Validation, Reviewing and Editing.
Acknowledgement
We would like to extend our appreciation for the financial support provided by The major project of the Humanities and Social Sciences Base of the Ministry ofEducation (No. 22JJD630008). We are grateful to Prof. Magnus Karlsson who has provided opportunities and suggestions to help this paper move forward. And many thanks to the Research Initiative on Sustainable Industry and Society (IRIS) at KTH Royal Institute of Technology.
Disclosure statement
No potential conflict of interest was reported by the author(s).
Correction Statement
This article has been corrected with minor changes. These changes do not impact the academic content of the article.
Additional information
Funding
Notes on contributors
Qian Chen
Qian Chen received her PhD at the KTH Royal Institute of Technology in 2019. She is a Postdoctoral Researcher in research area on Innovation management, innovation eco-systems and entrepreneurship as part of the Research Initiative on Sustainable Industry and Society (IRIS) Program. The main research method she used is quantative analysis based on text mining. Her research interests are Front end of innovation, Crowdsourcing, Sustainable innovation. She has published her work in journals such as the Journal of Knowledge Management, European Journal of Innovation Management.
Mats Magnusson
Mats Magnusson is Professor of Product Innovation Engineering at KTH Royal Institute of Technology in Stockholm and Permanent Visiting Professor at LUISS School of Business and Management in Rome. His research and teaching activities address different strategic and organisational aspects of innovation, and he has published extensively on these topics in, e.g., Organisation Studies, Research Policy, Journal of Product Innovation Management, and Long Range Planning.
Jennie Björk
Jennie Björk is Associate Professor in Product Innovation at Integrated Product Development, KTH Royal Institute of Technology, in Stockholm. Her main research interests are ideation, ideation management, knowledge sharing, knowledge creation and social networks. A substantial part of her research is based on social network analysis of innovation activities in firms and communities, based on studies undertaken in close collaboration with companies. She has published her work in journals such as the Journal of Product Innovation Management, Creativity and Innovation Management, and Industry & Innovation.
References
- Afuah, A., & Tucci, C. L. (2012). Crowdsourcing as a solution to distant search. Academy of Management Review, 37(3), 355–375.
- Aldrich, J. H., & Nelson, F. D. (1984). Linear probability, logit, and probit models (no. 45). Sage.
- Allison, P. D. (1999). Multicollinearity. In Logistic regression using the SAS system: Theory and application (pp. 48–51).
- Audia, P. G., & Locke, E. A. (2004). Benefiting from negative feedback. Human Resource Management Review, 13(4), 631–646. https://doi.org/10.1016/j.hrmr.2003.11.006
- Beretta, M. (2019). Idea selection in web‐enabled ideation systems. Journal of Product Innovation Management, 36(3), 5–23. https://doi.org/10.1111/jpim.12439
- Beretta, M., & Søndergaard, H. A. (2021). Employee behaviours beyond innovators in internal crowdsourcing: What do employees do in internal crowdsourcing, if not innovating, and why? Creativity and Innovation Management, 30(3), 542–562. https://doi.org/10.1111/caim.12449
- Bergendahl, M., & Magnusson, M. (2015). Creating ideas for innovation: Effects of organizational distance on knowledge creation processes. Creativity and Innovation Management, 24(1), 87–101. https://doi.org/10.1111/caim.12097
- Brand-Gruwel, S., Wopereis, I., & Vermetten, Y. (2005). Information problem solving by experts and novices: Analysis of a complex cognitive skill. Computers in Human Behavior, 21(3), 487–508. https://doi.org/10.1016/j.chb.2004.10.005
- Bunduchi, R. (2017). Legitimacy‐seeking mechanisms in product innovation: A qualitative study. Journal of Product Innovation Management, 34(3), 315–342. https://doi.org/10.1111/jpim.12354
- Chan, K. W., Li, S. Y., Ni, J., & Zhu, J. J. (2021). What feedback matters? The role of experience in motivating crowdsourcing innovation. Production and Operations Management, 30(1), 103–126.
- Chan, K. W., Li, S. Y., & Zhu, J. J. (2018). Good to be novel? Understanding how idea feasibility affects idea adoption decision making in crowdsourcing. Journal of Interactive Marketing, 43, 52–68. https://doi.org/10.1016/j.intmar.2018.01.001
- Chen, Q., Magnusson, M., & Björk, J. (2020). Collective firm-internal online idea development: Exploring the impact of feedback timeliness and knowledge overlap. European Journal of Innovation Management, 23(1), 13–39. https://doi.org/10.1108/EJIM-02-2018-0045
- Chen, Q., Magnusson, M., & Björk, J. (2022). Exploring the effects of problem- and solution-related knowledge sharing in internal crowdsourcing. Journal of Knowledge Management, 26(11), 324–347. https://doi.org/10.1108/JKM-10-2021-0769
- Cho, K., & MacArthur, C. (2010). Student revision with peer and expert reviewing. Learning and Instruction, 20(4), 328–338. https://doi.org/10.1016/j.learninstruc.2009.08.006
- Cohen, J., Cohen, P., West, S. G., & Aiken, L. S. (2013). Applied multiple regression/correlation analysis for the behavioral sciences. Routledge.
- Courtney, C., Dutta, S., & Li, Y. (2017). Resolving information asymmetry: Signaling, endorsement, and crowdfunding success. Entrepreneurship Theory & Practice, 41(2), 265–290. https://doi.org/10.1111/etap.12267
- Coussement, K., Debaere, S., & De Ruyck, T. (2017). Inferior member participation identification in innovation communities: The signaling role of linguistic style use. Journal of Product Innovation Management, 34(5), 565–579. https://doi.org/10.1111/jpim.12401
- Deichmann, D., Gillier, T., & Tonellato, M. (2021). Getting on board with new ideas: An analysis of idea commitments on a crowdsourcing platform. Research Policy, 50(9), 104320. https://doi.org/10.1016/j.respol.2021.104320
- DiGangi, P. M., & Wasko, M. (2009). Steal my idea! Organizational adoption of user innovations from a user innovation community: A case study of dell IdeaStorm. Decision Support Systems, 48(1), 303–312. https://doi.org/10.1016/j.dss.2009.04.004
- Di Vincenzo, F., Mascia, D., Björk, J., & Magnusson, M. (2020). Attention to ideas! Exploring idea survival in internal crowdsourcing. European Journal of Innovation Management, 24(2), 213–234. https://doi.org/10.1108/EJIM-03-2019-0073
- Dormann, C. F., Elith, J., Bacher, S., Buchmann, C., Carl, G., Carré, G., Lautenbach, S., Marquéz J. R., Gruber B., Lafourcade B., Leitão P. J., Münkemüller T. (2013). Collinearity: A review of methods to deal with it and a simulation study evaluating their performance. Ecography, 36(1), 27–46. https://doi.org/10.1111/j.1600-0587.2012.07348.x
- Dougherty, D., & Heller, T. (1994). The illegitimacy of successful product innovation in established firms. Organization Science, 5(2), 200–218. https://doi.org/10.1287/orsc.5.2.200
- Drori, I., & Honig, B. (2013). A process model of internal and external legitimacy. Organization Studies, 34(3), 345–376. https://doi.org/10.1177/0170840612467153
- Erickson, D., Holderness, D. K., Jr., Olsen, K. J., & Thornock, T. A. (2022). Feedback with feeling? How emotional language in feedback affects individual performance. Accounting, Organizations and Society, 99, 101329.
- Florén, H., & Frishammar, J. (2012). From preliminary ideas to corroborated product definitions: Managing the front end of new product development. California Management Review, 54(4), 20–43.
- Gutiérrez, E., & Magnusson, M. (2014). Dealing with legitimacy: A key challenge for Project Portfolio Management decision makers. International Journal of Project Management, 32(1), 30–39.
- Heusinkveld, S., & Reijers, H. A. (2009). Reflections on a reflective cycle: Building legitimacy in design knowledge development. Organization Studies, 30(8), 865–886. https://doi.org/10.1177/0170840609334953
- Hoornaert, S., Ballings, M., Malthouse, E. C., & Van den Poel, D. (2017). Identifying new product ideas: Waiting for the wisdom of the crowd or screening ideas in real time. Journal of Product Innovation Management, 34(5), 580–597. https://doi.org/10.1111/jpim.12396
- Hornik, K., & Grün, B. (2011). Topicmodels: An R package for fitting topic models. Journal of Statistical Software, 40(13), 1–30. https://doi.org/10.18637/jss.v040.i13
- Iacobucci, D., Schneider, M. J., Popovich, D. L., & Bakamitsos, G. A. (2016). Mean centering helps alleviate “micro” but not “macro” multicollinearity. Behavior Research Methods, 48(4), 1308–1317. https://doi.org/10.3758/s13428-015-0624-x
- Ivory, S. B. (2013). The Process of Legitimising: How practitioners gain legitimacy for sustainability within their organisation. University of Edinburgh Business School.
- Jensen, M. B., Hienerth, C., & Lettl, C. (2014). Forecasting the commercial attractiveness of user‐generated designs using online data: An empirical study within the LEGO user community. Journal of Product Innovation Management, 31, 75–93. https://doi.org/10.1111/jpim.12193
- Jiang, J., & Wang, Y. (2020). A theoretical and empirical investigation of feedback in ideation contests. Production and Operations Management, 29(2), 481–500. https://doi.org/10.1111/poms.13127
- Jockers, M. (2017). Package ‘syuzhet’. https://cran.r-project.org/web/packages/syuzhet
- Jost, L. (2006). Entropy and diversity. Oikos, 113(2), 363–375. https://doi.org/10.1111/j.2006.0030-1299.14714.x
- Jurka, T. P. (2012). Sentiment, R package. Retrieved January, 2016, from http://www.inside-r.org/packages/cran/sentiment
- Kannan-Narasimhan, R. (2014). Organizational ingenuity in nascent innovations: Gaining resources and legitimacy through unconventional actions. Organization Studies, 35(4), 483–509. https://doi.org/10.1177/0170840613517596
- Kannan-Narasimhan, R. (2014). Organizational ingenuity in nascent innovations: Gaining resources and legitimacy through unconventional actions. Organization Studies, 35(4), 483–509.
- Kijkuit, B., & Van Den Ende, J. (2007). The organizational life of an idea: Integrating social network, creativity and decision‐making perspectives. Journal of Management Studies, 44(6), 863–882. https://doi.org/10.1111/j.1467-6486.2007.00695.x
- Kluger, A. N., & DeNisi, A. (1996). The effects of feedback interventions on performance: A historical review, a meta-analysis, and a preliminary feedback intervention theory. Psychological Bulletin, 119(2), 254. https://doi.org/10.1037/0033-2909.119.2.254
- Kruft, T., Tilsner, C., Schindler, A., & Kock, A. (2019). Persuasion in corporate idea contests: The moderating role of content scarcity on decision‐making. Journal of Product Innovation Management, 36(5), 560–585. https://doi.org/10.1111/jpim.12502
- Larkin, J., McDermott, J., Simon, D. P., & Simon, H. A. (1980). Expert and novice performance in solving physics problems. Science, 208(4450), 1335–1342. https://doi.org/10.1126/science.208.4450.1335
- Lindner, T., Puck, J., & Verbeke, A. (2020). Misconceptions about multicollinearity in international business research: Identification, consequences, and remedies. Journal of International Business Studies, 51(3), 283–298. https://doi.org/10.1057/s41267-019-00257-1
- Masisi, L., Nelwamondo, V., & Marwala, T. (2008, November). The use of entropy to measure structural diversity. 2008 IEEE International Conference on Computational Cybernetics, Stara Lesna, Slovakia (pp. 41–45). IEEE.
- McGarrell, H., & Verbeem, J. (2007). Motivating revision of drafts through formative feedback. ELT Journal, 61(3), 228–236. https://doi.org/10.1093/elt/ccm030
- Mueller, J. S., Melwani, S., & Goncalo, J. A. (2012). The bias against creativity: Why people desire but reject creative ideas. Psychological Science, 23(1), 13–17.
- Nasukawa, T., & Yi, J. (2003). Sentiment analysis: Capturing favorability using natural language processing. Proceedings of the 2nd International Conference on Knowledge Capture, Sanibel Island, FL, USA (pp. 70–77). ACM. https://doi.org/10.1145/945645.945658
- Ogink, T., & Dong, J. Q. (2019). Stimulating innovation by user feedback on social media: The case of an online user innovation community. Technological Forecasting and Social Change, 144, 295–302. https://doi.org/10.1016/j.techfore.2017.07.029
- O’Leary, D. E. (2016). On the relationship between number of votes and sentiment in crowdsourcing ideas and comments for innovation: A case study of Canada’s digital compass. Decision Support Systems, 88, 28–37. https://doi.org/10.1016/j.dss.2016.05.006
- Özaygen, A., & Balagué, C. (2018). Idea evaluation in innovation contest platforms: A network perspective. Decision Support Systems, 112, 15–22.
- Paul, S. A. (2016). Find an expert: Designing expert selection interfaces for formal help-giving. Proceedings of the 2016 CHI Conference on Human Factors in Computing Systems, San Jose, California, USA (pp. 3038–3048). ACM.r
- Piezunka, H., & Dahlander, L. (2015). Distant search, narrow attention: How crowding alters organizations’ filtering of suggestions in crowdsourcing. Academy of Management Journal, 58(3), 856–880.
- Rasoolimanesh, S. M., Wang, M., Roldan, J. L., & Kunasekaran, P. (2021). Are we in right path for mediation analysis? Reviewing the literature and proposing robust guidelines. Journal of Hospitality and Tourism Management, 48, 395–405. https://doi.org/10.1016/j.jhtm.2021.07.013
- Ruiz, É., & Beretta, M. (2021). Managing internal and external crowdsourcing: An investigation of emerging challenges in the context of a less experienced firm. Technovation, 106, 102290.
- Schemmann, B., Herrmann, A. M., Chappin, M. M., & Heimeriks, G. J. (2016). Crowdsourcing ideas: Involving ordinary users in the ideation phase of new product development. Research Policy, 45(6), 1145–1154. https://doi.org/10.1016/j.respol.2016.02.003
- Suchman, M. C. (1995). Managing legitimacy: Strategic and institutional approaches. Academy of Management Review, 20(3), 571–610. https://doi.org/10.2307/258788
- Suddaby, R., & Greenwood, R. (2005). Rhetorical strategies of legitimacy. Administrative Science Quarterly, 50(1), 35–67. https://doi.org/10.2189/asqu.2005.50.1.35
- Tingley, D., Yamamoto, T., Hirose, K., Keele, L., & Imai, K. (2014). Mediation: R package for causal mediation analysis. Journal of Statistical Software, 59(5). https://doi.org/10.18637/jss.v059.i05
- Vatcheva, K. P., Lee, M., McCormick, J. B., & Rahbar, M. H. (2016). Multicollinearity in regression analyses conducted in epidemiologic studies. Epidemiology (Sunnyvale, Calif.), 6(2). https://doi.org/10.4172/2161-1165.1000227.
- Wooten, J., & Ulrich, K. (2017). Idea generation and the role of feedback: Evidence from field experiments with innovation tournaments. Production and Operations Management, 26(1), 80–99. https://doi.org/10.1111/poms.12613
- Zhao, X., Lynch, J. G., Jr., & Chen, Q. (2010). Reconsidering Baron and Kenny: Myths and truths about mediation analysis. The Journal of Consumer Research, 37(2), 197–206. https://doi.org/10.1086/651257
- Zhou, J. (1998). Feedback valence, feedback style, task autonomy, and achievement orientation: Interactive effects on creative performance. The Journal of Applied Psychology, 83(2), 261. https://doi.org/10.1037/0021-9010.83.2.261
- Zhu, H., Kock, A., Wentker, M., & Leker, J. (2019). How does online interaction affect idea quality? The effect of feedback in firm‐internal idea competitions. Journal of Product Innovation Management, 36(1), 24–40. https://doi.org/10.1111/jpim.12442
- Zhu, J. J., Li, S. Y., & Andrews, M. (2017). Ideator expertise and cocreator inputs in crowdsourcing based new product development. Journal of Product Innovation Management, 34(5), 598–616. https://doi.org/10.1111/jpim.12400
- Zimmerman, M. A., & Zeitz, G. J. (2002). Beyond survival: Achieving new venture growth by building legitimacy. Academy of Management Review, 27(3), 414–431. https://doi.org/10.2307/4134387