Abstract
Research into state appropriations across the United States frequently examines the influence of socio-demographic characteristics and political characteristics on particular levels of funding. We extend this literature to examine the year-to-year volatility of funding in order to test hypotheses surrounding the interlocks among multiple actors in the political stage. Our findings suggest that, when state higher education leaders are politically close to governors and have no formal budgetary power they capture more revenue volatility.
Keywords:
1. Introduction
Accurately assessing and predicting future trends in revenue is an important component in prudent financial management across both the for-profit and not-for-profit sectors. However, the importance of revenue predictability extends far beyond the financial management of organizations. Uncertainty surrounding future revenue streams derails organizational management, requiring managers to adjust existing plans to compensate for unexpected shocks that may lead to revenues and expenditures becoming disjointed. This instability can have numerous implications for organizations, including the disruption of effective, efficient and consistent delivery of services. In the context of higher education there exist numerous organizations that in and of themselves are actors in the securing of public revenue vis a vis government appropriations. These organizations, in turn, contain individuals who act on behalf of their organizations as well as particular ideological segments. Noteworthy across the 50 US states is the differing structural arrangements within states as well as the formal and informal powers afforded to particular individuals. Taken as a whole, the states produced a broad and intriguing constellations of interlocks between these multiple actors who lie on multiple levels.
Within the higher education literature, many have examined factors that relate to levels of state support for public higher education, with numerous researchers noting the trend of eroding state appropriations. As many within the field have noted, this, in part, is due to the formal arrangement of public higher education in the US as both a public and private good which is reflected in its funding streams. While on one hand, state governments provide direct appropriations to institutions to subsidize their operations, virtually all states and higher education sectors have the additional revenue stream of tuition dollars. Thus, when faced with declining tax revenues states will more strongly rely on tuition dollars as other public sectors (eg prisons, public secondary schools) lack alternative sources through which they can generate revenue. Recent literature has shown that, despite this arguably rational trend for the erosion of state funding, that there is a strong relationship to levels of appropriations and a state’s political climate (for a review, see Tandberg & Griffith, Citation2013). Despite the continued interest in the topic of state appropriations, little research looks at the stability and predictability of these revenue streams over time, limiting our understanding of the relationship between states, postsecondary institutions and students, particularly in an era of increasing uncertainty. That is, while decreasing state support has profound consequences for postsecondary education, the extent to which higher education leaders and policy-makers can predict the future changes their ability to pre-emptively compensate for future budgetary shortfalls or appropriately plan for potential increases in revenue.
There are a handful of studies outside the field of higher education that point to the potential consequences of revenue volatility in the postsecondary domain. For example, Tuckman and Chang (Citation1991) argue that unanticipated revenue volatility forces managers to shift focus away from long-term planning and towards shorter term, day-to-day management, thereby creating a pernicious Catch-22: the uncertainty of revenue streams inhibits the ability of the organization to focus on long-term planning, while the inability to focus on long-term planning inhibits the organization’s ability to compensate for volatile revenue streams in a dynamic manner. As policy-makers and the public continue to question public higher education’s role in society and the economy, this vicious cycle may have serious implications for the ability of our public systems of higher education to meet evolving public demands. Public postsecondary education, however, is not a passive bystander in this process and through formal and informal political processes may have opportunities to shape the stability surrounding the state appropriations it receives.
We address this gap through an exploration of the volatility in state appropriations for higher education both across states and over time from a multi-actor perspective. Drawing on a unique panel of state-level variables covering all states from 1985 to 2009, we find that, although volatility in appropriations to postsecondary education is powerfully predicted by overall volatility in state revenue streams and economic conditions, other factors can either inhibit or promote stability and even enable higher education agencies to ‘capture’ volatility. More specifically, we find that interplay between the agency’s executive officer and the governor and the former’s lack of ability to set the agenda through budgetary recommendation.
2. The impact of revenue volatility
Delaney and Doyle (Citation2007, 2011) provide theoretical and empirical evidence linking economic conditions to state higher education funding. Their ‘Hovey Hypothesis’ – named for public finance and state policy scholar Harold Hovey – posits that higher education essentially plays the role of a residual claimant with respect to public coffers: in good economic times, higher education is generously supported; conversely, when economic conditions sour, higher education receives aggressive cuts (Hovey, Citation1999). The authors’ analysis documents this powerful relationship between state economic cycles and state higher education funding – further, they also demonstrate that these boom–bust cycles in appropriations are qualitatively and quantitatively unique to higher education as compared to other public expenditure categories.
A rich descriptive and empirical literature documents the historical trend in decreasing levels of state support for higher education (support that is measured variously as appropriations per full-time equivalent (FTE) student, appropriations per capita, or appropriations as a percentage of total state spending). A good deal of scholarly and public discourse has addressed both the causes of these declines as well as the implications they hold for the performance of public institutions of higher education. This attention is certainly warranted as economic prosperity (considered at the individual, state, or national level) has become increasingly tied to educational attainment. However, we contend that the overall funding level, though important, is only one of many potentially important metrics that capture the adequacy of state funding. This is a point famously made by Groves and Kahn (Citation1952) in their seminal study of state and local tax yields. Analysing the stability of the revenue streams upon which state and municipal governments depend, the authors argue that considering stability as a component of adequacy forces one to think about adequacy as not a static but dynamic concept that considers both today and tomorrow.
Adding this additional dimension to adequacy necessarily shifts the focus away from simply considering the level of appropriations in aggregate and towards the incorporation of the volatility in appropriations as well. When revenues are moving steadily (regardless of direction), university administrators face less uncertainty in formulating budgets, developing strategies to achieve longer term goals and making decisions about which types and how much of particular services to offer. Conversely, volatility in revenues – represented as unexpected deviations from these long-term trends – induce administrators to focus away from the longer and on to the shorter term:
when financial conditions become tight, the time horizon that institutional stakeholders are willing to consider shrinks. Administrators become focused on the next few months (‘how will this affect the institution?’) rather than the longer term (‘what can this institution become?’). (Lasher & Sullivan, Citation2004, p. 200)
In other words, it is reasonable to suspect that unanticipated year-to-year changes may have distinct impacts as compared to more predictable longer term decreases in funding levels. Further, failure to distinguish between the two may lead to incorrect inferences about their respective effects.
Research on public and not-for-profit organizations provides empirical evidence of the potential negative consequences of revenue volatility. Much of this literature focuses on revenue diversification strategies to manage volatility, studies that are broadly grounded in modern portfolio theory. Tuckman and Chang (Citation1991) argue that financially stable organizations – defined in part by the diversity of revenues upon which the organization relies – are more mission-focused and less likely to have to reduce services unexpectedly or face the more serious threat of closure. Yan (Citation2011) argues that the benefits of diversification are contingent upon the nature of the economic base(s) upon which the organization relies, thereby providing a more nuanced approach to evaluating the stability benefits of diversification. Carroll and Stater (Citation2009) find that revenue volatility decreases as the revenue portfolio of not-for-profit organization becomes more diverse, although they caution that external environments and other industry-specific exogenous factors may trump the ability of prudent financial management to promote organizational stability and longevity through diversification of revenues.
This last point is particularly relevant to the study of public higher education as we consider likely avenues through which institutions can manage revenue volatility. Scholars of higher education finance note a trend of increased reliance on tuition and fee revenue (and other revenue sources such as external research grants or alumni donations) as public appropriations have decreased (eg Bok, Citation2004; Cheslock & Gianneschi, Citation2008; Slaughter & Leslie, Citation1997; Slaughter & Rhoades, Citation2004; State Higher Education Executive Officers [SHEEO], Citation2012). In this sense, institutions may intentionally pursue other streams of revenue to minimize the reliance on public appropriations, and thereby, avoid the negative consequences of revenue shocks. Further, endowment income or deficit financing can, in theory, stabilize revenues and overcome short-term financial volatility, much the same as an individual can draw on savings or credit in hard times.Footnote1 However, the extent to which these solutions can effectively mitigate shorter term revenue volatility in practice may be limited. In many states, the ability of institutions to unilaterally raise tuition and/or fee prices is curtailed, either officially by statute or unofficially by the nature of state politics, and the infrastructure needed to shift to a funding model that relies more heavily on research grants and other external dollars is not only costly to develop, but counter to the core missions of many public institutions. Empirical evidence from the recent financial downturn indicates that in many cases, endowment spending rules and hesitancy on the part of administrators to spend down endowment principal meant that these funds were largely ineffective in balancing unanticipated shortfalls, especially given that endowment earnings were negatively influenced by the same changes in economic conditions driving state budgetary shortfalls themselves (Commonfund Institute, Citation2010). Further, the median public institution’s endowment assets are simply not of sufficient size to provide much practical relief and many public institutions lack direct access to credit markets (Moody, Citation2008). As Breneman laments, it seems that ‘the states and higher education are locked into an entirely predictable and repetitive dance, with few signs that we are learning how to avoid its worst features’ (Citation2002, p. B8). A germane question – and the one motivating our analysis – is a simple one: what factors explain the observed volatility in state appropriations for higher education across states and over time?
3. Governors, governance and state higher education finance
In seeking an answer to this question, we note that recent scholarship has decisively shown that state systems of public higher education – and the individual institutions that comprise these systems – are influenced by state political dynamics. The nature of this relationship is evident in the original creation and chartering of public institutions: Glenny (Citation1959), for instance, notes that ‘The successful politician proved his merit by bringing home an insane asylum, a prison, or a college. Frequently it made little difference which’ (p. 13). Fast forwarding to more recent times, an emerging literature demonstrates the continued influence of politics on how public institutions are funded and managed.
Contemporary studies in the area of state higher education finance and policy scholarship frequently employ a ‘new institutionalist’ perspective. New institutionalism is, as March and Olsen (Citation1984) assert, ‘an argument that the organization of political life makes a difference’ (p. 747); institutions, government and political life define the goals, meaning and actions of individuals who are interacting within governments and therefore impact the decisions and outputs of governments. Shepsle (Citation1989) explains new institutionalism in this way:
Like the rational choice theories that preceded them, and in contrast to the older institutional traditions … these efforts are equilibrium theories. They seek to explain characteristics of social outcomes on the basis not only of agent preferences and optimizing behavior, but also on the basis of institutional features. (p. 135)
This perspective has been used to explain state political actors’ higher education policy decisions (e.g. Cornwell, Mustard, & Sridhar, Citation2006; Doyle, McLendon, & Hearn, Citation2010; McLendon, Deaton, & Hearn, Citation2007; McLendon, Hearn, & Deaton, Citation2006; McLendon, Heller, & Young, Citation2005; McLendon, Mokher, & Flores, Citation2011). It has also been used to explain the level of state support of higher education (eg Dar & Spence, Citation2011; McLendon, Hearn, & Mokher, Citation2009; Nicholson-Crotty & Meier, Citation2003; Rizzo, Citation2006; Tandberg, Citation2010a, 2010b, 2013; Weerts & Ronca, Citation2006). The results of these studies lead scholars to adopt a new perspective on the factors associated with state support of higher education that move beyond theories that argue that differences are driven entirely by economic and higher education contextual factors and instead adopt a multi-actor, multi-level perspective and recognize the potential impact of various political and governmental institutions and other political characteristics of the states. These researchers demonstrate that there are multiple actors and conditions that can influence state appropriations; partisanship, ideology, various attributes of the legislative branch, variations in higher education governance arrangements and the makeup of state interest group ecologies, among other things, have significant and predictable impacts on the level of state support of higher education.
Along these lines, a long-standing vein of literature in political science highlights the role of the state governor as one important actor in setting state policy agendas and influencing state spending priorities (Barrilleaux & Berkman, Citation2003; Beyle, Citation1996; Hendrick & Garand, Citation1991). Some recent scholarship has sought to apply these broader ‘power of the governor’ measures to higher education finance. McLendon et al. (Citation2009) find that gubernatorial power over the state budget to be negatively related to state appropriations per $1000 of personal income, arguing that governors who possess greater budget authority often use their power as a check against legislative spending (see also Bails & Tieslau, Citation2000; Barrilleaux & Berkman, Citation2003; Beyle, Citation1996; Dearden & Husted, Citation1993). Several studies also suggest that the party affiliation of the governor matters in the budgeting process for higher education (eg Archibald & Feldman, Citation2006; McLendon et al., Citation2009; Tandberg, Citation2008, 2010a, 2010b). These authors generally find that Democratic governors are more generous to higher education as compared to their Republic counterparts.
However, to date, these streams of literature largely ignore an additional state actor – the SHEEO, who is the leader of the statewide agency charged with oversight and management of the state’s system for public higher education. Scholars of state politics have long noted the importance of bureaucratic appointments as a mechanism through which governors can exert control over the direction of state administrative agencies (Beyle, Citation1968; Dometrius, Citation1979; Wright, Citation1967); in fact, surveys of governors reveal that most regard appointive authority as their single most important power (Beyle, Citation1983). A cursory glance across the states reveals a surprising (and to date largely unacknowledged) degree of heterogeneity in this regard with respect to higher education: in some states, the governor unilaterally appoints a majority of the members of the state’s postsecondary agency; in others, the members are determined through direct election or legislative appointment. Even in those states with gubernatorial appointment, differences exist in terms of whether appointments are subject to legislative confirmation. Similar differences exist across the states with respect to the SHEEO whose influence and power is constrained by the structure and power of the agency he leads as well as his relationship to other actors in state government. For example, in some states, that position is filled by, and serves exclusively at the pleasure of, the governor. In others, the SHEEO is chosen by the agency’s members or legislatively. Finally, in some states, the governor him or herself is a full member of the agency’s board; in others, the governor is an ex-officio member without voting privileges or is wholly excluded from formal membership. While an institutionalist perspective suggests that these differences may have very important ramifications for state higher education policy and finance, to date no literature of which we are aware has sought to explore how these dynamics impact outcomes in practice.
In considering the impact of the appointive powers of governors on state budgetary and policy outcomes for public higher education, it is also critical to consider the relative position occupied by the state postsecondary agency itself as another important actor on the state higher education funding stage. In comparison to the structures that have oversight over primary and secondary education, postsecondary governance structures exhibit a wide degree of variation in both their organization, composition and the powers delegated to them to oversee, manage and control the individual institutions under their purveyance. On one extreme, some states have adopted highly decentralized models in which the state postsecondary agency oversees minimal compliance and planning and, perhaps, the administration and distribution of state financial aid programs. On the other extreme, some states have created large, centralized bureaucratic agencies that approve and review all academic programs, set admission standards, hire and fire the presidents of the individual public universities and directly control institutional financial activities such as budgeting and even purchasing.
A recent but growing body of literature finds that the way a state arranges its higher education governance matters for both higher education policy and finance (see Tandberg and Griffith (Citation2013) for a concise review of this literature). While the first ‘wave’ of scholarly research in this area simply included a measure of governance as an independent variable, more recent scholarship suggests that the influence of governance is not direct; rather, governance influences outputs and outcomes by moderating the effect of other key variables and actors. This indirect impact was pointed out by Nicholson-Crotty and Meier (Citation2003), whose study of higher education governance concludes that future research needs to explore ‘what kinds of political forces are mediated [or enabled] by the various structures and how in combination these affect higher education policy’ (p. 95). Tandberg (Citation2013) responds to this call, finding that consolidated governing boards exert a ‘conditioning effect’ on the influence of state political actors (including legislatures, interest groups and the governor) on state higher education spending.
Broadly, our focus here is to combine these two distinct streams of research, thereby developing a better understanding of the interrelationships between various actors and specifically structure and political control and funding stability. Numerous higher education policy scholars argue that higher education governance serves as a boundary-spanning organization – transmitting the preferences of the public to the institutions and the interests of the institutions to the public (Glenny, Citation1959, 1970; Hearn & Griswold, Citation1994; McLendon, Citation2003; Ness, Citation2010; SHEEO, Citation2009; Tandberg, Citation2013). But which role dominates? Does governance function primarily to ‘facilitate [political] access’ (consistent with the first role) or ‘create autonomy’ (consistent with the second) (Nicholson-Crotty & Meier, Citation2003)? Drawing on the theoretical and empirical literature cited above, we posit that structure, political control and power are three key dimensions which come together to explain the orientation of a particular organization.
4. Theoretical model, data and method
To test this supposition, we developed a unique data-set that is comprised of a variety of state-level variables covering 49 states for the years 1985–2009 (Nebraska is excluded due to its unique unicameral legislature). The specifics of the variables included in the analysis are included below.
4.1. Dependent variable
Our dependent variable of interest is the volatility in state higher education appropriations. To capture volatility, we follow the approach detailed by Carroll (Citation2009), Carroll and Stater (Citation2009) and White (Citation1983), who argue that revenue volatility is defined as the difference between expected revenue and actual revenue. Accordingly, we straightforwardly posit that the annual amount appropriated for higher education in each state in each year (yit) is a function of two factors: a long-term, predictable trend () and a cyclical component (eit) which captures the impact of short-term, exogenous and temporary economic shocks due to fluctuations in the business cycle and the like. Equation (Equation1
(1) ) denotes this relationship:
(1)
We analytically distinguish these two components by applying the Hodrick–Prescott (HP) filter to the natural logarithm of real state higher education appropriations (Hodrick & Prescott, Citation1997). A simpler approach would be to simply estimate an OLS regression fitting a linear time trend to each state’s appropriation data and use the regression fitted values and residuals as estimates of the time trend component and the cyclical variation, respectively. However, this approach presumes that the time trend itself is time-invariant. If the time trend exhibits medium run changes (implying that the slope of the trend line is not constant over time), the simple OLS linear trend estimates would produce spurious estimates of cyclical variations since those estimates would be confounded by the changes in the trend line slope as they may occur over time. Accordingly, we instead utilize the HP filter, which is the ‘standard method for removing trend movements in the business cycle literature’ (Ravn & Uhlig, Citation2002). It estimates the trend parameter () for each i state through penalized optimization of the function in the following equation:
(2)
As this equation demonstrates, the HP filter extracts the time trend from each state’s annual appropriation by minimizing the sum of squared deviations of yit from plus the sum of the squared second differences, the latter of which are weighted by a predetermined smoothing parameter,
. It is this second term that allows the HP filter to explicitly penalize for the variability in the underlying growth trend, with a penalty that increases in magnitude as
increases. If
is set to zero, the extracted trend is deterministically set equal to the observed data, and, accordingly, cyclical variability does not exist. Conversely, as the value assigned for
approaches infinity, the HP trend estimates approach the linear time trend model. Since our data are measured annually, we set
as suggested by Ravn and Uhlig (Citation2002), although we note that following other conventions in the literature for setting
yield no substantive differences in our empirical results
Differencing the HP-estimated trend parameter from the actual observed value in each year () provides our estimates of eit, which we scale by dividing it by the estimated trend parameter, thereby facilitating comparison across states with differing absolute levels of public appropriations. To provide a sense of our specifications of volatility, Figure presents the absolute value of the average volatility by state.
Figure 1. Scatterplot of average state general own-source revenue volatility and volatility in state higher education appropriations (absolute value presented).
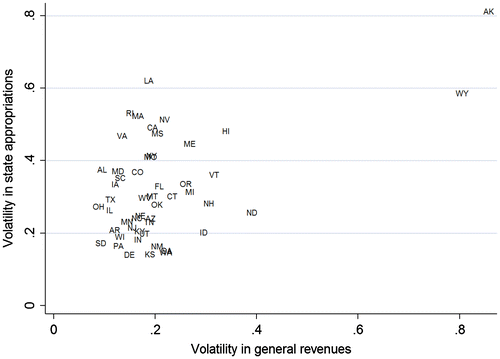
Do note that the model does not utilize the absolute value, however it is used in this presentation to illustrate which states are the most unstable in their year-to-year funding. Of note is that Alaska (AK), Wyoming (WY) and Louisiana (LA) are among the most volatile states and are three states with economies heavily reliant on the natural resource industries.
4.2. Independent variables
Our selection of independent variables recognizes both the demographic and economic conditions we posit could contribute to volatility but also the multiple actors (both organizational and individual) who are part of the process. Figure illustrates the broad categories outlined by Tandberg (Citation2010b) which we expand for this study. Based on the research hypotheses specified above, our empirical analysis focuses on three key variables and their interaction. While the interaction term is a literal, mathematical operation in our model, it is used to capture the connection between multiple conditions states may have among the relationship between the governor and the state SHEEO and the latter’s power.Footnote2
Figure 2. State fiscal policy framework for higher education. Source: Adapted from Tandberg (Citation2010b); Figure 2; With permission of Springer, Copyright © 2010.
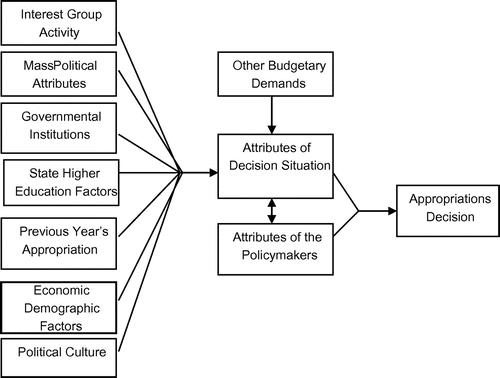
The first term we include in our interaction is the ability of governor to appoint a SHEEO. This variable, we argue, captures the linkage between the chief executive of the entire state and the leader of postsecondary education in the state. On one hand, when these two are connected through appointments, we can envision the SHEEO to be a more politicized actor and subordinate to the governor while, on the other, we can see scenarios in which this connection allows for the development of coalitions between the gubernatorial branch and the postsecondary sector.
Next we have an indicator to measure whether or not the SHEEO is able to recommend a budget. This proxies the theoretical belief that the SHEEO’s ability to recommend a budget is a powerful agenda setting tool that can shape policy outcomes as well as measuring the formal power held by this actor.
Our principal variable of interest is a term that interacts whether or not the governor appoints the SHEEO and the SHEEO’s ability to recommend a budget. This interaction permits the effect of the governor’s power over the agency with the SHEEO’s ability to influence the budget-making process. As noted previously, this is a formal way to specify the influence across these three actors across states, thus capturing the potential differences in outcomes according to the unique configurations of these attributes as observed across the individual states.
To capture variation among higher education agencies across states and time, we include a variable measuring the level of centralization of the state’s postsecondary governance agency. While studies frequently use a dummy variable indicating whether a state possesses a consolidated governing board, we utilize a new, continuous measure that places all state structures on a ‘continuum of centralization’, thereby permitting a higher degree of variance in measurement than is possible with the binary variable.Footnote3 Additional utility of this measure for researchers is its ability to extend the relationship of centralization beyond the typically used binary indicator for ‘Consolidated Governing Board’ as well as its ability to be used as a polynomial, which yields potential new insights into the relationships between postsecondary governance and broad state outcomes. Figure , provides this measure by state in 2009, the final year of our analysis.
We also include a measure of the volatility of state own-source general revenue, a variable calculated utilizing the same process discussed above for capturing volatility in higher education appropriations. Certainly, based on the above discussion, we expect that state revenue shocks should be associated with volatility in appropriations. Further, we include the one year lag of this variable to account for the potential impact of the budget cycle on the relationship between these two variables as well as the biennial budgetary process used by some states.
Based on the extant literature and our adaptation of Tandberg’s (Citation2010a) framework, we also include variables to control for relevant state political, socio-economic and demographic variables. First, building on the work of McLendon et al. (Citation2007), we include variables capturing partisan turnover in the state legislature and the governor’s office, as well as a variable capturing divided partisan control of state government. All else equal, we might posit that increased political competition and turnover may yield higher volatility in appropriations.
We also include an indicator that measures the governor’s power in the budgetary process. Specifically, we utilize the index created by Krupnikov and Shipan (Citation2012) that extends the well-known Formal Powers Index developed by Thad Beyle (2003). Their work includes: (1) the governor’s ability to spend federal funds without legislative approval, (2) the governor’s line-item veto power, (3) the governor’s power to reorganize departments related to the budget without legislative approval, (4) the governor’s ability to reduce the budget without legislative approval and (5) whether the governor has authority for budget preparation (Krupnikov & Shipan, Citation2012, p. 446). A limitation of this index is that it is not measured annually. To overcome this limitation, we carry forward the most immediate previous year’s values to impute values for the missing years.
Legislative professionalism has a significant and positive impact on state support of higher education measured a number of different ways. (e.g. McLendon et al., Citation2009; Nicholson-Crotty & Meier, Citation2003; Peterson, Citation1976; Tandberg, Citation2008, 2010a, 2010b; Tandberg & Ness, Citation2011). Developed by political scientist Peverill Squire in 1992, legislative professionalism is an index that uses three components to capture the degree of institutional resources invested in the legislature (salary and benefits, time demands of service and staff and resources) (Squire, Citation1992, Citation2000, Citation2007). At its core, the measure attempts to help explain the extent to which public service in a given state has the infrastructure to which it may make informed choices. There is substantial variation across states in terms of the professionalism of legislatures, which makes the variable quite useful for empirical analyses. Legislative professionalism has been linked with higher public spending generally (Squire & Hamm, Citation2005) and, as indicated earlier, increases in spending for higher education, thus its inclusion in our model.
We also include several variables to capture other relevant economic characteristics of the states as well as relevant characteristics of the state system for higher education. First, we include state median household income and the state unemployment rate. All else equal, we might expect that states with lower median wealth and higher unemployment to have more volatility in support for higher education. Finally, we include the log of total state public FTE enrollment and state higher education appropriations per capita. Holding all else constant, we would expect states with larger enrollments and more generous support would have lower levels of volatility.
Table provides descriptive statistics variables included in the analysis.
Table 1 Descriptive Statistics, standard deviation in parentheses.
4.3. Method
Because our data are states over time, our analysis employs a standard two-way fixed effect model specified as:(3)
where i and t index individual states and years. A is the volatility in state higher education appropriations and our dependent variable of interest. The next two parameters (β1, β2,); G is an indicator set to one if the state’s governor appoints the SHEEO; S an indicator variable set to 1 if the SHEEO is able to recommend the budget. The parameter β4 is the technical specification of the interaction which, said plainly requires the multiplication of our two terms of interest.
Outside of these key variables of interest, the rest of the equation R and its parameter β5 captures the volatility in state revenues from the prior year, hence the subscript of t − 1; P and D represent vectors of the political and socioeconomic and demographic controls mentioned above and the parameters associated with them; and e is the idiosyncratic error term. We also employ state (u) and year (v) fixed effects to capture the impact of any unobserved, time-invariant state characteristics and common exogenous shocks, respectively, although for parsimony we do not report these sets of coefficients in our results. Finally, we cluster our standard errors on the individual states under the assumption that observations of a given state over time are unlikely to be independent (Primo, Jacobsmeier, & Milyo, Citation2007). We also with to highlight that the fixed-effect model creates parameters that should be interpreted as changes within states as opposed to capturing between-state variation. That is, the coefficients should be conceived of the effect of a variable changing for a given state.Footnote4
5. Results
The results of estimating Equation (Equation3(3) ) are presented in Table below. Model 1 presents the model without the interaction while Model 2 presents our fully interacted model. Although the coefficient on the interaction term is highly statistically significant, we caution the reader from drawing inferences based solely on the reported parameters in Table (Brambor, Clark, & Golder, Citation2006), results which we will discuss.
Table 2 Results from the base and fully interacted models.
While our interacted parameter is statistically significant, the combined effect of the three parameters is not different from zero, with the governor appointing the SHEEO and the interaction term effectively washing each other out. However, what the model does demonstrate is that when SHEEO’s are closely aligned with the governor but have little authority themselves over budgets they are better able to capture state volatility. To us this echoes the multi-level and multi-actor theme of the issue in that there is a structural component across states that leads to the particular actor combinations wherein the governor can appoint the SHEEO yet the latter has no structural authority.
In addition to this, we find that gubernatorial control change, state higher education appropriations, and median house hold income positively relate to capturing volatility while legislative professionalism has a negative influence. We find the negative effect of legislative professionalism particularly notable and an area for future research that explores which sectors may capture volatility as legislative professionalism increases.
We also find that, while current year state own-source general revenue volatility does not have a significant impact on the volatility of higher education appropriations, prior year own-source general revenue volatility does. These findings support our original hypothesis that state revenue shocks would be associated with volatility in appropriations, however our findings suggest that these effects are delayed – likely due to the biennial nature of the budget cycle of many states. Finally, we find that increased student enrollments decrease volatility in appropriations. Often state appropriations are tied in either explicitly (often through state funding formulas) or implicitly to student enrollments. High public enrollments then also provide a measure of stability. While at first blush increasing enrollments may be a strategy employable by institutions, from a statewide and system view this results in a zero sum game. It may be that in many of these states the high enrollments proxy the centrality of postsecondary education among the state’s public enterprises.
6. Discussion & conclusion
Agenda setting in comparative state research frequently focuses on the governor’s ability to utilize the bully pulpit and shape discussion of state policies, a perspective that is also applied in research on higher education. Our results suggest that this process is entwined with the SHEEO’s relationship to the governor. That is, our results point toward the importance of multi-actor processes as this effect exists when the governor appoints the SHEEO and the latter has no less formal budgetary authority – indicating that not just actors but the power constraints surrounding them shape policy.
Research documents the shift in public higher education from a reliance on state appropriations to an increasing reliance on other revenue sources (Cheslock & Gianneschi, Citation2008; SHEEO, Citation2012). It is certainly possible that as public institutions observe increased volatility they may seek to stabilize their overall revenue through an increased reliance on other forms of revenue, most likely tuition and fees, which provide the most reliable form of institutional revenue. Therefore increased volatility may have exacerbated the rapid increases observed in cost of higher education over the last several decades.
However, research shows that decreases in levels in state appropriations limit the ability of institutions to seek out other forms of revenue beyond tuition and fees (Cheslock & Gianneschi, Citation2008). This has been described as the ‘Matthew Effect’, a phenomenon in which more is given to those possessing an initial advantage. The initial advantage gained from increased state appropriations assists institutions in seeking additional revenue in the form of private giving or grants and contracts (Cheslock & Gianneschi, Citation2008; Merton, Citation1973; Trow, Citation1993; Winston, Citation1999). As revenue volatility negatively impacts organizations’ abilities to plan strategically and utilize resources efficiently, it is not difficult to imagine that volatility in state appropriations would make seeking alternative forms of revenue difficult for public colleges and universities. That is, increased volatility may both drive institutions to seek other forms of revenue and limit their ability to do so effectively. These issues should be considered in greater depth in future research.
Another possible consequence of revenue volatility, relating directly to institutions in ability to engage in long term planning, may be the increased use of part-time and other non-tenure track faculty. Observers of colleges and universities and higher education researchers have been concerned about the increase usage of part-time and other non-tenure track faculty members. These hiring patterns have been seen as efforts to save money and to deal with uncertain environments. As one study concludes, higher education institutions ‘actively design and adopt contingent work arrangements to save on labor costs and to manage their resource dependence’ (Liu & Zhang, Citation2007, p. 22). In the face of uncertain resources institutions may choose to hire faculty members who are easily let go and who cost less to hire in the first place. However, there is some evidence that the increasing reliance on part-time and other non-tenure track full-time faculty members has negative implications for student achievement and institutional performance. For example two studies have shown that relying on adjunct faculty members increases college dropout rates (Bettinger & Long, Citation2006; Ehrenberg & Zhang, Citation2005). Another study showed that adjunct and teaching assistants generally reduce subsequent student interest in a subject relative to full-time faculty members (Bettinger & Long, Citation2004). Future research ought to specifically explore the possibility that volatility in state appropriations may, in part, be driving the increased use of part-time and other non-tenure track faculty. These are only a few of the potential fruitful avenues for further research.
Returning to the theme of multiple state actors, since the conclusion of our data-set in 2009, policy-makers in several states have tweaked, modified, or in some cases, wholly reinvented their governance of higher education. Simultaneously states have increased the number of SHEEOs from outside of academia, instead turning to politics and industry to lead these agencies. Perhaps even more notable are recent, highly publicized gubernatorial involvement in management of public higher education in Ohio and New Jersey which resulted in the executive offices gaining a perhaps unprecedented level of control. Although the results presented here suggest some potential longer term ramifications for these efforts, systematic scholarly evidence on these topics is lacking. While it remains to be seen how public higher education will adapt to these new challenges, the implications for access, affordability and quality necessarily means that these are topics worthy of future research.
Disclosure statement
David Tandberg works for the State Higher Education Executive Officers association which is a membership organization made up of state higher education executive officers from all 50 states and Puerto Rico. These results are solely the authors’ independent research.
Notes
1 Other examples include universities’ embrace of technology transfer, copyright protection and explicit contractual relationships with the private sector.
2 Initial specifications interacted these two variables with our measure of agency centralization, however, very few states experience a change on all three components. In contrast, there were eleven changes in this relationship across time.
3 Specifically, this measure uses a Bayesian latent variable model with fully informed priors. The 19 items in the model focus on a governing body’s coverage over public institutions, programmatic and budgetary powers and the informed priors utilize the pre-existing typology of planning agency, coordinating board and consolidated governing board. They are listed in Appendix 1. For additional details, see Lacy (Citation2011).
4 For an introductory yet thoughtful discussion on the nuances of fixed and random effects (and their within and between interpretations) we direct readers to Chapter 9 of Gelman and Hill (Citation2007).
References
- Archibald, R. B., & Feldman, D. H. (2006). State higher education spending and the tax revolt. The Journal of Higher Education, 77, 618–644.
- Bails, D., & Tieslau, M. A. (2000). The impact of fiscal constitutions on state and local expenditures. The Cato Journal, 20, 255–277.
- Barrilleaux, C., & Berkman, M. (2003). Do governors matter? Budgeting rules and the politics of state policy making. Political Research Quarterly, 56, 409–417.10.1177/106591290305600403
- Bettinger, E., & Long, B. T. (2004). Do college instructors matter? The effects of adjuncts and graduate assistants on students’ interests and success ( Working Paper No. 10370). NBER. http://www.nber.org/.
- Bettinger, E., & Long, B. T. (2006). The increasing use of adjunct instructors at public institutions: Are we hurting students? In R. Ehrenberg (Ed.), What’s happening to public higher education (pp. 51–69). Westport, CT: Greenwood Press for the American Council on Education.
- Beyle, T. L. (1968). The governor’s formal powers: A view from the governor’s chair. Public Administration Review, 540–545.
- Beyle, T. (1996). Governors: The middlemen and women in our political system. In V. Gray & H. Jacobs (Eds.), Politics in the American States: A comparative analysis (6th ed., pp. 207–252). Washington, DC: CQ Press.
- Beyle, T. L. & Muchmore, L. R. (1983). Being governor: The view from the office. Durham, NC: Duke University Press.
- Bok, D. (2004). Universities in the marketplace: The commercialization of higher education. Princeton, NJ: Princeton University Press.
- Brambor, T., Clark, W. R., & Golder, M. (2006). Understanding interaction models: Improving empirical analyses. Political Analysis, 13, 63–82.
- Breneman, D. W. (2002). For colleges, this is not just another recession. The chronicle of higher education, 48, B7–B9.
- Carroll, D. A. (2009). Diversifying municipal government revenue structures: Fiscal illusion or instability? Public Budgeting & Finance, 29, 27–48.10.1111/pbaf.2009.29.issue-1
- Carroll, D. A., & Stater, K. J. (2009). Revenue diversification in nonprofit organizations: Does it lead to financial stability? Journal of Public Administration Research and Theory, 19, 947–966.10.1093/jopart/mun025
- Cheslock, J., & Gianneschi, M. (2008). Replacing state appropriations with alternative revenue sources: The case of voluntary support. The Journal of Higher Education, 79, 208.10.1353/jhe.2008.0012
- Commonfund Institute. (2010). 2009 NACUBO-commonfund study of endowments. Wilton, CT: Author.
- Cornwell, C., Mustard, D. B., & Sridhar, D. J. (2006). The enrollment effects of merit-based financial aid. Journal of Labor Economics, 24, 761–786.10.1086/506485
- Dar, L., & Spence, M. J. (2011). Partisanship, political polarization, and state budget outcomes: The case of higher education. SSRN eLibrary. Retrieved from http://ssrn.com/abstract=1577365
- Dearden, J. A., & Husted, T. A. (1993). Do governors get what they want?: An alternative examination of the line-item veto. Public Choice, 77, 707–723.10.1007/BF01047990
- Delaney, J. A., & Doyle, W. R. (2007). The role of higher education in state budgets. In K. M. Shaw & D. E. Heller (Eds.), State postsecondary education research: New methods to inform policy and practice (pp. 55–76). Sterling, VA: Stylus Publishing LLC.
- Delaney, J. A., & Doyle, W. R. (2011). State spending on higher education: Testing the balance wheel over time. Journal of Education Finance, 36, 343–368.
- Dometrius, N. C. (1979). Measuring gubernatorial power. The Journal of Politics, 41, 589–610.
- Doyle, W. R., McLendon, M. K., & Hearn, J. C. (2010). Why states adopted prepaid tuition and college savings programs: An event history analysis. Research in Higher Education, 51, 659–686.10.1007/s11162-010-9173-4
- Ehrenberg, R. G., & Zhang, L. (2005). Do tenured and tenure-track faculty matter? Journal of Human Resources, XL, 647–659.10.3368/jhr.XL.3.647
- Gelman, A., & Hill, J. (2007). Data analysis using regression and multilevel/hierarchical models (Vol. 1). New York, NY: Cambridge University Press.
- Glenny, L. A. (1959). Autonomy of public colleges: The challenge of coordination. New York: McGraw-Hill.
- Glenny, L. A. (1970). Institutional autonomy for whom? In G. K. Smith (Ed.), The troubled campus: Current issues in higher education (pp. 153–163). Washington, DC: AAHE.
- Groves, H. M., & Kahn, C. H. (1952). The stability of state and local tax yields. The American Economic Review, 42, 87–102.
- Hearn, J. C., & Griswold, C. P. (1994). State-level centralization and policy innovation in U.S. postsecondary education. Educational Evaluation and Policy Analysis, 16, 161–190.
- Hendrick, R. M., & Garand, J. C. (1991). Expenditure tradeoffs in the US states: A pooled analysis. Journal of Public Administration and Theory, 1, 295–318.
- Hodrick, R. J., & Prescott, E. C. (1997). Postwar US business cycles: An empirical investigation. Journal of Money, Credit and Banking, 29, 1–16.
- Hovey, H. A. (1999). State spending for higher education in the next decade: The battle to sustain current support. San Jose, CA: California State Policy Research.
- Kane, T. J., Orszag, P. R., & Gunter, D. L. (2003). State fiscal constraints and higher education spending: The role of Medicaid and the business cycle. Washington, DC: Brookings Institution.
- Krupnikov, Y., & Shipan, C. (2012). Measuring gubernatorial budgetary power: A new approach. State Politics & Policy Quarterly, 12, 438–455.10.1177/1532440012453591
- Lacy, A. (2011). Measuring state postsecondary governance: Developing a new continuum of centralization ( Unpublished doctoral dissertation). University of Georgia, Athens, Georgia.
- Lasher, W., & Sullivan, C. (2004). Follow the money: The changing world of budgeting in higher education. In J. C. Smart (Ed.), Higher education: Handbook of theory and research (Vol. 19, pp. 197–240). Norwell, MA: Kluwer Academic.
- Liu, X., & Zhang, L. (2007). What determines employment of part-time faculty in higher education institutions? ( WP105). Cornell Higher Education Research Institute (CHERI). Unnecessary, part of Cornell Digital Commons: http://digitalcommons.ilr.cornell.edu/cgi/viewcontent.cgi?article=1120&context=workingpapers
- March, J. G., & Olsen, J. P. (1984). The new institutionalism, organizational factors in political life. American Political Science Review, 78, 734–749.
- McLendon, M. K. (2003). State governance reform of higher education: Patterns, trends, and theories of the public policy process. In J. Smart (Ed.), Higher education: Handbook of theory and research (Vol. 18, pp. 57–143). London: Kluwer.10.1007/978-94-010-0137-3
- McLendon, M. K., Deaton, S. B., & Hearn, J. C. (2007). The enactment of reforms in state governance of higher education: Testing the political instability hypothesis. The Journal of Higher Education, 78, 645–675.10.1353/jhe.2007.0041
- McLendon, M. K., Hearn, J. C., & Deaton, R. (2006). Called to account: Analyzing the origins and spread of state performance-accountability policies for higher education. Educational Evaluation and Policy Analysis, 28(1), 1–24.
- McLendon, M. K., Hearn, J. C., & Mokher, C. G. (2009). Partisans, professionals, and power: The role of political factors in state higher education funding. The Journal of Higher Education, 80, 686–713.10.1353/jhe.0.0075
- McLendon, M. K., Heller, D. E., & Young, S. P. (2005). State postsecondary policy innovation: Politics, competition, and the interstate migration of policy ideas. The Journal of Higher Education, 76, 363–400.10.1353/jhe.2005.0029
- McLendon, M., Mokher, C., & Flores, S. (2011). Legislative agenda setting for in-state resident tuition policies: Immigration, representation, and educational access. American Journal of Education, 117, 563–602.
- Merton, R. K. (1973). The sociology of science: Theoretical and empirical investigations. Chicago, IL: University of Chicago Press.
- Moody, M. J. (2008). State debt policies and the credit ratings of public universities. Public Finance Review, 36, 287–307.10.1177/1091142107311460
- Ness, E. C. (2010). The politics of determining merit aid eligibility criteria: An analysis of the policy process. The Journal of Higher Education, 81, 33–60.
- Nicholson-Crotty, J., & Meier, K. J. (2003). Politics, structure, and public policy: The case of higher education. Educational Policy, 17, 80–97.10.1177/0895904802239287
- Peterson, R. G. (1976). Environmental and political determinants of state higher education appropriations policies. The Journal of Higher Education, 47, 523–542.
- Primo, D. M., Jacobsmeier, M. L., & Milyo, J. (2007). Estimating the impact of state policies and institutions with mixed-level data. State Politics & Policy Quarterly, 7, 446–459.10.1177/153244000700700405
- Ravn, M. O., & Uhlig, H. (2002). On adjusting the Hodrick-Prescott filter for the frequency of observations. Review of Economics and Statistics, 84, 371–376.
- Rizzo, M. J. (2006). State preference for higher education spending: A panel data analysis, 1977-2001. In R. G. Ehrenberg (Ed.), What's happening to public higher education? The Shifting Financial Burden (pp. 3–36). Westport, CT: Greenwood Press.
- Shepsle, K. A. (1989). Measuring state legislative professionalism: The squire index revisited. Journal of Theoretical Politics, 1, 131–147.10.1177/0951692889001002002
- Slaughter, S., & Leslie, L. L. (1997). Academic capitalism: Politics, policies, and the entrepreneurial university. Baltimore, MD: Johns Hopkins University Press.
- Slaughter, S., & Rhoades, G. (2004). Academic capitalism and the new economy: Markets, state, and higher education. Baltimore, MD: Johns Hopkins University Press.
- Squire, P. (1992). Legislative professionalism and membership diversity in state legislatures. Legislative Studies Quarterly, 17, 69–79.10.2307/440081
- Squire, P. (2000). Uncontested seats in state legislative elections. Legislative Studies Quarterly, 25, 131–146.10.2307/440396
- Squire, P. (2007). Measuring state legislative professionalism: The squire index revisited. State Politics & Policy Quarterly, 7, 211–227.10.1177/153244000700700208
- Squire, P., & Hamm, K. E. (2005). 101 chambers: Congress, state legislatures, and the future of legislative studies. Columbus, OH: Ohio State University Press.
- State Higher Education Executive Officers (SHEEO). (2009). State budgeting for higher education in the United States. Boulder, CO: Author.
- State Higher Education Executive Officers (SHEEO). (2012). State higher education finance report. Boulder, CO: Author.
- Tandberg, D. A. (2008). The politics of state higher education funding. Higher Education in Review, 5, 1–36.
- Tandberg, D. A. (2010a). Interest groups and governmental institutions the politics of state funding of public higher education. Educational Policy, 24, 735–778.10.1177/0895904809339163
- Tandberg, D. A. (2010b). Politics, interest groups and state funding of public higher education. Research in Higher Education, 51, 416–450.10.1007/s11162-010-9164-5
- Tandberg, D. A., & Ness, E. C. (2011). State Capital Expenditures for Higher Education: “Where the real politics happens”. Journal of Education Finance, 36, 394–423.
- Tandberg, D. A. (2013). The conditioning role of state higher education governance structures. The Journal of Higher Education, 84, 506–543.10.1353/jhe.2013.0026
- Tandberg, D. A., & Griffith, C. (2013). State support of higher education: Data, measures, findings, and directions for future research. In M. B. Paulsen (Ed.), Higher education: Handbook of theory and Research (pp. 613–685). Netherlands: Springer.
- Toutkoushian, R. K., & Hollis, P. (1998). Using panel data to examine legislative demand for higher education. Education Economics, 6, 141–157.10.1080/09645299800000012
- Trow, M. A. (1993). The analysis of status. In B. Clark (Ed.), Perspectives on higher ed- ucation: Eight disciplinary and comparative views (pp. 132–164). Berkeley: Univer- sity of California Press.
- Tuckman, H. P., & Chang, C. F. (1991). A methodology for measuring the financial vulnerability of charitable nonprofit organizations. Nonprofit and Voluntary Sector Quarterly, 20, 445–460.10.1177/089976409102000407
- White, F. C. (1983). Trade-off in growth and stability in state taxes. National Tax Journal, 36, 103–114.
- Weerts, D. J., & Ronca, J. M. (2006). Examining differences in state support for higher education: A comparative study of state appropriations for research universities. The Journal of Higher Education, 77, 935–967.10.1353/jhe.2006.0054
- Winston, G. C. (1999). Subsidies, hierarchy and peers: The awkward economics of higher education. Journal of Economic Perspectives, 13, 13–36.10.1257/jep.13.1.13
- Wright, D. S. (1967). Executive leadership in state administration. Midwest Journal of Political Science, 11, 1–26.
- Yan, W. (2011). The interactive effect of revenue diversification and economic base on US local government revenue stability. Public Money & Management, 31, 419–426.10.1080/09540962.2011.618766
Appendix 1.
Indicators included in the governance centralization measure