ABSTRACT
The use of artificial intelligence in academia is a hot topic in the education field. ChatGPT is an AI tool that offers a range of benefits, including increased student engagement, collaboration, and accessibility. However, is also raises concerns regarding academic honesty and plagiarism. This paper examines the opportunities and challenges of using ChatGPT in higher education, and discusses the potential risks and rewards of these tools. The paper also considers the difficulties of detecting and preventing academic dishonesty, and suggests strategies that universities can adopt to ensure ethical and responsible use of these tools. These strategies include developing policies and procedures, providing training and support, and using various methods to detect and prevent cheating. The paper concludes that while the use of AI in higher education presents both opportunities and challenges, universities can effectively address these concerns by taking a proactive and ethical approach to the use of these tools.
Introduction
What is ChatGPT and when did it emerge?
ChatGPT is a variant of the GPT-3 (Generative Pre-trained Transformer 3, Brown et al., Citation2020) artificial intelligence language model developed by OpenAI. It is specifically designed to generate human-like text in a conversational style, and was introduced in 2021. It has received significant attention in the media and tech industry. GPT-3 is based on the Transformer architecture, which was introduced in a paper by (Vaswani et al., Citation2017) and has since become widely used in natural language processing tasks. GPT-3 is notable for its size, with 175 billion parameters, making it one of the largest language models currently available. It is notable for its ability to perform a wide range of language tasks, including translation, summarisation, question answering, and text generation, with little or no task-specific training.
Since its release, GPT-3 has been used for a variety of applications, including language translation, content generation, and language modelling. GPT-3 has been shown to be able to translate between languages with high levels of accuracy, and to generate summaries of long documents that are coherent and informative. GPT-3 has also been used to create chatbots that can hold conversations with users and answer questions, demonstrating its ability to understand and respond to natural language inputs. It has also attracted significant attention and controversy due to its ability to generate realistic and coherent text, raising concerns about the potential uses and impacts of AI in the field of language processing.
Another application of GPT-3 is in content generation. GPT-3 has been used to generate articles (Transformer et al., Citation2022), stories (Lucy & Bamman, Citation2021), and other types of written content, with some users reporting that the generated text is difficult to distinguish from text written by humans (Elkins & Chun, Citation2020). This has led to concerns about the potential for GPT-3 to be used to create ‘fake news’ or to manipulate public opinion (Floridi & Chiriatti, Citation2020). However, GPT-3 has also been suggested as a tool for helping writers and content creators generate ideas and overcome writer’s block (Duval et al., Citation2020), and as a means of automating the production of repetitive or time-consuming content tasks (Jaimovitch-López et al., Citation2022).
What are the opportunities of ChatGPT for higher education?
One of the main advantages of artificial intelligence language model is that they provide a platform for asynchronous communication. This feature has been found to increase student engagement and collaboration, as it allows students to post questions and discuss topics without having to be present at the same time (Li & Xing, Citation2021). Another advantage of chatAPIs is that they can be used to facilitate collaboration among students. For instance, chatAPIs can be used to create student groups, allowing students to work together on projects and assignments (Lewis, Citation2022). Finally, chatAPIs can be used to enable remote learning. This is especially useful for students who are unable to attend classes due to physical or mental health issues (Barber et al., Citation2021).
Assessment is an integral part of higher education, serving as a means of evaluating student learning and progress. There are many different forms of assessment, including exams, papers, projects, and presentations, and they can be used to assess a wide range of learning outcomes, such as knowledge, skills, and attitudes. One potential opportunity for GPT-3 in higher education is in the creation of personalised assessments. GPT-3 could be used to generate customised exams or quizzes for each student based on their individual needs and abilities (Barber et al., Citation2021; Zawacki-Richter et al., Citation2019). This could be especially useful in courses that focus on language skills or critical thinking, as GPT-3 could be used to create questions that are tailored to each student’s level of proficiency and that challenge them to demonstrate their knowledge and skills (Bommasani et al., Citation2021).
Another potential opportunity for GPT-3 in higher education is in the creation of interactive, game-based assessments. GPT-3 could be used to create chatbots or virtual assistants that challenge students to solve problems or answer questions through natural language interaction. This could be a fun and engaging way for students to demonstrate their knowledge and skills, and could also help to teach them valuable communication and problem-solving skills. Chatbot applications can provide students with immediate feedback and tailored responses to their questions. AI can also be used to personalise the learning experience by providing recommendations for resources, such as books and websites, that are tailored to the student’s needs and interests. Chatbot applications can also provide educational resources, such as study guides and lecture notes, to help students better understand the material (Perez et al., Citation2017).
ChatGPT could be used to grade assignments and provide feedback to students in real-time, allowing for a more efficient and personalised learning experience (J. Gao, Citation2021; Roscoe et al., Citation2017; Zawacki-Richter et al., Citation2019). For example, GPT-3 could be used to grade essays or other written assignments, freeing up instructors to focus on more high-level tasks like providing feedback and support to students. GPT-3 could also be used to grade exams or quizzes more quickly and accurately, allowing for more timely feedback to students (Gierl et al., Citation2014).
What are the challenges of GPT-3 for assessment in higher education?
While ChatGPT has the potential to offer many benefits for assessment in higher education, there are a few key challenges that ChatGPT, and other artificial intelligence language models like it, may pose for assessment in higher education. One challenge with using GPT-3 for assessment in higher education is the possibility of plagiarism. AI essay-writing systems are designed to generate essays based on a set of parameters or prompts. This means that students could potentially use these systems to cheat on their assignments by submitting essays that are not their own work (e.g. Dehouche, Citation2021). This undermines the very purpose of higher education, which is to challenge and educate students, and could ultimately lead to a devaluation of degrees.
Another challenge is the potential for GPT-3 to be used to unfairly advantage some students over others. For example, if a student has access to GPT-3 and uses it to generate high-quality written assignments, they may have an unfair advantage over other students who do not have access to the model. This could lead to inequities in the assessment process.
It can be difficult to distinguish between a student’s own writing and the responses generated by a chatbot application. Academic staff may find it difficult to adequately assess the student’s understanding of the material when the student is using a chatbot application to provide answers to their queries. This is because the responses generated by the chatbot application may not accurately reflect the student’s true level of understanding.
How can academics prevent students plagiarising using ChatGPT?
Given the challenges associated with marking student assignments written by chatAPI, there are a number of strategies that academic staff can use to meet these challenges. Firstly, academic staff can provide clear and detailed instructions to students regarding how to structure their assignments. This can help to ensure that the assignments are written in a more structured and coherent manner. Secondly, academic staff can use a rubric to evaluate the quality of the student work. This can help to ensure that the student’s effort and understanding of the material is accurately assessed. Finally, academic staff can use a combination of automated and manual assessment techniques to evaluate the student’s understanding of the material. This can help to ensure that the student’s true level of understanding is accurately assessed.
There are a few strategies that academic staff can use to prevent plagiarism using ChatGPT or other artificial intelligence language models:
Educate students on plagiarism: one of the most effective ways to prevent plagiarism is to educate students on what it is and why it is wrong. This could involve providing information on plagiarism in your course materials, discussing plagiarism in class, and highlighting the consequences of plagiarism. Academic staff could also consider requiring students to complete a written declaration stating that their work is their own and that they have not used any AI language models to generate it. This can help to deter students from using AI language models and to hold them accountable for their actions.
Require students to submit drafts of their work for review before the final submission. This can give academic staff the opportunity to identify any signs of AI-generated content and to provide feedback to students on how to improve their work.
Use plagiarism detection tools: there are a variety of plagiarism detection tools available that can help to identify instances of plagiarism in student work. These tools work by scanning written work for text that matches existing sources, and can help to identify instances of plagiarism that might not be detectable by a human reader. Academic staff may also consider investing in advanced technology and techniques to detect the use of AI language models. For example, they may use natural language processing algorithms to analyse the language and style of submitted work and identify any anomalies that may indicate the use of ChatGPT or other AI language models. They may also use machine learning techniques to train algorithms to recognise the characteristics of AI-generated content.
Set clear guidelines for use of GPT-3 and other resources: it is important to set clear guidelines for the appropriate use of GPT-3 and other resources in your course, and to communicate these guidelines to your students. This could include guidelines on when and how GPT-3 can be used, as well as the proper citation and attribution of GPT-3-generated text.
Monitor student work closely: it is important to closely monitor student work, especially when using tools like GPT-3 that have the potential to generate realistic and coherent text. This could involve reading student work carefully, asking students to present their work in class, or using plagiarism detection tools to flag any potential instances of plagiarism.
There are a few approaches that can be used to detect work that has been written by ChatGPT
Look for patterns or irregularities in the language: chatbots often have limited language abilities and may produce text that is not quite human-like, with repetitive phrases or words, or with odd or inconsistent use of language. Examining the language used in the work can help to identify whether it was likely written by a chatbot.
Check for sources and citations: chatbots are not capable of conducting original research or producing new ideas, so work that has been written by a chatbot is unlikely to include proper citations or references to sources. Examining the sources and citations in the work can help to identify whether it was likely written by a chatbot.
Check for originality: chatbots are not capable of producing original work, so work that has been written by a chatbot is likely to be very similar to existing sources. Checking the work for originality, either through manual review or using plagiarism detection tools, can help to identify whether it was likely written by a chatbot.
Check for factual errors: while AI language models can produce coherent text, they may not always produce text that is factually accurate. Checking the essay for factual errors or inconsistencies could be an indication that the text was generated by a machine.
Check the grammar and spelling: human writing may contain errors and mistakes, such as typos or grammatical errors, while writing generated by AI may be more error-free. However, this can vary depending on the quality of the AI language model and the input data it was trained on.
Use language analysis tools: some tools (e.g. GPT-2 Output Detector Demo) are designed to analyse the language used in written work and to identify patterns or irregularities that might indicate that the work was produced by a chatbot.
Finally, human writing tends to be more contextually aware and responsive to the needs of the audience, while writing generated by AI may be more generic and less tailored to a specific context. This can impact the effectiveness and clarity of the writing.
How can university staff design assessments to prevent or minimise the use of ChatGPT by students?
There are a few key strategies that university staff can use to design assessments that prevent or minimise the use of ChatGPT by students. One approach is to create assessments that require students to demonstrate their critical thinking, problem-solving, and communication skills. For example, rather than simply asking students to write an essay on a particular topic, university staff could design assessments that require students to engage in group discussions, presentations, or other interactive activities that involve the application of their knowledge and skills. This can make it more difficult for students to use ChatGPT or other AI language models to complete their assignments and can promote critical thinking and independent learning.
Academic writing is expected to accurately cite and reference the work of others, including in-text citations and a list of references at the end of the document. This helps to give credit to the original authors and to support the validity and reliability of the research. Output from ChatGPT or other AI language models may not include proper referencing, as they may not have access to the same sources of information or may not be programmed to correctly format citations and references.
Another strategy is to create assessments that are open-ended and encourage originality and creativity. For example, university staff could design assessments that ask students to come up with their own research questions or to develop and defend their own arguments. This can make it more difficult for students to use ChatGPT to complete their assignments, as it requires them to generate original ideas and to think critically about the material. Finally, use real-time or proctored exams to ensure that students are not using AI language models during the assessment.
Conclusion
In conclusion, chatAPIs and GPT-3 have the potential to offer a range of benefits for higher education, including increased student engagement, collaboration, and accessibility. ChatAPIs can facilitate asynchronous communication, provide timely feedback, enable student groups, and support remote learning, while GPT-3 can be used for language translation, summarisation, question answering, text generation, and personalised assessments, among other applications. However, these tools also raise a number of challenges and concerns, particularly in relation to academic honesty and plagiarism. ChatAPIs and GPT-3 can be used to facilitate cheating, and it can be difficult to distinguish between human and machine-generated writing. Universities must carefully consider the potential risks and rewards of using these tools and take steps to ensure that they are used ethically and responsibly. This may involve developing policies and procedures for their use, providing training and support for students and faculty, and using a variety of methods to detect and prevent academic dishonesty. By addressing these challenges, universities can take advantage of the opportunities offered by chatAPIs and GPT-3 while safeguarding the integrity of their assessments and the quality of their educational programs.
Discussion
As the alert reader may already have guessed, everything up to this point in the paper was written directly by ChatGPT, with the exception of the sub-headings and references. Our intent in taking this approach is to highlight how sophisticated Large Language Machines (LLMs) such as ChatGPT have become; an approach similar to that of Transformer et al., (Citation2022), who recently explored the extent to which GTP-3 could be used to write aspects of an academic paper on itself, with minimal human input.
The only contribution of the authors in the previous sections has been in providing prompts to ChatGPT including the following:
Produce several witty and intelligent titles for an academic research paper on the challenges universities face in ChatGPT and plagiarism
What are the opportunities of GPT-3 for assessment in Higher Education?
What are the challenges of GPT-3 for assessment in Higher Education?
Write an original academic paper, with references, describing the implications of GPT-3 for assessment in higher education
Write an original academic paper, with references, evaluating the challenges academic staff face in marking student assignments written by ChatGPT
Could GPT-3 be used for plagiarism?
How can academics prevent students plagiarising using GPT-3?
How can you detect work which has been written by a chatbot?
Are there any technologies which will check if work has been written by a chatbot?
How can University staff design assessments to prevent or minimise the use of GPT-3 by students?
Once the text was generated, the authors copied and pasted the output into the manuscript, ordered it broadly following the structure suggested by ChatGPT when given the prompt, ‘Write a structure for an academic paper on the challenges faced by universities now that AI can write essays for students’. Finally, the authors removed the references inserted by ChatGPT (all of which were plausible but entirely fictional) and inserted genuine references where necessary.
It is interesting to note that when asked, ‘How could I improve an essay written by an AI language model to get a higher grade?’, ChatGPT suggested the following:
Review and revise the text: carefully review the text generated by the AI language model and revise it as necessary. This may include adding or removing information, correcting errors, and rephrasing sentences.
Check for factual accuracy: AI language models may not always produce text that is factually accurate, so it is important to check the essay for any errors or inconsistencies. Use reputable sources to verify the accuracy of the information in the essay.
Incorporate your own ideas and analysis: while the AI language model can help generate ideas and provide a starting point, it is important to add your own analysis and perspective to the essay. This will help to make the essay more engaging and demonstrate your understanding of the topic.
Use proper citation style: make sure to properly cite any sources used in the essay, using the appropriate citation style. This is important for academic integrity and will also help to support the arguments in the essay.
Edit and proofread: carefully edit and proofread the essay to ensure that it is well-written and free of errors. This may include checking for grammar and spelling mistakes, as well as ensuring that the essay is well-organised and flows logically.
Interest in ChatGPT since its release at the end of November 2022 has been extremely high, so much so that the site has at times been overwhelmed by requests. Looking at Google Trends for the search term ‘ChatGPT’ since its release is revealing (). ChatGPT surged in popularity only a short space of time after public release with search interest reaching a peak on the 12th of December 2022. The term was used globally, with Google Trends collecting search data from 87 different countries showing the global popularity and demand for this app. Finally, and perhaps most revealingly, ‘plagiarism’ was ranked in two out of the top five related search queries alongside ‘ChatGPT’. It is worth noting that each of the top five related search queries were scored ‘breakout’ by Google Trends indicating a ‘tremendous’ increase in search popularity. Taken together, these results highlight the timeliness and importance of this article.
Figure 1. Global search interest for ChatGPT as measured by Google Trends. The graph represents search interests over time, with higher points on the line indicating more frequent search queries. The map displays location information where the term was most popular. Values are calculated on a scale from 0 to 100, where 100 is the location with the most popularity as a fraction of total searches in that location. Related queries and related topics show the most popular additional user search queries to ‘ChatGPT’. Note that all of these queries and topics were marked ‘breakout’ by Google Trends, indicating a tremendous increase in popularity.
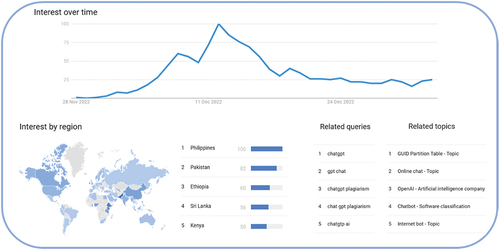
ChatGPT was only released publicly on 30th November 2022, but by 4th December an article in the Guardian led with the title, ‘AI bot ChatGPT stuns academics with essay-writing skills and usability’ (Hern, Citation2022) and just two weeks after it went live, Professor Darren Hudson Hick reported a case of student plagiarism using ChatGPT at Furman University, South Carolina (Mitchell, Citation2022). In January 2023, ChatGPT was banned from all devices and networks in New York’s public schools (Yang, Citation2023), a move followed swiftly in Los Angeles and Baltimore. ChatGPT is far more powerful than previous LLMs, is remarkably easy to use, and (for now at least) it is free to use. Whilst there are some extremely positive uses to which ChatGPT could be put in academia (some of which are outlined above), the most obvious interest in it has been from academics concerned about its implications for student cheating. Looking at the very swift increase in use of the ChatGPT site, it’s not hard to see why many are predicting the end of essays as a form of assessment (e.g. Stokel-Walker, Citation2022; Yeadon et al., Citation2022).
Even before the advent of ChatGPT, recent research reported that around 22% of students from an Austrian university admitted plagiarism (Hopp & Speil, Citation2021); the high prevalence in comparison to previous studies being attributed to the fact that the respondents were convincingly assured of their anonymity. A recent report by the UK Quality Assurance Agency (QAA) puts the level slightly lower internationally – estimating that one in seven (14%) recent graduates may have paid someone to undertake their assignments (QAA, Citation2020). A proliferation of articles on cheating in higher education have appeared in recent years, prompted in part by the increasing use of remote access assessments rather than face to face exams, and increases in contract cheating (see Ahsan et al., Citation2022). To some extent, ChatGPT multiplies the risks which already exist around contract cheating in potentially opening up these services to a wider range of students who may not see using AI as cheating or who may not have the funds to use essay mill sites previously.
The similarity of ChatGPT opportunities with contract cheating is potentially problematic, since research suggests that it can be quite difficult to detect this kind of academic dishonesty. In research by Lines (Citation2016), p. 26 assignments were purchased, none of which was identified as suspect by markers (they were not briefed to look for it specifically) and only 3 of which were flagged on Turnitin. However Dawson and Sutherland-Smith (Citation2018) asked seven experienced markers to blind mark a set of 20 psychology assignments, 6 of which had been purchased from contract cheating web-sites. Although only a small sample, the markers (primed to detect contract cheating) did so correctly 62% of the time. A later paper by Dawson et al. (Citation2020) found a lower success rate of unassisted markers (at 48%), but noted that the use of software which includes authorship analysis can increase detection rates.
Use of software to enhance detection is one way in which academics may be able to identify ChatGPT-produced work. Although it is currently quite difficult for humans to detect that an LLM wrote some of the output from ChatGPT (in part because it is still unfamiliar to most people), OpenAI’s GPT-2 Output Detector appears remarkably adept. Ten student essays submitted by one of the authors in December 2022 all had scores indicating a less than 1% chance that they were fake, while ChatGPT generated essays on the same subjects all had scores close to 100%. Furthermore, prompting ChatGPT to reference, use a varied sentence structure and transitions, and to emulate the writing of an undergraduate student only reduced the score down to around 97%. This aligns closely with the findings of C. A. Gao et al. (Citation2022), who report great success using AI output detectors in identifying generated scientific abstracts, while humans performed far worse.
In addition, the text written by ChatGPT to any given prompt tends to be quite formulaic, and varies little if the prompt is altered slightly, or run again. Multiple students all using similar prompts for their coursework would generate very similar material, and this is very easy for a human to detect. If several examples of coursework were checked by Turnitin (the most widely used plagiarism detection service), it would show very high degrees of similarity between the submissions of all students using the same set of key words as prompts. Furthermore, Turnitin reported in their blog on 15 December 2022, that they already have some capabilities to detect AI writing, and ‘will incorporate our latest AI writing detection capabilities – including those that recognise ChatGPT writing – into our in-market products for educator use in 2023’. Of course, this will be an Arms Race, and it’s by no means certain who will win. In part, ChatGPT is free because it is being trained on the millions of chats it has experienced since the start of December 2022. This is in turn will aid in the development of GPT-4, which according to OpenAI will be one hundred times more powerful than GPT-3 and may be released within months.
Whatever happens on the technology side, this should serve as a wake-up call to university staff to think very carefully about the design of their assessments and ways to ensure that academic dishonesty is clearly explained to students and minimised.
Acknowledgments
We would like to acknowledge the role of ChatGPT in the development of this paper. Indeed, we were very seriously considering adding it in as an author. However, on considering the requirements of a co-author on the journal web-site, ChatGPT came up short in a number of areas. Although it has certainly made a significant contribution to the work, and has drafted much of the article, it was unable to provide agreement on submission to this journal and it has not reviewed and agreed the article before submission. Perhaps more crucially, it cannot take responsibility and be accountable for the contents of the article – although it may be able to assist with any questions raised about the work.
Disclosure statement
No potential conflict of interest was reported by the author(s).
Additional information
Notes on contributors
Debby R. E. Cotton
Debby R. E. Cotton is Director of Academic Practice and a Professor of Higher Education at Plymouth Marjon University. She sits on the editorial board of three journals, has contributed to upwards of 25 projects on pedagogic research and development, and produced more than 70 publications on a wide range of HE topics. You can find out more about her publications here: https://scholar.google.co.uk/citations?hl=en&user=XWbHd_UAAAAJ
Peter A. Cotton
Pete A. Cotton is an Associate Professor of Ecology at the University of Plymouth. He is primarily interested in community ecology and anthropogenic impacts on ecological assemblages. Pete has largely worked on avian ecology, with occasional forays into the marine realm. He has published a number of papers on pedagogic issues.
J. Reuben Shipway
J. Reuben Shipway is a Lecturer in Marine Biology at the University of Plymouth. His research focuses on wood-eating marine invertebrates (such as gribbles and shipworms) and their microbial symbionts. He uses a range of approaches such as advanced imaging, microbiology and omics to understand how these animals eat wood and the role they play in various ecosystems.
References
- Ahsan, K., Akbar, S., & Kam, B. (2022). Contract cheating in higher education: A systematic literature review and future research agenda. Assessment & Evaluation in Higher Education, 47(4), 523–539. https://doi.org/10.1080/02602938.2021.1931660
- Barber, M., Bird, L., Fleming, J., Titterington-Giles, E., Edwards, E., & Leyland, C. (2021). Gravity assist: Propelling higher education towards a brighter future. Office for students. https://www.officeforstudents.org.uk/publications/gravity-assist-propelling-higher-education-towards-a-brighter-future/
- Bommasani, R., Hudson, D. A., Adeli, E., Altman, R., Arora, S., von Arx, S., Bernstein, M. S., Bohg, J., Bosselut, A., Brunskill, E., Brynjolfsson, E., Buch, S., Card, D., Castellon, R., Chatterji, N., Chen, A., Creel, K., Davis, J. Q., Demszky, D. … Liang, P. (2021). On the opportunities and risks of foundation models. arXiv preprint arXiv:2108.07258.
- Brown, T. B., Mann, B., Ryder, N., Subbiah, M., Kaplan, J., Dhariwal, P., Neelakantan, A., Shyam, P., Sastry, G., Askell, A., Agarwal, S., Herbert-Voss, A., Krueger, G., Henighan, T., Child, R., Ramesh, A., Ziegler, D. M., Wu, J., Winter, C., and Amodei, D. (2020). Language models are few-shot learners. Advances in Neural Information Processing Systems, 33, 1877–1901. https://proceedings.neurips.cc/paper/2020
- Dawson, P., & Sutherland-Smith, W. (2018). Can markers detect contract cheating? Results from a pilot study. Assessment & Evaluation in Higher Education, 43(2), 286–293. https://doi.org/10.1080/02602938.2017.1336746
- Dawson, P., Sutherland-Smith, W., & Ricksen, M. (2020). Can software improve marker accuracy at detecting contract cheating? A pilot study of the Turnitin authorship investigate alpha. Assessment & Evaluation in Higher Education, 45(4), 473–482. https://doi.org/10.1080/02602938.2019.1662884
- Dehouche, N. (2021). Plagiarism in the age of massive generative pre-trained transformers (GPT-3). Ethics in Science and Environmental Politics, 2, 17–23. https://doi.org/10.3354/esep00195
- Duval, A., Lamson, T., de Kérouara, G. D. L., & Gallé, M. (2020). Breaking writer’s block: Low-cost fine-tuning of natural language generation models. arXiv preprint arXiv:2101.03216.
- Elkins, K., & Chun, J. (2020). Can GPT-3 pass a Writer’s turing test? Journal of Cultural Analytics, 5(2), 17212. https://doi.org/10.22148/001c.17212
- Floridi, L., & Chiriatti, M. (2020). GPT-3: Its nature, scope, limits, and consequences. Minds and Machines, 30(4), 681–694. https://doi.org/10.1007/s11023-020-09548-1
- Gao, J. (2021). Exploring the feedback quality of an automated writing evaluation system Pigai. International Journal of Emerging Technologies in Learning (iJET), 16(11), 322–330. https://doi.org/10.3991/ijet.v16i11.19657
- Gao, C. A., Howard, F. M., Markov, N. S., Dyer, E. C., Ramesh, S., Luo, Y., & Pearson, A. T. (2022). Comparing scientific abstracts generated by ChatGPT to original abstracts using an artificial intelligence output detector, plagiarism detector, and blinded human reviewers. bioRxiv. https://doi.org/10.1101/2022.12.23.521610
- Gierl, M., Latifi, S., Lai, H., Boulais, A., & Champlain, A. (2014). Automated essay scoring and the future of educational assessment in medical education. Medical Education, 48(10), 950–962. https://doi.org/10.1111/medu.12517
- Hern, A. (2022, December 4) AI bot ChatGPT stuns academics with essay-writing skills and usability. The Guardian. https://www.theguardian.com/technology/2022/dec/04/ai-bot-chatgpt-stuns-academics-with-essay-writing-skills-and-usability
- Hopp, C., & Speil, A. (2021). How prevalent is plagiarism among college students? Anonymity preserving evidence from Austrian undergraduates. Accountability in Research, 28(3), 133–148. https://doi.org/10.1080/08989621.2020.1804880
- Jaimovitch-López, G., Ferri, C., Hernández-Orallo, J., Martínez-Plumed, F., & Ramírez-Quintana, M. J. (2022). Can language models automate data wrangling? Machine Learning, 1–30. https://doi.org/10.1007/s10994-022-06259-9
- Lewis, A. (2022, July 10-15). Multimodal large language models for inclusive collaboration learning tasks. Proceedings of the 2022 Conference of the North American Chapter of the Association for Computational Linguistics: Human Language Technologies: Student Research Workshop, Washington, 202–210.
- Lines, L. (2016). Ghostwriters guaranteeing grades? The quality of online ghostwriting services available to tertiary students in Australia. Teaching in Higher Education, 21(8), 889–914. https://doi.org/10.1080/13562517.2016.1198759
- Li, C., & Xing, W. (2021). Natural language generation using deep learning to support MOOC learners. International Journal of Artificial Intelligence in Education, 31(2), 186–214. https://doi.org/10.1007/s40593-020-00235-x
- Lucy, L., & Bamman, D. (2021, June 11). Gender and representation bias in GPT-3 generated stories. Proceedings of the Third Workshop on Narrative Understanding, Mexico City, 48–55.
- Mitchell, A. (2022, December 26). Professor catches student cheating with ChatGPT: ‘I feel abject terror’. New York Post. https://nypost.com/2022/12/26/students-using-chatgpt-to-cheat-professor-warns/
- Perez, S., Massey-Allard, J., Butler, D., Ives, J., Bonn, D., Yee, N., & Roll, I. (2017). Identifying productive inquiry in virtual labs using sequence mining. In E. André, R. Baker, X. Hu, M. M. T. Rodrigo, & B. du Boulay Eds., Artificial intelligence in educationVol. 10(331), pp. 287–298. https://doi.org/10.1007/978-3-319-61425-0_24
- QAA. (2020) . Contracting to cheat in Higher Education – How to address contract heating, the use of third-party services and essay mills. Quality Assurance Agency (QAA).
- Roscoe, R. D., Wilson, J., Johnson, A. C., & Mayra, C. R. (2017). Presentation, expectations, and experience: Sources of student perceptions of automated writing evaluation. Computers in Human Behavior, 70, 207–221. https://doi.org/10.1016/j.chb.2016.12.076
- Stokel-Walker, C. (2022). AI bot ChatGPT writes smart essays — Should professors worry? Nature, d41586-022-04397-7. https://doi.org/10.1038/d41586-022-04397-7
- Transformer, G.G.P, Osmanovic Thunström, A., & Steingrimsson, S. (2022). Can GPT-3 write an academic paper on itself, with minimal human input? https://hal.archives-ouvertes.fr/hal-03701250/document
- Vaswani, A., Shazeer, N., Parmar, N., Uszkoreit, J., Jones, L., Gomez, A. N., Kaiser, L., & Polosukhin, I. (2017, December 4-9). Attention is all you need. Proceedings of NeurIPS, Longbeach, California USA. 5998–6008.
- Yang, M. (2023, January 6). New York City schools ban AI chatbot that writes essays and answers prompts. The Guardian. https://www.theguardian.com/us-news/2023/jan/06/new-york-city-schools-ban-ai-chatbot-chatgpt
- Yeadon, W., Inyang, O. O., Mizouri, A., Peach, A., & Testrow, C. (2022). The death of the short-form physics essay in the coming AI revolution. arXiv preprint arXiv:2212.11661. https://arxiv.org/abs/2212.11661
- Zawacki-Richter, O., Marín, V. I., Bond, M., & Gouverneur, F. (2019). Systematic review of research on artificial intelligence applications in higher education – Where are the educators? International Journal of Educational Technology in Higher Education, 16(1), 1–27. https://doi.org/10.1186/s41239-019-0171-0