ABSTRACT
We tested whether ChatGPT can play a role in designing field courses in higher education. In collaboration with ChatGPT, we developed two field courses; the first aimed at creating a completely new field trip, while the second was tailored to fit an existing university module, and then compared to the human module design. From our case studies, several insights emerged. These include the importance of precise prompt engineering and the need to iterate and refine prompts to achieve optimal results. We outlined a workflow for effective prompt engineering, emphasising clear objectives, sequential prompting, and feedback loops. We also identify best practices, including highlighting the importance of collaborating with human expertise, validating AI suggestions, and integrating adaptive management for continual refinement. While ChatGPT is a potent tool with the potential to save a significant amount of time and effort in field course design, human expertise remains indispensable for achieving optimal results.
Introduction
ChatGPT is an artificial intelligence (AI) chatbot, launched in November 2022 by OpenAI, that represents a significant advancement in natural language processing (NLP) and AI technologies (OpenAI, Citation2022). ChatGPT is a language model trained on a vast corpus of text data from the internet, allowing it to generate coherent and contextually relevant responses to user inputs (Rudolph et al., Citation2023). It has demonstrated impressive capabilities in understanding and generating human-like text, making it a valuable tool in various domains (Atlas, Citation2023; Dwivedi et al., Citation2023; Metz, Citation2022). This includes academia where ChatGPT has proven useful in developing ideas, teaching and learning activities, marking, and curricula design (Atlas, Citation2023; Dwivedi et al., Citation2023; Kiryakova & Angelova, Citation2023; Metz, Citation2022). This is timely considering that university faculty have faced an increase in teaching and bureaucracy workloads (Martin, Citation2016) which is linked to stress, depression, anxiety, and a reduction in work-life balance (Pace et al., Citation2021). Despite the deluge of publications exploring the role of ChatGPT in academia, its role in field course design and implementation has yet to be evaluated.
Field courses are highly popular, off-campus university courses that provide students with experiential learning through a hands-on approach to critical problem-solving skills and in-person engagement with faculty experts (Biggs & Tang, Citation2011; Durrant & Hartman, Citation2015; Geange et al., Citation2021). However, they are complex to design and implement because of logistical and safety implications, and the need to maintain academic rigour. As such, they can be time-consuming to develop, making them an excellent target for ChatGPT-assisted design. In this paper, we seek to determine the efficacy of using ChatGPT in planning and designing field courses. Our focus is on developing a marine biology field course, but the workflow outlined herein will be widely applicable to many courses involving fieldwork. It also extends beyond higher education into any profession or practice that involves fieldwork, such as geosciences and environmental consulting, biodiversity conservation, fisheries management, or public engagement efforts. Specific examples could include, but are not limited to, environmental impact assessment of built infrastructure, citizen science events such as biodiversity and ecological surveys, and habitat assessments.
We take the approach outlined in Cotton et al. (Citation2023), where case studies were constructed in dialogue with ChatGPT, to demonstrate the capabilities and ease of use, and the challenges and limitations of using AI chatbots for field course planning and design. We develop two case studies in collaboration with ChatGPT; the first with the aim of designing a de novo field trip, and the second to fit within the course requirements of a pre-existing university module. In doing so, we showcase the versatility of the AI model in addressing different planning requirements and its utility across educational contexts. Both were developed specifically for domestic (in this case, within the UK), single-day field trips, but the workflow we provide establishes the foundation for developing longer field courses including those in international destinations. We outline the lessons learned from developing a field course through ChatGPT, provide a workflow for effective prompt engineering, and set guidelines for best practice.
Methods
To test ChatGPT’s ability to produce meaningful and useable field course curricula and itineraries, we developed two case studies integrating ChatGPT in field course design. As marine biologists, we focussed our case studies on coastal and marine ecological processes. Being among one of the first studies to explore the use of ChatGPT in field course design, this paper centres its attention on prompt engineering, workflow, best practice, and evaluating the strengths and weaknesses of ChatGPT output. Student evaluations were beyond the scope of this paper due to the time and resources needed to collect such data. However, ChatGPT’s output was evaluated against the authors’ extensive fieldwork and field course curriculum design experience with over 50,000 combined hours of fieldwork and field course curriculum design experience, including field courses successfully accredited by the Institute of Marine Engineering, Science and Technology (IMarEST), The New England Commission of Higher Education, and Royal Society of Biology.
For both case studies, a series of prompts were input to ChatGPT 3.5 to develop the course content, itinerary, logistics, and health and safety considerations. We used ChatGPT 3.5 because it is freely available to the public and therefore most widely accessible to users, unlike version 4.0 which requires a paid subscription. All output was recorded and used to develop the next prompt in an iterative process.
Case Study 1 asked the user to choose the subject, content, and location of the field course, rather than supplying a pre-determined subject and location as in Case Study 2. The second case study involved developing a one-day field trip to the Gower Peninsula in South Wales (UK), to study the hydrological processes that are important to management of coastal habitats. The authors had recently planned a field trip to the Gower Peninsula from The University of Wales Trinity Saint David, thus Case Study 2 allowed an opportunity to compare a ChatGPT-generated design to an original human-designed field trip.
This section provides an overview of the general methodology; details of the prompt engineering used to generate the case study outputs can be found in sections 3.1 and 3.2 and ChatGPT output. We provide author prompts and ChatGPT output in supplemental appendices (Appendices A through S), with summaries of prompts and output presented in the Case Studies below. The full supplemental appendices can be found here: https://doi.org/10.6084/m9.figshare.25146062.v1
Maps and route plans in Case Study 2 were created using Google Maps.
Case studies
Case study 1: Integrating ChatGPT in marine biology module design (no specified subject or location)
For the first case study, we prompted ChatGPT to suggest a series of activities for a marine biology field course. This initial prompt was deliberately vague, as we wanted ChatGPT to consider a range of possible activities and suggest multiple options. It came up with a list of potential activities, including: (1) a workshop on marine research techniques, including underwater survey methods, species identification, and data collection; (2) hands-on experience with equipment such as plankton nets, underwater cameras, and water quality testing kits, (3) snorkelling or boat trip to explore a nearby coral reef or kelp forest, guided by a marine biologist (Appendix A). Some of the suggestions, such as snorkelling on a coral reef, were obviously not possible in the UK. This highlights the need for a more structured prompt that informs ChatGPT of the specific locations and/or ecosystems available to be studied. However, one of the suggestions was a beach clean-up activity to raise awareness about marine pollution and its impact on marine ecosystems. As marine litter is a ubiquitous and pervasive problem impacting almost all marine ecosystems (Napper & Thompson, Citation2020), and is therefore broadly applicable regardless of surrounding geographic environments, we decided to develop this idea further.
The second prompt asked ChatGPT to elaborate on the marine pollution activity, and to list the key learning objectives. A series of detailed and logically structured activities were provided, including an ‘Introduction and briefing’, notes on how to prepare for the activity (e.g. equipment for the activity and how to divide the class into groups), how to conduct the clean, how to organise and subsequently analyse the data, and then points for a ‘Reflection and Discussion’ (Appendix B). ChatGPT then provided seven varied and relevant learning objectives, from ‘Analyze and interpret data collected during the clean-up to identify trends and patterns’, to ‘Foster a sense of responsibility and stewardship towards the marine environment’. These broadly align with the guidelines for writing effective learning objectives provided by Orr et al. (Citation2022), in that they are clear, measurable, and attainable, simply communicate what students should know and be able to do, and help guide the design of assessments and instructional activities. However, we do note that several of these learning objectives lacked specificity. For example, objective 5, ‘Analyze and interpret data collected during the clean-up to identify trends and patterns’ could be enhanced by providing further guidance on the specific data to be collected during the clean-up and the analytical techniques to be applied for identifying trends and patterns. So, while ChatGPT provides a solid learning objectives workflow, further refining is required to be more specific and actionable, and to enhance their overall clarity and effectiveness.
Next, we asked ChatGPT to provide suggestions for the best place in the southwest UK to run this marine litter field course (Appendix C). The first answer was too broad, as the response simply listed three counties in the UK (Cornwall, Devon and Dorset) and provided a brief description of these regions. However, this allowed us to refine our prompt by being more specific and detailed by asking ChatGPT to provide a rationale why the suggested location would be suitable for studying marine litter (Appendix D). ChatGPT subsequently suggested that St. Ives in Cornwall as a suitable location, based on the likely impact of tourism on marine litter and the diverse coastal environments in the region. These reasons are indeed valid. St. Ives is a popular tourist destination and would provide an opportunity for students to explore the connection between tourism and marine litter. Moreover, the region features a combination of rocky shores, sandy beaches and salt marshes in close proximity, with Porthmeor Beach being a prime example. This ease of access to several different types of marine habitat would allow students to compare and contrast the effects of marine litter across various ecosystems.
However, we note that one of the justifications provided by ChatGPT is an example of a ‘hallucination’ – a now well-documented phenomenon (Alkaissi & McFarlane, Citation2023; OpenAI, Citation2022) where incorrect or nonsensical answers are provided to prompts. In one of the justifications for selecting St. Ives, ChatGPT stated that ‘St. Ives is situated near marine protected areas, such as the St. Ives Bay Marine Conservation Zone’. While St. Ives is located close to the Cape Bank Marine Conservation Zone (Department for Environment, Citation2019), the St. Ives Bay Marine Conservation Zone does not exist. As wisely noted by Salvagno et al. (Citation2023), it is always worth considering the veracity of ChatGPT output, and that human judgement and expert consideration is still required before ‘any critical decision-making or application’.
In continuing the development of marine litter activity, we then prompted ChatGPT to provide a full equipment list (Appendix E). Importantly, we provided a workflow for this equipment list, which included the activity (marine litter collection and examination), the location and date of said activity (St Ives, Cornwall, in May), and the types of environments where the activity would take place (sandy beach and rocky shore at low tide). This workflow also listed the cohort size (60 students, 4 staff), stipulated that students would be organised into groups of 4, then requested that the equipment list reflected these criteria. The resulting equipment list was comprehensive and matched the planned activity well, although there were several items that would not be required (e.g. environmental monitoring equipment like water quality testing kits). In addition, ChatGPT competently provided the exact number of items required and separated these out into groups as requested. However, as ChatGPT follows the prompt instructions exactly, it did not provide any additional equipment for contingency, for example, if the equipment was damaged or accidentally lost during the activity. Overall, these are minor critiques, and it is easy for course designers to remove equipment that is non-relevant and add surplus equipment where required.
With the activity, location, participant details, and equipment list in place, we then asked ChatGPT to identify the potential hazards of this course and assess the overall risk of this activity from low to high (Appendix F). The results were mixed: in some instances, ChatGPT was able to identify hazards specific to this activity (like the increased risk of slipping on seaweed-covered rocks exposed at low tide), but in other instances, ChatGPT exaggerated threats. For example, we find the risk of students suffering from physical strain and fatigue of carrying bags of collected litter to be low, and risks associated with waves ‘potentially causing participants to be caught off-guard or swept away’ to be highly unlikely given this activity will take place at low tide. Overall, ChatGPT provided a comprehensive list of hazards associated with the marine litter activity and suggests practical measures to mitigate each risk. It also broadly aligns with guidelines for planning safe fieldwork, such as careful planning, hazard identification, risk evaluation, communication, and management strategies, as outlined by Daniels and Lavallee (Citation2014). We recommend that ChatGPT-generated risk assessments can be further improved through an iterative prompt engineering process, where further specific questions are asked leading to a more focused response (i.e. likelihood of specific hazards occurring, potential consequences, measures to mitigate specific risks, or any other relevant queries) which can then be added or incorporated into the risk assessment as needed.
Then, we directed ChatGPT to provide input into the teaching and assessment component of this field activity. This included outlining the structure of a brief 15-minute introductory talk on marine litter to prepare students at the beginning of the field activity (Appendix G), provide a series of ideas for the coursework and assessment (Appendix H, I), elaborate on how students would analyse the data, and finally, produce a marking rubric for the assessment (Appendix I).
The introductory talk was very well structured and flowed logically, i.e. introducing the topic, providing a background on the different types of marine litter and their sources, the impact this would have on the environment and marine life, the importance of the activity and beach clean, tips for an effective clean, and then a ‘hearts and minds’ conclusion highlighting the importance of individual action for environmental protection (Appendix G).
Similarly, the types of assessments offered were varied and appropriate for the topic, ranging from writing an environmental impact assessment to a group project for a community engagement initiative to raise awareness about marine pollution and its impact on local marine ecosystems (Appendix H). We decided to further develop one of these coursework ideas ‘Data analysis and visualisation’ and asked ChatGPT to elaborate on how the students would collect, analyse, and then present data collected from the beach litter activity (Appendix I). ChatGPT provided a breakdown of the data to be collected, including categorising the type or debris (plastic, metal, organic waste, etc.), the quantity, size, location, and condition (intact or degraded), and provided a rationale for why the data was important. For example, recording litter condition could provide insights into the persistence and durability of different types of marine debris.
Continuing with the data analysis component of the coursework, we prompted ChatGPT to provide a title for this assessment, the learning objectives and then a marking rubric (Appendix J). The given title was generic and repetitive (Analysing Marine Debris: Data Analysis and Visualisation), but this aspect of the coursework description is of lowest priority and easiest to change. ChatGPT then listed four learning objectives that were varied, consistent with the marine litter theme, and appropriate for the analysis and visualisation of data collected during fieldwork. Finally, the marking rubric was spot-on, and included four main components (Data Analysis, Data Visualisation, Report Organisation and Clarity, Reflection and Critical Thinking) that were appropriately weighted (40:40:15:5) and then sub-compartmentalised with a description of the marker’s expectations (i.e. ‘demonstrates a thorough understanding of the collected data and its implications for marine pollution’ in the ‘Data Analysis’ section, or ‘uses proper grammar, punctuation, and formatting, and adheres to any specified report guidelines’ in ‘Report Organisation and Clarity’).
In summarising the teaching and assessment component provided by ChatGPT, the field course briefing, assessment design, and marking rubric were all competently produced and provided a robust workflow that could be easily changed, expanded, or customised as required. This support enables academics to focus on refining and adapting the generated content to their specific needs, ultimately enhancing the overall quality of the coursework and assessment process.
Case study 2: Integrating ChatGPT in field course design (specified subject and location)
Coastal hydrological processes
In the second case study, we prompted ChatGPT to design a one-day field trip to study hydrological processes and their impact on coastal habitat management on the Gower Peninsula, Southwest Wales, for a class of 20 Level 4 (first-year) undergraduate students enrolled in a Coastal Ecology module. ChatGPT suggested a full-day course structure with visits to 5 sites on the Gower Peninsula, each with a focus on a different hydrological process (Appendix L). The sites included Rhossili Bay, the Loughor Estuary, and Burry Inlet (), and the processes to be assessed included coastal erosion, sediment transport, runoff, and freshwater seepage.
Figure 1. Google map of the initial ChatGTP-generated field trip itinerary, taking a total of 2 hours and 23 minutes driving time.
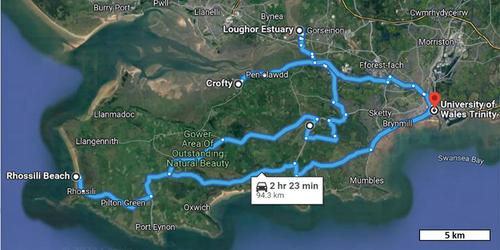
The itinerary included a lunch break in the middle of the day and ‘group discussion and recommendations’ following the site visits, prior to returning to the start location. ChatGPT did not suggest course learning objectives, specific start/end locations or times, or specific methodologies for assessing the hydrological processes until further prompted. Moreover, given the specific geography of the Gower Peninsula, there were several problems with this proposed course structure. First, travel to and from the sites was 2 hours and 23 minutes (), and assessing all sites in one day would be an estimated 10–14-hour endeavour. While there may have been sufficient daylight hours at the time of the prompt (early June), a 14-hour field trip would likely lead to boredom and exhaustion – serious safety considerations on a field trip in a potentially dangerous area with high cliffs and large waves, high tidal range, and deep mud. Similarly, ChatGPT did not recognise that several of the suggested assessments would need to be conducted at low tide, and the length of the suggested course would necessitate working throughout an entire tidal cycle. Finally, ChatGPT made no suggestions related to field trip health and safety issues, such as risk assessments and general health and safety standard operation procedures.
We next prompted ChatGPT to explain in detail how the various assessments would be conducted. ChatGPT produced 2 sentences of methodology for each of the processes being studied (Appendix M). However, ChatGPT did not fully differentiate between what assessments would be conducted and how they would be conducted. There was only a cursory coverage of equipment needed, and no detail with respect to the number of samples to be collected. For example, ‘conduct soil infiltration tests’ is meaningless to someone unfamiliar with that technique, as is ‘using sieves and sedimentation techniques’. ChatGPT did not produce the level of detail necessary for students to understand how the data were to be collected. To extract the necessary level of detail for the assessment methods, we had to iteratively prompt ChatGPT to provide a detailed step-by-step methodology, including all necessary materials and equipment, for each individual activity (i.e. a separate prompt for each assessment).
We then prompted ChatGPT to use downtown Swansea as the departure and return point for the trip and asked if it would be possible to complete the trip within an 8-hour window. ChatGPT produced the following output:
Traveling from downtown Swansea to all five sites within the Gower Peninsula and completing comprehensive assessments within an 8-hour day might be challenging. The Gower Peninsula is a diverse area with varying distances between sites, and assessments require time for observation, data collection, and analysis. To ensure a meaningful and thorough field trip experience, it is important to allocate sufficient time at each site. Considering travel time and the need for breaks, it is advisable to prioritize and focus on a selection of sites to ensure a more in-depth exploration and assessment.
The words ‘might be challenging’ are rather an understatement, as it would be physically impossible for a group of 20 students to perform all the necessary assessments at all 5 sites within 8 hours (including travel time). ChatGPT did correctly advise us to prioritise the sites to finish within the allotted time.
We then prompted ChatGPT to prioritise the sites and assessments based on an 8-hour trip from departure to return (Appendix N). ChatGPT then suggested spending 1 hour at Rhossili Bay for ‘site exploration, observations, and discussions’, including conducting both visual observation of erosion and beach profile measurement. Even using simple beach profile measurements, like the Emery method, a wide beach like Rhossili can take a long time to profile (Andrade & Ferreira, Citation2006), and 1 hour would likely be insufficient time to conduct both visual observations and beach profiles. Approximately 1.5 to 2 hours were allocated for assessment of tidal processes at Loughor Estuary, followed by 30 minutes to an hour for lunch. Following lunch, an hour was allocated for assessing agricultural runoff impacts at an unspecified site, and another hour for assessing freshwater seepage dependent habitats, again at an unspecified site. Finally, one hour was allocated to conducting water quality testing at the Burry Inlet. Note that the time at each site included discussion of the processes in addition to the data collection.
Note that ChatGPT returned the same 5 site visits and did not prioritise any of them over the others – it simply suggested how much time should be spent at each. It was apparent that site visits 3 and 4 will be conducted in the Loughor Estuary. The trip was still too long to fit into an 8-hour day. In our experience, the 5.5 hours available after travel time would be inadequate to conduct all the suggested assessments and have meaningful discussions between the faculty and students, especially given the fact that students would not be experienced with the methods being performed. Knowing that the trip involved too much travel and fieldwork to realistically fit into an 8-hour duration, we prompted ChatGPT to suggest alternative sites closer to the Swansea departure point. The prompt text was: ‘Considering the above processes and the time needed to assess them, suggest alternative sites that would be closer to Swansea but still suitable for demonstrating the hydrological processes and their interaction with coastal development’. ChatGPT suggested several sites more proximate to Swansea, including Caswell Bay and Swansea Bay (Appendix O):
Using these sites reduced the total duration sufficiently to finish the course within the allotted time, but the order of site visits needed to be changed to achieve the lowest travel time (). We next prompted ChatGPT to regenerate the field trip proposal using the new sites, assuming a start time of 8:30 am, an end time of 4:30 pm, and tide tables from June 3–9, 2023. We asked for a specific itinerary, with arrival and departure times at each site. We also asked for further detail of the methodology for each assessment. This field course plan had sufficient itinerary and methodological detail, but generated incorrect high and low tide times that were less than 2 hours apart. This is a clear example of AI hallucination (Alkaissi & McFarlane, Citation2023; OpenAI, Citation2022) and may have resulted from ChatGPT not having access to recent (post-2021) data on the Internet, including 2023 tide tables. However, tide tables are available years in advance, so this failure was surprising.
Figure 2. Google map of the revised ChatGTP-generated field trip itinerary, taking a total of 1 hour and 6 minutes driving time.
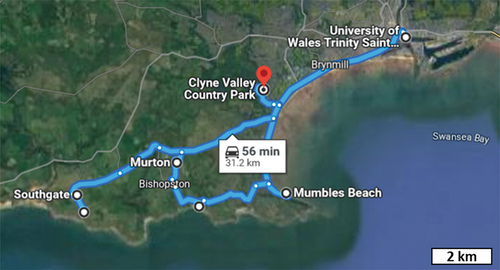
Finally, we prompted ChatGPT to include learning objectives, coursework assessments, and a risk assessment for the course. The proposed learning objectives (Appendix P) focused on assessing the impacts of coastal erosion, runoff and drainage on coastal habitats and the biodiversity they support. These were appropriate learning objectives that are similar to what we as faculty would have suggested for the Gower Peninsula field trip. It appeared that ChatGPT had a good handle on the purpose of the field trip and what students would need to learn in order to understand typical coastal hydrological processes and their impact on coastal habitat management. However, the language in one of the learning objectives (‘Understand the role of hydrological processes in coastal habitat management’, Appendix P) could be improved by using appropriate terminology such as ‘classify’, ‘describe’ or ‘explain’.
For suggested coursework assessments, ChatGPT returned a range of 7 activities (Appendix Q), five of which were related to course content rather than assessment per se. Only items ‘report writing and presentation’ and ‘reflective exercises’ describe actual assessments. The risk assessment produced by ChatGPT included a list standard risk assessment item (Appendix F), including physical risks such as slips and falls, environmental exposure to adverse weather, and health risks such as plant or insect allergies, and suggested mitigation steps for each risk. Note that ChatGPT did not mention any of the risks associated with travel to and from the sites (i.e. driving/road safety). This was a serious oversight in health and safety protocol (Love & Roy, Citation2019).
Once the field course curriculum and itinerary were complete, we compared the ChatGPT output to our original human-designed field trip (). The output was very similar to our original design, but with some key oversights, particularly in terms of trip logistics, as discussed above. We then prompted ChatGPT to conduct a self-critique of its own course design (Appendix R). Interestingly, ChatGPT considered its plan feasible in a general sense, but it identified several issues that it had not considered when initially designing the course. These included site access and permissions, collaboration with local stakeholders and experts, and availability of functioning equipment and other resources. It appears that ChatGPT learned from the iterative process of prompt engineering, which involved a series of questions aimed at extracting further information on course design. We suggest that prompting ChatGPT for a post-design self-critique is a useful step in ensuring the feasibility of AI-designed field course itineraries.
Table 1. Comparison of human-generated vs. ChatGPT-generated marine field course design in case study 2.
In summary, the key lesson from comparing human-generated and ChatGPT-generated field course designs is that ChatGPT can rapidly produce a competent course design that is generally quite similar to human designs, saving time and effort and reducing faculty workload. However, we found that the human design gave greater emphasis to the connections between the fieldwork conducted and the broader socio-economic aspects of coastal zone management. In addition, the human design held an advantage in terms of practical issues, such as ensuring site accessibility and feasibility of travel times and distances, scheduling shoreline tasks at low tide, and setting practical assessments of relevant field skills. These advantages likely stemmed from years of practical experience in conducting field courses that ChatGPT could not duplicate without specific human input. Despite this, ChatGPT can be used to build a solid field course design that is complemented and improved by human experience.
Best practice workflow
We found that ChatGPT was broadly competent at planning and designing all components of a marine field course, from producing ideas for course topics and activities, to providing detailed and relevant equipment lists and risk assessments, then subsequently designing a robust workflow for coursework and assessment. ChatGPT was proficient at designing an original course (Case Study 1) but was also capable of designing a course to fit the requirements of a pre-existing curriculum (Case Study 2). From these Case Studies, we developed a series of best practices for producing optimal ChatGPT output for field course design (), which we detail below.
Figure 3. Best practice workflow for ChatGPT prompt engineering in field course design, including optimal input and adaptive management principles.
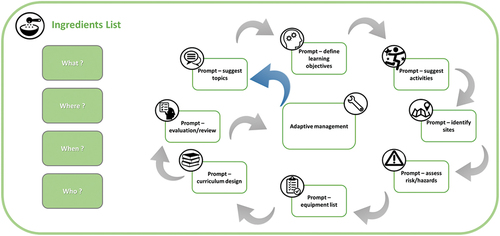
Ingredient list
Optimal output necessitates optimal input; prompt engineering requires a detailed list of factors, requirements, and conditions (an ‘ingredient list’ of what, where, when, who – see ), which provide guide rails for ChatGPT’s responses. For example, to produce a useful equipment list, the prompt should detail the activity, location, type of environment, and cohort size, so that the list will provide the correct equipment for the specific number of students (Case Study 1, Appendix E). To produce an optimal schedule, the prompt should indicate the desired date, start and end time, and environmental conditions (e.g. tides, daylight hours) (Case Study 2, Appendices M and N). Offering relevant background information and setting context will ensure that the model understands the query better.
Go with the flow
The specific order of prompts and the structure of the conversation is key to obtaining good responses. ChatGPT can answer each individual prompt well, but to achieve optimal results, prompts should be given in a logical order. Prompt engineering is an iterative process that often requires experimentation and refinement to achieve the desired outcomes. For example, in Case Study 2, Appendix L, ChatGPT suggested what type of hydrological assessments needed to be done at each site but did not describe how to do them. A separate prompt asked ChatGPT for a step-by-step methodology for each assessment was needed to obtain the necessary information (Appendix M). ChatGPT works best when using a continuous conversation that builds on each successive prompt (ChatGPT remembers all previous info within a given conservation).
Due diligence
As ‘hallucinations’ are a well-documented phenomenon in ChatGPT (Alkaissi & McFarlane, Citation2023; OpenAI, Citation2022), output requires due diligence. Both case study outputs included an element of hallucination. In Case Study 1, ChatGPT suggested a site (St. Ives Bay Marine Conservation Zone – Case Study 1, Appendix I) that we cannot confirm as a Marine Conservation Zone on official government websites. In Case Study 2, ChatGPT suggested a field course itinerary that was unrealistic due to fictional tide times (Appendix N), and schedules had to be adjusted to coincide with the actual tidal cycle. This illustrates the need for human judgement, particularly with respect to course logistics.
Adaptive management
We recommend consulting ChatGPT on the information it requires to ensure effective prompt engineering for course design. For example, we asked ChatGPT what questions would be useful for designing a field course. ChatGPT suggested several questions, mainly focused on objectives, resources available, safety considerations, and promoting active participation. We used similar questions throughout our case studies to improve the iterative prompts. For example, we queried ChatGPT for any additional topics or interdisciplinary aspects that could be integrated into the field course to enhance its educational value (Appendix S). ChatGPT made several useful and interesting suggestions that would increase interdisciplinarity. This approach helps to improve the overall field course design by ensuring a more comprehensive output.
Additionally, we recommend adaptive management (Galimov & Khadiullina, Citation2018; Hecht & Crowley, Citation2020) of the course design () by retrospectively prompting ChatGPT to evaluate its output and updating the design accordingly (Appendix K). For example, in Case Study 1, ChatGPT queried the long-term impact of the beach clean-up activity and suggested follow-up initiatives that translate into sustained behavioural changes beyond the duration of the field course. This is a sensible suggestion that is easily incorporated into post-field trip lectures or other educational activities. Asking these questions helps refine and enhance the design of the field course and flags any potential pitfalls or blind spots.
Conclusions
Although our case studies focused on marine biology and environmental topics, ChatGPT could potentially be used for many field course designs and could competently complete most of the tasks involved, from choosing learning objectives and activities to assessment and evaluation. Based on our in-depth experience of fieldwork and field course curriculum design, ChatGPT is highly efficient and can reduce the time and effort involved in course design. Future studies could look to evaluate ChatGPT-designed field courses against student feedback and develop other field courses beyond those in the marine sciences. We have provided a series of best practices to prompt engineer a successful field course design, including optimising input, logical flow of prompts, due diligence of output, and adaptive management to evaluate and improve course design. ChatGPT can be piloted to excellent results by experienced prompt engineers who understand all the variables that make a field course successful. Without those variables, ChatGPT may deliver suboptimal output. Thus, the human dimension is still necessary to achieve the best results.
Supplemental Material
Download MS Word (52.8 KB)Acknowledgments
We acknowledge the role of ChatGPT in the development of this paper and the feedback from three anonymous reviewers.
Disclosure statement
No potential conflict of interest was reported by the author(s).
Supplementary material
Supplemental data for this article can be accessed online at https://doi.org/10.1080/14703297.2024.2316716.
Additional information
Notes on contributors
Mark Tupper
Mark Tupper is a Senior Lecturer in Marine Biology at the University of Portsmouth and a Marine Ecosystem Scientist at CGG Services (UK) Ltd. His research focuses on fish habitat ecology, with a specialisation in coral reef fishes. He also consults extensively in the fields of fisheries management and coastal and marine environmental impact assessment. He has over 20,000 hours of fieldwork experience and field course curriculum design in coastal and marine environments. You can find out more about his publications here: https://scholar.google.com/citations?user=VPI8yNAAAAAJ&hl=en&oi=ao
Ian W. Hendy
Ian W. Hendy is a Senior lecturer in Marine Biology at the University of Portsmouth. His research focuses on tropical marine biology, including mangrove forest ecosystems. He has over 20,000 hours of fieldwork experience and field course curriculum design, including work in Indonesia, Mexico, and the UK. You can find out more about his publications here: https://scholar.google.co.uk/citations?user=ibTxTy8AAAAJ&hl=en&oi=ao
J. Reuben Shipway
J. Reuben Shipway is a Lecturer in Marine Biology at the University of Plymouth, National Geographic Explorer, and Editorial Board Member for the Royal Society journal, Biology Letters. He has over 10,000 hours of fieldwork experience and field course curriculum design, including work in Central America, Europe, North America, and SE Asia. You can find out more about his publications here: https://scholar.google.com/citations?user=EzEIEVMAAAAJ&hl=en
References
- Alkaissi, H., & McFarlane, S. I. (2023). Artificial hallucinations in ChatGPT: Implications in scientific writing. Cureus, 15(2). https://doi.org/10.7759/cureus.35179
- Andrade, F., & Ferreira, M. A. (2006). A simple method of measuring beach profiles. Journal of Coastal Research, 224(4), 995–999. https://doi.org/10.2112/04-0387.1
- Atlas, S. (2023). ChatGPT for higher education and professional development: A guide to conversational AI. https://digitalcommons.uri.edu/cba_facpubs/548
- Biggs, J. B., & Tang, C. (2011). Teaching for quality learning at university. McGraw-Hill/Society for Research into Higher Education/Open University Press.
- Cotton, D. R. E., Cotton, P. A., & Shipway, J. R. (2023). Chatting and cheating: Ensuring academic integrity in the era of ChatGPT. Innovations in Education and Teaching International, 1–12. https://doi.org/10.1080/14703297.2023.2190148
- Daniels, L. D., & Lavallee, S. (2014). Better safe than sorry: Planning for safe and successful fieldwork. The Bulletin of the Ecological Society of America, 95(3), 264–273. https://doi.org/10.1890/0012-9623-95.3.264
- Department for Environment. (2019). Marine conservation zones: Cape Bank, GOV.UK. https://www.gov.uk/government/publications/marine-conservation-zones-cape-bank
- Durrant, K. L., & Hartman, T. P. (2015). The integrative learning value of field courses. Journal of Biological Education, 49(4), 385–400. https://doi.org/10.1080/00219266.2014.967276
- Dwivedi, Y. K., Kshetri, N., Hughes, L., Slade, E. L., Jeyaraj, A., Kar, A. K., Baabdullah, A. M., Koohang, A., Raghavan, V., Ahuja, M., Albanna, H., Albashrawi, M. A., Al-Busaidi, A. S., Balakrishnan, J., Barlette, Y., Basu, S., Bose, I., Brooks, L. … Wright, R. (2023). Opinion paper: “so what if ChatGPT wrote it?” multidisciplinary perspectives on opportunities, challenges and implications of generative conversational AI for research, practice and policy. International Journal of Information Management, 71, 102642. https://doi.org/10.1016/j.ijinfomgt.2023.102642
- Galimov, A. M., & Khadiullina, R. R. (2018). Conceptual foundations of adaptive management of higher education based on the integrated approach. Modern Journal of Language Teaching Methods (MJLTM), 8(7), 70–75.
- Geange, S. R., von Oppen, J., Strydom, T., Boakye, M., Gauthier, T. L. J., Gya, R., Halbritter, A. H., Jessup, L. H., Middleton, S. L., Navarro, J., Pierfederici, M. E., Chacón‐Labella, J., Cotner, S., Farfan‐Rios, W., Maitner, B. S., Michaletz, S. T., Telford, R. J., Enquist, B. J., & Vandvik, V. (2021). Next‐generation field courses: Integrating open science and online learning. Ecology and Evolution, 11(8), 3577–3587. https://doi.org/10.1002/ece3.7009
- Hecht, M., & Crowley, K. (2020). Unpacking the learning ecosystems framework: Lessons from the adaptive management of biological ecosystems. Journal of the Learning Sciences, 29(2), 264–284. https://doi.org/10.1080/10508406.2019.1693381
- Kiryakova, G., & Angelova, N. (2023). ChatGPT—A challenging tool for the university professors in their teaching practice. Education Sciences, 13(10), 1056. https://doi.org/10.3390/educsci13101056
- Love, T. S., & Roy, K. R. (2019). Field trip safety in K-12 and higher education. Technology and Engineering Teacher, 78(7), 19–23.
- Martin, B. R. (2016). What’s happening to our universities? Prometheus, 34(1), 7–24. https://doi.org/10.1080/08109028.2016.1222123
- Metz, A. (2022). Six exciting ways to use ChatGPT – from coding to poetry. TechRadar. https://www.techradar.com/features/6-exciting-ways-to-use-chatgpt-from-coding-to-poetry.
- Napper, I. E., & Thompson, R. C. (2020). Plastic debris in the marine environment: History and future challenges. Global Challenges, 4(6), 1900081. https://doi.org/10.1002/gch2.201900081
- OpenAI. (2022). ChatGPT: Optimizing language models for dialogue. https://web.archive.org/web/20230225211409/https://openai.com/blog/chatgpt/.
- Orr, R. B., Csikari, M. M., Freeman, S., Rodriguez, M. C., & Wilson, K. J. (2022). Writing and using learning objectives. CBE—Life Sciences Education, 21(3), fe3. https://doi.org/10.1187/cbe.22-04-0073
- Pace, F., D’Urso, G., Zappulla, C., & Pace, U. (2021). The relation between workload and personal well-being among university professors. Current Psychology, 40(7), 3417–3424. https://doi.org/10.1007/s12144-019-00294-x
- Rudolph, J., Tan, S., & Tan, S. (2023). ChatGPT: Bullshit spewer or the end of traditional assessments in higher education? Journal of Applied Learning & Teaching, 6(1), 342–362.
- Salvagno, M., Taccone, F. S., & Gerli, A. G. (2023). Can artificial intelligence help for scientific writing? Critical Care, 27(1), 1–5. https://doi.org/10.1186/s13054-023-04380-2