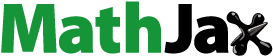
ABSTRACT
The depletion of conventional energy resources has created a bunyanesque energy problem in the current world order. In this article, the byzantine issues of optimisation i.e. modelling, simulations, sustainability assessment and economic analysis examples are discussed briefly. Life span analysis and associated capital costs for various solar drying systems are elucidated with comparative graphics for insights into the economic benchmarking of different models. This compendium work highlights the suitable combinations of numerical modelling and simulation softwares frequently used. In addition, the recent studies on environmental assistance are reviewed which is a crucial parameter for future studies on solar dryer optimisation as they should not only limited to exergy-sustainability criteria but to expand the studies on social impact as well.
Highlights
Modelling, Simulations, Environmental and Economic assessments are the most pertinent approaches that evolved in optimising solar dryers.
GA-ANN and MATLAB/CFD-ANN have emerged as contemporary combinations for experimental modelling and validation.
The capital costs of solar dryers could range from 100- 2000 USD depending on the model of the dryer.
The lifespan range of solar dryers typically ranges between 10–25 years.
Nomenclature | ||
= | Response data | |
= | Number of experiments or treatments | |
= | Coefficient of determination | |
= | Root mean square error | |
= | Reduced Chi-square value | |
= | Annualised cost of dryer | |
= | Annualised capital cost | |
= | Annualised Salvage value | |
= | Blower running cost of electricity | |
= | Capital cost | |
= | Capital recovery factor | |
= | Number of hours blower runs in a year | |
= | Rated power of the blower | |
= | Unit cost of electricity | |
= | Salvage value | |
= | Salvage fund factor | |
= | interest rate on long-term investment | |
= | Salvage fund factor | |
= | Drying cost per Kg of the final product | |
= | number of days dryer runs annually | |
= | Mass output of dryer per batch | |
= | Number of days per batch | |
= | Annual product outcome of drying system | |
= | Cost of fresh product per Kg of dried product | |
= | Cost of fresh product per Kg | |
= | Drying capacity of dryer per batch | |
= | Moisture content | |
= | Initial weight of product | |
= | Final weight of product | |
= | Efficiency of the drying system | |
= | Heat utilised in KJ | |
= | Heat consumed in KJ | |
= | Thermal efficiency of the drying system | |
= | Mass flow rate (Kg/s) | |
= | Specific heat of air (J/kg/oC) | |
= | Collector area (m2) | |
= | Temperature difference between inlet and outlet of temperature | |
= | Solar Radiation intensity (W/m2) | |
= | Weight of removal of water content in kg | |
= | Latent heat of vapourisation in kJ/kg | |
= | Energy consumed for running the fan or the blower (Watts) | |
= | Mass of biomass in Kg | |
= | Lower calorific value in kJ/kg. | |
= | Exergy in Watts | |
= | Inlet of Exergy in Watts | |
= | Outlet of Exergy in Watts | |
= | Exergy efficiency |
1. Introduction
In the twenty-first century, the whole world is in pursuit of sustainable innovations. Among the several renewable sources, solar energy has emerged as one of the frontrunner solutions. Moreover, solar solutions have emerged as one of the most user-friendly technologies for energy generation. Apart from power generation, there is a vast range of solar energy applications. Solar thermal applications are one such example. Drying is one of the major concerns for agricultural products. Solar drying has been a traditional solution in most developing nations, primarily in rural areas. Though open sun drying is used extensively, it also has some cons. One of the major concerns is intermittency. Research is being carried out worldwide to find solutions for ensuring continuous drying through hybridisation. Several factors affect the drying performance of the solar dryer. Also, the drying phenomena depend on the product under observation. Today, the usage of solar dryers is not only limited to agricultural and farming products. It has vast industrial use potential in textile, waste sludge, wood, pharma, and cement industries. There is considerable scope in understanding the solar drying potential, especially in industrial processes. Energy and exergy analysis could be very useful for understanding the efficiency of these processes (Pirasteh et al. Citation2014).
Industries, mainly big commercial manufacturers, include several dryers such as freeze, steam, spray, etc. for largescale drying of intermediate or final products. However, the potential of solar dryers remains untapped in big industries. The possible reason could be the efficiency of solar drying in comparison to other drying techniques. Henceforth, its usage is mainly confined to rural areas or lower-level business agricultural firms. Several other factors make solar dryers more friendly to agriculture-based products. First, it gives farmers a cheap and faster mechanism to dry the harvest. The harvest could be of any form, whichever needs drying. Second, it has been observed that drying the products at optimal temperatures keeps the product’s nutritional value and makes it hygienic.
Optimised by efficiency and working conditions, the dryers could help the farmers significantly enhance their revenue. There are two broad classifications of solar dryers based on airflow: the active type (forced convection) and the passive type (natural convection). The user needs to understand the drying requirements of the product before selecting and fabricating the solar dryer (Sharma, Chen, and Vu Lan Citation2009). Conduction, convection, and radiation are the three basic modes of heat transfer. Solar dryers primarily work on the combinations of these modes of heat transfer. Several factors are vital in designing or fabricating solar dryers: portable design, thermally efficiency, and cost-effectiveness. A study by Chavan et al. (Citation2021a) reveals that a conduction mechanism could consolidate the innovations in solar drying techniques in the case of natural convective and direct-type dryers. Airflow and temperature are the most important parameters affecting the drying phenomenon, among several other factors. The other parameters, which govern the optimisation, could be the sun’s radiation, the air’s humidity, the load of product, and resistance to airflow. Spoilage of crops and food products is a big concern for rural farmers with limited sources. Thus, a user-friendly low-cost solar drying solution could be a boon for them. Air and temperature control circulation should be of prime concern in manufacturing such dryers. Hence, the dryers, which are scientifically optimised, are better performing (Prakash and Kumar Citation2013).
One of the big reasons for the low acceptability of solar dryers is their intermittent nature. Energy storage is the only option to curb this inconsistency and provide the user with an optimised dryer with continuous drying support. There are principally three ways to store energy in the context of solar dryers, which include mechanical, thermal, and electrical storage of the energy. The efficiency of the solar dryers is hard to maintain, especially in low-temperature conditions. The solar dryers working in lower temperature range thus need optimisation, several approaches for such optimisation is presented schematically in (). The process of enhancing the performance of the equipment under given conditions is known as ‘optimization.’
Figure 1. Numerical methods, Simulation tools, and Economic assessments and simulation criteria for optimisation of solar drying systems.
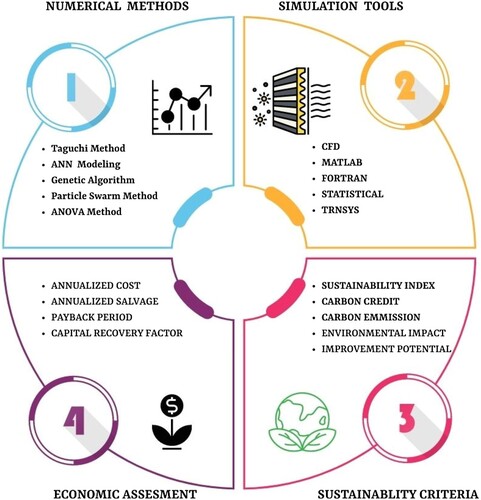
This work is an attempt to provide cynosure to the optimisation techniques for solar drying systems by briefly focussing on objectives as mentioned below:
To discern and analyse the most common optimisation approaches prevalent is solar drying systems.
To understand the methodology of numerical and mathematical modelling used in optimising solar dryers.
To comprehend the various simulation tools and software packages and their utility in optimisation with particular products and types of dryers.
To inspect the role of sustainability criteria in optimising solar drying systems.
To delve into the possible parameters and calculations involved in the economic optimisation of various dryers.
2. Background knowledge on solar drying
Moisture removal from a product during drying in any form needs the simultaneous transfer of mass and heat, which affects the product’s physical structure and chemical composition. Through a variety of mechanisms, including moisture and liquid diffusion, vapour diffusion, etc., moisture is transferred from the inside surface of the product to the outside surface. Some of the key parameters must be carefully considered in order to comprehend the entire drying process such as moisture content (dry and wet basis), moisture balance content of moisture content models, falling and constant drying times, thin layer drying rate, deep bed drying, drying rate, dryer efficiency, and drying constant. The drying performance for an organic product in any drying condition is evaluated by the wet and dry moisture content as below mentioned in Equations (1) and (2) (Prakash and Kumar Citation2020).
(1)
(1)
(2)
(2)
The most crucial factor used to evaluate the dryer’s performance is drying efficiency. It is the proportion of heat used to all heat used throughout the drying process. In addition, the ratio of heat used to remove moisture from a product to the total heat produced by thermal energy is known as the thermal efficiency of a solar dryer. It can be stated mathematically as follows respectively:
(3)
(3)
(4)
(4)
The heat transfer in various types of solar dryers can be categorised into three categories based on the design and mode of convection. Henceforth, the system efficiency calculation is also different for the three cases as mentioned below (Prakash and Kumar Citation2020).
Natural Convection solar dryer,
(5)
(5)
Forced convection solar dryer,
(6)
(6)
Hybrid-type solar dryer,
(7)
(7)
Another considerable part of calculations is ‘Exergy of the system’. The second law of thermodynamics serves as the foundation for exergy analysis assessment of thermal efficiency, which is related to mass, heat, and work transfer in the given system.
(8)
(8)
(9)
(9)
(10)
(10)
By evaluating innovative solar dryers only on the basis of these metrics, researchers have validated their designs. In general, dryer is first put through a no-load test in order to determine its thermal profile. However, these calculations are part of the modelling and validation process considering the design and performance of all types of solar dryers which is discussed briefly further.
3. Modelling based optimisation
A mathematical model describes the characteristics and attributes of a real system in terms of mathematical variables and operations. Depending on the foundation from which a model is built, mathematical models can be broadly divided into physics-based and observation-based models. The experimental data are the foundation on which observation-based models are formed and are empirical in nature. The universal physical principles that are supposed to characterise the presumptive physical occurrences are the starting point for physics-based models, in contrast. Experimental data are used to evaluate physics-based models as well, however with physics-based models, the experimental data are not required to exist prior to the model. To understand the suitability of the optimisation technique, it is essential to understand the interlinked relationships of the various dependent or independent parameters. Mathematical modelling is inevitable to understand the drying kinetics of the drying matter. The modelling of the process considering drying conditions holds percipient significance in the overall optimisation of solar dryers. The modelling analysis is pivotal in designing and controlling the dryer process. The shapes, heat transfer module, and type of agricultural product have a critical role, and mathematical models help get better insights into it (Tzempelikos et al. Citation2015). Some of the modelling techniques frequently used in solar dryer optimisation are as below (Joshi, Kumar, and Baredar Citation2019a):
Taguchi Method Modelling
Artificial Neural Network Modelling
Genetic Algorithm Modelling
Particle Swarm Modelling
ANOVA Modelling
3.1. Taguchi method modelling
Many researchers and scientists for optimisation use Taguchi method, named after Dr. Taguchi, Japan, extensively. In Taguchi’s optimisation method, the main focus is to understand the best form of control factors. The objective functions are the log functions of the desired output variables affecting the drying process. The experimental data is combined with optimisation of control parameters under investigations. The optimisation problems through Taguchi’s method are generally classified as static or dynamic. The signal-to-noise ratio analysis is the critical factor of this methodology. This method is widely accepted in optimising devices, equipment, materials, and processes. The control parameter levels are evaluated for better results by maximising the signal-to-noise ratio. The methodology to investigate the optimisation of operations can be done following the steps mentioned in .
Taguchi model suggests that the combination of parameters having the lowest variability is the most optimal condition. To determine the variability S/N (signal-to-noise) ratio, the quality of parameters observed during the experiments is calculated. In the S/N ratio, the signal is considered the desired value, whereas noise is regarded as the undesired value. The ratio also shows how the observed data is scattered around the targeted data (Hussein et al. Citation2021). Experimental observations have two operational parameters: controllable (signal) and uncontrollable (noise). Signals are dependent on the person designing the system and cannot be changed by end-users.
Similarly, noise parameters cannot be controlled by the system’s designers (Boran Citation2006). Thus, the suitable S/N model is selected depending on the situation to optimise the process. The problems can be broadly classified into static and dynamic conditions depicted in .
(11)
(11)
(12)
(12)
(13)
(13)
Figure 3. Classification of Taguchi optimisation problems S/N ratio can be calculated by: (Hussein et al. Citation2021).
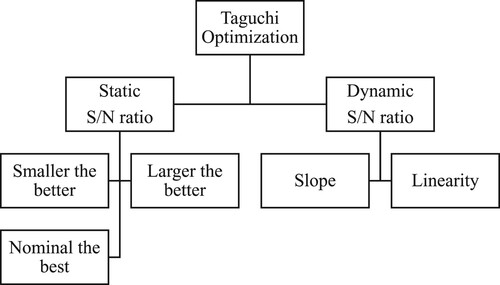
3.2. Artificial neural network modelling
The artificial neural network (ANN) is a computational model that derives its inspiration from working human biological features. The functional elements of these models are similar to the neurons of human brains. The connection between them with the coefficients forms the neuronal structure. The algorithms of the working structure are attached to these neuronal networks. The network works based on the connections inside the network hence sometimes referred to as ‘connectionist models.’
The background for ANN is Artificial intelligence (AI). Today in the age of fast technological advancements, AI-based solutions are getting more user-friendly. Any AI solution first understands the human brain’s behaviour through simulations and forms patterns based on rules. After this, mathematical models such as ANN come into the frame, which models this simulation in real-time solutions. ANN models are often used in combination for modelling drying systems. Dhalsamant (Citation2021) conducted experiments on potato cylinders using the FE model, which was validated with the ANN model and simulated with COMSOL software. ANN also served to be helpful in comparative drying capacities of the natural convective dryers with that of conventional electric dryers. This study used the CFD-ANN combination for modelling and simulation (Capossio et al. Citation2022).
3.3. Genetic algorithm modelling
The genetic algorithm (GA) is a field of operations research that deals with a heuristic approach to optimisation. The basis of GA and its operating parameters comes from the broad study of evolutionary algorithms. The parameters are derived from various natural phenomena such as mutations and crossovers. The two main components of GA optimisation are the genetic representatives and the fitness functions. The fitness functions help in evaluating the solution domain. In the field of solar drying, some researchers used GA optimisation sometimes for design optimisation and sometimes with fuzzy logic depending on the requirement of their study. Rahman et al. performed optimisation of solar drying of grains on this methodology. For this experiment, one of the parameters was varying, and the others remained fixed. The program was repeated five times, and its fitness was tested using MATLAB (Rahman et al. Citation2015). Saliha and Koksal compared the experimental outcomes of the neural network v/s GA optimisation in some studies related to thin-layer drying optimisation of carrots. The results revealed neural networks provided 0.05% better accuracy than GA-based results (Erenturk and Erenturk Citation2007).
The uniqueness of GA also lies in the fact that it is inspired by nature and chromosome structures. The first step is to define the length of the genetic strings. Rahman et al. performed experiments on mushroom drying with the GA approach of optimisation. The parameters of the study were moisture content and enzymatic activity. The GA approach showed promising results in the study with better values of amylase enzymes (Rahman et al. Citation2014). Curvelo Santana et al. conducted similar analyses for solar drying of corn malt using GA. The study aimed to maximise the movement of amylase enzymes in the dried product. The related simulations with GA provided promising results for the experiments (Santana et al. Citation2010).
briefly describes the steps that can be followed for a GA algorithm-based analysis. Firstly, the algorithm is initiated to solve any problem by defining the ‘population,’ which is the collection of the most probable solutions to the problem under study. These could be random sets of binary strings. After this, the selected population’s fitness is analysed, which determines the reproduction capability of the population. The better the score fitness higher the chances of reproducing. The pairing of the individuals is performed based on the selection of individuals paired. These paired individuals provide more effective solutions than the previous individuals. And the ‘Crossover’ basically swaps the gene information to produce new child individuals equal in size to their parent individuals, and ‘Mutation’ by flipping chromosomes adds further gene information to child individuals (Muthee Citation2021). Hence, the binary strings change before and after mutation. After these steps, the fitness reaches its threshold limit, and the algorithm is closed or terminated. This way, the GA algorithm analysis could be performed for solar drying and any other such analysis.
3.4. Particle swarm modelling
Eberhart and Kennedy proposed particle swarm theory to optimise problems related to nonlinear functions in 1995 (Eberhart and Kennedy Citation1995). Based on conceptualisation, the (Particle Swarm Optimisation) PSO is stochastic and lies in genetic and evolutionary optimisation techniques. The basic working methodology is based on two paradigms one is locally oriented based on particles called (PBEST), and another is globally oriented called (GBEST). PBEST considers particles taking the coordinates to find the local particles’ fittest, and GBEST finds the overall fittest values and location (Wang, Tan, and Liu Citation2018). Nowadays, PSO is used in most renewable energy problems and specifically solar-related optimisations. For example, Dong et al. used PSO optimisation to select parameters in support vector regression to forecast solar irradiance (Dong et al. Citation2015). The PSO can potentially be used as an optimisation tool for solar drying studies related to the drying of various agricultural products (Joshi, Kumar, and Baredar Citation2019b).
3.5. ANOVA (analysis of variance) modelling
ANOVA (Analysis of variance) is a statistical tool comprising the model to investigate the differences among mean values. It works on the law of total variance and reflects the different sources responsible for variation when substituted into components or variables. Statistician Mr. Ronald Fisher developed ANOVA in the early twentieth century to investigate experiments related to agricultural products. ANOVA works on simple logic with easy to feed input data. However, selecting the input variables is an arduous and complex part of the investigation (Larson Citation2008). In the context of solar drying optimisation, it is commonly used to investigate orthogonal experiments. Mustapha et al. performed an ANOVA analysis to microencapsulate volatile phase change materials (PCM). PCM plays a critical role in the efficiency optimisation of hybrid solar dryers. In such an analysis, the total variation is the sum of the squares of deviations and their error components (Mustapha et al. Citation2021). Also, ANOVA has been used to optimise the drying processes of various agricultural products. It can be used along with response surface methodology (RSM) to study optimal drying results. The parameter variation could reveal parameters’ dependence on the optimal results. For example in the case of ginger, the drying temperature and slice thickness affected the rehydration ratio but had almost no effect on relative humidity (Madamba Citation1997).
As shown in the ANOVA analysis could be performed using three different models based on the parameters. The fixed-effect model provides ranges of the response variables values for a given solution. On the other hand, the random effect model is used when solutions are not fixed. In such models, various factor levels are selected for massive datasets. The mixed model is commonly used as a mixture of fixed and random variables, and based on the problem statement, the model’s suitability is judged. In solar drying, the ANOVA model is used in limited content. However, with continuous technological growth and hybridisation of solar dryers, it could be potentially used for finding optimal results in optimisation.
3.6. Role of mathematical modelling in solar drying optimisation
To answer a particular set of unknowns in solar drying, a ‘model’ needs to be developed. There are several ways to do this, many of which are mathematical in nature. We could employ a set of differential equations, a definition of optimisation with constraints and goals, or distribution modelling based on stochastic or probabilistic processes to represent the system. In few examples are presented in which distinct studies are briefly presented. Depending on the type of product, dryer and the drying conditions the distinct methods which were dicussed in the previous sections are presented and can be selected as inspiration for future studies.
Table 1. Optimisation techniques using numerical/mathematical modelling.
Simulation-based optimisation
4.1. Simulations of solar drying systems
Modelling, any scientific system requires the design and validation of the system based on the various dependent and independent functions. Designers and engineers often use various simulation tools to validate the system’s efficiency, process, or optimisation. Among several other techniques of validation and optimisation, simulating the system is very common. It could also be referred to as a magic tool converting the inputs to outputs, given that the boundary conditions and operating functions are provided rightfully. The basic modelling process works in two stages. Stage one is where the designer imagines the system with the desired outputs and operating parameters. In the second stage, the interactive physic of the system and the environment is considered. These interactions are governed by various laws of sciences, which need numerical validation and optimisation. This process is the primary analytical approach followed by scientists and engineers in developing most systems. gives an insight into the role of inference in modelling. Sanchez described these modelling and simulation phenomena in (Sanchez Citation2007). Many modelling problems are solved analytically, but sometimes it requires an Algorithmic approach that needs computer programming support to study which we refer as ‘simulations’ often. This approach is necessary for long-chain inferences, where simulation comes into play.
Figure 6. (a) Inference in modelling stage (b) Inference in simulation stage (Sanchez Citation2007).
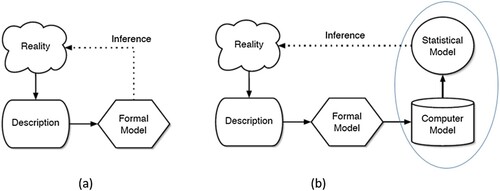
The analysis of the model need not be successful in the first attempt. Hence, various iterations are required to obtain a heuristic model overview. The simulation starts the model as a process step by step incrementally to obtain the correct or suitable boundary conditions. The more iterations, the better the output results get in the analysis – also, several attempts to define the best possible solutions considering several solutions. Several forms of simulation are being used in the solar drying field extensively.
Over time, the design structures of solar dryers have evolved in various forms. There could be multiple possible design structures based on the airflow movement inside the dryer. Also, the designs sometimes are based on the product to be dried. However, The design validation and overall performance of the solar dryer are also based on several other factors. Mathematical models and numerical analysis are vital in observing the drying behaviour, especially for food crops. The other essential parameters or functions affecting the performance of solar dryers could be drying rate, moisture content, temperature profile, statistical data, and the colour or texture of the product to be dried. Considering all these functions, the simulation of the solar drying systems becomes inevitable. The four broad classifications of the solar dryers are direct type, indirect type, mixed type, and Hybrid type. Chauhan et al. provided a brief study on the various simulation software frequently used in which type of dryer (Singh Chauhan, Kumar, and Tekasakul Citation2015). gives insight into the kind of dryer and related simulation platform commonly used in this study.
Figure 7. Frequently used simulation platforms classified on the design of the solar dryer (own creation) based on literature from Chauhan et al. (Citation2015).
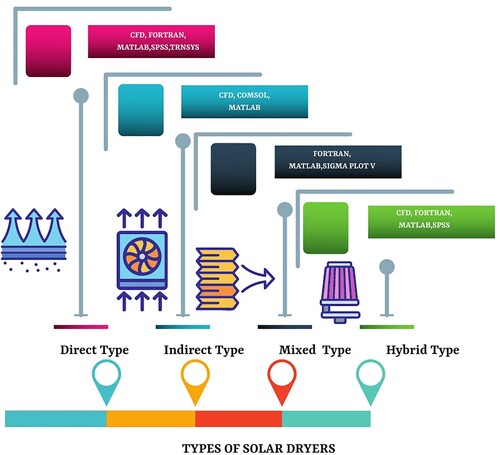
The design and development of a solar dryer vary from product to product. Sometimes, the environmental functions also come into consideration. Similarly, the numerical or the software model analyses or validates the solar dryer’s optimal performance. As suggested in , several platforms are available for the designer based on the type of dryer under investigation.
4.2. CFD (computational fluid dynamics) simulations
Computational Fluid dynamics is a software tool frequently used to optimise and analyse various types of solar dryers. It has been extensively used in the drying behaviours of a long-range of products. Benhamza et al. performed uniformity analysis using the ANSYS FLUENT software (Citation2021). The interrelation between quality of the product and the drying parameters could be investigated with this approach, along with image processing technology. The air movement inside the drying chamber also plays a vital role in the heat transfer capabilities of the solar dryer. Mainly in the big industrial batch type dryers, air velocity governs the drying rate to an extent. In such cases, CFD FLUENT could be used to observe the flow behaviour. In addition, many farmers use medium or large-scale greenhouse dryers for crop drying. Such greenhouse dryers require ample ventilation for the airflow inside the chamber (Bartzanas, Boulard, and Kittas Citation2004). FLUENT analysis can help optimise the ventilation size and the placement of the ventilators by generating airflow contours through the software. In some peculiar investigations, such as sludge drying also, it has been helpful to create mathematical models for the unsteady system investigations. In some specific studies, the CFD has been fruitful in comparative analysis between on-load and offload conditions, primarily in greenhouse dryers. The uneven or unsteady designs are complex to study and provide detailed numerical results (Mellalou et al. Citation2021). CFD analysis is recommended in such cases to visualise the actual flow matrix using substantive boundary conditions. Some examples are presented in and for visualisation of different parameters in solar drying ().
Figure 8. 3D streamline flow behaviour of airflow in inflatable solar dryer with (a) top view and (b) side view for drying of paddy rice (Salvatierra-Rojas et al. Citation2021).
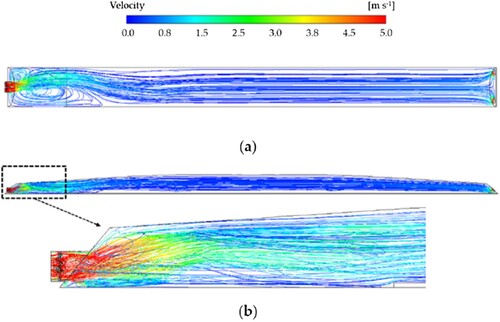
Figure 9. Difference between (a) simulated and (b) experimental temperature profiles over time along the length of an inflatable solar dryer for paddy rice drying (Salvatierra-Rojas et al. Citation2021).
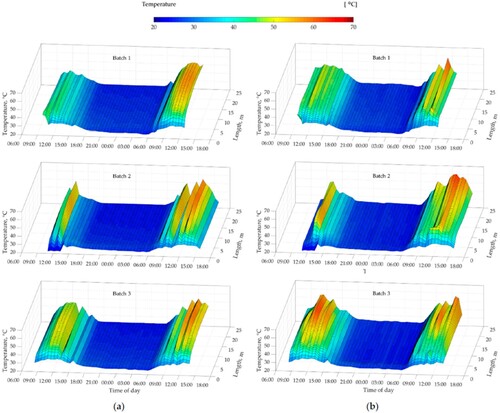
Table 2. Software tools and numerical model combinations using simulations.
4.3. MATLAB simulations
MATLAB has been an essential software in simulations related to solar drying systems. The software eases the overall process of optimisation through programming solutions. Such simulations using MATLAB provide a platform for numerical equations analysis and data visualisation through interactive algorithms. Wang et al. used MATLAB simulations and SAPGD-2004 as visualisation software to understand the drying dynamics in thin layer drying and deep bed drying of grains (Wang and Fon ). Prakash and Kumar developed an adaptive network-based fuzzy interference system (ANFIS) through MATLAB for a natural convective greenhouse solar drying system (Prakash and Kumar Citation2014a). The experiments were performed on various sizes of jaggery to simulate product temperature, the temperature inside the greenhouse, and the moisture removal rate. This approach demonstrates the feasibility of MATLAB utility in fuzzy interference systems. Similarly, Abubakar et al. (Citation2020) used MATLAB R2013a to subroutine data using TRANSYS 16.0. TRNSYS has been widely used in heating, ventilation, and air-conditioning (HVAC) related experiments for simulations. The software package is transient and provides insights into the thermal behaviour of the systems. The study investigated efficiency quotients and the corresponding root means square errors for drying yam slices. Hence, the TRNSYS package could be coupled with MATLAB simulations for better insights into solar dryers. Barnwal and Tiwari conducted MATLAB simulations to analyse the drying of grapes (Citation2008). They used MATLAB 7.0 to program an algorithm that helped evaluate the hourly variation of governing drying factors such as convective mass transfer coefficient, heat utilised for moisture removal, amount of moisture evaporated, etc. In such cases, various grades of the same product varying in colour, size, shape, etc., can be modelled using MATLAB. Neural Networks (NN) available in MATLAB are another valuable feature for solar drying simulations. The number of nodes in such cases denotes the input parameters available in the study. Seginer and Bux performed experiments on solar drying of wet sludge using this approach and predicted the solid content drying rates (Citation2006). This approach shows that the evident use of MATLAB in neural network-related simulations could also be fruitful while simulations of various industrial drying systems. Fruits drying are widespread in solar drying, and mostly slices of fruits such as mango, banana, etc., are investigated for drying output efficiencies. MATLAB is also helpful in modelling thin-layer drying phenomenon in various solar dryers. Dissa et al. performed simulations of mango slices in solar drying in a three-day experimental investigation (Citation2009). The authors used MATLAB 7.0.1 for energy flux balancing in the system through nodes and neural approaches. Another example of thin-layer drying using MATLAB can be observed in crops such as onions drying. Jain investigated the drying of onions in a packed bed thermal storage dryer, a mixed form of the solar dryer, using MATLAB 6.1. (Jain Citation2007). Results suggest using MATLAB in such design forms where various trays are compiled one over the other, and temperature distributions on each tray can be numerically simulated using the energy balancing equations and analysing them through the software. Hybrid solar dryers are futuristic solar drying solutions in which photovoltaics (PV) is used as the combined heat generating source. The integration of PV and thermal solar sources is commonly known as PV/T hybrid dryers. Tiwari et al. used MATLAB 2013a to validate the experimental data with design parameters in the algorithm to establish a good correlation coefficient and validate the results (Tiwari, Tiwari, and Al-Helal Citation2016). Hence, over time MATLAB has evolved as an essential tool for numerical simulations of various parameters governing the drying condition in solar dryers. By coupling different visualisation to neural networks, the software goes to extended reach in the validation of extensive experimental studies. MATLAB will evolve parallelly in future numerical analysis related to various types of solar drying systems.
4.4. FORTRAN simulations
FORTRAN is a multi-purpose computer programming language initially developed in 1950. This language works on the implementation of compilers. The model of FORTRAN programs is imperative, making it useful in many scientific numerical and computational investigations. It also comes under high-performance computing and thus is used in many computational fluid dynamics and finite element analysis-related studies. Over time, FORTRAN has evolved many versions, adding better-performing extensions to the language. Some of the versions of FORTRAN are FORTRAN 77, FORTRAN 90, FORTRAN 95, FORTRAN 2003, FORTRAN 2008, and FORTRAN 2018. All versions contain various dimensions of programming and are helpful in multiple forms.
Similar to the analysis of many other systems, many researchers have investigated solar dryers using the FORTRAN program for different simulations based on the system’s environment. For example, in the early 90s, Mahapatra et al. studied the heat flow performance of a directly irradiated solar dryer with an integrated heat collector (Citation1994). The authors used FORTRAN 77 to create a program for solving the energy equations for the solar drying system of freshly harvested camomile. The system was discretised in several elements, and a heat flow network was created to understand the heat performance of the dryer. The program of FORTRAN helped predict various parameters under investigation, such as temperature, humidity, and moisture content in the different dryer segments. FORTRAN has also been used as a simulation tool in hybrid systems such as water-assisted heat pump dryers and water heaters combined. A comparative analysis was conducted by Hawlader et al., making solar fraction (SF) and coefficient of performance (COP) the benchmark variables. A simulation program was developed to analyse the variation in the various parameters governing the drying conditions of the product (Hawlader et al. Citation2003). The COP for the system was found to be 7.0 and 5.0 for simulation and experimental data, respectively. Bennamoun and Belhamri tried to develop an inexpensive batch-type solar dryer. The investigation was performed for drying onions using two different sets of differential equations leading to the formation of one empirical model (Bennamoun and Belhamri Citation2003). The two sets of equations were solved using the FORTRAN program. The Gauss-Siedel iterative method was performed by solving the equations and writing them in matrix form. The two case studies were compared, resulting in the (with heater) system performing better than the (without heater) method. A PV ventilated greenhouse dryer was examined by Janjai et al. for peeled longan and banana drying. The ventilation fans were powered by a photovoltaics module of 50 W capacity (Janjai et al. Citation2009). To investigate the heat transfer, nonlinear differential equations were generated with the finite difference method. And to solve these equations, the FORTRAN 6.5 version was used to create a program solving the equations of the system. Thus, promoting the FORTRAN software even for design optimisation case studies. Another greenhouse deep bed solar dryer was developed by Aghbashlo et al. to study the drying of chamomile flowers (Citation2015). The authors used Compaq visual FORTRAN programming to solve the thin layer drying and equilibrium model equations. The experimental data was observed at different load capacities of 15, 30, 45, and 60 kg/m2. Along with this, TRNSYS software was used for results validation. The simulation and experimental data agreement showcase the importance of FORTRAN as a programming language in solar drying systems.
4.5. Statistica/SPSS simulations
Researchers from different sectors frequently use some simulation software packages for statistical simulations. Statistical Package for the Social Sciences (‘SPSS’) and ‘statistica’ are two such software that few researchers used in the simulation of solar dryers, mostly of direct type. Midilli and Kucuk performed a thin layer drying analysis for pistachio samples in forced and natural convection solar dryers (Midilli and Kucuk Citation2003). In this analysis, the nonlinear regression analysis was performed using the ‘statistica’ computer program. ‘Statistica’ analysis helped verify the logarithmic and two-term model suitability for forced and natural convection dryers, respectively. SPSS has been used in a variety of sun-drying studies. Hossain and Bala investigated colour values and pungency indices using SPSS 9.0 through analysis of variance (Hossain and Bala Citation2007). Both the factors were graded under Duncan’s multiple range test (DMRT). Chavan et al. performed mathematical modelling to dry Indian Mackerel in a solar-biomass hybrid-type dryer (Chavan, Yakupitiyage, and Kumar Citation2008). A comparative study was done between open sun drying and hybrid dryer under eight experimental trials. The coefficient of determination , root mean square error
, and reduced chi-square
values were observed for the perfect fitting curve with a significance level of (P = 0.5). The SPSS software package determined the nonlinear regression analysis and Midilli model. The two-term model was most suitable for the hybrid drying and open sun drying of Mackerel, respectively. Similarly, Mithun et al. used DMRT with (P < 0.5) in statistical biochemical quality analysis of traditional and solar dried fish (Citation2021). For this study, samples of Bombay duck (Harpodon nehereus) fish were observed in a comparative study revealing better nutritional values than the traditional drying method. Fu et al. investigated the correlation between protein oxidation and quality and studied the changes in various indexes of solar-dried shrimp (Penaeus vannamei) (Fu et al. Citation2021). The authors used SPSS 23 for statistical findings in differential significance with the DUNCAN method and ORIGIN 2018 to generate the charts.
4.6. TRNSYS simulations
TRNSYS software is used globally for numerical simulation, mostly in investigations related to the sun drying behaviour of crops. The software helps ease the calculations associated with complex differential equations in a shorter time. The TRNSYS simulations work closely related to the heat transfer, moisture removal, and energy balancing equations and provide accurate results in fewer iterations. These qualities make it favourable software for simulations in solar drying of various agricultural products (Singh Chauhan, Kumar, and Tekasakul Citation2015). Reuss et al. introduced the TRNSYS module for wood drying, as shown in . The authors performed simulations for drying staple wood with input parameters such as temperature and absolute humidity (Reuss et al. Citation1997). The system was fragmented with a finite element analysis approach into 60 segments depicting various layers of the wood sample with a time step of 15 s. The whole process is briefly described in the flow chart and could be used to investigate the drying behaviour of various wood types. However, the TRNSYS simulation software consists of an extensive toolset for the proper different transient systems. However, sometimes the tools are not available for product-specific drying. For example, Lamrani et al. coupled the TRNSYS software with a FORTRAN program to analyse specific parameters in wood drying (Lamrani, Khouya, and Draoui Citation2019). The authors used the output of the TRNSYS program as an input to the coupled software and gave insightful results. Furthermore, Xie et al. examined the performance of a solar-assisted heat pump drying system with waste heat recovery. The authors performed TRNSYS-based simulations for variable volume experiments (Xie et al. Citation2021). In addition, TRNSYS has been used in industrial-level hybrid indirect-type solar drying systems. In an annual investigation, Sanchez et al. developed yearly simulations for comparative theoretical-experimental study for solar air heating systems in Morelos, Mexico (Álvarez-sánchez, Flores-prieto, and García-valladares Citation2021). Such examples establish the TRNSYS software as a promising tool in a wide range of simulations related to solar drying systems.
Figure. 10. TRNSYS module Flowchart for wood drying reproduced from Reuss et al. (Citation1997).
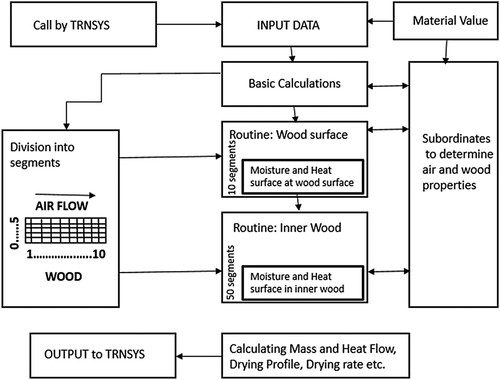
5. Environmental impact assessment
The cost and the environmental impact are some significant criterias that decide the feasibility of a developed product for commercial scaling and its suitability from a sustainable point of view. The environmental impact is mostly a neglected part of optimising solar dryers. However, in recent years, it has gained popularity among researchers (Singh and Gaur Citation2021). The daily thermal output when multiplied by the number of sunshine days gives the annual energy output. Taking base of the annual energy output the carbon calculations can be performed with the help of formulae mentioned in . The dryer’s CO2 emissions are a function of its embodied energy; therefore they can be cut by switching to less-heavy raw materials like aluminium instead of iron frames (Singh and Gaur Citation2021). The earned carbon credit has been reported to have significant financial benefits of approximately 800 USD to 2400 USD compared to sun drying of fenugreek leaves in a study conducted in India with energy payback time of around 4 years (Shrivastava and Kumar Citation2017). The environmental impact factor and improvement potential could help in further improving the overall design and raw materials involved in solar drying.
Table 3. Sustainability related criteria and its evaluation for solar dryers.
6. Economic assessment-based optimisation
The agro-based industries are constantly growing worldwide, and so is the demand for organically dried products. The quality assessment of the solar-dried products thus becomes crucial in the market development of the dried products. Even the biofuels such as wood chips which are used in largescale quantities for energy generation suffer degraded quality due to high moisture content. Labscale investigations reveal better drying efficiency with natural convective dryers for wood chips (Baibhaw, Szepesi, and Szamosi Citation2021; Kumar, Szepesi, and Szamosi Citation2021). In addition, fruits and vegetables are highly perishable items, which could lead to post-harvest losses if not handled properly (Getahun et al. Citation2021). Thus, optimisation of the solar drying conditions also depends on factors apart from scientific parameters. Time calculations are the prime focus areas for developing economically feasible dryers in the economic optimisation life cycle analysis and payback period. Singh et al. performed financial analysis for various agricultural products in an active indirect type solar dryer (Singh et al. Citation2021). The results proved that the payback period is a direct economic parameter for economic optimisation. In regions where the environment’s humidity level is higher, such places are suitable for growing crops such as millets, rice, mint, etc. Greenhouse solar dryers are ideal for crop drying. gives insights into some functional calculations which can be performed for the economic feasibility of greenhouse solar dryers (Chauhan, Kumar, and Nuntadusit Citation2018; Mishra, Sinha, and Gupta Citation2021).
Table 4. Parameters used for economic optimisation in solar dryers.
Investments play a crucial role from the start of any project development. Naturally, in solar drying systems as well the assets and their return value are essential too. The internal rate of return (IRR) must be evaluated from socioeconomic and financial aspects. IRR can be calculated through the balancing of inflow and outflow accumulations. Microsoft Excel tool was found helpful in IRR calculations. The discussed parameters directly or indirectly affect the overall economic feasibility of the solar drying systems. The mentioned calculations can help develop economically sound drying systems for longer life (Chavan and Thorat Citation2022). provides visualisation for a few recent economic analyses on various types of products and dryers. The assessment covered multiple types of dryers along with different agricultural products. The capital cost depends on the type of dryer and the volume of the dried product. The household-type solar dryer can be constructed for products such as Banana slices(Nabnean and Nimnuan Citation2020), Onion slices (Hadibi et al. Citation2022), or Bitter gourds(Zachariah and Maatallah Citation2021) in the range of 150–400 USD. For active (Singh et al. Citation2021) and indirect dryers(Reddy Mugi and Chandramohan Citation2021) with forced convection arrangements, the capital cost increases to 400–700 USD. The study observed that the greenhouse dryers(ELkhadraoui et al. Citation2015; Shrivastava, Gaur, and Singh Citation2022) and the dryers with thermal energy are the most expensive to build (Gilago and Reddy Mugi Citation2022). The average capital cost could lie between 100–2000 USD. All the dryers investigated have a minimum lifespan of 10 years and a maximum of 25 years(Chavan and Thorat Citation2022). Economic analysis is thus critical for the long-life sustainability of drying process optimisation.
7. Conclusion
In this compendium analysis work, the authors compiled the various facets of optimisation techniques used by researchers and academicians for the performance analysis of solar dryers. Numerical modelling is essential in understanding the relevant scientific parameters affecting dryer performance. Various modelling techniques, their classification, and the stepwise analysis procedure are discussed briefly for some modelling techniques such as Taguchi, ANN, GA, Particle Swarm, and ANOVA. Validation of the numerical models or the theoretical study with experimental results is inevitable in any optimisation study. Few persistently used software packages and their use in the visual validation of dryers are presented as comparative analogisation. Researchers often visualise the airflow patterns and heat dissipation inside the dryers, persistently using software such as MATLAB, FORTRAN, STATISTICA, SPSS, etc. The economic analysis coupled with sustainability criteria could be beneficial in future research related to optising solar dryers. The business model canvas and value proposition analysis could also be further explored for entrepreneurship opportunities in the solar drying of agricultural products (Kumar et al. Citation2022). Few conclusions drawn out of this review work are mentioned below:
The modelling of drying systems can provide a short-term conjecture to the insights into performance. Simulations proffer validation with visualisations with a long repetitive range of inferences through software programs.
The modelling and simulation combinations are often clubbed for validation of results, and the distinctness and righteous use are critical in overall performance optimisation.GA-ANN, and MATLAB/CFD-ANN are found as evolving combinations for modelling and validation.
CFD and MATLAB simulations have evolved as the most sought visualisation tools and fit with most mathematical modelling approaches.
Sustainability assessments of solar drying systems could add value to optimisation with calculations of sustainability index, environmental impact factor and the improvement potential of the solar drying systems. Also, future research should consider exergo-economic and social impact of using solar drying equipment.
The comparative economic analysis observed that the solar dryers with thermal storage facilities cost mostly over 1000 USD. At the same time, a typical household or greenhouse dryer’s capital cost could be 100–700 USD with some added indirect or forced convection modes.
Further work is required to find the best possible combinations of modelling and simulation which could be usefull for researcher’s future optimisation investigations.
Author contributions
BK and ZS: Conceptualisation; BK: Data curation; BK and GLS: Formal analysis; BK and ZS: Investigation; BK and GLS: Methodology; GLS and ZS: Supervision; BK and GLS: Validation; BK: Visualisation; BK: original draft; BK, ZS and GLS review & editing.
Disclosure statement
No potential conflict of interest was reported by the author(s).
References
- Abubakar, S., F. O. Anafi, M. U. Kaisan, S. Narayan, S. Umar, and U. A. Umar. 2020. “Comparative Analyses of Experimental and Simulated Performance of a Mixed-Mode Solar Dryer.” Proceedings of the Institution of Mechanical Engineers, Part C: Journal of Mechanical Engineering Science 234 (7): 1393–1402. doi:10.1177/0954406219893394.
- Aghbashlo, M., J. Müller, H. Mobli, A. Madadlou, and S. Rafiee. 2015. “Modeling and Simulation of Deep-Bed Solar Greenhouse Drying of Chamomile Flowers.” Drying Technology 33 (6): 684–695. doi:10.1080/07373937.2014.981278.
- Akowuah, J. O., A. Bart-plange, and K. A. Dzisi. 2021. “Thin Layer Mathematical Modelling of White Maize in a Mobile Solar-Biomass Hybrid Dryer.” Research in Agricultural Engineering 2021 (no. 2): 74–83. doi:10.17221/56/2020-RAE..
- Al-Amri, A. M., M. A. Ismail, Y. A. Al Hassan, and E. A. Almuhanna. 2021. “Effect of Solar Drying on I: Some Physico-Chemical Properties of Fruits of two Date Palm (Phoenix Dactylifera L.) Varieties.” Solar Energy 218: 425–434. doi:10.1016/j.solener.2021.02.023.
- Álvarez-sánchez, F., J. Flores-prieto, and O. García-valladares. 2021. “Annual Thermal Performance of an Industrial Hybrid Direct– Indirect Solar air Heating System for Drying Applications in Morelos-México.” Energies 14 (17), doi:10.3390/en14175417.
- Amouiri, R., and A. Belhamri. 2022. “CFD Investigations on the Behavior of a Solar Dryer Used for Medicinal Herbs Dehydration Under Climatic Conditions of Constantine, Algeria.” Materials Today: Proceedings 51: 2123–2130. doi:10.1016/j.matpr.2021.12.475.
- Arunsandeep, G., A. Lingayat, V. P. Chandramohan, V. R. K. Raju, and K. S. Reddy. 2018. “A Numerical Model for Drying of Spherical Object in an Indirect Type Solar Dryer and Estimating the Drying Time at Different Moisture Level and air Temperature.” International Journal of Green Energy 15 (3): 189–200. doi:10.1080/15435075.2018.1433181.
- Baibhaw, K., G. L. Szepesi, and Z. Szamosi. 2021. “Design and Development of Natural Convective Solar Dryer.” Multidiszciplináris Tudományok 11 (4): 144–150. doi:10.35925/j.multi.2021.4.18.
- Barnwal, P., and G. N. Tiwari. 2008. “Grape Drying by Using Hybrid Photovoltaic-Thermal (PV/T) Greenhouse Dryer: An Experimental Study.” Solar Energy 82 (12): 1131–1144. doi:10.1016/j.solener.2008.05.012.
- Bartzanas, T., T. Boulard, and C. Kittas. 2004. “Effect of Vent Arrangement on Windward Ventilation of a Tunnel Greenhouse.” Biosystems Engineering 88 (4): 479–490. doi:10.1016/j.biosystemseng.2003.10.006.
- Benhamza, A., A. Boubekri, A. Atia, T. Hadibi, and M. Arıcı. 2021. “Drying Uniformity Analysis of an Indirect Solar Dryer Based on Computational Fluid Dynamics and Image Processing.” Sustainable Energy Technologies and Assessments 47 (June): 101466. doi:10.1016/j.seta.2021.101466.
- Bennamoun, L., and A. Belhamri. 2003. “Design and Simulation of a Solar Dryer for Agriculture Products.” Journal of Food Engineering 59 (no. 2–3): 259–266. doi:10.1016/S0260-8774(02)00466-1.
- Boran, S. 2006. “The use of Taguchi Method for the Optimization of Bakers Yeast Drying.pdf.” Proceedings of 5th International Symposium on Intelligent Manufacturing Systems (no. December 2015).
- Capossio, J. P., Fabani MP, Reyes-Urrutia A, Torres-Sciancalepore R, Deng Y, Baeyens J, Rodriguez R, et al. 2022. “Sustainable Solar Drying of Brewer’s Spent Grains: A Comparison with Conventional Electric Convective Drying.” Processes 10 (no. 2), doi:10.3390/pr10020339.
- Chauhan, P. S., A. Kumar, and C. Nuntadusit. 2018. “Thermo-Environomical and Drying Kinetics of Bitter Gourd Flakes Drying Under North Wall Insulated Greenhouse Dryer.” Solar Energy 162 (no. 2018): 205–216. doi:10.1016/j.solener.2018.01.023.
- Chavan, A., and B. Thorat. 2022. “Techno-economic Comparison of Selected Solar Dryers: A Case Study.” Drying Technology 40: 2105–2115. doi:10.1080/07373937.2021.1919141.
- Chavan, A., V. Vitankar, A. Mujumdar, and B. Thorat. 2021a. “Natural Convection and Direct Type (NCDT) Solar Dryers: A Review.” Drying Technology 39 (0): 1969–1990. doi:10.1080/07373937.2020.1753065.
- Chavan, A., V. Vitankar, N. Shinde, and B. Thorat. 2021b. “CFD Simulation of Solar Grain Dryer.” Drying Technology 39 (8): 1101–1113. doi:10.1080/07373937.2020.1863422.
- Chavan, B. R., A. Yakupitiyage, and S. Kumar. 2008. “Mathematical Modeling of Drying Characteristics of Indian Mackerel (Rastrilliger Kangurta) in Solar-Biomass Hybrid Cabinet Dryer.” Drying Technology 26 (12): 1552–1562. doi:10.1080/07373930802466872.
- Dhalsamant, K. 2021. “Development, Validation, and Comparison of FE Modeling and ANN Model for Mixed-Mode Solar Drying of Potato Cylinders.” J Food Sci. 86: 3384–3402. doi:10.1111/1750-3841.15847.
- Dissa, A. O., J. Bathiebo, S. Kam, P. W. Savadogo, H. Desmorieux, and J. Koulidiati. 2009. “Modelling and Experimental Validation of Thin Layer Indirect Solar Drying of Mango Slices.” Renewable Energy 34 (4): 1000–1008. doi:10.1016/j.renene.2008.08.006.
- Dong, Z., D. Yang, T. Reindl, and W. M. Walsh. 2015. “A Novel Hybrid Approach Based on Self-Organizing Maps, Support Vector Regression and Particle Swarm Optimization to Forecast Solar Irradiance.” Energy 82: 570–577. doi:10.1016/j.energy.2015.01.066.
- Eberhart, R., and J. Kennedy. 1995. “A new Optimizer Using Particle Swarm Theory.” Proceeding of International Symposium on Micro Machine and Human Science, 39–43. doi:10.1109/MHS.1995.494215.
- ELkhadraoui, A., S. Kooli, I. Hamdi, and A. Farhat. 2015. “Experimental Investigation and Economic Evaluation of a new Mixed-Mode Solar Greenhouse Dryer for Drying of red Pepper and Grape.” Renewable Energy 77: 1–8. doi:10.1016/j.renene.2014.11.090.
- Erenturk, S., and K. Erenturk. 2007. “Comparison of Genetic Algorithm and Neural Network Approaches for the Drying Process of Carrot.” Journal of Food Engineering 78 (3): 905–912. doi:10.1016/j.jfoodeng.2005.11.031.
- Fabani, M. P., J. P. Capossio, M. C. Román, W. Zhu, R. Rodriguez, and G. Mazza. 2021. “Producing non-Traditional Flour from Watermelon Rind Pomace: Artificial Neural Network (ANN) Modeling of the Drying Process.” Journal of Environmental Management 281: 111915. doi:10.1016/j.jenvman.2020.111915.
- Fu, Y., Y. Liu, W. Wang, R. Suo, and J. Wang. 2021. “Models for Predicting Quality of Solar-Dried Shrimp (Penaeus Vannamei) During Storage Based on Protein Oxidation.” Journal of Food Quality 2021. doi:10.1155/2021/1493927.
- Getahun, E., M. A. Delele, N. Gabbiye, S. W. Fanta, P. Demissie, and M. Vanierschot. 2021. “Importance of Integrated CFD and Product Quality Modeling of Solar Dryers for Fruits and Vegetables: A Review.” Solar Energy 220 (March): 88–110. doi:10.1016/j.solener.2021.03.049.
- Gilago, M. C., and V. Reddy Mugi. 2022. “Energy-exergy and Environ-Economic (4E) Analysis While Drying ivy Gourd in a Passive Indirect Solar Dryer Without and with Energy Storage System and Results Comparison.” Solar Energy 240: 69–83. doi:10.1016/j.solener.2022.05.027.
- Hadibi, T., Abdelghani B, Djamel M, Abderrahmane B, Khaled M, Anil K, Hong-Wei X, Karim A. 2022. “Energy, Environmental, Economic, and Color Analysis of geo-Exchange Energy Assisted-Insulated North Wall Solar Dryer for Onion Slices Under Relatively Cloudy and Rainy Conditions.” Solar Energy 236: 1–16. doi:10.1016/j.solener.2022.02.037.
- Hamdi, I., S. Kooli, A. Elkhadraoui, Z. Azaizia, F. Abdelhamid, and A. Guizani. 2018. “Experimental Study and Numerical Modeling for Drying Grapes Under Solar Greenhouse.” Renewable Energy 127: 936–946. doi:10.1016/j.renene.2018.05.027.
- Hawlader, M. N. A., S. K. Chou, K. A. Jahangeer, S. M. A. Rahman, and K. W. Eugene Lau. 2003. “Solar-assisted Heat-Pump Dryer and Water Heater.” Applied Energy 74 (no. 1–2): 185–193. doi:10.1016/S0306-2619(02)00145-9.
- Hossain, M. A., and B. K. Bala. 2007. “Drying of hot Chilli Using Solar Tunnel Drier.” Solar Energy 81 (1): 85–92. doi:10.1016/j.solener.2006.06.008.
- Hussein, J. B., M. O. Oke, O. O. Abiona, and Q. Adebayo. 2021. “Optimization of Processing Parameters for Drying of Tomatoes (Solanum Lycopersicum L.var) Slices Using Taguchi Technique.” Journal of Food Processing and Preservation 45 (2): 0–2. doi:10.1111/jfpp.15149.
- Iranmanesh, M., H. Samimi Akhijahani, and M. S. Barghi Jahromi. 2020. “CFD Modeling and Evaluation the Performance of a Solar Cabinet Dryer Equipped with Evacuated Tube Solar Collector and Thermal Storage System.” Renewable Energy 145: 1192–1213. doi:10.1016/j.renene.2019.06.038.
- Ismail, A. F., A. S. Abd Hamid, A. Ibrahim, H. Jarimi, and K. Sopian. 2022. “Performance Analysis of a Double Pass Solar Air Thermal Collector with Porous Media Using Lava Rock.” Energies 15 (3), doi:10.3390/en15030905.
- Jain, D. 2007. “Modeling the Performance of the Reversed Absorber with Packed bed Thermal Storage Natural Convection Solar Crop Dryer.” Journal of Food Engineering 78 (2): 637–647. doi:10.1016/j.jfoodeng.2005.10.035.
- Janjai, S., et al. 2009. “Experimental and Simulated Performance of a PV-Ventilated Solar Greenhouse Dryer for Drying of Peeled Longan and Banana.” Solar Energy 83 (9): 1550–1565. doi:10.1016/j.solener.2009.05.003.
- Jha, A., and P. P. Tripathy. 2021. “Optimization of Process Parameters and Numerical Modeling of Heat and Mass Transfer During Simulated Solar Drying of Paddy.” Computers and Electronics in Agriculture 187: 106215. doi:10.1016/j.compag.2021.106215.
- Joshi, M., N. Kumar, and P. Baredar. 2019a. “Optimization of Solar Dryer Using Taguchi Method.” International Journal of Recent Technology and Engineering (IJRTE) 8 (3): 3320–3326. doi:10.35940/ijrte.C5684.098319.
- Joshi, M., N. Kumar, and P. Baredar. 2019b. “The Optimization Techniques Used in Solar Dryers, A Review.” International Journal of Mechanical and Production Engineering Research and Development 9 (4): 83–92. doi:10.24247/ijmperdaug20199.
- Kolioak, Y., M. Radhakrishna, and A. M. K. Prasad. 2020. “Optimization of Heat Energy Based on Phase Change Materials Used in Solar Collector Using Taguchi Method.” Materials Today: Proceedings 22: 2404–2411. doi:10.1016/j.matpr.2020.03.365.
- Kumar, B., L. Berényi, Z. Szamosi, and G. L. Szepesi. 2022. “Business Model Analysis for the Scope of Entrepreneurship in a Solar Drying Field in the European Region.” Entrepreneurship in the Raw Materials Sector, 85–92. doi:10.1201/9781003259954-9.
- Kumar, B., L. G. Szepesi, and Z. Szamosi. 2021. “Drying Behaviour Observations for Wood Chips of Grade EN14961.” Multidiszciplináris Tudományok 11 (4): 151–156. doi:10.35925/j.multi.2021.4.19.
- Lamrani, B., and A. Draoui. 2020. “Modelling and Simulation of a Hybrid Solar-Electrical Dryer of Wood Integrated with Latent Heat Thermal Energy Storage System.” Thermal Science and Engineering Progress 18: 100545. doi:10.1016/j.tsep.2020.100545.
- Lamrani, B., A. Khouya, and A. Draoui. 2019. “Energy and Environmental Analysis of an Indirect Hybrid Solar Dryer of Wood Using TRNSYS Software.” Solar Energy 183 (March): 132–145. doi:10.1016/j.solener.2019.03.014.
- Larson, M. G. 2008. “Analysis of Variance.” Circulation 117 (1): 115–121. doi:10.1161/CIRCULATIONAHA.107.654335.
- Madamba, P. S. 1997. “Optimization of the Drying Process: An Application to the Drying of Garlic.” Drying Technology 15 (1): 117–136. doi:10.1080/07373939708917221.
- Mahapatra, A. K., L. Imre, J. Barcza, A. Bitaif, and I. Farkast. 1994. “Simulation of a Directly Irradiated Solar Dryer with Integrated Collector.” International Journal of Ambient Energy 15 (4): 195–204. doi:10.1080/01430750.1994.9675654.
- Majdi, H., and J. A. Esfahani. 2019. “Energy and Drying Time Optimization of Convective Drying: Taguchi and LBM Methods.” Drying Technology 37 (6): 722–734. doi:10.1080/07373937.2018.1458036.
- Mellalou, A., W. Riad, S. K. Hnawi, A. Tchenka, A. Bacaoui, and A. Outzourhit. 2021. “Experimental and CFD Investigation of a Modified Uneven-Span Greenhouse Solar Dryer in No-Load Conditions Under Natural Convection Mode.” International Journal of Photoenergy 2021. doi:10.1155/2021/9918166.
- Midilli, A., and H. Kucuk. 2003. “Mathematical Modeling of Thin Layer Drying of Pistachio by Using Solar Energy.” Energy Conversion and Management 44 (7): 1111–1122. doi:10.1016/S0196-8904(02)00099-7.
- Mishra, L., A. Sinha, and R. Gupta. 2021. “Energy, Exergy, Economic and Environmental (4E) Analysis of Greenhouse Dryer in no-Load Condition.” Sustainable Energy Technologies and Assessments 45 (March): 101186. doi:10.1016/j.seta.2021.101186.
- Mithun, B. D., M. S. Hoque, M. L. Van Brakel, M. M. Hasan, S. Akter, and M. R. Islam. 2021. “Comparative Quality Assessment of Traditional vs. Improved Dried Bombay Duck (Harpodon Nehereus) Under Different Storage Conditions: Solar Chimney Dryer a low-Cost Improved Approach for Nutritional Dried Fish.” Food Science & Nutrition 9: 6794–6805. doi:10.1002/fsn3.2631.
- Mustapha, A. N., Y. Zhang, Z. Zhang, Y. Ding, Q. Yuan, and Y. Li. 2021. “Taguchi and ANOVA Analysis for the Optimization of the Microencapsulation of a Volatile Phase Change Material.” Journal of Materials Research and Technology 11: 667–680. doi:10.1016/j.jmrt.2021.01.025.
- Mutabilwa, P., and K. N. Nwaigwe. 2020. “Design, Construction and CFD Modeling of a Banana-Solar Dryer With Double Pass Solar Air Collector.” doi:10.1115/ES2020-1614.
- Muthee, A. 2021. “The Basics of Genetic Algorithms in Machine Learning,” 2021. [Online]. Accessed 21 September 2021. https://www.section.io/engineering-education/the-basics-of-genetic-algorithms-in-ml/.
- Nabnean, S., and P. Nimnuan. 2020. “Experimental Performance of Direct Forced Convection Household Solar Dryer for Drying Banana.” Case Studies in Thermal Engineering 22: 100787. doi:10.1016/j.csite.2020.100787.
- Ndukwu, M. C., Mathew I, Pomise E, Christopher U A, Inemesit E E, Akuwueke L, Linus O, et al. 2022. “Assessment of eco-Thermal Sustainability Potential of a Cluster of low-Cost Solar Dryer Designs Based on Exergetic Sustainability Indicators and Earned Carbon Credit.” Cleaner Energy Systems 3 (October): 100027. doi:10.1016/j.cles.2022.100027.
- Pirasteh, G., R. Saidur, S. M. A. Rahman, and N. A. Rahim. 2014. “A Review on Development of Solar Drying Applications.” Renewable and Sustainable Energy Reviews 31: 133–148. doi:10.1016/j.rser.2013.11.052.
- Prakash, O., and A. Kumar. 2013. “Historical Review and Recent Trends in Solar Drying Systems.” International Journal of Green Energy 10 (7): 690–738. doi:10.1080/15435075.2012.727113.
- Prakash, O., and A. Kumar. 2014a. “ANFIS Modelling of a Natural Convection Greenhouse Drying System for Jaggery: An Experimental Validation.” International Journal of Sustainable Energy 33 (2): 316–335. doi:10.1080/14786451.2012.724070.
- Prakash, O., and A. Kumar. 2014. “Environomical Analysis and Mathematical Modelling for Tomato Flakes Drying in a Modified Greenhouse Dryer Under Active Mode.” International Journal of Food Engineering 10 (4): 669–681. doi:10.1515/ijfe-2013-0063.
- Prakash, O., and A. Kumar. 2020. Solar Drying Systems. 1st. Oxfordshire: CRC Press.
- Rahman, M. M., A. G. M. M. Billah, S. Mekhilef, and S. Rahman. 2014. “Application of Genetic Algorithm for Optimization of Solar Powered Drying.” IEEE Innov. Smart Grid Technol. – Asia, ISGT ASIA 2014, pp. 647–651. doi:10.1109/ISGT-Asia.2014.6873868.
- Rahman, M. M., A. G. M. B. Mustayen, S. Mekhilef, and R. Saidur. 2015. “The Optimization of Solar Drying of Grain by Using a Genetic Algorithm.” International Journal of Green Energy 12 (12): 1222–1231. doi:10.1080/15435075.2014.890106.
- Reddy Mugi, V., and V. P. Chandramohan. 2021. “Energy, Exergy and Economic Analysis of an Indirect Type Solar Dryer Using Green Chilli: A Comparative Assessment of Forced and Natural Convection.” Thermal Science and Engineering Progress 24: 100950. doi:10.1016/j.tsep.2021.100950.
- Reuss, M., et al. 1997. “Modelling and Experimental Investigation of a Pilot Plant for Solar Wood Drying.” Solar Energy 59 (no. 4-6): 259–270. doi:10.1016/S0038-092X(97)00013-3.
- Salvatierra-Rojas, A., I. Ramaj, S. Romuli, and J. Müller. 2021. “CFD-simulink Modeling of the Inflatable Solar Dryer for Drying Paddy Rice.” Applied Sciences 11 (7), doi:10.3390/app11073118.
- Sanchez, P. J. 2007. “Fundamentals of Simulation Modeling.” Proceedings 2007 Winter Simulation Conference, pp. 54–62, doi:10.1109/WSC.2007.4419588.
- Santana, J. C. C., S. A. Araújo, A. F. H. Librantz, and E. B. Tambourgi. 2010. “Optimization of Corn Malt Drying by use of a Genetic Algorithm.” Drying Technology 28 (11): 1236–1244. doi:10.1080/07373937.2010.500439.
- Seginer, I., and M. Bux. 2006. “Modeling Solar Drying Rate of Wastewater Sludge.” Drying Technology 24 (11): 1353–1363. doi:10.1080/07373930600952362.
- Sekyere, C. K. K., F. W. Adams, F. Davis, and F. K. Forson. 2020. “Mathematical Modelling and Validation of the Thermal Buoyancy Characteristics of a Mixed Mode Natural Convection Solar Crop Dryer with Back up Heater.” Scientific African 8: e00441. doi:10.1016/j.sciaf.2020.e00441.
- Sharma, A., C. R. Chen, and N. Vu Lan. 2009. “Solar-energy Drying Systems: A Review.” Renewable and Sustainable Energy Reviews 13 (no. 6–7): 1185–1210. doi:10.1016/j.rser.2008.08.015.
- Shrivastava, A., M. K. Gaur, and P. Singh. 2022, January. “Mango Leather (Aam Papad) Drying in Hybrid Greenhouse Solar Dryer with Evacuated Tube Collector and Finned Drying Tray: Drying Behavior and Economic Analysis.” Energy Sources, Part A: Recovery, Utilization, and Environmental Effects, 1–18. doi:10.1080/15567036.2022.2029974.
- Shrivastava, V., and A. Kumar. Apr. 2017. “Embodied Energy Analysis of the Indirect Solar Drying Unit.” International Journal of Ambient Energy 38 (3): 280–285. doi:10.1080/01430750.2015.1092471.
- Siddhartha, N. Sharma, and Varun. 2012. “A Particle Swarm Optimization Algorithm for Optimization of Thermal Performance of a Smooth Flat Plate Solar air Heater.” Energy 38 (1): 406–413. doi:10.1016/j.energy.2011.11.026.
- Singh, P., and M. K. Gaur. 2021. “Sustainability Assessment of Hybrid Active Greenhouse Solar Dryer Integrated with Evacuated Solar Collector.” Current Research in Food Science 4: 684–691. doi:10.1016/j.crfs.2021.09.011.
- Singh, S., R. S. Gill, V. S. Hans, and M. Singh. 2021. “A Novel Active-Mode Indirect Solar Dryer for Agricultural Products: Experimental Evaluation and Economic Feasibility.” Energy 222: 119956. doi:10.1016/j.energy.2021.119956.
- Singh Chauhan, P., A. Kumar, and P. Tekasakul. 2015. “Applications of Software in Solar Drying Systems: A Review.” Renewable and Sustainable Energy Reviews 51: 1326–1337. doi:10.1016/j.rser.2015.07.025.
- Tegenaw, P. D., M. G. Gebrehiwot, and M. Vanierschot. 2019. “On the Comparison Between Computational Fluid Dynamics (CFD) and Lumped Capacitance Modeling for the Simulation of Transient Heat Transfer in Solar Dryers.” Solar Energy 184 (no. 2019): 417–425. doi:10.1016/j.solener.2019.04.024.
- Templalexis, Giorni P, Lentzou D, Sabrina M , Georgios X , Mesisca S, Tsitsigiannis D I, Battilani P, et al. 2021. “Environmental Conditions Affecting Ochratoxin A During Solar Drying of Grapes: The Case of Tunnel and Open Air-Drying.” Toxins 13 (6), doi:10.3390/toxins13060400.
- Tiwari, S., G. N. Tiwari, and I. M. Al-Helal. 2016. “Performance Analysis of Photovoltaic-Thermal (PVT) Mixed Mode Greenhouse Solar Dryer.” Solar Energy 133: 421–428. doi:10.1016/j.solener.2016.04.033.
- Tzempelikos, D. A., D. Mitrakos, A. P. Vouros, A. V. Bardakas, A. E. Filios, and D. P. Margaris. 2015. “Numerical Modeling of Heat and Mass Transfer During Convective Drying of Cylindrical Quince Slices.” Journal of Food Engineering 156: 10–21. doi:10.1016/j.jfoodeng.2015.01.017.
- Wang, D., and D. Fon. 2005. “Applications of MATLAB-Based Software to Drying Simulation.” Mechatronics, 1–17. http://140.112.183.23/.
- Wang, D., D. Tan, and L. Liu. 2018. “Particle Swarm Optimization Algorithm: An Overview.” Soft Computing 22 (2): 387–408. doi:10.1007/s00500-016-2474-6.
- Xie, Z., Y. Gong, C. Ye, Y. Yao, and Y. Liu. 2021. “Numerical Analysis and Optimization of Solar-Assited Heat Pump Drying System with Waste Heat Recovery Based on Trnsys.” Processes 9 (7), doi:10.3390/pr9071118.
- Zachariah, A. M., and Richu Taher Maatallah. 2021. “Environmental and Economic Analysis of a Photovoltaic Assisted Mixed Mode Solar Dryer with Thermal Energy Storage and Exhaust air Recirculation.” International Journal of Energy Research 45: 1879–1891. doi:10.1002/er.5868.