Abstract
Objective: The aims of the current n200 study were to assess the structural relations between three classes of test variables (i.e. HEARING, COGNITION and aided speech-in-noise OUTCOMES) and to describe the theoretical implications of these relations for the Ease of Language Understanding (ELU) model. Study sample: Participants were 200 hard-of-hearing hearing-aid users, with a mean age of 60.8 years. Forty-three percent were females and the mean hearing threshold in the better ear was 37.4 dB HL. Design: LEVEL1 factor analyses extracted one factor per test and/or cognitive function based on a priori conceptualizations. The more abstract LEVEL 2 factor analyses were performed separately for the three classes of test variables. Results: The HEARING test variables resulted in two LEVEL 2 factors, which we labelled SENSITIVITY and TEMPORAL FINE STRUCTURE; the COGNITIVE variables in one COGNITION factor only, and OUTCOMES in two factors, NO CONTEXT and CONTEXT. COGNITION predicted the NO CONTEXT factor to a stronger extent than the CONTEXT outcome factor. TEMPORAL FINE STRUCTURE and SENSITIVITY were associated with COGNITION and all three contributed significantly and independently to especially the NO CONTEXT outcome scores (R2 = 0.40). Conclusions: All LEVEL 2 factors are important theoretically as well as for clinical assessment.
Introduction
Hearing loss (HL) affects approximately 15% of the general population, including every second person aged 65 and over (Lin et al, Citation2011). Recent advances in hearing aid technology benefit some persons with HL, but far from all can take advantage of the advanced signal processing available in modern hearing aids (Kiessling et al, Citation2003; Lunner & Sundewall-Thorén, Citation2007; Souza et al, Citation2015a,Citationb for a review). These differences in benefit occur even when different individuals have similar audiograms. In addition, factors like auditory temporal processing ability and specific cognitive functions explain some of these differences (Pichora-Fuller et al, Citation2007; Rönnberg et al, Citation2011a,Citationb; Humes et al, Citation2013; Rönnberg et al, Citation2013, Citation2014; Schoof & Rosen, Citation2014; Füllgrabe et al, Citation2015).
Over the last decade, the research community has increasingly acknowledged that successful (re)habilitation of persons with HL must be individualized and based on a finer analysis of details of the impairment at all levels of the auditory system from cochlea to cortex in combination with individual differences in cognitive capacities pertinent to language understanding (e.g., Souza et al, Citation2015; Wendt et al, Citation2015). Relevant cognitive capacities include working memory capacity (WMC; Lunner, Citation2003; Gatehouse et al, Citation2006; Foo et al, Citation2007; Arlinger et al, Citation2009; Stenfelt & Rönnberg, Citation2009; Arehart et al, Citation2013; Füllgrabe et al, Citation2015; Keidser et al, Citation2015; Souza et al, Citation2015; Wendt et al, Citation2015) and phonological skills (Lyxell et al, Citation1998; Lazard et al, Citation2010; Classon et al, Citation2013; Rudner & Lunner, Citation2014). Furthermore, these cognitive capacities underpin communicative and social competence, and this cognitive-social relationship is presumably reinforced among older people whose cognitive capacities are known to be variable and vulnerable (Danielsson et al, Citation2015; Pichora-Fuller et al, Citation2013; Rönnberg et al, Citation2013; Rönnlund et al, Citation2015; Schneider et al, Citation2010). There is also convincing evidence that sensory loss, including HL, is associated with age-related differences in cognition (Humes et al, Citation2013) and with incident dementia (Albers et al, Citation2015). Less well understood are the complex interactions among type of HL, aging and specific aspects of cognition, including phonological awareness, executive function and WMC (Harrison Bush et al, Citation2015). In addition to the associations between pure-tone threshold elevation and cognitive decline, there is evidence that decline in central auditory processing abilities is associated with cognitive declines, including specific executive function deficits (Gates et al, Citation2002, Citation2010, Citation2011; Idrizbegovic, Citation2011).
Recent cross-sectional data suggest that HL is associated with poorer long-term memory (LTM; both semantic and episodic), but only to a lesser degree with short-term or working memory (Rönnberg et al, Citation2011a,Citationb, Citation2014). This is also true when age is accounted for (the Betula database, Nilsson et al, Citation1997; Rönnberg et al, Citation2011a,Citationb), and even when episodic LTM is assessed using visuo-spatial materials (Rönnberg et al, Citation2014; the UK Biobank Resource). The use of hearing aids may interact with the relationship between memory and hearing (Amieva et al, Citation2015). For example, Rönnberg et al (Citation2014) were also able to show that hearing aid users performed slightly better than non-users on a visual working memory task. This cross-modal effect of auditory intervention on visuo-spatial processing suggests that in the long-term, HL may also impair central (or multi-modal) WMC, not only modality-specific working memory abilities (see also Dupuis et al, Citation2015; Verhaegen et al, Citation2014).
However, what is still lacking is a more comprehensive and longitudinal account (cf. Lin et al, Citation2011, Citation2013) of the mechanisms underlying the correlations between HL and cognition, including the transitions between healthy and pathological functioning such as in incident dementia. Also lacking is a more detailed and theoretically driven description of cognitive and perceptual predictors of speech-in-noise criterion variables.
Purpose
The current study is the first investigation in a series of studies based on the n200 database. The name n200 reflects the fact that the number of participants in each of three groups will be around 200. The data collection for the first of those three groups has been completed; it forms the basis for a cross-sectional study of 200 participants with HL who use hearing aids in daily life (age range: 37–77 years). It has also been extended to include a longitudinal study with three test occasions (T1–T3, with 5 years between test occasions) and two control groups of around 200 persons each. The two control groups, one comprised of individuals with normal hearing and the other of individuals with HL who do not use hearing aids, are matched for age and gender. The current paper is based on data collected from the 200 participants with HL who use hearing aids.
The aim of the current paper is threefold: (1) to present a test battery which is based on well-established tests as well as new, theoretically motivated (cognitive) experimental measures belonging to three classes of tests: hearing, cognitive and speech-in-noise outcome test variables, (2) to statistically examine how the test variables from the three classes of tests are structurally related to each other, taking age into account and (3) to address theoretical and clinical implications from the overall picture of the data.
The focus of the current study was to present the overall pattern of data, which means that detailed analyses of specific hearing and cognitive predictor variables and specific outcome test conditions, subjective or objective, are not included this study. These kinds of studies, where we, for example, relate different kinds of working memory tests to certain signal processing conditions, or where we (as is typically done) analyse subjective ratings into their component scales (i.e. the SSQ, see below), are planned for subsequent reports.
The research and the overall rationale for the selection of the types of cognitive tests have been generally driven by the fact that the tests have been found to be associated with communicative outcomes, primarily with speech understanding under adverse or challenging conditions (for reviews, see Mattys et al, Citation2012; Rönnberg et al, Citation2013; Schmulian Taljaard et al, Citation2016). More specifically, the tests included in the battery tap into different operationalisations of the components of and the predictions by the Ease of Language Understanding (ELU) model (Rönnberg, Citation2003; Rönnberg et al, Citation2008, Citation2011a,Citationb, Citation2013). The research and the overall rationale for the selection of the types of cognitive tests have also been driven by accounts of speech processing involving measures of executive function (Mishra, Citation2014; Rudner & Lunner, Citation2014).
Hearing tests
The hearing tests were selected for their ability to identify different aetiologies of the hearing impairment based on the reasoning in Stenfelt and Rönnberg (Citation2009, see also Neher et al, Citation2012). The hearing thresholds were measured to obtain the hearing sensitivity of the participant and together with the bone conduction thresholds conductive hearing losses could be identified. The distortion product oto-acoustic emissions (DPOAEs) were tested to determine the function of the outer hair cells and Threshold-Equalizing Noise – Hearing Level (TEN HL) for the integrity of larger areas of inner hair cells. Phonetically balanced (PB) words in quiet together with pure tone thresholds can be used to estimate the influence of any auditory neuropathy. The Spectro-Temporal Modulation (STM) test by Bernstein et al (Citation2013) was used as a general test of modulation detection and the Temporal Fine Structure Low Frequency test (TFS-LF), developed by Hopkins and Moore (Citation2010), was used to test the general ability to use pitch information.
Cognitive mechanisms and ELU
The ELU-model is primarily defined at a linguistic-cognitive level. It assumes that processing of spoken input involves Rapid Multimodal Binding of PHOnology (called RAMBPHO, Rönnberg et al, Citation2008), completed around 150–200 ms after stimulus presentation (Stenfelt & Rönnberg, Citation2009). The RAMBPHO component is assumed to contain short-lived phonological information in a buffer that binds and integrates multimodal information (typically audiovisual) about syllables, which in turn feed forward to semantic LTM in rapid succession. If the RAMBPHO-delivered sub-lexical information matches a corresponding syllabic phonological representation in semantic LTM, then lexical access will be successful, including speed of access, both of which are crucial to speech understanding success (Ng et al, Citation2013b; Rönnberg, Citation1990, Citation2003). In its most general form, the ELU-model encompasses phonological information mediated by the tactile sense, lip-reading and sign language (Rönnberg, Citation2003). In the current study, only auditory and audiovisual speech are studied.
RAMBPHO concerns automatic and implicit processing. Lexical activation is compromised if there is a mismatch between the incoming RAMBPHO-processed stimuli and phonological representations in semantic LTM; i.e. when there are too few overlapping (matching) phonological and semantic attributes, immediate lexical entry is denied (Rönnberg et al, Citation2013 for details; cf. Luce & Pisoni, Citation1998). In such cases, the basic prediction is that explicit, elaborative and relatively slower WMC-based inference-making processes are invoked to aid the reconstruction of what was said. Mismatches may be due to one or more variables, including the type of HL, adverse signal-to-noise ratio conditions, competing talkers, unfamiliar output from signal processing in the hearing aid or excessive demands on cognitive or lexical processing speed. The relative effects of these variables on recovery from a mismatch in the ELU model can be evaluated and estimated with the current test battery.
WMC: In the Rönnberg et al (Citation2013) version of the ELU-model, WMC plays two different roles: a post-dictive role that supports communication repair (cf. above) and a predictive role that involves priming or pre-tuning of RAMBPHO processing. Evidence of the latter role is based on the findings that WMC affects early attention processes in the brainstem (cf. Sörqvist et al, Citation2012; Anderson et al, Citation2013; Kraus &White-Schwoch, 2015; cf. the early filter model by Marsh & Campbell, Citation2016), which propagates to the cortical level, especially under conditions where memory load is high and concentration is demanded (Mattys et al, Citation2012; Sörqvist et al, Citation2016). Higher levels of linguistic predictions also involve WMC (Huettig & Janse, Citation2016).
Hearing impairment: The post-cochlear input to the linguistic-cognitive level of analysis is assumed to consist of neural patterns that can be recognized as particular phonemes (cf. Stenfelt & Rönnberg, Citation2009). These neural patterns will presumably be altered depending on the type of impairment (damage to the inner and/or outer hair cells and/or auditory nerve pathology, see Stenfelt & Rönnberg, Citation2009) and by temporal fine structure functions (suprathreshold measures) of the auditory system (e.g., Neher et al, Citation2012; Füllgrabe et al, Citation2015; Marsh & Campbell, Citation2016). In order to better understand the interplay between the auditory and cognitive systems, including speech perception and understanding abilities, we have collected the first wave of data on 200 participants with respect to degree and type of impairment, tapping into suprathreshold properties of the auditory system. This group was fitted with hearing aids.
The current study is an ELU-based comprehensive first account of how the impairment, RAMBPHO-related phonological skills, WMC and executive and inference-making functions, as well as speech understanding in noise (with and without signal processing) relate and interact. The extensive test battery and the large number of participants enable unique comparisons. Thus, the cognitive components that have been grouped conceptually in the current analyses may be found to have hitherto unexpected interrelationships and factor structures (latent variable constructs), as well as new kinds of relationships with the speech-in-noise outcome constructs. In addition, and as noted previously, we have observed associations between degree of HL and episodic LTM (Rönnberg et al, Citation2011a,Citationb) and we have claimed that the effect is multi-modal and represents a potential link to dementia (Lin et al, Citation2011; Rönnberg et al, Citation2014). In a recent paper, Waldhauser et al (Citation2016) suggested a mechanism that fits with the above claim: episodic memory retrieval is dependent on the ability to generate short-lived sensory representations of the encoding experience. In the study by Waldhauser et al (Citation2016), the fidelity of the original sensory representation was reflected by lateralized alpha/beta (10–25 Hz) power of the electroencephalographic (EEG) signal. This mechanism agrees with the suggested ELU-mechanism behind episodic memory loss in people with HL (Rönnberg et al, Citation2011a,Citationb): due to a less than optimal auditory input, short-lived RAMBPHO-related information will mismatch more frequently with representations in semantic LTM, hence causing poorer encoding and subsequent retrieval from episodic memory. This in turn causes a relative disuse of episodic LTM compared to WM, which in turn is occupied with reconstructing and predicting what is to come in a dialogue, and hence, would be used more frequently than episodic LTM.
Therefore, partly for the purposes of the cross-sectional analyses but also for longitudinal reports to come, examining putative links between HL and cognitive impairment in aging (e.g., Lin et al, Citation2011), tests of general cognitive function were added to the battery. Also, semantic, but not episodic memory tests, were included together with the on-line storage and processing tests related to the ELU-model, to make a conservative evaluation of the existence of negative relationships between HL and cognition.
The test descriptions below are brief, with the purpose of relating and conceptually organizing the tests according to the ELU model components. The detailed test descriptions can be found under Methods in Supplementary materials.
Phonological processing mechanisms and ELU
Gating: The ELU model proposes that sub-lexical phonological functions as represented by RAMBPHO are crucial. We tested the participants’ phonological abilities by using the gating paradigm (Grosjean, Citation1980; Moradi et al, Citation2013, Citation2014a,Citationb, Citation2016). This paradigm measures the duration of the initial portion of the signal that is required for correct identification of speech stimuli (in this case vowel and consonant identification). Phonological, sub-lexical representations were also assessed by means of vowel duration discrimination (Lidestam, Citation2009) and rhyme (e.g. Classon et al, Citation2013).
Semantic LTM access speed and ELU
Physical matching (PM) and lexical decision (LD) speed measures, as well as rhyme decision speed were included (cf. Rönnberg, Citation1990). Speed of phonologically accessing the lexicon in semantic LTM is a crucial factor in the ELU model (Rönnberg, Citation2003).
Working memory mechanisms and ELU
The choice of WM tasks was guided by two principles: first, the emphasis was on storage and processing, and second, stimulus modality. Regarding the first principle, we used one task that emphasized storage only (i.e. a non-word serial recall task (NSR); Sahlén, Citation1999; Baddeley, Citation2012), and three tasks that in different ways demanded both storage and semantic processing (i.e. the reading span (RST), semantic word-pair span (SWPST) and the Visuo-Spatial Working Memory test (VSWM); Rönnberg et al, Citation1989; Lunner, Citation2003; Foo et al, Citation2007, see details under Methods section). Two of the latter three tasks required the participant to recall target words in sentences/word-pairs (storage aspect) and to make semantic verification judgments of the content of sentences (RST) or by means of semantic classification into pre-defined semantic categories (SWPST). The semantic judgement/classification decisions thus represent the processing aspect of the tasks.
Second, while the NSR, the reading span and SWPST tasks were text-based, we also included the VSWM. In this task, ellipsoids are shown in spatial locations in a matrix. Participants make decisions regarding the visual similarity of pairs of ellipsoids in a given cell of the matrix while having to remember the order of locations of the pairs of ellipsoids (cf. Olsson & Poom, Citation2005). The dual task requirement is analogous to the requirements for the RST and SWPST.
Executive and inference-making mechanisms and ELU
The selection of executive tests was based on the subdivision of executive functions into shifting, updating and inhibition proposed by Miyake and Shah (Citation1999, see also Diamond, Citation2013) Executive functions (EF) are important in speech perception, e.g. when tracking a talker in a cocktail party situation while inhibiting other voices (Sörqvist & Rönnberg, 2012). EF may also interact with WM when there is a need for storage to support explicit executive processing demands (cf. Sörqvist & Rönnberg, 2012; Rudner & Lunner, Citation2014). Verbal inference-making tests represent another explicit mechanism that interacts with WMC (here operationalised as the sentence completion test, SCT, Lyxell & Rönnberg, Citation1987, Citation1989; and the Text-Reception Threshold, TRT, Zekveld et al, Citation2007), and were, therefore, included in the battery.
Shifting was tested by the speed of categorizing items (e.g. digit–letter pairs presented on a screen) according to a relevant dimension (whether it is an odd/even number or a Capital/small letter). Depending on the position of the stimuli on the screen, shifting between dimensions is necessary.
Updating was operationalised as a memory task and concerns monitoring and keeping track of information according to some criterion and then appropriately revising the items held in working memory by replacing old, no longer relevant information with newer, more relevant information (keeping in mind the last word of four pre-defined semantic categories, e.g. fruits).
Inhibition errors were measured in a simple fashion: the participants watched a sequence of random digits and their task was to press the “space bar” every time they saw a digit, unless it was the digit 3.
The SCT (Lyxell & Rönnberg, Citation1987) was employed as another verbal inference-making test administered under time pressure, with prior contextual cues offered.
The TRT test (Zekveld et al, Citation2007) is based on an adaptive procedure that determines the percentage of unmasked text needed to read 50% of the words in a sentence correctly. The TRT is based on inference making from the sentence without use of prior contextual cues. Both the TRT and SCT have been proven to predict speech in noise understanding (Zekveld et al, Citation2007) and contextually driven speechreading (Lyxell & Rönnberg, Citation1987, Citation1989).
A Hannon–Daneman (Citation2001) type of test was employed to assess the participant’s ability to combine information about real and imaginary worlds from several written statements and to draw related inferences, here denoted Logical Inference-making Test (LIT).
General cognitive functioning
The standard Mini Mental State Examination test (MMSE, Folstein et al, Citation1975) was used for cognitive screening along with a Rapid Automatised Naming test (RAN) test. RAN is a quickly administered cognitive speed test (e.g. Wiig & Nielsen (Citation2012)) which has also been shown to be sensitive to Alzheimers disease (Palmqvist et al, Citation2010). Raven’s matrices (Raven, Citation1938; Raven, Citation2008) were used as a basic non-verbal IQ estimate.
Outcome variables and ELU
The Hagerman sentences (Hagerman, Citation1982; Hagerman & Kinnefors, Citation1995), which are Swedish matrix-type sentences, were used to test speech understanding in noise. The speech signal was presented using an experimental hearing aid that allowed us to assess and compare the effects of linear amplification, compression and noise reduction in different noise backgrounds on speech understanding and their interactions (Foo et al, Citation2007; Lunner & Sundewall-Thorén, Citation2009; Souza et al, Citation2015; Ng et al, Citation2015; Souza & Sirow, 2015). For the purpose of this overview paper, we will statistically treat all Hagerman conditions together. Details of the interactions between signal processing conditions and specific cognitive functions will be analysed in papers to come. In addition to the Hagerman sentences, we also employed other outcome variables where modality, contextual and semantic components were emphasized and manipulated (Samuelsson & Rönnberg, Citation1993; Hannon & Daneman, Citation2001; Sörqvist et al, Citation2012).
The general prediction from previous research is that there are speech understanding situations (e.g. in mismatch conditions; Foo et al, Citation2007; Rudner et al, Citation2009, Citation2011) in which the dependence on WMC (and WMC-related cognitive functions) increases when there is less contextual support (i.e., comparing context-free Hagerman with daily Swedish HINT sentences, Hällgren et al, Citation2006). By implication, we predict weaker associations between WMC and understanding of contextually rich (cued) spoken sentences in noise (e.g. Samuelsson & Rönnberg, Citation1993). We also expected that the understanding of audiovisual sentences compared to auditory-only would be less dependent on WMC (cf. previous sentence and phoneme-based gating data, Moradi et al, Citation2013).
An auditory version of the Hannon and Daneman (Citation2001) materials was used to provide an indicator of the predictive nature of Auditory Inference-Making (here denoted AIM) ability in relation to speech understanding (see Supplementary materials).
In addition to the objective criterion variables, ratings were obtained using the Swedish version of the Speech, Spatial and Qualities of Hearing Scale (SSQ) questionnaire developed by Gatehouse and Noble (Citation2004, see website: http://www.ihr.mrc.ac.uk/pages/products/ssq).
Summary of expectations and predictions
In sum, with the current test battery of hearing, cognitive and outcome variables, the following general predictions were made:
First, since the ELU-model consists of independent, but interacting cognitive components (e.g. Rönnberg et al, Citation2013), one general expectation is that all cognitive variables would be subsumed under one factor in an overall exploratory factor analysis.
Second, because of the different hearing and cognitive functions they tap into, one expectation was that the outcome variables would result in one context-driven factor (mainly the HINT, Hällgren et al, Citation2006, and Samuelsson & Rönnberg test, Citation1993) and one where context is less important (mainly the Hagerman, Citation1982). The hearing variables would also be split up into sensitivity (i.e. thresholds) on one hand and suprathreshold, temporal fine structure tests on the other hand (mainly STM and TFS-LF).
Third, we predicted that performance on the outcome tests that are relatively context-independent (e.g. the Hagerman sentences) would be associated to a higher degree with the hearing and cognition factors than would performance on the context-dependent tests (e.g. Rönnberg et al, Citation2013; Samuelsson & Rönnberg, Citation1993).
Fourth, we expected the measures of inner/outer hair cells and temporal fine structure to be related to speech understanding in noise, via relations to cognitive components (Stenfelt & Rönnberg, Citation2009).
Fifth, we expected that hearing sensitivity (i.e. thresholds) would relate to the cognitive functions, but less so than in previous work because the current test battery included on-line cognitive processing and excluded episodic memory functions (Rönnberg et al, 2011, Citation2014).
Methods
All tests were conducted by two clinical audiologists. In , we provide executive summary test descriptions and measurement objectives for all tests variables included. gives the descriptive statistical information for each test. For detailed test descriptions, see Supplementary materials at http://tandfonline.com/doi/suppl.
Table 1. Summary test descriptions and measurement objectives for all tests variables.
Table 2. Summary of means and SDs for all the main dependent variables used in the factor analyses.
Hearing-impaired participants
Two-hundred and fifteen experienced hearing aids users with bilateral, symmetrical mild to severe sensorineural HL were recruited from the patient population at the University Hospital of Linköping. Out of the 215 consenting participants, 15 dropped out at either Session two or three (see below). All participants fulfilled the following criteria: they were all bilaterally fitted with hearing aids and had used the aids for more than 1 year at the time of testing. Audiometric testing included ear-specific air-conduction and bone-conduction thresholds. Participants with a difference of more than 10 dB between the bone-conduction and air-conduction threshold at any two consecutive frequencies were not included in the study.
The 200 included participants were on average 61.0 years old (SD = 8.4, range: 33–80) and 86 were females. They had an average four-frequency pure-tone average (PTA4 at .5, 1, 2 and 4 kHz) in the better ear of 37.4 dB HL (SD = 10.7, range: 10–75). They reported having had hearing problems for an average of 14.8 years (SD = 12.4, range: 1.5–67) and they had used their hearing aids on average for 6.7 years (SD = 6.6, range: 1–45). They had on average 13.4 years of education (SD = 3.4, range: 6–25.5) and 168 were married or co-habiting. All participants gave informed consent. All participants had normal or corrected-to normal vision, and they were all native Swedish speakers.
Aetiology of the impairment varied in this sample. Therefore, inner and outer hair cell integrity and auditory processing abilities were measured to unveil the possible effects of different types of distorted auditory neural signals on the linguistic-cognitive level of cortex-based processing (see Stenfelt & Rönnberg, Citation2009). To study how inner ear damage is related to auditory and cognitive performance, DPOAE (Neely et al, Citation2003) were obtained to measure outer hair cell integrity, and a threshold-equalizing noise test with calibrations in dB HL, or TEN(HL) (Moore et al, Citation2004), was applied to estimate inner hair cell integrity.
Two experimental tests assessed the ability to detect spectro-temporal modulations (STM), which is predictive of speech in noise (Bernstein et al, Citation2013). The second test examines the ability to detect changes in temporal fine structure for low frequencies (TFS-LF, Hopkins & Moore, Citation2010; Neher et al, Citation2012). Finally, an unaided word recognition accuracy score (in quiet) was also collected using phonemically balanced (PB) word-lists (see Methods section in Supplementary materials for detail).
Results and discussion
Descriptive data
First, we present the descriptive data for all tests below (see for the mean, SD and range for each variable). Where there are other published data collected under similar circumstances, we compare them with the current data set to check for similarities and replication. Note that the variables selected for the purpose of this overview of the database are main variables or composites for each test. Later reports will deal with the details of each test and with multivariate statistical modelling.
In , we have organized the data according to the order of the three classes of test variables presented in the Methods section, which is also the basis for subsequent factor analyses.
Hearing variables
The current sample is within the mild to severe hearing impairment range, measured with air conduction thresholds, here averaged over four frequencies (PTA4 = 0.5, 1, 2 and 4 kHz), with 39.09 dB HL (SD = 10.88 dB) for the left ear and 38.91 dB HL (SD = 11.27 dB) for the right ear. Bone conduction thresholds were on average 1.7–1.9 dB lower for the two sides, indicating that participants with conductive losses were successfully excluded from the study (see for audiometric thresholds across the whole frequency range measured).
Figure 1. Hearing thresholds, means and SDs for the entire frequency range measured for the 200 hearing-impaired participants.
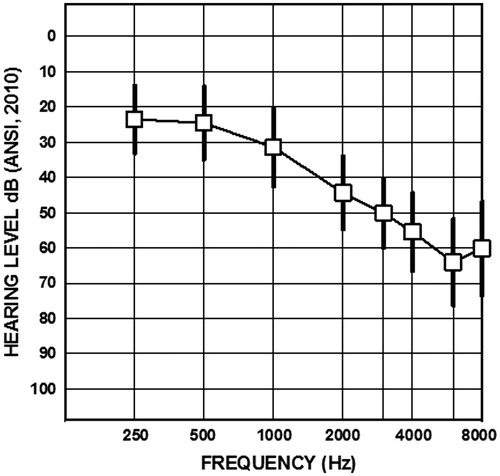
The TEN HL was scored for number of dead regions identified. A masked threshold value of 15 dB SL or more was used here as the limit for an identified dead region. Four frequencies (0.5, 1, 2 and 4 kHz) were tested for each ear meaning that the possible scores ranges from 0 (no dead region) to 8 (dead regions at all tested frequencies). Most of the tested participants (n = 155, 77.1%) had no indication of any dead region (score 0), 30 participants had one dead region (14.9%) and 16 had two or more dead regions (8.0%).
The DPOAEs were scored as either present (SNR> =6 dB) at some level (40–60 dB SPL) or absent (SNR < 6 dB) at all levels (40–60 dB SPL) for the three test frequencies (1, 2 and 4 kHz) and for each ear. This gives a maximum score of 6 (1 score given for presence of DPOAEs at each frequency and each ear and 0 for the absence) and the average score here was 2.12 (SD = 1.14). This means that the participants had, on an average, detectable OAEs at two of the six tested frequencies.
TFS-LF: The average result of the TFS-LF test was 59.3° (SD = 75.13°). This result is markedly larger than the data in Hopkins and Moore (2011) where they report an average TFS-LF of just below 20° in a group of hearing impaired participants. Those participants had PTA4s that were lower than in the present study (PTA4, right ear: 31.2 dB HL), but the average age was similar to our study (62.8 years). Consequently, our participants seemed to have a significantly worse ability to process low-frequency fine structure information than people with normal hearing or persons with slightly milder HL. One possible reason for the higher means here than in the Hopkins and Moore (2011) study is that the distribution of the variable is rather skewed. This has larger effects for larger sample sizes since the extreme values could be so much higher. In our data, 163 participants had measures with the normal procedure and the rest had measures with the non-adaptive percent-correct procedure described in the method section. The participants in the latter group had much higher TFS values, indicating that the standard procedure for this calculation might need a revision. The first group had a mean of 29 but a median of 22 which indicates that the distribution is somewhat skewed even in this group. Twenty-two is relatively close to the Hopkins and Moore mean so we conclude that the data here are in line with Hopkins and Moore (2011).
STM: The modulation depth was around −3 dB SNR in the current study and around −9 dB SNR in the Bernstein et al (Citation2013) study. However, that study did not use a low-pass filter, which we did (the low-pass filter was 2 kHz, which could explain at least some of the difference). Rather than employing a broadband carrier in the current study, we used the low-pass filter at 2 kHz for two reasons. First, Mehraei et al (Citation2014) investigated the effects of carrier-frequency on the relationship between STM sensitivity and speech-reception performance in noise and found that the relationship was strongest with a low-frequency STM carrier. Second, the goal of the study was to identify a psychophysical test that could account for variance in speech-performance not accounted for by the audiogram, and the high-frequency components of the audiogram (i.e. 2 kHz or greater) that are typically most highly correlated with speech perception (see also Bernstein et al, Citation2015).
The PB word test scores were at 95% (SD = 5.92%) showing a high performance on word recognition in quiet.
Cognitive variables
Some of the test parameters presented below are collapsed into one main variable and can be directly compared with previous data, whereas other experimental tests that consist of several manipulated variables, especially those which are relatively new, were analyzed with t-tests or ANOVAs for future reference.
Phonological tests
Consonant gating: The mean IP for correct consonants for A presentation was 288 ms (SD = 102 ms) and, for AV presentation, it was 196 ms (SD = 54 ms), t(198) = 13.64, p < 0.001, d = 0.97, showing that AV identification occurs earlier than A identification. The present results replicate earlier research (Munhall & Tohkura, Citation1998; Moradi et al, Citation2014a,Citationb) reporting a mean percentage correct for correct consonants for A presentation to be 81% (SD = 22%, range 20–100%) and for AV presentation 96% (SD = 9%, range 60–100%), t(199) = 9.67, p < 0.001, d = 0.68, showing also better accuracy for AV identification.
Vowel gating task: The mean IP for entirely correct vowels was, for A presentation, 222 ms (SD = 85 ms), and, for AV presentation, 208 ms (SD = 81 ms), t(198) = 2.60, p = 0.01, d = 0.18, thus showing a small but significant benefit of AV vowel identification in terms of identification speed. The mean percentage correct for A presentation was 71% (SD = 21%, range 20–100%), and, for AV presentation, it was 77% (SD = 20%, range 20–100%), t(199) = 4.20, p < 0.001, d = 0.30, meaning that AV identification was more accurate.
Vowel duration discrimination task: For A presentation, the mean was 7.18 errors (SD = 4.62 errors, range 0–20 errors), while AV presentation resulted in 7.05 errors (SD = 4.77 errors, range 0–20 errors), t(198) = 0.39, p = 0.70, thus revealing no significant benefit of AV over A presentation.
The rhyme judgment test is a phonological representation test (Lyxell et al, Citation1996; Classon et al, Citation2013), where the matching and mismatching conditions are not analysed separately in this overview paper. Since most conditions produce high proportions correct in this sample (i.e. 87%) compared with samples with more pronounced hearing impairment (e.g. Lyxell et al, Citation1996; Classon et al, Citation2013), we here focus on the latency data for correct responses (showing a mean of 1685 ms, SD = 404), which is in line with previous studies (Lyxell et al, Citation1996, Citation1998).
Semantic LTM access speed
The PM and LD tasks replicate very closely the results of previous independent studies (i.e. with access speeds just below one second; cf. Perfetti, Citation1983; Hunt, Citation1985; Rönnberg, Citation1990). There seems to be good agreement across studies for our computer-based applications of the tasks (e.g. Rönnberg et al, Citation1989; Rönnberg, Citation1990), with the current sample being around 0.1 s slower than the earlier samples, but the current sample is also around 10 years older.
Working memory
NSR: The level of performance (24/42 = 57%) is comparable with other independent data on adults (Majerus & van der Linden, 2010).
RST: The RST has been used in many studies from our research group and the average score from all these studies is just below 45% for participants with hearing loss and long versions of the test (cf. Besser et al, Citation2013; Ng et al, Citation2014). When a short version of the task is used (with 24 or 28 items), the accuracy goes up 10–15% percent (see Hua et al, Citation2014; Ng et al, Citation2014), which is quite comparable with our 57% performance in the current sample (16 out of max = 28). Other independent labs show comparable scores with the long versions (e.g. Besser et al, Citation2013; Keidser et al, Citation2015; Souza et al, Citation2015a).
SWPST: Since the SWPST is lacking the grammatical component that is included in the RST, but similar to the RST in terms of a semantic processing task and post-cuing of recall, we expected performance levels to be lower than for the RST, which was found to be the case (41% vs. 57%).
VSWM: The data (29/42, 69% in total score) suggest a span size of close to 4, which is quite comparable with the data reported in Olsson and Poom (Citation2005).
Executive and inference-making tasks
Shifting: The shifting cost was 760 ms, which is slightly greater than in a younger hearing-impaired sample (Hua et al, Citation2014).
Updating: The current sample performed at a 63% level (i.e. 10/16, SD = 2.87), which again would be compatible with the 70% obtained in the Hua et al (Citation2014) study.
Inhibition: The error rate in the inhibition task was 3.1 in the Hua et al (Citation2014) study and in the current sample it was 1.72 (SD = 2.04), which actually is relatively low, but still within 1 SD compared with the Hua et al (Citation2014) study.
TRT: The current mean value in percentage of unmasked text, i.e. 53%, to reach the criterion of 50% correct responses, agrees very well with previous studies. These showed that the average TRT (in percentage unmasked text) is around 54% (Zekveld et al, Citation2007; Kramer et al, Citation2009) for younger participants (mean age around 35 years) and around 56% for somewhat older participants (mean age around 45 years, in Besser et al, Citation2012).
SCT: In a similar test version, Andersson et al (Citation2001) found that mean SCT performance was 71%, but that level of performance applied to a sample with more profound hearing impairment. The current high performance level (0.83) is still within one SD of the Andersson et al. studies.
LIT: The average score was 64.50, with an SD of 16.81.
General cognitive functioning
Mini Mental State Examination Test (MMSE): The current sample scored on average 28.4/30 on the MMSE. This average score is similar to a previous sample of 160 hearing-aid wearers (Rönnberg et al, 2011), who scored 27.3/30, thus within 1 SD of the current sample. Only 16 participants scored below 27.
Rapid Automatised Naming test (RAN) Test: The RAN time for the colour and form naming combined (RANCS) was 60 s. This result is comparable with other samples (Wiig & Al-Halees, Citation2013). The difference score between RANCS and the sum of RANC + RANS is also positive and similar in magnitude to previous research (Warkentin et al, Citation2005; Wiig et al, Citation2010).
Raven test: The Raven test score on sets D and E of Raven was 64%, and it was 81% on set D. These results are very close to independent and recent data by Kilman et al (Citation2015), where performance for a slightly younger adult hearing impaired sample was 83% for set D.
Outcome variables
The Swedish HINT
The 50% threshold was −1.43 (SD = 1.85), which is comparable with an average of −1.9 (SD = 2.2) in a study by Kilman et al (Citation2015) on another sample of hearing impaired participants.
The Hagerman test conditions
To give an overview of the test results, an analysis of variance (ANOVA) with three within-subject factors, background noise (unmodulated stationary noise, 4-talker babble), signal processing (linear amplification with NR, linear amplification without NR, fast-acting compression without NR) and SNR (50% and 80%) was performed (overall result = −2.87 dB SNR (SD = 1.77), but see for means in each condition, and for insertion gain response curves). Overall, there was a main effect of SNR, indicating that the average SNR obtained at 50% was lower than that at 80%, F(1, 199) = 2295.55, p < 0.001 (e.g. Foo et al, Citation2007). The main effect of background noise was significant, F (1, 199) = 3864.86, p < 0.001, demonstrating that 4-talker babble had a stronger masking effect than stationary noise. The magnitude of this effect is comparable with that in previous studies (cf. , Wang et al, Citation2009; Ng et al, Citation2013b). There was also a significant main effect of signal processing, F(2, 398) = 2233.67, p < 0.001. The post hoc t-tests (Bonferroni adjusted for multiple comparisons) showed that test performance with linear amplification with NR was better (i.e. with a lower average SNR) than test performance with the linear amplification without NR (p < 0.001) (Wang et al, Citation2009), which again was better than the performance found in the fast-acting compression without NR condition (p < 0.001). In other words, linear amplification generally resulted in better speech recognition performance than non-linear amplification with fast-acting compression.
Figure 2. Insertion gain response curves for the linear amplifications (upper panel) and amplification with fast-acting compression (lower panel). Curves in solid lines represent insertion gain based on the average hearing thresholds at 125 through 8000 Hz. Curves in dotted lines represent insertion gain response based on hearing thresholds one standard deviation above/below average.
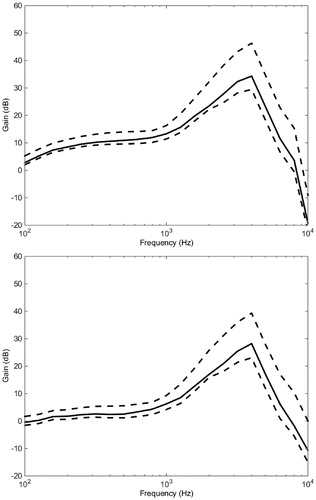
Compared with the original test results in a study by Hagerman and Kinnefors (Citation1995), the current sample produced somewhat flatter slopes (ranging from 5.33 to 6.98%/dB in the six test conditions). Slightly worse hearing sensitivity in the sample tested in the present study (average PTA4 = 39 dB HL vs. 34 dB HL for the sample in the study of Hagerman and Kinnefors) and different configurations of HL (slight sloping HL in the present study versus sloping HL in the Hagerman and Kinnefors study) could have contributed to flatter psychometric functions (see e.g. Takahashi & Bacon, Citation1992). As a final general observation, the standard deviations (SDs) in the present study were larger in the harder 80% condition (SDs from 2.95 to 3.58) than in the 50% condition (SDs from 1.59 to 1.88), which makes sense from the perspective of individual differences that play out with the higher cognitive load in the 80% condition.
The Samuelsson & Rönnberg sentences
For reasons of simplicity in this overall analysis, we focused on the context and modality manipulations only. The modality manipulation was not present in the original Samuelsson and Rönnberg (Citation1993) study, as the study focused on lip-reading only. An ANOVA with two within-subject variables, namely context (with, without) and modality (auditory, audiovisual), was performed. As was the case in the original experiment, we observed a main effect of context, F(1, 199) = 17.16, p < 0.001. The main effect of modality was also significant, F(1, 199) = 989.61, p < 0.001, such that test performance in the audiovisual modality was better than that in the auditory modality. No significant interaction was present.
We did not analyse the effects of sentence type (typical vs. atypical), (abstract vs. detailed), temporal order (early or late event in the script) and type of script (three scripts) in this overall context, but all data are retained at that level of scoring for future reports.
The AIM
The average accuracy in terms of percent correct was 71% and the average reaction times were 6.68 s for the AIM.
The SSQ
The total score used for the present overall purpose was 324 out of 500. These results are comparable (within 1 SD) to previous studies on experienced hearing aid users (e.g. Gatehouse & Noble, Citation2004; Ng et al, Citation2013a).
Factor analyses
First, all variables were investigated for potential outliers. However, we believe that it is important to reflect as much variability as possible in this initial n200 paper. Therefore, we used a liberal criterion: Only values more than four standard deviations from the mean were removed. This resulted in the removal of four data points from the HINT, and one data point from the MMSE and inhibition tests, respectively. All analyses were first conducted for the results of the participants who had no missing data and then re-computed with imputed values for those with missing data. Imputation was done using the data imputation module in the AMOS software version 23 for each factor separately. No differences in the pattern of results were found between the analyses with or without imputed data and, therefore, only the analyses on the imputed data are reported in this article.
Data from the tests were analysed with exploratory factor analyses using maximum likelihood extraction and direct oblimin rotation using SPSS version 23 (see for an overview of the steps involved in the factor analyses). Factors were saved using the regression method. The factor analyses followed the a priori conceptualizations and categorizations of the tests included in the overall battery. For tests with many variables, we computed what is here called LEVEL 1 factor analyses to determine one score per test which then were used in the next level of factor analyses. LEVEL 1 factor analyses were computed for the following tests including several sub-scores: Audiograms, STM sensitivity, Hagerman sentences, and Samuelsson & Rönnberg sentences. LEVEL 1 factor analyses were also computed for the cognitive sub-functions Phonology, Semantic LTM access speed, WM, Executive and Inference-making functions, and General Cognitive Functioning. After the LEVEL 1 factor analyses, the individual factor scores were saved for the next level. In the LEVEL 2 factor analyses, HEARING, COGNITION and speech understanding OUTCOMES were analysed separately and here the number of factors extracted was determined by an Eigenvalue larger than 1 (see ). Finally, a correlation matrix was constructed based on the factor scores of the LEVEL 2 factors in the overall matrix (see and ).
Table 3. Summary of all factor analyses.
Table 4. Summary of LEVEL 2 Factor analyses. The scale has been reversed for some variables (marked with *) such that higher values on all variables represent better HEARING, COGNITION, and speech in noise OUTCOME abilities. This was done to make the interpretations of the factors easier.
Table 5. Correlations among the LEVEL 2 components.
Table 6. Correlations among the LEVEL 2 components, with age partialled out.
The overall results of all factor analyses are seen in . Data were promising for the factor analyses as indicated by the fact that all analyses had significant results on Bartlett’s test of sphericity (all ps < 0.001) and most Kaiser–Meyer–Olkin measure (KMO) values were 0.60 or higher. The analyses of Executive and inference-making tests and Phonological processing mechanisms (see under Cognitive Mechanisms and ELU for tests included under these conceptual headings) would have resulted in two and three factors, respectively, meeting the Eigenvalue criteria, but since we used the a priori conceptualization of one factor per sub-function, only 31% and 34% of the variance was explained by the first factor in those cases. The General Cognitive Functioning factor also explained a relatively small amount of variance, which is reasonable since the sample is relatively well functioning at this point in time.
For the remaining LEVEL 1 cases, the factor solutions were clear-cut and strong (see ). All factor analyses are discussed in more detail below. For a summary of all LEVEL 1 factor analyses, again see . For the interested reader, each of the LEVEL 1 factor analyses with factor loadings is presented in the Supplementary materials (in ).
HEARING
LEVEL 1: The first large class of variables is based on the hearing tests. Here, we computed one separate factor analysis for the PTA, based on air- and bone-conduction thresholds, for the left and the right ear, giving four parameters. Each parameter was pooled over the values for 0.5, 1, 2 and 4 kHz. The results show unequivocally that the four parameters load on one factor with factor loadings of 0.93 and higher (denoted PTA). We also computed a separate factor analysis of the STM test based on the three frequencies used, for the left and the right ear, giving six parameters. The analysis resulted in a one-factor solution, with factor loadings of 0.78 and higher (denoted STM). These two LEVEL 1 analyses thus indicate that the test variables converge on the same hearing mechanism for each test in a very clear-cut way (see Table 1 in Supplementary materials).
LEVEL 2: As a LEVEL 2 factor analysis of the HEARING variables, the factor scores from LEVEL 1 for PTA and STM (which best represent these tests) were further factor analysed together with the remaining HEARING parameters: TEN HL, DPOAE, TFS LF and PB. A two-factor solution was obtained and only factor loadings above .40 will be interpreted (see ). It is clear that the PTA loads heavily (0.91) on factor 1 (here labelled SENSITIVITY), the TFS-LF and STM tests load on factor 2, here labelled TEMPORAL FINE STRUCTURE (cf. Bernstein et al, Citation2015, submitted). The inner (TEN HL) and outer hair cell measures (DPOAE) do not seem to build on any of the other two factors (the factor loading is lower than .40 for each of the two tests, respectively), at least not with the current pooled measures.
COGNITION
LEVEL 1 factor analyses were run separately for the Phonological tests (i.e. the four summary variables of gating, i.e. the auditory or audiovisual conditions for vowels and consonants, rhyme accuracy and the auditory/audiovisual vowel durations), Long-term Memory Access indices (i.e. PM speed, LD speed, Rhyme reaction time, and the RANCS), the Working memory tests (i.e. NSR, RST, SWPST and VSWM span tests), the Executive and Inference-making tests (i.e. Shifting, Updating, Inhibition, TRT, SCT and LIT) and finally a separate factor analysis on the General Cognitive Functioning tests (i.e. the MMSE, RAN diff and Raven tests). See Table 2 in Supplementary materials for details of factor loadings.
The results showed that the Phonological tests yielded a one-factor solution where only the vowel gating conditions (A/AV) load above the .40 traditional criterion (AV = 0.73 and A = 0.69). Therefore, vowel processing (CV syllabic format in the test) is presumably the most indicative of the syllabic RAMBPHO processing for this hearing-impaired sample. The results are in line with previous research attesting to the importance for participants with hearing loss to be able to perceive vowels (Richie & Kewley-Port, Citation2008).
The Long-term Memory Access one-factor solution resulted in factor loadings above 0.58, for all three tests, with LD speed having the highest factor loading (1.00), hence being in line with a conceptualization of a more general speed factor for Long-Term Memory (e.g. Salthouse, Citation2000).
The Working Memory factor also produced an interesting domain-general factor across the four tests, with loadings between 0.57 and 0.68 (see Sörqvist et al, Citation2012, for comparable domain-general composites).
For the Executive and Inference-making factor the TRT, SC and RANC came out with reasonable loadings, the TRT and SCT having the highest (0.76 and −0.70, respectively). It thus seems like they both draw on some general linguistic closure ability (Besser et al, Citation2013).
The General Cognitive Functioning tests resulted in one factor with the MMSE predictably being the test with the highest factor load 1.00.
LEVEL 2: Finally, we computed a LEVEL 2 factor analysis and found an interesting one-factor solution with all LEVEL 1 factors loading higher than 0.43, and the Executive and Inference-making factor loading the highest (0.92), and with the LEVEL 1 Working memory factor loading the second highest (−0.63) (see ). Interestingly, both these factor constructs belong to the explicit part of the ELU model (Rönnberg et al, 2011, Citation2013), which in turn suggests that the global COGNITION factor should be interpreted from that perspective (see under Correlations among the LEVEL 2 factors, and ).
OUTCOMES
LEVEL 1: In the LEVEL 1 analyses, we tested whether all 12 Hagerman conditions loaded on one factor only. We found this to be the case, with loadings from 0.57 to 0.85 (see Table 3, Supplementary materials). Similarly, we tested whether the Samuelsson and Rönnberg main conditions (with/without context, combined with the auditory/audiovisual test modalities) also loaded on one single factor, and again, this was the case, with factor loadings from 0.56 to 0.89. At a general level, this is very reassuring because the basic mechanisms that each of the two tests measures seem reliable and valid across conditions (see Table 3 in Supplementary materials).
LEVEL 2: Our aim was to test the hypothesis that the OUTCOME variables tap into two conceptually different categories of OUTCOME variables, that is, the context-free and stereotypical Hagerman sentences and the more context-bound and naturalistic HINT and Samuelsson and Rönnberg sentences. To accomplish this, we computed a LEVEL 2 analysis together with the remaining OUTCOME variables (SSQ, the HINT and the AIM). According to our prediction, the analysis resulted in two factors, with the HINT and the Samuelsson & Rönnberg sentences loading on the first factor (here denoted the CONTEXT outcome). The Hagerman, the AIM and SSQ loaded on the second factor (here denoted NO CONTEXT, because the Hagerman sentences constituted the variable that loaded the highest on this second factor, and, therefore, must determine the interpretation of this factor; see ). This means that the hypothesis was confirmed in the sense that the Hagerman sentences tap an outcome dimension that is different from that tapped by the HINT and Samuelsson and Rönnberg materials (cf. Foo et al, Citation2007; Rudner et al, Citation2009, Citation2011). Furthermore, this finding is vital to future studies of intervention or rehabilitation outcomes because the most sensitive OUTCOME seems to be the NO CONTEXT type of materials. It is interesting that the AIM also loaded on this factor, but in the same way as for the LIT, it taps into logical thinking rather than the use of context for its solution. SSQ total loads on the Hagerman factor as well. However, we refrain from interpretation as the factor loading for the SSQ was very low and a future study will analyze how the subscales relate to the CONTEXT–NO CONTEXT outcomes.
In all, the two expectations regarding factorial structure were confirmed (see also ): first, the LEVEL 2 analysis revealed that a global COGNITION factor could be established, and that the explicit functions (executive and working memory) that have proven to be good predictors of speech understanding under adverse conditions also demonstrated the highest loadings on this ELU-factor. Second, the prediction of a division of the HEARING variables into threshold (i.e. SENSITIVITY) and suprathreshold factors (i.e., TEMPORAL FINE STRUCTURE) was also confirmed. Although not explicitly predicted, neither the cochlear measures of inner (TEN HL) and outer hair cells (DPOAE) loaded on any of the two hearing factors nor did they contribute to a separate third factor. Future studies will go deeper into potential subgroups of inner/outer hair cell damage.
With respect to the COGNITION factor, further evidence for the argument that WMC and Executive/inference-making components play important roles in the explicit part of the ELU-model can be found in the results of studies that have combined the executive component (especially inhibition) and WMC in one test. One such example is the size comparison span test (SIC span), which, in some cases, has been shown to be a better predictor of speech perception than the RST (Rönnberg et al, 2011; Sörqvist & Rönnberg, Citation2012). The same logic applies to the TRT test (Besser et al, Citation2013), which was the test with the highest load on the Executive-Inference-making factor in the LEVEL 1 analysis. This research track, where new tests are based on theoretically motivated components and then combined together, seems to be an important area on which future studies should focus.
Correlations among the LEVEL 2 factors
Pearson correlations were used to analyse the relations between the LEVEL 2 factors. Differences between correlations were analysed using the Fisher r-to-z transformation. As shown in , the global COGNITION factor is positively and significantly related to the NO CONTEXT OUTCOME factor, and also to the CONTEXT OUTCOME factor, albeit it to a lesser degree. The difference between the strengths of the correlation coefficients was significant (Z = 3.12, p < 0.01, one-tailed). This pattern of data generally replicates that found in a number of independent studies, both from within our laboratory (Lunner, Citation2003; Foo et al, Citation2007; Rudner et al, Citation2009, Citation2011; Zekveld et al, Citation2013) and from other independent laboratories (e.g., Akeroyd, Citation2008; Arehart et al, Citation2013; Besser et al, Citation2013; Souza & Sirow, Citation2014; Souza et al, Citation2015a,Citationb, see also Füllgrabe et al, Citation2015).
The significant difference in the strength of the relationships supports the hypothesis that when speech processing in noise is contextually driven, fewer explicit cognitive resources are required to resolve ambiguity than when contextual information is lacking (Moradi et al, Citation2013; Meister et al, Citation2016). Other independent studies suggest that the strength of correlations may vary depending on the type of WMC test (Sörqvist & Rönnberg, 2012; Smith & Pichora-Fuller, Citation2015) and the complexity of sentence materials (Heinrich et al, Citation2015; DeCaro et al, Citation2016). The strength of the current study is the sample size, and the fact that these correlations are based on abstract, general factors, which in turn are based on several test conditions (LEVEL 1) and tests (LEVEL 2). Even after statistically removing the effects of chronological age, the COGNITION–NO CONTEXT association and the COGNITION–CONTEXT associations remain significant (see ). This allows us to demonstrate that the strength of correlations between cognition and speech recognition in noise varies with context and knowledge. Thus, the third prediction was confirmed, a finding further supported by the final multiple regression analyses.
The TEMPORAL FINE STRUCTURE factor was positively and significantly related to the global COGNITION factor (see and ). The existence of a relationship between temporal fine structure and cognition has been the subject of relatively little research, but has been reported in recent studies by Lunner et al (Citation2012), Neher et al (2013) and Füllgrabe et al (Citation2015), and replicated in this study. This is in line with the general fourth prediction by Stenfelt and Rönnberg (Citation2009) about how distorted neural output from the cochlea and brainstem may affect linguistic and cognitive functions at the cortical level. However, the above studies have not pinpointed exact mechanisms as to why this is the case. How the DPOAE and TEN HL parameters relate to cognitive subfunctions such as phonology (Stenfelt & Rönnberg, Citation2009) has also yet to be determined.
One possible cognitive hearing science interpretation of this relationship relates to the cognitive task demands of the STM and TFS-LF tests. In both cases, sounds have to be kept in mind before internal temporal fine structure comparisons and decisions are made: that is, there is also an auditory memory component involved here. This memory component may well relate to some of the core components of the ELU-system (i.e. Phonology (RAMBPHO), which is a bottleneck of WM; parts of the Speed component (especially rhyme speed), but certainly also WM per se).
Another alternative interpretation relates to a general temporal processing ability that may support all components in the COGNITION factor (cf. Pichora-Fuller, Citation2003). This kind of reasoning is based on the fact that fine structure is dependent on the resolution in coding of the supra-threshold spectro-temporal speech sound patterns in the cochlea as well as from the auditory nerve and upwards. This may in turn be related to the SENSITIVITY aspect of hearing impairment. Both aspects are dependent on cochlear integrity. We also found that TEMPORAL FINE STRUCTURE was significantly and positively correlated with SENSITIVITY, even after partialling out for age ( and ). Temporal fine structure information can be important for pitch perception and for the ability to perceive speech in the dips of fluctuating background noise (Quin & Oxenham, 2003; Moore, Citation2008), the latter ability also being modulated by WMC (as part of the COGNITION factor, e.g., Lunner & Sundewall-Thorén, Citation2007; Rönnberg et al, 2010; Füllgrabe et al, Citation2015). Here, we again come back to the first interpretation, namely that our TFS tests actually place demand on an element of WM. Thus, the inter-correlations among COGNITION, SENSITIVITY and TEMPORAL FINE STRUCTURE factors suggest interesting theoretical and experimental possibilities that must be pursued to reach firm conclusions.
Of the two interpretations we have suggested, one is a cognitive more of a top-down, WM interpretation and the other is primarily a sensorineural, cochlear, or bottom-up interpretation. Presumably, there are other levels of interaction in the auditory system that are related. Two examples from brain research illustrate the complexity.
The first example relates to the possibility that at least part of the effects of temporal fine structure are finally determined at the cortical level. It has been proposed (e.g. Yonelinas, Citation2013) that hippocampal binding is important for the rapid computation of evolving percepts, as well as for working memory and LTM. Even more specifically, recent data suggest that the human brain (at least in the case of listeners with normal hearing) is extremely efficient in picking up temporal patterns within rapidly evolving sound sequences, presumably serving to aid the prediction of upcoming auditory events. Again, the hippocampus interacts with the primary auditory cortex and the inferior frontal gyrus to accomplish this feat (Barascud et al, Citation2016). The role played by the inferior frontal gyrus in sentence-based speech-in-noise tasks has recently been demonstrated by Zekveld et al (Citation2012). The findings show that the inferior frontal gyrus and mid temporal regions interact such that participants with low WMC activate these areas more than participants with high WMC do when they use semantic cues to aid speech perception in noise (see also Hassanpour et al, Citation2015).
Another example can be found at the level of the brainstem. Attending to a sound (counting deviants in a sequence of tones) increases the amplitude of the brainstem response. However, the brainstem response decreases when attention is shifted from the auditory modality to a visually based, n-back WM task, based on letter sequences. The brainstem response is further suppressed when the cognitive load of the n-back task increases, especially for participants with a high WMC (Sörqvist et al, Citation2012, see also Anderson et al, Citation2013; Kraus & White-Schwoch, Citation2015). Recent conceptualizations of a top-down governed, subcortical, early auditory filter model are in line with these results and account for the WMC data and interpretation referred to above (Marsh & Campbell, Citation2016).
The common denominator in these examples is that the temporal (Pichora-Fuller, Citation2003; Vaughn et al, 2006) and sensitivity aspects of the cochlear/brainstem output may be modulated both sub-cortically and cortically by cognitive factors such as WMC. The inter-correlation matrix supports that kind of interaction, but the level(s) at which the crucial interactions take place and the specific cognitive subcomponent(s) of the COGNITION factor that are the most influential in this process remain to be determined. According to an ELU-perspective (Rönnberg et al, Citation2013), it may be argued that WMC can serve a pre-dictive function, affecting brainstem processing of sound (Marsh & Campbell, Citation2016; Sörqvist et al, Citation2012). The arguments and data supporting a post-dictive and reconstructive function of WMC have already been presented by Rönnberg et al (Citation2013), and emphasize the role that WMC plays when there are conditions that for some individuals cause a mismatch between input and semantic LTM representations.
SENSITIVITY (dominated by PTA4) was positively and significantly related to COGNITION. The difference in strength between the COGNITION–SENSITIVITY and COGNITION–TEMPORAL FINE STRUCTURE correlations was significantly different, Z = 2.45, p < 0.05. After partialling out for age, the association became non-significant for the SENSITIVITY correlation (cf. Humes, Citation2007; Humes et al, Citation2013), but the TEMPORAL FINE STRUCTURE correlation with COGNITION remained significant (see ). The finding of a significant association between SENSITIVITY and COGNITION is in line with previous studies (Lin et al, Citation2011; Deal et al, Citation2015; Harrison Bush et al, Citation2015), but the relatively weak correlation that vanishes when age is partialled out seems to give partial support only to the fifth prediction. Nevertheless, as already stated we have employed a conservative test of the prediction in the sense that the majority of studies have typically targeted one cognitive function or memory system at the time instead of using a composite COGNITION score, as is the case in the current study (e.g. Tun et al, 2009; Rönnberg et al, 2011; Rönnberg et al, Citation2014; Verhaegen, 2014).
Also, as most of the components of the current COGNITION factor are related to the on-line processing of speech (especially the Executive/Inference-making and WMC components), we should expect smaller or non-existent effects relative to other memory systems such as episodic LTM, which is known to be more sensitive to hearing loss (Rönnberg et al, 2011; Rönnberg et al, Citation2014; Leverton, Citation2015). It may also be the case that episodic memory loss, not WMC, is coupled to the heightened risk of developing Alzheimer’s dementia (Lin et al, Citation2011, 2014; Rönnberg et al, Citation2014; Leverton, Citation2015). However, as the present study did not take a memory systems approach to cognition, and as episodic LTM indices were not employed, we can only partially evaluate this proposition. Additionally, the current sample (at this point in time) did not show very much variability on the MMSE, and hence, we expect that later data collections (when SENSITIVITY and MMSE scores are lower), and when we have included episodic LTM indices, will show greater impact on the COGNITION factor.
The fact that both the SENSITIVITY and the TEMPORAL FINE STRUCTURE factors both predict the NO CONTEXT outcome factor is very much in line with the results reported by Bernstein et al (Citation2013), where both factors account for variance independently. SENSITIVITY and TEMPORAL FINE STRUCTURE also significantly influence the CONTEXT outcome factor, albeit to a lesser extent than they influence the NO CONTEXT outcome factor.
Finally, to get a more general handle on how the factors relate to each other and how they collectively predict outcomes, we computed two regression analyses (one for each OUTCOME LEVEL 2 factor). This approach is in many ways similar to the approach taken by Humes et al (Citation1994) in their classic paper, where they used auditory (e.g. thresholds, suprathreshold discrimination tests), cognitive (i.e. Wechsler Adult Intelligence Scale-Revised measure) and speech perception measures, e.g. ranging from recognition of nonsense syllables to final words in sentences, combined with noise-no noise conditions. They also factor analyzed their battery and did subsequent prediction analyses. However, we have used other kinds of tests in each of the three categories, which might explain why thresholds (i.e. SENSITIVITY) dominated as a predictor construct in the Humes et al (Citation1994) study, while we have reached a somewhat different conclusion.
We used stepwise linear regression with backward elimination to predict the CONTEXT and NO CONTEXT outcomes from age, COGNITION, SENSITIVITY and TEMPORAL FINE STRUCTURE. CONTEXT was significantly predicted by COGNITION (β = 0.12, t(195) = 1.69, p = 0.09), SENSITIVITY (β = 0.18, t(195) = 2.25, p = 0.02) and TEMPORAL FINE STRUCTURE (β = 0.14, t(195) = 1.83, p = 0.07), R2 = 0.10, F(3, 196) = 7.33, p < 0.001. Age was not a significant predictor (p = 0.39). Note that common practice with a backward elimination strategy is to view predictors as significant when p < 0.10. The NO CONTEXT was significantly predicted by COGNITION (β = 0.26, t(195) = 4.18, p < 0.001), SENSITIVITY (β = 0.31, t(195) = 5.23, p < 0.001), TEMPORAL FINE STRUCTURE (β = 0.21, t(195) = 3.26, p = 0.001), and age (β = −0.15, t(195) = −2.43, p = 0.02), R2 = 0.40, F(4, 196) = 31.73, p < 0.001.
Thus, we obtain a robust prediction of variance especially for the NO CONTEXT outcome factor, where all three LEVEL 2 factors contribute independently with roughly equal beta weights in the equation, i.e., the HEARING factors and COGNITION contribute to performance. This is different from the Humes et al (Citation1994) study, one possible reason being that the COGNITION factor was motivated in the on-line ELU-context and not from a general intelligence test. Nevertheless, SENSITIVITY – similar to the Humes study – is an important general predictor variable for both of our outcome factors as well.
Conclusions
Taken together, the present overall and introductory study of the n200 database generally shows that LEVEL 1 and LEVEL 2 factorial structures are clear-cut, and replicate findings observed in previous studies that have been based on much smaller samples. The results indicate theoretically and clinically challenging LEVEL 2 inter-correlations.
First prediction: The fact that all cognitive tests significantly load on one factorial structure (i.e. COGNITION) gives general support for the unity, integration and interaction among the subcomponents (especially executive and WM functions) of the ELU-system.
Second prediction was also confirmed in the sense that we obtained two-factor solutions for both the HEARING and OUTCOME LEVEL 2 scores.
Third prediction: we demonstrated that the COGNITION factor predicts the NO CONTEXT outcome scores more than it predicts the CONTEXT outcome scores and that for the NO CONTEXT outcome, all three LEVEL 2 factors independently predicted the NO CONTEXT factor more than they predicted the CONTEXT factor and the difference was similar for all three factors.
Fourth prediction: TEMPORAL FINE STRUCTURE plays an interesting role relative to SENSITIVITY, COGNITION and to both OUTCOME factors and was given a cognitive hearing science interpretation in terms of WM (Stenfelt & Rönnberg, Citation2009). Recent brain-based findings pertinent to cognitive modulation of both subcortical and cortical levels of processing in the auditory system were discussed, and in line with a new early filter model (Marsh & Campbell, Citation2016).
Fifth prediction: SENSITIVITY was weakly correlated to COGNITION. Possible reasons for the weakness of the relation were discussed and it was suggested that the most likely explanation was that we did not use episodic memory tests during this test occasion.
Future studies using data from the n200 database will focus on the more detailed relationships between the subcomponents of the LEVEL 2 factors and their specific test contributions.
Supplementary material available online
tija-2016-03-0082-File005.docx
Download MS Word (53.4 KB)Acknowledgements
Helena Torlofson and Tomas Bjuvmar are thanked for their help with data collection. The study was approved by the regional ethics committee (Dnr: 55-09 T122-09).
The n200 data base can be accessed by requesting variables from the first author and after having signed a Data Transfer Agreement, where Rönnberg (PI), Danielsson and Stenfelt are the main responsible researchers.
Declaration of interest
The research was supported by a Linnaeus Centre HEAD excellence center grant (349-2007-8654) from the Swedish Research Council and by a program grant from FORTE (2012-1693), awarded to the first author.
References
- Acoustics – Audiometric test methods. Part 1 – Basic pure tone air and bone conduction threshold audiometry.
- Akeroyd, M.A. 2008. Are individual differences in speech reception related to individual differences in cognitive ability? A survey of twenty experimental studies with normal and hearing-impaired adults. Int J Audiol, 47, S53–S71.
- Albers, M.W., Gilmore, G.C., Kaye, J., Murphy, C., Wingfield, A., et al. 2015. At the interface of sensory and motor dysfunctions and Alzheimer’s disease. Alzheimers Dement J Alzheimers Assoc, 11, 70–98.
- Amieva, H., Ouvrard, C., Giulioli, C., Meillon, C., Rullier, L., et al. 2015. Self-reported hearing loss, hearing aids, and cognitive decline in elderly adults: A 25-year study. J Am Geriatr Soc, 63, 2099–2104.
- Anderson, S., White-Schwoch, T., Parbery-Clark, A. & Kraus, N. 2013. A dynamic auditory-cognitive system supports speech-in-noise perception in older adults. Hear Res, 300, 18–32.
- Andersson, U., Lyxell, B., Rönnberg, J., & Spens, K-E. 2001. Cognitive predictors of visual speech understanding. J Deaf Studies Deaf Ed, 6, 103–115.
- Arehart, K.H., Souza, P., Baca, R. & Kates, J.M. 2013. Working memory, age, and hearing loss: Susceptibility to hearing aid distortion. Ear Hear, 34, 251–260.
- Arlinger, S., Billermark, E., Oberg, M., Lunner, T. & Hellgren, J. 1998. Clinical trial of a digital hearing aid. Scand Audiol, 27, 51–61.
- Arlinger, S., Lunner, T., Lyxell, B. & Pichora-Fuller, M.K. 2009. The emergence of cognitive hearing science. Scand J Psychol, 50, 371–384.
- Baddeley, A. 2012. Working memory: Theories, models, and controversies. Annu Rev Psychol, 63, 1–29.
- Baddeley, A., Logie, R., Nimmosmith, I. & Brereton, N. 1985. Components of fluent reading. J Mem Lang, 24, 119–131.
- Barascud, N., Pearce, M.T., Griffiths, T.D., Friston, K.J. & Chait, M. 2016. Brain responses in humans reveal ideal observer-like sensitivity to complex acoustic patterns. Proc Natl Acad Sci, 113, E616–E625.
- Bernstein, J.G.W., Mehraei, G., Shamma, S., Gallun, F.J., Theodoroff, S.M., et al. 2013. Spectrotemporal modulation sensitivity as a predictor of speech intelligibility for hearing-impaired listeners. J Am Acad Audiol, 24, 293–306.
- Bernstein, J.G.W., Danielsson, H., Hällgren, M., Stenfelt, S., Rönnberg, J. and Lunner, T. (2016). Spectrotemporal modulation sensitivity as a predictor of speech-reception performance in noise with hearing aids. Trends Hear. (in press)
- Besser, J., Koelewijn, T., Zekveld, A.A., Kramer, S.E. & Festen, J.M. 2013. How linguistic closure and verbal working memory relate to speech recognition in noise – a review. Trends Amplif, 17, 75–93.
- Besser, J., Zekveld, A.A., Kramer, S.E., Rönnberg, J. & Festen, J.M. 2012. New measures of masked text recognition in relation to speech-in-noise perception and their associations with age and cognitive abilities. J Speech Lang Hear Res, 55, 194–209.
- Brand, T. 2000. Analysis and Optimization of Psychophysical Procedures in Audiology Bibliotheks- und Informationssystem., Oldenburg.
- Brungart, D.S., Chang, P.S., Simpson, B.D. & Wang, D. 2006. Isolating the energetic component of speech-on-speech masking with ideal time–frequency segregation. J Acoust Soc Am, 120, 4007–4018.
- Classon, E., Rudner, M. & Rönnberg, J. 2013. Working memory compensates for hearing related phonological processing deficit. J Commun Disord, 46, 17–29.
- Daneman, M. & Carpenter, P.A. 1980. Individual differences in working memory and reading. J Verbal Learn Verbal Behav, 19, 450–466.
- Danielsson, H., Zottarel, V., Palmqvist, L. & Lanfranchi, S. 2015. The effectiveness of working memory training with individuals with intellectual disabilities – a meta-analytic review. Front Psychol, 6, 1230. [Epub ahead of print]. doi:10.3389/fpsyg.2015.01230
- Deal, J.A., Sharrett, A.R., Albert, M.S., Coresh, J., Mosley, T.H., et al. 2015. Hearing impairment and cognitive decline: A pilot study conducted within the atherosclerosis risk in communities neurocognitive study. Am J Epidemiol, 181, 680–690.
- DeCaro, R., Peelle, J.E., Grossman, M. & Wingfield, A. 2016. The two sides of sensory–cognitive interactions: Effects of age, hearing acuity, and working memory span on sentence comprehension. Front Psychol, 7, 236. [Epub ahead of print]. doi: 10.3389/fpsyg.2016.00236
- Diamond, A. 2013. Executive functions. Ann Rev Psychol, 64, 135–168.
- Dupuis, K., Pichora-Fuller, M.K., Chasteen, A.L., Marchuk, V., Singh, G., et al. 2015. Effects of hearing and vision impairments on the Montreal Cognitive Assessment. Neuropsychol Dev Cogn B Aging Neuropsychol Cogn, 22, 413–437.
- Folstein, M.F., Folstein, S.E. & McHugh, P.R. 1975. “Mini-mental state”. A practical method for grading the cognitive state of patients for the clinician. J Psychiatr Res, 12, 189–198.
- Folstein, M.F., Folstein, S.E., McHugh, P.R. & Fanjiang, G. 2001. Mini-mental State Examination user’s guide. Odessa, FL: Psychological Assessment Resources. Odessa FL Psychol. Assess. Resour.
- Foo, C., Rudner, M., Rönnberg, J. & Lunner, T. 2007. Recognition of speech in noise with new hearing instrument compression release settings requires explicit cognitive storage and processing capacity. J Am Acad Audiol, 18, 618–631.
- Füllgrabe, C., Moore, B.C.J. & Stone, M.A. 2015. Age-group differences in speech identification despite matched audiometrically normal hearing: Contributions from auditory temporal processing and cognition. Front Aging Neurosci, 6, 347. [Epub ahead of print]. doi: 10.3389/fnagi.2014.00347
- Gatehouse, S., Naylor, G. & Elberling, C. 2006. Linear and nonlinear hearing aid fittings – 2. Patterns of candidature. Int J Audiol, 45, 153–171.
- Gatehouse, S. & Noble, W. 2004. The speech, spatial and qualities of hearing scale (SSQ). Int J Audiol, 43, 85–99.
- Gates, G.A., Anderson, M.L., McCurry, S.M., Feeney, M.P. & Larson, E.B. 2011. Central auditory dysfunction as a harbinger of Alzheimer dementia. Arch Otolaryngol Head Neck Surg, 137, 390–395.
- Gates, G.A., Beiser, A., Rees, T.S., D’Agostino, R.B. & Wolf, P.A. 2002. Central auditory dysfunction may precede the onset of clinical dementia in people with probable Alzheimer’s disease. J Am Geriatr Soc, 50, 482–488.
- Gates, G.A., Gibbons, L.E., McCurry, S.M., McCusrry, S.M., Crane, P.K., et al. 2010. Executive dysfunction and presbycusis in older persons with and without memory loss and dementia. Cogn Behav Neurol Off J Soc Behav Cogn Neurol, 23, 218–223.
- Grosjean, F. 1980. Spoken word recognition processes and the gating paradigm. Percept Psychophys, 28, 267–283.
- Hagerman, B. 1982. Sentences for testing speech intelligibility in noise. Scand Audiol, 11, 79–87.
- Hagerman, B. & Kinnefors, C. 1995. Efficient adaptive methods for measuring speech reception threshold in quiet and in noise. Scand Audiol, 24, 71–77.
- Hällgren, M., Larsby, B. & Arlinger, S. 2006. A Swedish version of the Hearing In Noise Test (HINT) for measurement of speech recognition. Int J Audiol, 45, 227–237.
- Hällgren, M., Larsby, B., Lyxell, B. & Arlinger, S. 2005. Speech understanding in quiet and noise, with and without hearing aids. Int J Audiol, 44, 574–583.
- Hannon, B. & Daneman, M. 2001. Susceptibility to semantic illusions: An individual-differences perspective. Mem Cognit, 29, 449–461.
- Harrison Bush, A.L., Lister, J.J., Lin, F.R., Betz, J. & Edwards, J.D. 2015. Peripheral hearing and cognition: Evidence from the Staying Keen in Later Life (SKILL) study. Ear Hear, 36, 395–407.
- Hassanpour, M.S., Eggebrecht, A.T., Culver, J.P. & Peelle, J.E. 2015. Mapping cortical responses to speech using high-density diffuse optical tomography. NeuroImage, 117, 319–326.
- Heinrich, A., Henshaw, H. & Ferguson, M.A. 2015. The relationship of speech intelligibility with hearing sensitivity, cognition, and perceived hearing difficulties varies for different speech perception tests. Front. Psychol, 6, 782. [Epub ahead of print]. doi: 10.3389/fpsyg.2015.00782
- Hopkins, K. & Moore, B.C.J. 2010. Development of a fast method for measuring sensitivity to temporal fine structure information at low frequencies. Int J Audiol, 49, 940–946.
- Hua, H., Emilsson, M., Ellis, R., Widén, S., Möller, C., et al. 2014. Cognitive skills and the effect of noise on perceived effort in employees with aided hearing impairment and normal hearing. Noise Health, 16, 79–88.
- Huettig, F. & Janse, E. 2016. Individual differences in working memory and processing speed predict anticipatory spoken language processing in the visual world. Lang Cogn Neurosci, 31, 80–93.
- Humes, L. E. 2007. The contributions of audibility and cognitive factors to the benefit provided by amplified speech to older adults. J Am Acad Audiol, 18, 590–603. http://doi.org/10.3766/jaaa.18.7.6
- Humes, L.E., Busey, T.A., Craig, J. & Kewley-Port, D. 2013. Are age-related changes in cognitive function driven by age-related changes in sensory processing? Atten Percept Psychophys, 75, 508–524.
- Humes, L. E., Watson, B. U., Christensen, L. A., Cokely, C. G., Halling, D. C. & Lee, L. 1994. Factors associated with individual differences in clinical measures of speech recognition among the elderly. J Speech Lang Hear Res, 37, 465–474.
- Hunt, E. 1985. Verbal ability. In R. J. Sternberg (Ed.), Human abilities: An information processing approach (pp. 31–58). New York: Freeman and Company.
- Idrizbegovic, E., Hederstierna, C., Dahlquist, M., Kämpfe Nordström, C., Jelic, V., et al. 2011. Central auditory function in early Alzheimer’s disease and in mild cognitive impairment. Age Ageing, 40, 249–254.
- Keidser, G., Best, V., Freeston, K. & Boyce, A. 2015. Cognitive spare capacity: Evaluation data and its association with comprehension of dynamic conversations. Front Psychol, 6:597. [Epub ahead of print]. doi: 10.3389/fpsyg.2015.00597
- Kiessling, J., Pichora-Fuller, M.K., Gatehouse, S., Stephens, D., Arlinger, S., et al. 2003. Candidature for and delivery of audiological services: Special needs of older people. Int J Audiol, 42, 2S92–101.
- Kilman, L., Zekveld, A.A., Hällgren, M. & Rönnberg, J. 2015. Native and non-native speech perception by hearing-impaired listeners in noise-and speech maskers. Trends Hear, 19, 1–12. doi: 10.1177/2331216515579127
- Kramer, S.E., Zekveld, A.A. & Houtgast, T. 2009. Measuring cognitive factors in speech comprehension: The value of using the Text Reception Threshold test as a visual equivalent of the SRT test. Scand J Psychol, 50, 507–515.
- Kraus, N. & White-Schwoch, T. 2015. Unraveling the biology of auditory learning: A cognitive-sensorimotor-reward framework. Trends Cogn Sci, 19, 642–654.
- Lazard, D. S., Lee, H. J., Gaebler, M., Kell, C. A., Truy, E., & Giraud, A. L. 2010. Phonological processing in postlingual deafness and cochlear implant outcome. Neuroimage, 49, 3443–3451.
- Leverton, T. 2015. Hearing loss is important in dementia. BMJ, 351:h3650.
- Lidestam, B. 2009. Visual discrimination of vowel duration. Scand J Psychol, 50, 427–435.
- Li, Y. & Wang, D. 2009. On the optimality of ideal binary time-frequency masks. Speech Commun, 51, 230–239.
- Lunner, T. 2003. Cognitive function in relation to hearing aid use. Int J Audiol, 42, S49–S58.
- Lin, F.R., Thorpe, R., Gordon-Salant, S. & Ferrucci, L. 2011. Hearing loss prevalence and risk factors among older adults in the United States. J Gerontol A Biol Sci Med Sci, 66, 582–590.
- Lin, F.R., Yaffe, K., Xia, J., Xue, Q.-L., Harris, T.B., et al. 2013. Hearing loss and cognitive decline in older adults. JAMA Intern Med, 173, 293–299.
- Logan, G.D. 1994. On the ability to inhibit thought and action: A users’ guide to the stop signal paradigm. In: Dagenbach, D. & Carr, T.H. (eds.) Inhibitory Processes in Attention, Memory, and Language. San Diego, CA, US: Academic Press, p. 189–239.
- Luce, P.A. & Pisoni, D.B. 1998. Recognizing spoken words: The neighborhood activation model. Ear Hear, 19, 1–36.
- Lunner, T. 2003. Cognitive function in relation to hearing aid use. Int J Audiol, 42, S49–S58.
- Lunner, T., Hietkamp, R.K., Andersen, M.R., Hopkins, K. & Moore, B.C.J. 2012. Effect of speech material on the benefit of temporal fine structure information in speech for young normal-hearing and older hearing-impaired participants. Ear Hear, 33, 377–388.
- Lunner, T., Rudner, M. & Rönnberg, J. 2009. Cognition and hearing aids. Scand J Psychol, 50, 395–403.
- Lunner, T. & Sundewall-Thorén, E. 2007. Interactions between cognition, compression, and listening conditions: Effects on speech-in-noise performance in a two-channel hearing aid. J Am Acad Audiol, 18, 604–617.
- Lyxell, B., Andersson, J., Andersson, U., Arlinger, S., Bredberg, G., et al. 1998. Phonological representation and speech understanding with cochlear implants in deafened adults. Scand J Psychol, 39, 175–179.
- Lyxell, B., Andersson, J., Arlinger, S., Bredberg, G., Harder, H., et al. 1996. Verbal information-processing capabilities and cochlear implants: Implications for preoperative predictors of speech understanding. J Deaf Stud Deaf Educ, 1, 190–201.
- Lyxell, B. & Rönnberg, J. 1987. Guessing and speechreading. Br J Audiol, 21, 13–20.
- Lyxell, B. & Rönnberg, J. 1989. Information-processing skill and speech-reading. Br J Audiol, 23, 339–347.
- Lyxell, B., Rönnberg, J. & Samuelsson, S. 1994. Internal speech functioning and speechreading in deafened and normal hearing adults. Scand Audiol, 23, 179–185.
- Magnusson, L. 1995. Reliable clinical determination of speech recognition scores using Swedish PB words in speech-weighted noise. Scand Audiol, 24, 217–223.
- Majerus, S., & Van der Linden, M. 2003. Long-term memory effects on verbal short-term memory: A replication study. Br J Dev Psychol, 21, 303–310.
- Maki, W.S. 2007. Judgments of associative memory. Cognit Psychol, 54, 319–353.
- Marsh, J.E. & Campbell, T.A. 2016. Processing complex sounds passing through the rostral brainstem: The new early filter model. Front. Neurosci. doi: 10.3389/fnins.2016.00136.
- Mattys, S.L., Davis, M.H., Bradlow, A.R. & Scott, S.K. 2012. Speech recognition in adverse conditions: A review. Lang Cogn Process, 27, 953–978.
- Mehraei, G., Gallun, F. J., Leek, M. R. & Bernstein, J. G. W. 2014. Spectrotemporal modulation sensitivity for hearing-impaired listeners: Dependence on carrier center frequency and the relationship to speech intelligibility. J Acoust Soc Am, 136, 301–316. http://doi.org/10.1121/1.4881918
- Meister, H., Schreitmüller, S., Ortmann, M., Rählmann, S. & Walger, M. 2016. Effects of hearing loss and cognitive load on speech recognition with competing talkers. Front Psychol, 7, 301. [Epub head of print]. doi:10.3389/fpsyg.2016.00301
- Mishra, S., Lunner, T., Stenfelt, S., Rönnberg, J. & Rudner, M. 2013. Seeing the talker’s face supports executive processing of speech in steady state noise. Front Syst Neurosci, 7, 96. [Epub head of print]. doi:10.3389/fnsys.2013.00096
- Mishra, S., Stenfelt, S., Lunner, T., Rönnberg, J. & Rudner, M. 2014. Cognitive spare capacity in older adults with hearing loss. Front Aging Neurosci, 6, 96. [Epub head of print]. doi:10.3389/fnagi.2014.00096.
- Miyake, A., Friedman, N.P., Emerson, M.J., Witzki, A.H., Howerter, A., et al. 2000. The unity and diversity of executive functions and their contributions to complex “Frontal Lobe” tasks: A latent variable analysis. Cognit Psychol, 41, 49–100.
- Miyake, A. & Shah, P. 1999. Models of Working Memory: Mechanisms of Active Maintenance and Executive Control. Cambridge: Cambridge University Press.
- Moore, B.C.J. 2008. The role of temporal fine structure processing in pitch perception, masking, and speech perception for normal-hearing and hearing-impaired people. J Assoc Res Otolaryngol JARO, 9, 399–406.
- Moore, B.C.J., Glasberg, B.R. & Stone, M.A. 2004. New version of the TEN test with calibrations in dB HL. Ear Hear, 25, 478–487.
- Moore, B.C.J., Huss, M., Vickers, D.A., Glasberg, B.R. & Alcántara, J.I. 2000. A test for the diagnosis of dead regions in the cochlea. Br J Audiol, 34, 205–224.
- Moradi, S., Lidestam, B. & Rönnberg, J. 2013. Gated audiovisual speech identification in silence vs. noise: Effects on time and accuracy. Front Psychol, 4, 359. [Epub head of print]. doi:10.3389/fpsyg.2013.00359
- Moradi, S., Lidestam, B., Saremi, A. & Rönnberg, J. 2014a. Gated auditory speech perception: Effects of listening conditions and cognitive capacity. Front Psychol, 5, 531. [Epub head of print]. doi:10.3389/fpsyg.2014.00531
- Moradi, S., Lidestam, B., Hällgren, M. & Rönnberg, J. 2014b. Gated auditory speech perception in elderly hearing aid users and elderly normal-hearing Individuals: Effects of hearing impairment and cognitive capacity. Trends Hear, 18, 1–12. doi: 10.1177/2331216514545406
- Moradi, S., Lidestam, B. & Rönnberg, J. (2016). Comparison of gated audiovisual speech identification in elderly hearing aid users and elderly normal-hearing individuals: Effects of adding visual cues to auditory speech stimuli. Trends in Hearing. doi:10.1177/2331216516654022.
- Morris, N. & Jones, D.M. 1990. Memory updating in working memory: The role of the central executive. Br J Psychol, 81, 111–121.
- Munhall, K.G. & Tokhura, Y. 1998. Audiovisual gating and the time course of speech perception. Journal of the Acoustical Society of America, 104, 530–539.
- Neely, S.T., Gorga, M.P. & Dorn, P.A. 2003. Cochlear compression estimates from measurements of distortion-product otoacoustic emissions. J Acoust Soc Am, 114, 1499–1507.
- Neher, T., Lunner, T., Hopkins, K. & Moore, B.C.J. 2012. Binaural temporal fine structure sensitivity, cognitive function, and spatial speech recognition of hearing-impaired listeners (L). J Acoust Soc Am, 131, 2561–2564.
- Ng, E.H.N., Classon, E., Larsby, B., Arlinger, S., Lunner, T., Rudner, M. & Rönnberg, J. 2014. Dynamic relation between working memory capacity and speech recognition in noise during the first six months of hearing aid use. Trends Hear, 18. [Epub head of print]. doi:10.1177/2331216514558688.
- Ng, E.H.N., Rudner, M., Lunner, T., Pedersen, M.S. & Rönnberg, J. 2013b. Effects of noise and working memory capacity on memory processing of speech for hearing-aid users. Int J Audiol, 52, 433–441. http://doi.org/10.3109/14992027.2013.776181
- Ng, E.H.N., Rudner, M., Lunner, T. & Rönnberg, J. 2013a. Relationships between self-report and cognitive measures of hearing aid outcome. Speech Lang Hear, 16, 197–207.
- Ng, E.H.N., Rudner, M., Lunner, T. & Rönnberg, J. 2015. Noise reduction improves memory for target language speech in competing native but not foreign language speech. Ear Hear, 36, 82–91.
- Nielsen, N.P., & Wiig, E.H. 2011. AQT cognitive speed and processing efficiency differentiate adults with and without ADHD: A preliminary study. Int J Psychiatry Clin Pract, 15, 219–227.
- Nilsson, L.-G., Bäckman, L., Erngrund, K., Nyberg, L., Adolfsson, R., et al. 1997. The betula prospective cohort study: Memory, health, and aging. Aging Neuropsychol Cogn, 4, 1–32.
- Nilsson, M., Soli, S.D. & Sullivan, J.A. 1994. Development of the Hearing In Noise Test for the measurement of speech reception thresholds in quiet and in noise. J Acoust Soc Am, 95, 1085–1099.
- Noble, W. & Gatehouse, S. 2006. Effects of bilateral versus unilateral hearing aid fitting on abilities measured by the Speech, Spatial, and Qualities of Hearing Scale (SSQ). Int J Audiol, 45, 172–181.
- Olsson, H. & Poom, L. 2005. Visual memory needs categories. Proc Natl Acad Sci U S A, 102, 8776–8780.
- Palmqvist, S., Minthon, L., Wattmo, C., Londos, E. & Hansson, O. 2010. A Quick Test of cognitive speed is sensitive in detecting early treatment response in Alzheimer’s disease. Alzheimers Res Ther, 2, 29.
- Perfetti, C. A. 1983. Individual differences in verbal processes. In R.F. Dillon & R.R. Sehmeck (Eds.), Individual differences in cognition (vol. 1) (pp. 65–104). New York: Academic Press.
- Pichora-Fuller, M.K. 2003. Cognitive aging and auditory information processing. Int J Audiol, 42, 2S26–32.
- Pichora-Fuller, M.K., Dupuis, K., Reed, M. & Lemke, U. 2013. Helping older people with cognitive decline communicate: Hearing aids as part of a broader rehabilitation approach. Semin Hear, 34, 308–330.
- Pichora-Fuller, M.K., Schneider, B.A., Macdonald, E., Pass, H.E. & Brown, S. 2007. Temporal jitter disrupts speech intelligibility: A simulation of auditory aging. Hear Res, 223, 114–121.
- Qin, M.K. & Oxenham, A.J. 2003. Effects of simulated cochlear-implant processing on speech reception in fluctuating maskers. J Acoust Soc Am, 114, 446–454.
- Raven, J.C. 1938. Progressive Matrices: A Perceptual Test of Intelligence. London 7 H.K. Lewis., Available at: https://www.researchgate.net/publication/239009606_Progressive_Matrices_A_Perceptual_Test_of_Intelligence [Accessed March 14, 2016].
- Raven, J. 2008. General introduction and overview: The Raven Progressive Matrices Tests: Their theoretical basis and measurement model. In: Raven, J. & Raven, J. (eds.) Uses and Abuses of Intelligence: Studies Advancing Spearman and Raven’s Quest for Non-Arbitrary Metrics. Unionville, New York: Royal Fireworks Press; Edinburgh, Scotland: Competency Motivation Project; Budapest, Hungary: EDGE 2000; Cluj Napoca, Romania: Romanian Psychological Testing Services SRL (Chapter 1, pp. 17–68).
- Richie, C. & Kewley-Port, D. 2008. The effects of auditory-visual vowel identification training on speech recognition under difficult listening conditions. J Speech Lang Hear Res, 51, 1607–1619.
- Rogers, R.D. & Monsell, S. 1995. Costs of a predictable switch between simple cognitive tasks. J Exp Psychol Gen, 124, 207–231.
- Rönnberg, J. 1990. Cognitive and communicative function: The effects of chronological age and “handicap age”. Eur J Cognit Psychol, 2, 253–273.
- Rönnberg, J. 2003. Cognition in the hearing impaired and deaf as a bridge between signal and dialogue: A framework and a model. Int J Audiol, 42, S68–S76.
- Rönnberg, J., Andersson, U., Lyxell, B. & Spens, K.-E. 2001. Cognitive predictors of visual speech understanding. J Deaf Stud Deaf Educ, 6, 103–115.
- Rönnberg, J., Arlinger, S., Lyxell, B. & Kinnefors, C. 1989. Visual evoked potentials: Relation to adult speechreading and cognitive function. J Speech Hear Res, 32, 725–735.
- Rönnberg, J., Danielsson, H., Rudner, M., Arlinger, S., Sternäng, O., et al. 2011a. Hearing loss is negatively related to episodic and semantic long-term memory but not to short-term memory. J Speech Lang Hear Res, 54, 705–726.
- Rönnberg, J., Hygge, S., Keidser, G. & Rudner, M. 2014. The effect of functional hearing loss and age on long-and short-term visuospatial memory: Evidence from the UK biobank resource. Front Aging Neurosci, 6, 326. [Epub head of print]. doi:10.3389/fnagi.2014.00326
- Rönnberg, J., Lunner, T., Zekveld, A.A., Sörqvist, P., Danielsson, H., et al. 2013. The Ease of Language Understanding (ELU) model: Theoretical, empirical, and clinical advances. Front Syst Neurosci, 7, 31. [Epub head of print]. doi:10.3389/fnsys.2013.00031
- Rönnberg, J., Rudner, M., Foo, C. & Lunner, T. 2008. Cognition counts: A working memory system for ease of language understanding (ELU). Int J Audiol, 47, S99–S105.
- Rönnberg, J., Rudner, M. & Lunner, T. 2011b. Cognitive hearing science: The legacy of Stuart Gatehouse. Trends Amplif, 15, 140–148. DOI: 10.1177/1084713811409762
- Rönnlund, M., Sundström, A. & Nilsson, L.-G. 2015. Interindividual differences in general cognitive ability from age 18 to age 65 years are extremely stable and strongly associated with working memory capacity. Intelligence, 53, 59–64.
- Rudner, M., Foo, C., Rönnberg, J. & Lunner, T. 2009. Cognition and aided speech recognition in noise: Specific role for cognitive factors following nine-week experience with adjusted compression settings in hearing aids. Scand J Psychol, 50, 405–418.
- Rudner, M. & Lunner, T. 2014. Cognitive spare capacity and speech communication: A narrative overview. BioMed Res Int, 2014, 869726.
- Rudner, M., Rönnberg, J. & Lunner, T. 2011. Working memory supports listening in noise for persons with hearing impairment. J Am Acad Audiol, 22, 156–167.
- Sahlén, B., Reuterskiöld-Wagner, C., Nettelbladt, U. & Radeborg, K. 1999. Non-word repetition in children with language impairment – Pitfalls and possibilities. Int J Lang Commun Disord R Coll Speech Lang Ther, 34, 337–352.
- Salthouse, T.A. 2000. Aging and measures of processing speed. Biol Psychol, 54, 35–54.
- Samuelsson, S. & Rönnberg, J. 1993. Implicit and explicit use of scripted constraints in lip-reading. Eur J Cogn Psychol, 5, 201–233.
- Schneider, B.A., Pichora-Fuller, K. & Daneman, M. 2010. Effects of senescent changes in audition and cognition on spoken language comprehension. In: Gordon-Salant, S., Frisina, R.D., Popper, A.N. & Fay, R.R. (eds.) The Aging Auditory System. New York: Springer Handbook of Auditory Research, pp. 167–210. Available at: http://link.springer.com/chapter/10.1007/978-1-4419-0993-0_7 [Accessed March 14, 2016].
- Schoof, T. & Rosen, S. 2014. The role of auditory and cognitive factors in understanding speech in noise by normal-hearing older listeners. Front Aging Neurosci, 6, 307. [Epub ahead of print]. doi:10.3389/fnagi.2014.00307
- Smith, S.L. & Pichora-Fuller, M.K. 2015. Associations between speech understanding and auditory and visual tests of verbal working memory: Effects of linguistic complexity, task, age, and hearing loss. Front Psychol, 6, 1394. [Epub ahead of print]. doi:10.3389/fpsyg.2015.01394
- Sörqvist, P., Dahlström, O., Karlsson, T. & Rönnberg, J. 2016. Concentration: The neural underpinnings of how cognitive load shields against distraction. Front Hum Neurosci.
- Sörqvist, P., Stenfelt, S. & Rönnberg, J. 2012. Working memory capacity and visual–verbal cognitive load modulate auditory-sensory gating in the brainstem: Toward a unified view of attention. J Cogn Neurosci, 24, 2147–2154.
- Souza P, Arehart K & Neher T. 2015. Working memory and hearing aid processing: Literature findings, future directions, and clinical applications. Front. Psychol. 6:1894. doi: 10.3389/fpsyg.2015.01894.
- Souza, P.E., Arehart, K.H., Shen, J., Anderson, M. & Kates, J.M. 2015a. Working memory and intelligibility of hearing-aid processed speech. Front Psychol, 6, 1894. [Epub ahead of print]. doi:10.3389/fpsyg.2015.01894
- Souza, P.E., Arehart, K. & Neher, T. 2015b. Working memory and hearing aid processing: Literature findings, future directions, and clinical applications. Front Psychol, 6, 1894. [Epub ahead of print]. doi:10.3389/fpsyg.2015.01894
- Souza, P.E. & Sirow, L. 2014. Relating working memory to compression parameters in clinically fit hearing AIDS. Am J Audiol, 23, 394–401.
- Stenfelt, S. & Rönnberg, J. 2009. The signal-cognition interface: Interactions between degraded auditory signals and cognitive processes. Scand J Psychol, 50, 385–393.
- Takahashi, G.A. & Bacon, S.P. 1992. Modulation detection, modulation masking, and speech understanding in noise in the elderly. J Speech Hear Res, 35, 1410–1421.
- Taljaard, D.S., Olaithe, M., Brennan-Jones, C.G., Eikelboom, R.H. & Bucks, R.S. 2016. The relationship between hearing impairment and cognitive function: A meta-analysis in adults. Clin Otolaryngol, p.n/a–n/a.
- Tun, P.A. & Wingfield, A. 1999. One voice too many: Adult age differences in language processing with different types of distracting sounds. J Gerontol B Psychol Sci Soc Sci, 54, 317–327.
- Vaughan, N., Storzbach, D. & Furukawa, I. 2008. Sequencing versus nonsequencing working memory in understanding of rapid speech by older listeners. J Am Acad Audiol, 17, 506–518.
- Verhaegen, C., Collette, F. & Majerus, S. 2014. The impact of aging and hearing status on verbal short-term memory. Neuropsychol Dev Cogn B Aging Neuropsychol Cogn, 21, 464–482.
- Waldhauser, G.T., Braun, V. & Hanslmayr, S. 2016. Episodic memory retrieval functionally relies on very rapid reactivation of sensory information. J Neurosci, 36, 251–260.
- Wang, D. 2008. Time-frequency masking for speech separation and its potential for hearing aid design. Trends Amplif, 12, 332–353.
- Wang, D., Kjems, U., Pedersen, M.S., Boldt, J.B. & Lunner, T. 2009. Speech intelligibility in background noise with ideal binary time-frequency masking. J Acoust Soc Am, 125, 2336–2347.
- Warkentin, S., Tsantali, E., Kiosseoglou, G., Minthon, L., Wiig, E.H., et al. 2005. The AQT© as a useful short screening test for dementia. Evidence from two European cultures. Alzheimers Dement J Alzheimers Assoc, 1, S80.
- Wendt, D., Kollmeier, B. & Brand, T. 2015. How hearing impairment affects sentence comprehension: Using eye fixations to investigate the duration of speech processing. Trends Hear, 19. http://doi.org/10.1177/2331216515584149
- Wiig, E. H. & Nielsen, N.P. 2012. A Quick test of cognitive speed for comparing processing speed to differentiate adult psychiatric referrals with and without Attention-Deficit/Hyperactivity Disorders. Prim Care Companion CNS Disord . doi:10.4088/PCC.11m01273.
- Wiig, E.H. & Al-Halees, Y. 2013. A quick test of cognitive speed: Preliminary screening criteria for Arabic-speaking adults, ages 40 to 80 years. Percept Mot Skills, 117, 615.
- Wiig, E.H., Annas, P., Basun, H., Andreasen, N., Lannfelt, L., et al. 2010. The stability of AQT processing speed, ADAS-Cog and MMSE during acetylcholinesterase inhibitor treatment in Alzheimer’s disease. Acta Neurol Scand, 121, 186–193.
- Yntema, D.B. 1963. Keeping track of several things at once. Hum Factors, 5, 7–17.
- Yonelinas, A.P. 2013. The hippocampus supports high-resolution binding in the service of perception, working memory and long-term memory. Behav Brain Res, 254, 34–44.
- Zekveld, A.A., Deijen, J.B., Goverts, S.T. & Kramer, S.E. 2007. The relationship between nonverbal cognitive functions and hearing loss. J Speech Lang Hear Res, 50, 74–82.
- Zekveld, A.A., Rudner, M., Johnsrude, I.S., Heslenfeld, D.J., & Rönnberg, J. 2012. Behavioral and fMRI evidence that cognitive ability modulates the effect of semantic context on speech intelligibility. Brain Lang, 122, 103–113.
- Zekveld, A.A., Rudner, M., Johnsrude, I.S. & Rönnberg, J. 2013. The effects of working memory capacity and semantic cues on the intelligibility of speech in noise. J Acoust Soc Am, 134, 2225–2234.