Abstract
Various theorists have argued for the importance of a developmental approach to studying typical development (Karmiloff-Smith, 1998; Lerner, 1996; Lerner & Hood, 1986; Masten & Cicchetti, 2010; Overton, 2014; Overton & Lerner, 2012, 2014), and there are reasons to believe that this issue is even more critical to the study of atypical development (Thomas, 2016; Thomas et al., 2009). In this article, we bring together perspectives from a variety of theorists to outline the importance of conceptualizing both typical and atypical development as a not-necessarily-linear, interconnected, and multifaceted set of individual trajectories embedded in real-world contexts (Karmiloff-Smith, 1998; Masten & Cicchetti, 2010; Overton & Lerner, 2012). Using examples from studies of atypical brain and behavior, we describe 6 lenses that can be applied to developmental research.
Developmental psychology is the study of change, and individual people may follow widely variable paths to reach similar developmental endpoints. Change can occur quickly or may emerge gradually over time. There may be a single incipient event such as a trauma, or a constellation of factors may interact to produce change. A developmental approach focuses on this process and seeks to model change by investigating interacting domains over time. This is particularly relevant to the study of atypical development, where small differences early in life can have cascading effects on later outcomes (Karmiloff-Smith, Citation1998; Masten & Cicchetti, Citation2010). It is important to note that although longitudinal studies are valuable to this approach, they do not always capture everything necessary to understand the process of development. In the following paragraphs, we describe six lenses that encompass what we (and others) mean by taking a “developmental” approach to studying change. We use examples from brain and behavioral research in children with autism spectrum disorder (ASD), Williams syndrome (WS), and Down syndrome (DS) to illustrate how data interpretation may differ substantially when this developmental perspective is embraced.
WHAT IS A ‘DEVELOPMENTAL APPROACH’?
Development is complicated, and theorists have debated for years about the best way to approach the study of change, starting with very early work from Zigler and colleagues debating how to conceptualize development in children with intellectual disabilities (Zigler, Citation1969; Zigler & Hodapp, Citation1986). Karmiloff-Smith, Lerner, Werner, Overton, and Cicchetti, in particular, have written extensively about longitudinal, multimodal, relational approaches to developmental research. For example, Karmiloff-Smith (Citation1998, Citation2012, Citation2013) argued that the developmental process itself plays a role in constructing cognition. This neuroconstructivist approach (Elman et al., Citation1996; Karmiloff-Smith, Citation1998) suggests that the infant brain starts out with domain-relevant constraints (e.g., neural mechanisms) that guide infants toward “progressive selection and processing of different kinds of input” (Karmiloff-Smith, Citation1998, p. 390). Throughout the course of development, these constraints lead to greater specificity of processing, which allows children to gradually develop more specialized cognitive systems. This approach is founded on ideas from Werner’s orthogenetic principle, which states that development “proceeds from a state of relative globality and lack of differentiation to a state of increasing differentiation, articulation, and hierarchic integration” (Werner, Citation1957, p. 126). A similar process, wherein small differences or constraints early in development have large downstream effects, is referred to as a “developmental cascade” (Masten & Cicchetti, Citation2010).
Lerner and Overton, as well as Hodapp and colleagues, have highlighted the importance of taking into account multiple levels of development across the life span: social, biological, and cultural (Ford & Lerner, Citation1992; Hodapp, Burack, & Zigler, Citation1990; Lerner, Citation1996; Overton & Lerner, Citation2012, Citation2014; also see Bronfenbrenner, Citation1979). Lerner emphasized “goodness of fit,” which refers to the extent to which an individual fits with their environment across developmental time. The developmental approach described in this article encourages researchers to assess how these multiple levels and systems interface (or align) at different junctures. Disrupted timing in one domain, like gross motor development, may lead to deficits in other areas, such as language (Estes et al., Citation2015). Cross-domain studies that include multiple time points are more likely to capture dynamic interplay between levels of influence, thus adding nuance to our measurement of individual change. Like Overton and Lerner (Citation2012, Citation2014), we argue for the importance of considering the many interleaving parts of the system and environmental inputs as they interact across time (Karmiloff-Smith, Citation1998, Citation2012, Citation2013; Lerner, Citation2006; Overton & Lerner, Citation2012; Paterson, Girelli, Butterworth, & Karmiloff-Smith, Citation2006).
With these theoretical underpinnings in mind, we will discuss how six developmental lenses can help us learn a great deal about typical development from children who are following atypical developmental trajectories (delayed or qualitatively different; Karmiloff-Smith, Citation1998; Thomas, Citation2016). While significant longitudinal research efforts have focused on typical development (e.g., Bornstein & Tamis-Lemonda, Hahn, & Haynes, Citation2010; Siegler & Crowley, Citation1991), often across many years (Bloom, Citation1970; Brown, Citation1973; Dromi, Citation1987; Hoff, Rumiche, Burridge, Ribot, & Welsh, Citation2014), fewer studies of children with neurodevelopmental disorders have benefitted from this comprehensive, developmental approach. We highlight some studies that have employed this method successfully and discuss the relevance and implications of these studies for typical development.
SIX CONSIDERATIONS WHEN TAKING A DEVELOPMENTAL APPROACH
We have identified six central lenses that provide perspective on studying developmental disorders, and we illustrate each with examples from work on neurobiology and cognition. These six lenses are:
Developmental processes, not just end products, are crucial.
A dimensional approach that considers both cognitive strengths and weaknesses can be revealing.
Developmental timing may be key.
Probing the same process using multiple methods affords a complex view.
Individual differences are important (mean trajectories tell only part of the story).
Development is often nonlinear.
1. Developmental Processes, Not Just End Products, Are Crucial
A clear strength of the developmental approach espoused by Karmiloff-Smith (Citation1998, Citation2013), Lerner (Citation1996), Werner (Citation1937), Zigler (Citation1969), and Overton (Citation2014; Overton & Lerner, Citation2012) is that it deemphasizes behavioral outcomes and instead focuses on the process of change itself. Outcomes are important, but charting developmental trajectories and focusing on processes can show us how and when certain individuals veer away from the expected path.
Brain imaging research on infant siblings of children with ASD provides excellent evidence for how a process-focused lens can benefit neurobiological research (Courchesne, Carper, & Akshoomoff, Citation2003; Wolff et al., Citation2012). Such “infant sibling” studies are especially important for understanding how autism emerges, because younger siblings of children with ASD are 5 times more likely to be diagnosed with ASD than the general population (Grønborg, Schendel, & Parner, Citation2013; Ozonoff et al., Citation2011). There has been a great deal of speculation about the role of brain size in ASD, and some data have suggested that toddlers who are later diagnosed with ASD have larger brains than those who are not (Hazlett et al., Citation2005, Citation2012; Schumann et al., Citation2010). However, it is less well understood why differences in brain size might relate to ASD and whether this difference is a cause or a consequence of the disorder. ASD’s heterogeneous etiology further complicates the picture, with brain variation likely stemming from multiple causes.
Wolff et al. (Citation2012) studied brain development in infants who had an older sibling diagnosed with ASD by investigating differences between the white matter tracts of those infants who later received a diagnosis of ASD and those who did not. White matter was assessed using fractional anisotropy, which measures how water moves through fibers in the brain, and integrity was measured using a special type of magnetic resonance imaging (MRI) called diffusion tensor imaging (DTI). Wolff et al. speculated that perhaps differences in brain development might underlie the emergence of ASD features in some infants. Using a longitudinal multisampling approach, Wolff and colleagues examined the process of change by scanning 92 infants at 6 months, 12 months, and 24 months of age. Toddlers were assessed for signs of ASD at 24 months by qualified clinicians and underwent a battery of behavioral tasks at each age point.
An interesting pattern emerged from this study, which required three time points to fully grasp. At 6 months, infants who went on to receive a diagnosis of ASD (N = 28) had higher white matter integrity than those who did not (N = 64), suggesting that their white matter development was relatively more advanced at 6 months. At 12 months, no group differences were evident in any fiber tracts. By 24 months, the 6-month-old pattern had reversed, and white matter integrity was lower in the ASD-positive group. Thus, although infants later diagnosed with ASD started off with advanced connectivity, their development slowed and they ended up with poorer white matter integrity than infants who were not later diagnosed with ASD. White matter organization differences were not due to general cognitive ability, as developmental level was included as a covariate in all statistical models. This effect is evident in the fiber tract of the superior cerebellar peduncle and is similar to that in several other fiber tracts discussed in Wolff et al. (Citation2012; ; Wolff, personal communication, May 2016). A trajectory of rapid early development followed by slowed growth has also been found in older children and adults with ASD (Cheng et al., Citation2010; Keller, Kana, & Just, Citation2007; Shukla, Keehn, & Müller, Citation2011) and provides us with clues about where to look for the mechanisms that might underlie this developmental anomaly (e.g., genes responsible for neuronal proliferation from birth to 6 months of age).
Figure 1 Trajectories of white matter integrity (measured by fractional anisotropy) in tracts of the superior cerebellar peduncle, which connects the cerebellum to the midbrain, in 92 infants with an older sibling who has been diagnosed with autism spectrum disorder (ASD; courtesy of Wolff, unpublished data).
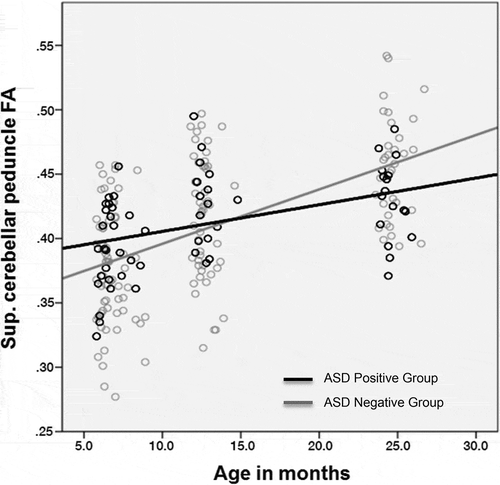
The biological mechanism underlying these findings is as yet unknown, but several candidates have been proposed. One possibility is that accelerated white matter tract organization could reflect earlier neuronal pruning and/or myelination in infants later diagnosed with ASD (Wolff et al., Citation2012). In early neuronal pruning, interneuron connections are pared away more quickly than they should be. Accelerated myelination, on the other hand, means that bundles of nerve fibers are insulated before they are able to connect to other fibers. At first blush, early development might sound like a good thing. However, in this case, it could mean that infants who later receive a diagnosis of ASD are less able to take advantage of experience-dependent learning opportunities that require flexible brains (Johnson, Citation2011). Neural systems that are organized too early in development could be less malleable (or plastic) and thus less able to change in response to stimulation (Wolff et al., Citation2012). Reduced plasticity may have a powerful impact on skills relying heavily on environmental input for their acquisition, such as early social and language skills (Meredith, Citation2015).
Differential patterns of growth in white matter integrity demonstrate the value of studying developmental trajectories and the process of change. If Wolff and colleagues (Citation2012) had only focused on data from 12-month-olds, they would have concluded that there were no brain differences in infants who go on to receive a diagnosis of ASD versus those who do not. Cross-sectional data at different time points (e.g., 6 or 24 months) may have shown differences between groups, but longitudinal data were necessary for understanding individual trajectories. By looking across areas of the brain and across individuals, Wolff and colleagues captured the richness of developmental change.
2. A Dimensional Approach That Considers Both Cognitive Strengths and Weaknesses Can Be Revealing
Assessing individual cognitive strengths and weaknesses provides a maximally nuanced view of change over time. The research domain criteria framework (RDoC) from the National Institutes of Health (NIH; Insel et al., Citation2010) provides a useful starting point for developmental psychologists (Casey, Oliveri, & Insel, Citation2014). Instead of focusing on categorical diagnoses such as attention-deficit hyperactivity disorder or autism, the RDoC approach examines differences in performance dimensionally (on a continuum) across disorders. This approach can be applied to different domains of functioning (e.g., cognition, arousal/self-regulation, social processing) and levels of analysis (e.g., genes, brain, behavior) in multiple disorders, offering insight into shared developmental mechanisms and pathways. For example, if two diagnostic groups struggle with (or excel at) the same cognitive tasks, do they also exhibit similar alternations in brain function? This approach has the potential to shed light on typical variation as well as outliers and highlights how important it is to measure these domains on a finely graded continuum (Casey et al., Citation2014; Thomas, Citation2016).
Cross-domain research is fundamental to the RDoC approach and may be especially important for understanding change in developmental disorders (Karmiloff-Smith, Citation1998, Citation2012, Citation2013; Paterson et al., Citation2006). For example, a detail-oriented approach to visual processing in persons with WS might lead to successful performance on an embedded figures task. This same approach might slow down that child’s construction of block patterns by interfering with their ability to see the pattern as a whole (Karmiloff-Smith, Brown, Grice, & Paterson, Citation2003). A different profile of strengths and weaknesses has been found in children with ASD, wherein block design performance is preserved despite the tendency toward a detail-oriented (or “local”) perceptual style (Wang, Mottron, Peng, Berthiaume, & Dawson, Citation2007). To explain this difference, some have argued that individuals with ASD might break block patterns into their constituent parts and solve the task at a local level without relying on global strategies to visualize the whole picture (Shah & Frith, Citation1993).
An RDoC-informed transdiagnostic study of children with ASD, WS, and DS suggests that prior research may have inadvertently oversimplified the issue of perceptual style in these three disorders (D’Souza, Booth, Connolly, Happé, & Karmiloff-Smith, Citation2015). Comparing a variety of tasks and stimuli across multiple domains (auditory-verbal and visuospatial), researchers found that children did not reliably fall into either the local or global processors category. Instead, depending on the type of stimulus and its complexity, children demonstrated local processing on some tasks and global processing on others (D’Souza et al., Citation2015). This finding, along with work on ASD from Plaisted, Swettenham, and Rees (Citation1999) and Mottron and colleagues (Mottron, Burack, Iarocci, Belleville, & Enns, Citation2003; Mottron, Burack, Stauder, & Robaey, Citation1999) has challenged ideas about diagnostic group differences, while espousing a nuanced and dimensional view of perceptual style in these diverse disorders. Cross-domain, cross-disorder approaches are especially useful for identifying constellations of challenges and abilities in atypical populations as well as for understanding the profiles of individuals who are developing typically. The RDoC movement has significant potential to enrich scientific inquiry by encouraging researchers to change their perspectives and ask novel, challenging research questions.
This approach also has implications for understanding how atypical development can inform typical development. Atypically developing individuals may exhibit performance on a measure that appears delayed, or their development may be qualitatively different and on a completely different trajectory from their typically developing counterparts (Thomas, Citation2016). The RDoC approach enables us to see very clearly when and how performance diverges across different domains and can provide very useful clues as to the mechanisms that underlie behavior. This fulcrum can pinpoint where something goes wrong but is also as likely to inform us about the mechanism that drives successful behavior.
3. Developmental Timing May Be Key
How the timing of events over development interacts across different domains of functioning has a significant impact on later behavioral outcomes (Estes et al., Citation2015; Karasik, Tamis-LeMonda, & Adolph, Citation2014; Winder, Wozniak, Parladé, & Iverson, Citation2013). In typically developing children, development within and across domains may be uneven but is ultimately coordinated. In contrast, atypical populations often experience unusual timing, which affects them in at least two ways: by altering environmental input and by misaligning growth across domains (Karmiloff-Smith, Citation1998).
First, when a child develops a particular skill more slowly or more quickly than usual, their proximal environment is altered and leads to atypical input. For example, parents provide more verbal responses to object-sharing bids by walking 13-month-olds than by crawling 13-month-olds (Karasik et al., Citation2014). Delayed walking might therefore impact the richness of language input children receive. Compounding effects of differential input, although not immediately evident, may emerge over time as part of a “developmental cascade” (Gottlieb, Citation1991; Masten & Cicchetti, Citation2010).
Second, timing delays in one domain, such as motor development, may lead to misalignment with other domains, such as language. Two domains that usually develop in tandem (and support one another) are no longer harmonized, leading to poorer outcomes in both (Elman, Citation1993; Lerner, Citation2006). In a study of 5- to 14-month-old infant siblings of children with ASD, for example, Winder et al. (Citation2013) found that the ordinarily tight relationship between rhythmic arm movements and the onset of babble was not observed. The authors suggest that vocal and motor systems typically develop in tandem, and when they do not, infant siblings of children with ASD fail to acquire the same repertoire of gestures and vocalizations as their peers. When the expected relationship between two developing systems is disrupted, leading to an atypical outcome, it allows us to establish timing markers for when certain critical processes must (or must not) interact for typical development to take place. These results can inform the study of typical development by providing guidance for when to measure interrelated systems in a longitudinal design.
Research by Estes and colleagues (Citation2015) further illustrates the effect of atypical developmental timing in the motor domain. Infants who later received a diagnosis of ASD demonstrated motor impairments at 6 months—well before they were formally diagnosed. These motor impairments preceded later deficits in cognitive and communicative skills (such as expressive language) at 12 months. Is it possible that motor impairments at 6 months might contribute to cascading deficits in cognitive and communicative skills? Estes et al. suggested that this might be the case and posited two explanations. First, they suggested that motor delays could reduce the number of gestures children produce, with fewer gestures leading to fewer responses from caregivers, thereby diminishing children’s overall communicative input. Rowe and Goldin-Meadow (Citation2009) found a similar link between gesture and language in typical development, such that children producing more gestures at 18 months had correspondingly larger vocabularies at 42 months. Second, Estes and colleagues suggested that if children are not mobile (as in the case of late crawlers with poor trunk control), they have fewer opportunities to interact with objects (Karasik et al., Citation2014). Fewer object interactions by late crawlers may lead to hearing fewer labels, thus reducing children’s language-learning opportunities (Estes et al., Citation2015). Thus, research from multiple groups suggests that timing may be an important variable to assess when studying atypical development.
4. Probing the Same Process Using Multiple Methods Affords a Complex View
The NIH suggests that researchers employ multiple methods in their work, which can reveal a more nuanced picture of highly complex processes and may lead to new findings that might otherwise have been missed. For example, Wolff et al. (Citation2012) used DTI to find fiber tract differences in the brains of 6-month-olds who went on to receive a diagnosis of ASD, while researchers using a different method, structural MRI, found differences in brain volume in the same children at a later time point (Hazlett et al., Citation2012). If researchers had only used structural MRI (and not DTI), they would not have captured this important difference between groups.
Similarly, in WS, children’s scores on explicit tests of face-processing skill (e.g., selecting a face that matches a target; Benton, Sivan, Hamsher, Varney, & Spreen, Citation1983) fall in the typical range (Grice et al., Citation2001; Karmiloff-Smith et al., Citation2003). However, face processing in this same group of children is atypical when assessed implicitly, via electrophysiological responses to pictures of faces. Karmiloff- Smith et al. (Citation2003) found that despite average performance on behavioral tests, children with WS did not demonstrate the typical electrophysiological effect seen when faces are turned upside down (diminished N170 amplitude).
A recent study of recognition memory in adults with ASD also demonstrates the importance of using multiple methods and reveals discrepancies between behavior and electrophysiological responses (Massand, Bowler, Mottron, Hosein, & Jemel, Citation2013). In this case, the behavior of adults with ASD was the same as that of typical adults (both were equally able to remember words), but their electrophysiological responses to old versus new words occurred in different brain areas and with different intensities. In the typically developing group, response differences to old versus new words were seen in anterior regions of the brain, whereas in the ASD group, they were seen in parietal and posterior regions.
Thus, a multimethod approach (e.g., behavior and physiology) can reveal variation that might otherwise be obscured. This variation informs us about the possible mechanisms that might underlie the behavior we are measuring. If atypically developing individuals exhibit brain activity in different brain areas than their typically developing peers, they may be using different strategies or mechanisms to reach the same behavioral outcome. Here, the developmental lens is not focused on building trajectories, but rather, it is focused on enhancing the richness of the data we have available.
5. Individual Differences Are Important (Mean Trajectories Tell Only Part of the Story)
Average group differences are meaningful, but it is especially important to capture individual trajectories of change when studying developmental disorders. Here, longitudinal methods can map unique developmental pathways, and statistical techniques such as latent-class growth curve modeling (McArdle & Epstein, Citation1987) or latent profile analysis (Cohan et al., Citation2008) can detect meaningful subgroups. For example, children with language delays can either catch up with their peers or continue to struggle; and these two groups follow different developmental pathways (Rice, Citation2004). Mapping individual trajectories can provide a powerful view into the emergence of a disorder and can identify specific areas of impairment (Karmiloff-Smith, Citation1998; Paterson et al., Citation2006).
Prospective studies of behavior in infant siblings of children with ASD highlight the importance of charting developmental profiles to track individual differences and demonstrate how divergent trajectories may elucidate different patterns of disorder onset (Estes et al., Citation2015; Landa, Gross, Stuart, & Faherty, Citation2013; Pzonoff, Heung, Byrd, Hansen, & Hertz-Piccotto, Citation2008; Ozonoff et al., Citation2010). For example, in a longitudinal study of 25 infant siblings of children with ASD and 25 infant siblings of typically developing children, Ozonoff et al. (Citation2010) found that 6-month-olds showed no differences in many social behaviors produced during the Mullen Scales of Early Learning assessment (Mullen, Citation1995). By 12 months, however, the groups differed on the number of directed vocalizations they produced and the number of times they looked at faces. By 18 months, the group of infants later classified as typically developing produced more social smiles. Differences between groups emerged gradually and unfolded throughout the course of developmental time. Ozonoff et al. (Citation2010) suggested that many of the atypicalities in social behavior were so subtle that parents found them hard to notice. Indeed, parents reported fewer incidences of regression (losing skills) at 36 months of age (assessed via a gold standard parent interview for ASD, the Autism Diagnostic Interview—Revised; Rutter, LeCouteur, & Lord, Citation2003) than expected based on clinical observations of the same children. In this case, granular longitudinal data allowed researchers to detect changes in behavior that, although small, nonetheless signaled emerging developmental difficulties that may have warranted early intervention. If examined cross-sectionally or retrospectively, these small changes may have been lost among bigger, more salient developmental shifts.
One complicating factor for developmental researchers is that individual children can follow vastly different trajectories on their way to the same diagnosis (Landa & Garrett-Mayer, Citation2006; Landa, Stuart, Gross, & Faherty, Citation2013; Ozonoff, Heung, Byrd, Hansen, & Hertz-Picciotto, Citation2008; Ozonoff et al., Citation2010). For example, Landa and colleagues (Citation2013) assessed cognitive, motor, and language development in 204 infants with an older sibling with ASD and 31 infants with a typically developing older sibling at six time points from 6 to 36 months of age. Infants were divided into two groups based on clinical impression of ASD at 14 months: early ASD (clinical impression of ASD before 14 months of age) and later ASD (clinical impression of ASD after 14 months of age). Landa et al. analyzed trajectories of growth in various domains (e.g., plateaus and declines in language and positive affect). The authors argued that individual trajectories might hint at the biological underpinnings of a disorder by pinpointing where development goes awry. In the case of early- and later-emerging ASD, they suggested that language, cognitive, and social differences emerging after 6 months of age might be caused by neural connectivity abnormalities occurring in the same temporal window (Müller et al., Citation2011) and that this might occur at different times for each individual (Landa et al., Citation2013). Cross-sectional data from multiple time points might show an average trajectory but do not permit this kind of rich growth curve analysis and examination of individual differences.
6. Development Is Often Nonlinear
Developing individuals may start out at the same point on a developmental trajectory (equipotentiality) and may even reach the same end point (equifinality) while traveling completely different routes, along different timelines, to get there. Charting individual trajectories through frequent sampling makes the heterogeneity of these routes explicit (Adolph, Robinson, Young, & Gill-Alvarez, Citation2008; Thomas et al., Citation2009) and has important implications for intervention. Landa et al. (Citation2013) found clear trajectory differences between groups of infants, especially after 14 months of age. Without multiple time points, it would be impossible to see receptive language plateaus in the early-ASD group, for example, or marked declines in positive affect in both the early- and later-ASD groups. Here, it is clear that developmental trajectories may be nonlinear—and information about nonlinearity may help hone the level and timing of language interventions for various subgroups. In this study, early- and late-diagnosed ASD groups started at a similar developmental level for receptive language, and at 36 months, their scores were also somewhat similar. Receptive language scores at 18 months were clearly different, however, as the early-ASD group plateaued from 14 to 18 months, and the late-ASD group continued to demonstrate growth. If similarity at the beginning and ending points of the trajectory had been used to plan treatment and a linear growth pattern had been projected for both groups, then the intervention would have been too challenging for the early-diagnosed group and not demanding enough for the late-diagnosed group. This example is from group data, but the same methods could be applied at the individual level. Compiling rich data about change over time could ultimately help us achieve the goal of personalized medicine.
BRINGING MULTIPLE LENSES TOGETHER
All six developmental lenses can be applied within a single line of research, as demonstrated by studies of numeracy development in toddlers and older children with WS and DS (Paterson, Brown, Gsodl, Johnson, & Karmiloff-Smith, Citation1999; Paterson et al., Citation2006). The studies described in this section highlight the importance of studying developmental processes rather than behavioral products, and because the conceptual underpinnings for acquiring number skills are different across developmental disorders, they demonstrate that similar-looking deficits might have distinct causes (Karmiloff-Smith et al., Citation2012; Paterson et al., Citation1999, Citation2006; van Herwegen, Ansari, Xu, & Karmiloff-Smith, Citation2008).
In typical development, two different systems are thought to underlie cognitive representations of number—a small exact number system based on object tracking and another system that supports larger approximate quantities (Feigenson, Dehaene, & Spelke, Citation2004). Comparative work with infants diagnosed with DS or WS has shown that infants with DS had difficulty with smaller number discrimination (two dots vs. three dots) when compared with typically developing infants but performed better in tasks with larger numbers. This pattern was reversed in the WS group (Karmiloff-Smith et al., Citation2012; Paterson et al., Citation1999, Citation2006; van Herwegen et al., Citation2008). Two possible mechanisms underlie this number discrimination behavior. It could be that domain-general processes, such as attention or an inability to track objects and compare them across arrays, cause infants with DS to have difficulty with the small-number task (see Karmiloff-Smith et al., Citation2012, for further discussion). This problem is not number-specific, but it nevertheless has an impact on numerical representation skills. For example, infants with DS may have difficulty switching between a two-dot array and a three-dot array, leading to trouble recognizing differences in one-to-one correspondence between arrays. The large-number task is not affected, however, because it does not rely on the same attentional processes, but rather on representations of approximate quantity, which are specific to the number domain.
Longitudinal work has revealed numeracy difficulties in the DS group that are more marked in infancy relative to individuals with WS, whereas individuals with WS have more difficulties than participants with DS in adulthood (Paterson et al., Citation2006). In older childhood and adulthood, for example, individuals with DS perform better than matched peers with WS on a battery of numerical cognition tasks, ranging from “What number comes before X?” questions to simple arithmetic problems. In experimental tasks, individuals with DS exhibit more robust magnitude representations than their peers with WS. In this case, extrapolating the infant profile from the adult profile might have led us to assume that infants with DS would be better at number processing than those with WS; however, longitudinal studies showed the opposite (Paterson et al., Citation2006). Furthermore, we would have missed the differing cognitive strengths and weaknesses exhibited by these infants when completing large- and small-number tasks. Taking an approach focused merely on one point in older childhood or adulthood would not have allowed us to fully understand the underlying processes used by each of these groups.
WHAT HAPPENS IF WE DO NOT TAKE A DEVELOPMENTAL APPROACH?
Most developmental researchers acknowledge that it is optimal to study longitudinal trajectories while incorporating multiple observations over time on a single individual and assessing patterns of growth and change. Longitudinal studies can chart nonlinear trajectories and can pinpoint where individuals’ development differs from the group, while capturing timing and individual differences. Although longitudinal studies are the goal, serious limitations in funding and feasibility often require researchers to begin by capturing multiple snapshots of development in cross-sectional research (e.g., comparing one group of 6-month-olds with a different group of 12-month-olds). Cross-sectional designs do not reveal what specific trajectory an individual has followed through development and consequently cannot characterize the developmental cascades that compound from very small differences early on to produce big effects in later behavior. Cross-sectional and single-point snapshot studies do have their utility, however, as they can help elucidate how development proceeds in a population on average and can help researchers identify critical start and stop points in longitudinal work. For example, focusing closely on one point is conducive to very detailed microanalyses of particular behaviors. If a small cross-sectional study reveals substantial variation among individuals in one developmental window (e.g., 9–12 months), it makes sense to focus on that time point in a follow-up longitudinal design. In this way, all research designs can contribute to the goal of high-quality developmental research.
DISCUSSION
Karmiloff-Smith, Werner, Lerner, and Overton argued that studying trajectories is essential for understanding developmental change (Karmiloff-Smith, Citation1998, Citation2013; Lerner, Citation1996; Overton, Citation2014; Overton & Lerner, Citation2012; Werner, Citation1937). Their theories are obviously relevant for research on typically developing children, but their approach is equally valuable to the study of developmental disorders. Most directly, this approach offers clinicians an opportunity to hone effective and personalized interventions through their knowledge of developmental change. Less recognized, however, is the possibility that a clearer picture of developmental trajectories in atypical populations may also offer insight into mechanisms that underlie typical development. Knowing when and how development veers from the normative path can provide clues about typical change.
Longitudinal research is not the only way to study development, but it may be optimal. Examining developmental patterns over time in the same individuals helps researchers avoid pitfalls like generalizing from a small number of snapshots taken from an average of different samples and sheds light on both the process of development and its product. In atypical development, differences in timing across trajectories might provide clues to mechanisms that underlie the emergence of a particular skill or mechanism (Wolff et al., Citation2012). Without longitudinal designs, these timing differences and their cascading influence on development would not be apparent. Points in development where atypical patterns begin to emerge are likely to be important fulcrums in the developing system—in typical and atypical children—and deserve further scrutiny. Data from trajectory-based research provides useful clues for scholars of both typical and atypical development alike.
On a practical level, documenting early atypicalities is extremely valuable for intervention design and planning. If clinicians knew the timing of trajectory deviations, they might be able to intervene early, while targeting underlying mechanisms. Charting trajectories may also reveal meaningful subgroups within disorders. A few distinct trajectories might cluster together, with individuals in these subgroups responding to different treatments, requiring different diagnoses, and sharing common genetic variants.
Challenges and Future Directions
The developmental approach described here does not come without challenges, both practical and theoretical. It requires sufficient resources to see the same children for multiple visits and the methodological skill to deal with missing data, which is inevitable in longitudinal research. It also requires sophisticated statistical models, such as latent profile analysis. Here, we can learn from our colleagues in other disciplines who have already successfully employed these methods in aging research (e.g., Hofer & Piccinin, Citation2010), developmental psychopathology (e.g., Cicchetti, Citation2013), and social psychology and education (e.g., Jackson & Allemand, Citation2014; Singer & Willett, Citation2003). As Lerner (Citation1996) noted, measuring change demands measures sensitive enough to detect change. Care must be taken when choosing tests and paradigms. In general, measures that capture process (such as infrared eye tracking or reaction-time tasks) are preferred when charting trajectories. They can be helpful for tapping into the same underlying constructs throughout the course of development, so that the same construct can be tracked from precursor to mature phenotype. Such marker tasks have proven very valuable in developmental research and work well as part of a multimodal battery (Johnson, Citation2005). Vigilance is required with respect to whether the constructs under consideration truly remain constant over developmental time. The number and timing of study observations is crucial; sparse observations may lead researchers to miss important differences in the shape of developmental change (Adolph, Robin Johnson, Young, & Gill-Alverez, Citation2008).
In summary, we have argued that a rich, developmental approach can be extremely informative when studying atypical populations. Moreover, we have illustrated that this approach can be carried out successfully with many different subgroups using a variety of paradigms. Finally, the virtues of the six strategies we discussed are not limited to the study of developmental disorders but can also help us gain purchase on the processes involved in typical development.
ACKNOWLEDGMENTS
We thank Jason Wolff for providing us with some unpublished data for .
FUNDING
The writing of this article was supported by grants to the third and fourth authors from the Institute of Education Science (R305A150435, R305A100215).
Additional information
Funding
References
- Adolph, K. E., Robinson, S. R., Young, J. W., & Gill-Alvarez, F. (2008). What is the shape of developmental change? Psychological Review, 115, 527–543. doi:10.1037/0033-295X.115.3.527
- Benton, A. L., Sivan, A. B., Hamsher, K., Varney, N. R., & Spreen, O. (1983). Contribution to neuropsychological assessment. New York, NY: Oxford University Press.
- Bloom, L. (1970). Language development: Form and function in emerging grammars. Cambridge, MA: MIT Press.
- Bornstein, M. H., & Tamis-LeMonda, C. S. (2010). Mother–infant interaction. In G. Bremner & T. Wachs (Eds.), Handbook of infancy (2nd ed., pp. 458–482). London, England: Blackwell.
- Bronfenbrenner, U. (1979). The ecology of human development: Experiments by design and nature. Cambridge, MA: Harvard University Press.
- Brown, R. (1973). A first language: The early stages. Cambridge, MA: Harvard University Press.
- Casey, B. J., Oliveri, M. E., & Insel, T. (2014). A neurodevelopmental perspective on the research domain criteria (RDoC) framework. Biological Psychiatry, 76, 350–353. doi:10.1016/j.biopsych.2014.01.006
- Cheng, Y., Chou, K.-H., Chen, I.-Y., Fan, Y.-T., Decety, J., & Lin, C.-P. (2010). Atypical development of white matter microstructure in adolescents with autism spectrum disorders. NeuroImage, 50, 873–882. doi:10.1016/j.neuroimage.2010.01.011
- Cicchetti, D. (2013). Developmental psychopathology. In P. Zelazo (Ed.), Oxford handbook of developmental psychology (Vol. 2, pp. 455–480). New York, NY: Oxford University Press.
- Cohan, S. L., Chavira, D. A., Shipon-Blum, E., Hitchcock, C., Roesch, S. C., & Stein, M. B. (2008). Refining the classification of children with selective mutism: A latent profile analysis. Journal of Clinical Child & Adolescent Psychology, 37, 770–784. doi:10.1080/15374410802359759
- Courchesne, E., Carper, R., & Akshoomoff, N. (2003). Evidence of brain overgrowth in the first year of life in autism. Journal of the American Medical Association, 290, 337–344. doi:10.1001/jama.290.3.337
- Dromi, E. (1987). Early lexical development. Cambridge, UK: Cambridge University Press.
- D’Souza, D., Booth, R., Connolly, M., Happé, F., & Karmiloff-Smith, A. (2015). Rethinking the concepts of ‘local or global processors’: Evidence from Williams syndrome, Down syndrome, and autism spectrum disorders. Developmental Science, 19, 452–468. doi:10.1111/desc.12312
- Elman, J. L. (1993). Learning and development in neural networks: The importance of starting small. Cognition, 48, 71–99. doi:10.1016/0010-0277(93)90058-4
- Elman, J. L., Bates, E., Johnson, M. H., Karmiloff-Smith, A., Parisi, D., & Plunkett, K. (1996). Rethinking innateness: Connectionism in a developmental framework. Cambridge, MA: MIT Press.
- Estes, A., Zwaigenbaum, L., Gu, H., St. John, T., Paterson, S., Elison, J. T., & IBIS Network. (2015). Behavioral, cognitive, and adaptive development in infants with autism spectrum disorder in the first 2 years of life. Journal of Neurodevelopmental Disorders, 7, 24. doi:10.1186/s11689-015-9117-6
- Feigenson, L., Dehaene, S., & Spelke, E. (2004). Core systems of number. Trends in Cognitive Sciences, 8, 307–314. doi:10.1016/j.tics.2004.05.002
- Ford, D. H., & Lerner, R. M. (1992). Developmental systems theory: An integrative approach. Newbury Park, CA: Sage.
- Gottlieb, G. (1991). Experiential canalization of behavioral development: Results. Developmental Psychology, 27, 35–39. doi:10.1037/0012-1649.27.1.35
- Grice, S. J., Spratling, M. W., Karmiloff-Smith, A., Halit, H., Csibra, G., De Haan, M., & Johnson, M. H. (2001). Disordered visual processing and oscillatory brain activity in autism and Williams syndrome. NeuroReport, 12, 2697–2700. doi:10.1097/00001756-200108280-00021
- Grønborg, T. K., Schendel, D. E., & Parner, E. T. (2013). Recurrence of autism spectrum disorders in full- and half-siblings and trends over time: A population-based cohort study. JAMA Pediatrics, 167, 947–953. doi:10.1001/jamapediatrics.2013.2259
- Hazlett, H. C., Gu, H., McKinstry, R., Shaw, D. W. W., Botteron, K. N., Dager, S., … Piven, J. (2012). Brain volume findings in 6-month-old infants at high familial risk for autism. American Journal of Psychiatry, 6, 601–608. doi:10.1176/appi.ajp.2012.11091425
- Hazlett, H. C., Poe, M., Gerig, G., Smith, R. G., Provenzale, J., Ross, A., … Piven, J. (2005). Magnetic resonance imaging and head circumference study of brain size in autism. Archives of General Psychiatry, 62, 1366–1376. doi:10.1001/archpsyc.62.12.1366
- Hodapp, R. M., Burack, J. A., & Zigler, E. (1990). The developmental perspective in the field of mental retardation. In R. M. Hodapp, J. A. Burack, & E. Zigler (Eds.), Issues in the developmental approach to mental retardation (pp. 3–26). New York, NY: Cambridge University Press.
- Hofer, S. M., & Piccinin, A. M. (2010). Toward an integrative science of life-span development and aging. Journals of Gerontology: Series B. Psychological Sciences and Social Sciences, 65B, 269–278. doi:10.1093/geronb/gbq017
- Hoff, E., Rumiche, R., Burridge, A., Ribot, K. M., & Welsh, S. N. (2014). Expressive vocabulary development in children from bilingual and monolingual homes: A longitudinal study from two to four years. Early Childhood Research Quarterly, 29, 433–444. doi:10.1016/j.ecresq.2014.04.012
- Insel, T., Cuthbert, B., Garvey, M., Heinssen, R., Pine, D. S., Quinn, K., & Wang, P. (2010). Research domain criteria (RDoC): Toward a new classification framework for research on mental disorders. American Journal of Psychiatry, 167, 748–751. doi:10.1176/appi.ajp.2010.09091379
- Jackson, J. J., & Allemand, M. (2014). Moving personality development research forward: Applications using structural equation models. European Journal of Personality, 28, 300–310. doi:10.1002/per.v28.3
- Johnson, M. H. (2005). Developmental cognitive neuroscience (2nd ed.). Oxford, UK: Blackwell.
- Johnson, M. H. (2011). Interactive specialization: A domain-general framework for human functional brain development? Developmental Cognitive Neuroscience, 1, 7–21. doi:10.1016/j.dcn.2010.07.003
- Karasik, L. B., Tamis-LeMonda, C. S., & Adolph, K. E. (2014). Crawling and walking infants elicit different verbal responses from mothers. Developmental Science, 17, 388–395. doi:10.1111/desc.2014.17.issue-3
- Karmiloff-Smith, A. (1998). Development itself is the key to understanding developmental disorders. Trends in Cognitive Sciences, 2, 389–398. doi:10.1016/S1364-6613(98)01230-3
- Karmiloff-Smith, A. (2012). Development is not about studying children: The importance of longitudinal approaches. American Journal on Intellectual and Developmental Disabilities, 117, 87–89. doi:10.1352/1944-7558-117.2.87
- Karmiloff-Smith, A. (2013). Challenging the use of adult neuropsychological models for explaining neurodevelopmental disorders: Developed versus developing brains. Quarterly Journal of Experimental Psychology, 66, 1–13. doi:10.1080/17470218.2012.744424
- Karmiloff-Smith, A., Brown, J. H., Grice, S., & Paterson, S. (2003). Dethroning the myth: Cognitive dissociations and innate modularity in Williams syndrome. Developmental Neuropsychology, 23, 227–242. doi:10.1080/87565641.2003.9651893
- Karmiloff-Smith, A., D’Souza, D., Dekker, T. M., van Herwegen, J., Xu, F., Rodic, M., & Ansari, D. (2012). Genetic and environmental vulnerabilities in children with neurodevelopmental disorders. Proceedings of the National Academy of Sciences of the United States of America, 109, 17261–17265. doi:10.1073/pnas.1121087109
- Keller, T. A., Kana, R. K., & Just, M. A. (2007). A developmental study of the structural integrity of white matter in autism. NeuroReport, 18, 23–27. doi:10.1097/01.wnr.0000239965.21685.99
- Landa, R., & Garrett-Mayer, E. (2006). Development in infants with autism spectrum disorders: A prospective study. Journal of Child Psychology and Psychiatry, 47, 629–638. doi:10.1111/jcpp.2006.47.issue-6
- Landa, R. J., Gross, A. L., Stuart, E. A., & Faherty, A. (2013). Developmental trajectories in children with and without autism spectrum disorders: The first 3 years. Child Development, 84, 429–442. doi:10.1111/j.1467-8624.2012.01870.x
- Lerner, R. M. (1996). Relative plasticity, integration, temporality, and diversity in human development: A developmental contextual perspective about theory, process, and method. Developmental Psychology, 32, 781–786. doi:10.1037/0012-1649.32.4.781
- Lerner, R. M. (2006). Developmental science, developmental systems, and contemporary theories of human development. In W. Damon & R. M. Lerner (Eds.), The handbook of child psychology: Vol. 1. Theoretical models of human development (6th ed., pp. 1–17). Hoboken, NJ: Wiley.
- Lerner, R. M., & Hood, K. E. (1986). Plasticity in development: Concepts and issues for intervention. Journal of Applied Developmental Psychology, 7, 139–152. doi:10.1016/0193-3973(86)90025-0
- Massand, E., Bowler, D. M., Mottron, L., Hosein, A., & Jemel, B. (2013). ERP correlates of recognition memory in autism spectrum disorder. Journal of Autism and Developmental Disorders, 43, 2038–2047. doi:10.1007/s10803-012-1755-x
- Masten, A. S., & Cicchetti, D. (2010). Developmental cascades. Development and Psychopathology, 22, 491–495. doi:10.1017/S0954579410000222
- McArdle, J. J., & Epstein, D. (1987). Latent growth curves within developmental structural equation models. Child Development, 58, 110–133. doi:10.2307/1130295
- Meredith, R. M. (2015). Sensitive and critical periods during neurotypical and aberrant neurodevelopment: A framework for neurodevelopmental disorders. Neuroscience & Biobehavioral Reviews, 50, 180–188. doi:10.1016/j.neubiorev.2014.12.001
- Mottron, L., Burack, J., Iarocci, G., Belleville, S., & Enns, J. (2003). Locally oriented perception with intact global processing among adolescents with high functioning autism: Evidence from multiple paradigms. Journal of Child Psychology and Psychiatry, 44, 904–913. doi:10.1111/jcpp.2003.44.issue-6
- Mottron, L., Burack, J., Stauder, J., & Robaey, P. (1999). Perceptual processing among high-functioning persons with autism. Journal of Child Psychology and Psychiatry, 40, 203–211. doi:10.1111/jcpp.1999.40.issue-2
- Mullen, E. M. E. (1995). Mullen Scales of Early Learning. Circle Pines, MN: AGS.
- Müller, R., Shih, P., Keehn, B., Deyoe, J. R., Leyden, K. M., & Shukla, D. K. (2011). Underconnected, but how? A survey of functional connectivity MRI studies in autism spectrum disorders. Cerebral Cortex, 21, 2233–2243. doi:10.1093/cercor/bhq296
- Overton, W. F. (2014). The process-relational paradigm and relational-development-systems metamodel as context. Research in Human Development, 11, 323–331. doi:10.1080/15427609.2014.971549
- Overton, W. F., & Lerner, R. M. (2012). Relational developmental systems: Paradigm for developmental science in the post-genomic era. Behavioral and Brain Sciences, 35, 375–376. doi:10.1017/S0140525X12001082
- Overton, W. F., & Lerner, R. M. (2014). Fundamental concepts and methods in developmental science: A relational perspective. Research in Human Development, 11, 63–73. doi:10.1080/15427609.2014.881086
- Ozonoff, S., Heung, K., Byrd, R., Hansen, R., & Hertz-Picciotto, I. (2008). The onset of autism: Patterns of symptom emergence in the first years of life. Autism Research, 1, 320–328. doi:10.1002/aur.v1:6
- Ozonoff, S., Iosif, A.-M., Baguio, F., Cook, I. C., Hill, M. M., Hutman, T., & Young, G. S. (2010). A prospective study of the emergence of early behavioral signs of autism. Journal of the American Academy of Child and Adolescent Psychiatry, 49, 256–266.
- Ozonoff, S., Young, G. S., Carter, A., Messinger, D., Yirmiya, N., Zwaigenbaum, L., … Hutman, T. (2011). Recurrence risk for autism spectrum disorders: A baby siblings research consortium study. Pediatrics, 128, e488–e495.
- Paterson, S. J., Brown, J. H., Gsodl, M. K., Johnson, M. H., & Karmiloff-Smith, A. (1999). Cognitive modularity and genetic disorders. Science, 286, 2355–2358. doi:10.1126/science.286.5448.2355
- Paterson, S. J., Girelli, L., Butterworth, B., & Karmiloff-Smith, A. (2006). Are numerical impairments syndrome specific? Evidence from Williams syndrome and Down syndrome. Journal of Child Psychology and Psychiatry, 47, 190–204. doi:10.1111/j.1469-7610.2005.01460.x
- Plaisted, K., Swettenham, J., & Rees, L. (1999). Children with autism show local precedence in a divided attention task and global precedence in a selective attention task. Journal of Child Psychology and Psychiatry, 40, 733–742. doi:10.1111/jcpp.1999.40.issue-5
- Rice, M. L. (2004). Growth models of developmental language disorders. In M. L. Rice & S. F. Waren (Eds.), Developmental language disorders: From phenotypes to etiologies (pp. 207–240). Mahwah, NJ: Erlbaum.
- Rowe, M. L., & Goldin-Meadow, S. (2009). Early gesture selectively predicts later language learning. Developmental Science, 12, 182–187. doi:10.1111/desc.2009.12.issue-1
- Rutter, M., Le Couteur, A., & Lord, C. (2003). Autism Diagnostic Interview-Revised. Los Angeles, CA: Western Psychological Services.
- Schumann, C. M., Bloss, C. S., Barnes, C. C., Wideman, G. M., Carper, R. A., Akschoomoff, N., … Courchesne, E. (2010). Longitudinal magnetic resonance imaging study of cortical development through early childhood in autism. Journal of Neuroscience, 30, 4419–4427. doi:10.1523/JNEUROSCI.5714-09.2010
- Shah, A., & Frith, U. (1993). Why do autistic individuals show superior performance on the Block Design Task? Journal of Child Psychology and Psychiatry, 34, 1351–1364. doi:10.1111/jcpp.1993.34.issue-8
- Shukla, D. K., Keehn, B., & Müller, R. (2011). Tract-specific analyses of diffusion tensor imaging show widespread white matter compromise in autism spectrum disorder. Journal of Child Psychology and Psychiatry, 52, 286–295. doi:10.1111/j.1469-7610.2010.02342.x
- Siegler, R. S., & Crowley, K. (1991). The microgenetic method: A direct means for studying cognitive development. American Psychologist, 46, 606–620. doi:10.1037/0003-066X.46.6.606
- Singer, J. D., & Willett, J. B. (2003). Applied longitudinal data analysis: Modeling change and event occurrence. New York, NY: Oxford University Press.
- Thomas, M. S. C. (2016). Understanding delay in developmental disorders. Child Development Perspectives, 10, 73–80. doi:10.1111/cdep.12169
- Thomas, M. S., Annaz, D., Ansari, D., Scerif, G., Jarrold, C., & Karmiloff-Smith, A. (2009). Using developmental trajectories to understand developmental disorders. Journal of Speech, Language, and Hearing Research, 52, 336–358. doi:10.1044/1092-4388(2009/07-0144)
- van Herwegen, J., Ansari, D., Xu, F., & Karmiloff-Smith, A. (2008). Small and large number processing in infants and toddlers with Williams syndrome. Developmental Science, 11, 637–643. doi:10.1111/desc.2008.11.issue-5
- Wang, L., Mottron, L., Peng, D., Berthiaume, C., & Dawson, M. (2007). Local bias and local-to-global interference without global deficit: A robust finding in autism under various conditions of attention, exposure time, and visual angle. Cognitive Neuropsychology, 24, 550–574. doi:10.1080/13546800701417096
- Werner, H. (1937). Process and achievement. Harvard Educational Review, 7, 353–368.
- Werner, H. (1957). The concept of development from a comparative and organismic point of view. In D. Harris (Ed.), The concept of development (pp. 125–148). Minneapolis: University of Minnesota Press.
- Winder, B. M., Wozniak, R. H., Parladé, M. V., & Iverson, J. M. (2013). Spontaneous initiation of communication in infants at low and heightened risk for autism spectrum disorders. Developmental Psychology, 49, 1931–1942. doi:10.1037/a0031061
- Wolff, J., Gu, H., Gerig, G., Elison, J., Styner, S., Gouttard, S., … Piven, J. (2012). Differences in white matter fiber tract development present from 6 to 24 months in infants with autism. American Journal of Psychiatry, 6, 589–600. doi:10.1176/appi.ajp.2011.11091447
- Zigler, E. (1969). Developmental versus difference theories of mental retardation and the problem of motivation. American Journal of Mental Deficiency, 73, 536–556.
- Zigler, E., & Hodapp, R. M. (1986). Understanding mental retardation. New York, NY: Cambridge University Press.