ABSTRACT
Traumatic brain injury (TBI) due to blast exposure or head impacts in accidents or contact sports is one of the most critical and poorly understood areas of research in the 21st century. To date, the unavailability of human brain tissues (grey and white matter especially) due to ethical and biosafety issues has not allowed for much experimental research into the study of the mechanics of brain tissues under impact or dynamic loading. In the current work, for the first time, biofidelic brain tissue surrogates have been developed using a low cost, castable (to any shape or size), two-part silicone-based material system to precisely mimic the nonlinear mechanical properties of both the white and the grey matter. The fabrication methodology involves the iterative mixing of the two parts of silicone at certain mix ratios (by weight) to generate a biomechanical behavior similar to the white and the grey matter tissues, respectively, at two different strain rates (low and high). The nonlinear behavior of these novel brain tissue surrogates have been characterized using five hyperelastic material models. These brain tissue simulant materials would be indispensable not only for the study of TBI, but also to allow doctors to practice brain surgeries (for training purposes) in a clinical setting. Additionally, crucial brain tissue modifications in Alzheimer's disease and dementia can be studied in the future with such accessible biofidelic brain tissue surrogate materials.
1. Introduction
Even after decades of studies, the brain remains one of the most mysterious parts of the human anatomy. The central operator of the nervous system, the brain, controls every thought and action performed by the body. The principal controlling part of the brain, the cerebrum, is composed of two unique tissues called white matter and grey matter Citation[1] (see ).
In addition to dendrites and axons for data collection, synapses for inter-neuron communication, glial cells for support and maintenance, and capillaries for microcirculation of blood, grey matter houses the majority of neuronal cell bodies in the brain. White matter, on the other hand, is composed primarily of long-range axon tracks for information transport. The color difference between the two arises from the white myelin coating present on the axons in white matter. Generally, the grey matter is responsible for processing stimuli leading to human cognition, while white matter allows for communication throughout the brain to generate a response to the stimuli. Typically, grey matter accounts for 40% of the cerebrum by mass, while white matter composes the remaining 60% Citation[1].Traumatic brain injury (TBI) is the damage to the brain caused by mechanical impact, which results in temporary or permanent damage of brain function Citation[2, 3]. In the last decade, over 60% of the soldiers deployed in Iraq and Afghanistan have been diagnosed with symptoms of TBI due to exposure to blasts. Blast-induced TBI has been studied extensively using computational modeling Citation[4–16] techniques. TBI has also been found to be a critical issue in contact sports where sudden head impacts are very common occurrences Citation[17–19]. Additionally, TBI is very common in accidents, such as automotive collisions or falls. It has been observed that TBI causes drastic changes in the mechanical properties of the brain tissues Citation[20–23]. Besides TBI, major tissue modifications have also been observed in diseases, such as Alzheimer's Citation[24–26], dementia Citation[27, 28], and even in the case of hypertension Citation[29]. Additionally, grey and white matter tissues have been found to change with age Citation[30, 31], which could be attributed to the age-related mental and physical changes in the human body.
To date, brain tissues are very difficult to obtain for experimental tests in a clinical and lab setting due to ethical and biosafety issues. Additionally, brain tissues obtained from a cadaver change significantly in mechanical behavior due to tissue dehydration and death Citation[32, 33]. In the current work, for the first time, a silicone-based material system is developed, which can precisely mimic the nonlinear mechanical behavior (biofidelity) of the human grey and white matter tissues. The fabrication and manufacturing methodology is based on a recent provisional patent application U.S. 62/189,504 named “Biofidelic Human Skin Simulant” by Chanda et al. Citation[34]. These biofidelic brain tissue surrogates are low cost, castable (in any shape or size), easy to handle, and possess no biosafety issues. The nonlinear material behavior of both the white and grey matter tissue surrogates was fully characterized using five hyperelastic curve fit models whose accuracies of prediction were also estimated. The major applicationarea for this state of the art technology is to study TBI through understanding the effects of impact and shockwave (i.e., blast) loads on white and grey matter tissues. Furthermore, brain surgeries can be practiced by doctors in a clinical setting with these tissue surrogates. Additionally, tissue modifications due to age, Alzheimer's disease, and dementia can be studied to understand their genesis and pathophysiology. Section 2 presents the fabrication and manufacturing methodology for the brain tissue surrogates, and also the nonlinear material modeling methodologies. Section 3 discusses the various results from our pioneering work, followed by conclusions in Section 4.
2. Materials and methods
2.1. Fabrication of brain tissue surrogates
Brain tissues (both white and grey matter) are very soft materials Citation[35], which disintegrate easily with the application of loads over a few hundred kilopascals (kPA). This is one of the principal reasons for brain tissues to be tested in compression more often than in tension in the literature Citation[35–40]. Recently, brain tissues (both grey and white matter) were mechanically tested in tension extensively at different strain rates (low and high) Citation[41]. Based on these tests and the recently developed biofidelic human skin simulants Citation[34] by Chanda et al. (U.S Patent Application 62/189,504), biofidelic brain tissues surrogates were fabricated using an extremely soft two-part silicone with Shore (Durometer) hardness (defined as per the American Society for Testing and Materials (ATSM) D2240 testing standard) of 10, procured from Smooth-On Inc. The part A of the silicone material was identified as the hardener, which polymerizes only when added to part B (which activates a silicone functional group to crosslink). A mold was designed () in Solidworks CAD software with 30 coupons of 5-cm length, 1-cm width, and 3-mm depth. The overall dimension of the mold was 21 cm × 18 cm × 1 cm. This mold design was exported as a steriolithographic (STL) file and 3D printed at the University of Alabama 3D-printing lab. The 3D printer used was a Stratasys Dimension SST 1200es and the material used for fabricating the mold was Acrylonitrile butadiene styrene (ABS), a very popular material used in the 3D printing industry, which provides a good surface finish and high strength. The 3D printed mold is shown in .
The two silicone parts (A and B) were mixed with different ratios by weight starting at 1:1, precisely measured using measuring cups and an Ohaus Adventurer Pro precesion weight measuring device. After a uniform mixing of the two parts, the average setting time for coupons was recorded to be between 2.5 and 3 h. The colorless coupons (see ) were marked appropriately to keep track of their composition during testing. Each coupon was mounted in between grips of a MTS universal testing machine (as shown in ) A small initial load (<0.1 N) was applied on each test specimen to ensure the absence of any initial slack in the specimen. Constant strain rates of 2.5 mm/s (low) and 30 mm/s (high), and specimen size of 35 mm × 10 mm × 2.5 mm (after clamping) were chosen for the tests based on the literature Citation[41]. For each of the tests, the load (N) versus extension (mm) graphs were plotted.
A total of 85 test coupons (with different two-part silicone compositions) of similar dimensions after clamping were tested at the constant strain rates. Out of the 85 tests, 30 coupons each were tested at a strain rate of 2.5 mm/s (low) and 30 mm/s (high), respectively. The other 25 test coupons were chosen as control specimens (discussed in Section 3) and tested multiple times to ensure repeatibility of the test results.
The load versus extension plots were postprocessed in four steps to obtain engineering stress versus engineering strain plots. First, any part of the plots with negative load values were trimmed off (which may arise from the initial specimen slack). Second, the calibration errors in the MTS machine were tracked and the graphs were shifted accordingly to start from the origin. Third, the calibrated load versus extension plots were replotted as engineering stress (σeng) versus engineering strain (ϵeng) plots using Eqs. (Equation1(1) ) and (Equation2
(2) ). Equation (Equation3
(3) ) was used to change strain (ϵeng) into stretch (λ), which is used for hyperelastic formulations in the following section.
(1)
(2)
(3)
2.2. Material modeling
Soft tissues and silicone rubbers have a nonlinear stress versus strain response Citation[42, 43]. Brain tissues have been observed in the literature to reduce in stiffness (or be more compliant) at high strains Citation[41]. Hyperelastic constitutivematerial models, such as Fung, Mooney–Rivlin, Yeoh, Veronda–Westmann, and Humphrey Citation[44], have been used in the current work to precisely characterize the nonlinear mechanical behavior of brain tissues (both grey and white matter). Hyperelastic curve fit models are based on the definition of the strain-energy function (denoted as Ψ), which further depends on the type of material Citation[45, 46]. Any hyperelastic model is dependent on either the principal stretches (λ1, λ2, and λ3) or the Cauchy–Green tensor invariants (I1, I2, and I3, which are also functions on the principal stretches) Citation[44] as shown in Eqs. (Equation4–7). Through uniaxial tests on any specimen and following the procedure outlined in the literature by Martins et al. Citation[44], the principal Cauchy stress is expressed in terms of the stretch and the strain energy function using Eq. (Equation8(8) ):
(4)
(5)
(6)
(7)
(8)
Solving Eq. (Equation8(8) ) using hyperelastic strain energy functions corresponding to the Fung, Mooney–Rivlin, Yeoh, Veronda–Westmann, and Humphrey models, the engineering stress versus stretch responses of the test coupons were curve-fit using Eqs. (Equation9–13), respectively. The parameters μ and b (Fung model), c1 and c2 (Mooney–Rivlin, Humphrey, and Veronda–Westmann model), c1, c2, and c3 (Yeoh), were estimated in Section 3.
(9)
(10)
(11)
(12)
(13)
To evaluate the accuracy of the hyperelastic model chosen in predicting the brain tissue simulant material behavior, an average R2 correlation value calculation was performed in the five cases, where R2 can have a value from 0 through 1, 1 representing the best fit and 0 representing the worst fit.
It should be mentioned that human brain shows regional material anisotropy. However, at a localized tissue level, grey matter can be considered to be an isotropic material Citation[47]. The white matter on the other hand, has been considered to be isotropic Citation[48, 49] or transversely isotropic Citation[50] under uniaxial loading conditions in the literature. Therefore, in this work, our assumption of isotropic material behavior for the grey and white matter tissue simulants is reasonable.
3. Results and discussions
3.1. Brain tissue simulant tests
Theparts A and B of the two-part silicone material were mixed in different ratios by weight as discussed in Section 2 to generate a total of 30 test specimens. Five specimens with 70% A–30% B, five specimens with 60% A–40% B, six specimens with 50% A–50% B, five specimens with 30% A–70% B, five specimens with 20% A–80% B, and four specimens with 10% A–90% B compositions were tested three times each at high (50 mm/s) and low (2.5 mm/s) strain rates, respectively, to obtain the average plots in . It was observed that the silicone specimens do not show significant variations with testing rates () unlike actual human brain tissues. Thereby, it was concluded that the mechanical behavior of brain tissues (white and grey matter) at two different strain rates can be characterized only with two different two-part silicone compositions.
Figure 5. Brain tissue simulant tests and comparison with data from the literature Citation[41].
![Figure 5. Brain tissue simulant tests and comparison with data from the literature Citation[41].](/cms/asset/2105a51e-617d-45d7-aed6-34648b5f4637/umcm_a_1143749_f0005_b.gif)
The range of mix ratios within which the literature based white and grey matter tissue properties (at high and low strain rate tests) lie were identified from and summarized in . The softest brain tissue simulants developed corresponded to the grey matter both at low and high strain rates. The hardest tissue simulant was for the white matter at a low strain rate.
Table 1. Silicone composition ranges for grey and white matter tissues at low and high strain rates.
3.2. Control specimens and repeatability results
Three sets of control brain tissue surrogates were identified corresponding to three different two-part silicone compositions (summarized in ). The first control specimen was a 50% A–50% B composition, which closely mimicks the mean white matter mechanical behavior Citation[41] at a low strain rate of 2.5 mm/s. Three sets of tests comprised of eight test coupons were tested two times each to evaluate the repeatability of test results, the average results of which are presented in .
Table 2. Control test specimen compositions for mimicking mean brain tissue biomechanical properties and for repeatibility tests.
Figure 6. Brain tissue composition (control specimen 1) mimicking mean white matter mechanical behavior Citation[41] at low strain rate testing, tested for repeatibility.
![Figure 6. Brain tissue composition (control specimen 1) mimicking mean white matter mechanical behavior Citation[41] at low strain rate testing, tested for repeatibility.](/cms/asset/51689ec3-e1f6-4c14-b8b5-26180d33258a/umcm_a_1143749_f0006_b.gif)
Thesecond control specimen was a 30% A–70% B composition simulating the mean grey matter mechanical properties Citation[41] at the low strain rate value of 2.5 mm/s. Similar to the case of control specimen 1, three sets of tests with 10 coupons were tested two times each. The average repeatibility results are shown in .
Figure 7. Brain tissue composition (control specimen 2) mimicking mean grey matter mechanical behavior Citation[41] at low strain rate testing, tested for repeatibility.
![Figure 7. Brain tissue composition (control specimen 2) mimicking mean grey matter mechanical behavior Citation[41] at low strain rate testing, tested for repeatibility.](/cms/asset/dfed93e3-88cb-4c21-915b-3d2816e9588e/umcm_a_1143749_f0007_b.gif)
A third control specimen with 10% A–70% B composition was used to simulate the mean nonlinear mechanical behavior (literature-based plots) of both the white and the grey matter at high strain rate (30 mm/s) testing. This was done as the white and grey matter plots at high test rates are reasonably close to each other. Seven test coupons were tested two times each to check for repeatability of test results (see ).
Figure 8. Brain tissue composition (control specimen 3) simulating mean grey and white matter mechanical properties Citation[41] at high strain rate testing (30 mm/s), tested for repeatibility.
![Figure 8. Brain tissue composition (control specimen 3) simulating mean grey and white matter mechanical properties Citation[41] at high strain rate testing (30 mm/s), tested for repeatibility.](/cms/asset/288aabec-799a-48d9-a170-cb1727bacc92/umcm_a_1143749_f0008_b.gif)
3.3. Hyperelastic nonlinear curve fits
Nonlinear hyperelastic curve fit models were used to precisely characterize the stress versus stretch response of the test specimens as discussed in Section 2. The average stress versus strain results of six two-part silicone compositions tested at high and low strain rates (shown in ) were fitted with second degree polynomial trend lines as shown in . The average of the high and low strain rate tests of the silicone specimens were considered as their stress versus strain (or stretch in ) are in close proximity to each other (see for reference where the high and low tests for any silicone composition are very close). The results of the Fung, Mooney–Rivlin, Yeoh, Veronda–Westmann, and Humphrey Citation[44] model curve fitting (with the precisely estimated parameters) are summarized in
Figure 9. Average polynomial trendline fits of the silicone specimens tested at high and low strain rates (based on ) for hyperelastic curve fitting.
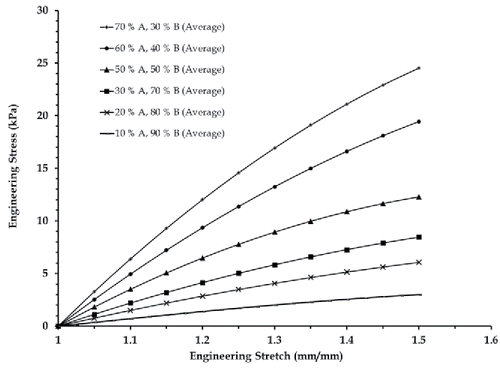
Table 3. Hyperelastic curve fit parameters for the average stress vs. stretch plots of silicone specimens (brain tissue simulants) corresponding to .
The accuracy of prediction of nonlinear behavior of brain tissue simulants was estimated for each hyperelastic model () through numerical estimation of average R2 corellation index in each case. summarizes the R2 corellation values, which fall within 0.9 < R2 < 1. The highest corellation index of (R2 = 0.997) was generated by the three-parameter Yeoh's hyperelastic model, and the least (R2 = 0.953) was for the primitive Fung's model. This finding was in line with the known comparative accuracy of the hyperelastic constitutive material models in the literature Citation[41].
Table 4. Accuracy of hyperelastic curve fitting estimated using an average R2 corellation value calculation.
4. Conclusions
In this work, slicone-based biofidelic human brain tissue simulants for the grey and white matter were developed for the first time. The fabrication methodology, experimental framework, and comparison study with human brain tissue test data from the literature were discussed. The brain tissue simulants for both the grey and the white matter were tested in tension at two different strain rates (low and high), and the two-part silicone compositions corresponding to white and grey matter tissue properties were estimated. The nonlinear stress versus stretch responses of the brain tissue surrogates were characterized using five hyperelastic constitutive models, namely, the Fung, Mooney–Rivlin, Yeoh, Veronda–Westmann, and Humphrey models. The three-parameter Yeoh's model was found to be the most accurate in predicting the nonlinear behavior of the brain tissue surrogates (both the white and the grey matter), with an average R2 correlation index of 0.997. Three control specimen compositions were identified to closely simulate the white and grey matter tissues at low and high strain rates, and were extensively tested for repeatability.
There are two limitations of the presented silicone-based material system. First, all of the compositions are isotropic and anisotropy effects are difficult to incorporate in such a material system. However, this was not of much concern as grey matter and white matter tissues have been claimed to be isotopic and transversely isotropic, respectively, under uniaxial tension in the literature. Second, strain rate variations do not affect the stress versus strain response of the silicone material significantly unlike in an actual brain tissue. Therefore, mechanical behavior of a brain tissue tested at two different strain rates can only be simulated using two different two-part silicone compositions.
The biofidelic brain tissue surrogates have no biosafety or handling issues, and would be indispensable for replacing cadavers and human brain tissues traditionally used for practicing brain surgeries in a clinical setting. Traumatic brain injury (TBI) in impact loading conditions (such as in blasts, contact sports, accidents, etc.) can be studied using the novel brain tissue simulants. Additionally, brain tissue material modifications due to age, and diseases, such as Alzheimer's disease and dementia, could be investigated to better understand their effects on human cognitive functions.
Additional information
Funding
References
- J.K. Mai, J. Assheuer, and G. Paxinos, Atlas of the Human Brain, Academic Press, San Diego, CA, 1997.
- E.D. Bigler, M. Weiner, and A. Lipton, Traumatic brain injury. In: Textbook of Alzheimer Disease and Other Dementias, The American Psychiatric Association Publishing, Arlington, VA, pp. 229–246, 2009.
- J. Ghajar, Traumatic brain injury, Lancet, vol. 356, pp. 923–929, 2000.
- M.S. Chafi, S. Ganpule, L. Gu, and N. Chandra, Dynamic response of brain subjected to blast loadings: Influence of frequency ranges, Int. J. Appl. Mech., vol. 3, pp. 803–823, 2011.
- N. Chandra, S. Ganpule, N. Kleinschmit, R. Feng, A. Holmberg, A. Sundaramurthy, V. Selvan, and A. Alai, Evolution of blast wave profiles in simulated air blasts: Experiment and computational modeling, Shock Waves, vol. 22, pp. 403–415, 2012.
- S. Ganpule, and N. Chandra, Mechanics of interaction of blast waves on surrogate head: Effect of head orientation, ASME 2013 Summer Bioengineering Conference, pp. V01BT55A028, June 26–29, Sunriver, OR, 2013.
- S. Ganpule, L. Gu, A. Alai, and N. Chandra, Role of helmet in the mechanics of shock wave propagation under blast loading conditions, Comput. Methods Biomech. Biomed. Eng., vol. 15, pp. 1233–1244, 2012.
- S. Ganpule, L. Gu, G. Cao, and N. Chandra, The effect of shock wave on a human head, ASME 2009 International Mechanical Engineering Congress and Exposition, pp. 339–346, November 13–19, Lake Buena Vista, FL, 2009.
- S. Ganpule, L. Gu, and N. Chandra, MRI-based three dimensional modeling of blast traumatic brain injury (bTBI), ASME 2010 International Mechanical Engineering Congress and Exposition, pp. 181–183, November 12–18, Vancouver, Canada, 2010.
- L. Gu, M.S. Chafi, S. Ganpule, and N. Chandra, The influence of heterogeneous meninges on the brain mechanics under primary blast loading, Composites Part B, vol. 43, pp. 3160–3166, 2012.
- R.K. Gupta, and A. Przekwas, Mathematical models of blast-induced TBI: Current status, challenges, and prospects, Front. Neurol., vol. 4, pp. 1–21, 2013.
- K. Kangarlou, Mechanics of blast loading on the head models in the study of traumatic brain injury, Nationalpark-Forschung In Der Schweiz (Switzerland Research Park Journal), vol. 12, no. 3, pp. 511–531, 2013.
- A. Sundaramurthy, A. Alai, S. Ganpule, A. Holmberg, E. Plougonven, and N. Chandra, Blast-induced biomechanical loading of the rat: An experimental and anatomically accurate computational blast injury model, J. Neurotrauma, vol. 29, pp. 2352–2364, 2012.
- A. Chanda, R. Graeter, and V. Unnikrishnan, Effect of blasts on subject-specific computational models of skin and bone sections at various locations on the human body, AIMS Mater. Sci., vol. 2, no. 4, pp. 425–447, 2015.
- D. Jenson, and V. Unnikrishnan, Multiscale simulation of ballistic composites for blast induced traumatic brain injury mitigation, ASME 2014 International Mechanical Engineering Congress and Exposition, pp. V009T12A072–74, November 14–20, Montreal, Canada, 2014.
- D. Jenson, and V.U. Unnikrishnan, Energy dissipation of nanocomposite based helmets for blast-induced traumatic brain injury mitigation, Compos. Struct., vol. 121, pp. 211–216, 2015.
- C. F. D. Control, and Prevention, Sports-related recurrent brain injuries—United States, Int. J. Trauma Nurs., vol. 3, pp. 88–90, 1997.
- J.P. Kelly, and J.H. Rosenberg, Diagnosis and management of concussion in sports, Neurology, vol. 48, pp. 575–580, 1997.
- S.T. DeKosky, M.D. Ikonomovic, and S. Gandy, Traumatic brain injury—Football, warfare, and long-term effects, New Engl. J. Med., vol. 363, pp. 1293–1296, 2010.
- A.I. Faden, P. Demediuk, S.S. Panter, and R. Vink, The role of excitatory amino acids and NMDA receptors in traumatic brain injury, Science, vol. 244, pp. 798–800, 1989.
- C. Werner, and K. Engelhard, Pathophysiology of traumatic brain injury, Br. J. Anaesthesia, vol. 99, pp. 4–9, 2007.
- R.O.S. Mejia, V.O. Ona, M. Li, and R.M. Friedlander, Minocycline reduces traumatic brain injury-mediated caspase-1 activation, tissue damage, and neurological dysfunction, Neurosurgery, vol. 48, pp. 1393–1401, 2001.
- A.S. Cunningham, R. Salvador, J.P. Coles, D.A. Chatfield, P.G. Bradley, A.J. Johnston, L.A. Steiner, T.D. Fryer, F.I. Aigbirhio, P. Smielewski, G.B. Williams, T.A. Carpenter, J.H. Gillard, J.D. Pickard, and D.K. Menon, Physiological thresholds for irreversible tissue damage in contusional regions following traumatic brain injury, Brain, vol. 128, pp. 1931–1942, 2005.
- K.A. Crutcher, S.A. Scott, S. Liang, W.V. Everson, and J. Weingartner, Detection of NGF-like activity in human brain tissue: Increased levels in Alzheimer's disease, J. Neurosci., vol. 13, pp. 2540–2550, 1993.
- E. Englund, A. Brun, and C. Alling, White matter changes in dementia of Alzheimer's type, Brain, vol. 111, pp. 1425–1439, 1988.
- T.D. Bird, S. Stranahan, S. Sumi, and M. Raskind, Alzheimer's disease: Choline acetyltransferase activity in brain tissue from clinical and pathological subgroups, Ann. Neurol., vol. 14, pp. 284–293, 1983.
- M.A. Ikram, H.A. Vrooman, M.W. Vernooij, T. den Heijer, A. Hofman, W.J. Niessen, et al., Brain tissue volumes in relation to cognitive function and risk of dementia, Neurobiol. Aging, vol. 31, pp. 378–386, 2010.
- R. Mielke, K. Herholz, M. Grond, J. Kessler, and W.-D. Heiss, Severity of vascular dementia is related to volume of metabolically impaired tissue, Arch. Neurol., vol. 49, pp. 909–913, 1992.
- F.E. de Leeuw, J.C. de Groot, M. Oudkerk, J. Witteman, A. Hofman, J. Van Gijn, et al., Hypertension and cerebral white matter lesions in a prospective cohort study, Brain, vol. 125, pp. 765–772, 2002.
- Y. Ge, R.I. Grossman, J.S. Babb, M.L. Rabin, L.J. Mannon, and D.L. Kolson, Age-related total gray matter and white matter changes in normal adult brain. Part II: Quantitative magnetization transfer ratio histogram analysis, Am. J. Neuroradiol., vol. 23, pp. 1334–1341, 2002.
- Y. Ge, R.I. Grossman, J.S. Babb, M.L. Rabin, L.J. Mannon, and D.L. Kolson, Age-related total gray matter and white matter changes in normal adult brain. Part I: volumetric MR imaging analysis, Am. J. Neuroradiol., vol. 23, pp. 1327–1333, 2002.
- L. Pantoni, J.H. Garcia, and J.A. Gutierrez, Cerebral white matter is highly vulnerable to ischemia, Stroke, vol. 27, pp. 1641–1647, 1996.
- T. Novitzky, D.K. Cooper, D. Morrell, and S. Isaacs, Change from aerobic to anaerobic metabolism after brain death, and reversal following triiodothyronine therapy, Transplantation, vol. 45, pp. 32–36, 1988.
- A. Chanda, V. Unnikrishnan, and Z. Flynn, Biofidelic human skin simulant, U.S. Patent No. 62/189,504, 2015.
- K. Miller, and K. Chinzei, Constitutive modelling of brain tissue: Experiment and theory, J. Biomech., vol. 30, pp. 1115–1121, 1997.
- B. Rashid, M. Destrade, and M.D. Gilchrist, Mechanical characterization of brain tissue in compression at dynamic strain rates, J. Mech. Behav. Biomed. Mater., vol. 10, pp. 23–38, 2012.
- F. Pervin, and W.W. Chen, Dynamic mechanical response of bovine gray matter and white matter brain tissues under compression, J. Biomech., vol. 42, pp. 731–735, 2009.
- K. Miller, Method of testing very soft biological tissues in compression, J. Biomech., vol. 38, pp. 153–158, 2005.
- M. Estes, and J.H. MacElhaney, Response of Brain Tissue to Compressive Loading, ASME, New York, 1970.
- J.E. Galford, and J.H. McElhaney, A viscoelastic study of scalp, brain, and dura, J. Biomech., vol. 3, pp. 211–221, 1970.
- X. Jin, F. Zhu, H. Mao, M. Shen, and K.H. Yang, A comprehensive experimental study on material properties of human brain tissue, J. Biomech., vol. 46, pp. 2795–2801, 2013.
- O.A. Shergold, N.A. Fleck, and D. Radford, The uniaxial stress versus strain response of pig skin and silicone rubber at low and high strain rates, Int. J. Impact Eng., vol. 32, pp. 1384–1402, 2006.
- A. Chanda, V. Unnikrishnan, S. Roy, and H.E. Richter, Computational modeling of the female pelvic support structures and organs to understand the mechanism of pelvic organ prolapse: A review, Appl. Mech. Rev., vol. 67, art. 040801, 2015.
- P. Martins, R. Natal Jorge, and A. Ferreira, A comparative study of several material models for prediction of hyperelastic properties: Application to silicone‐rubber and soft tissues, Strain, vol. 42, pp. 135–147, 2006.
- L.Y.S. Gonzalez, M.G. Botero, and M. Betancur, Hyperelastic Material Modeling, Universidad EAFIT, Medellín, Colombia, 2005.
- G.A. Holzapfel, Nonlinear Solid Mechanics (Vol. 24), Wiley, Chichester, UK, 2000.
- M.T. Prange, and S.S. Margulies, Regional, directional, and age-dependent properties of the brain undergoing large deformation, J. Biomech. Eng., vol. 124, pp. 244–252, 2002.
- D. Meaney, Relationship between structural modeling and hyperelastic material behavior: application to CNS white matter, Biomech. Model. Mechanobiol., vol. 1, pp. 279–293, 2003.
- A.F. Christ, K. Franze, H. Gautier, P. Moshayedi, J. Fawcett, R.J. Franklin, et al., Mechanical difference between white and gray matter in the rat cerebellum measured by scanning force microscopy, J. Biomech., vol. 43, pp. 2986–2992, 2010.
- F. Velardi, F. Fraternali, and M. Angelillo, Anisotropic constitutive equations and experimental tensile behavior of brain tissue, Biomech. Model. Mechanobiol., vol. 5, pp. 53–61, 2006.