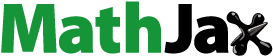
ABSTRACT
Objective: The goal of this study was to evaluate the biofidelity of the Total Human Model for Safety (THUMS; Ver. 4.01) pedestrian finite element models (PFEM) in a whole-body pedestrian impact condition using a well-characterized generic pedestrian buck model.
Methods: The biofidelity of THUMS PFEM was evaluated with respect to data from 3 full-scale postmortem human subject (PMHS) pedestrian impact tests, in which a pedestrian buck laterally struck the subjects using a pedestrian buck at 40 km/h. The pedestrian model was scaled to match the anthropometry of the target subjects and then positioned to match the pre-impact postures of the target subjects based on the 3-dimensional motion tracking data obtained during the experiments. An objective rating method was employed to quantitatively evaluate the correlation between the responses of the models and the PMHS. Injuries in the models were predicted both probabilistically and deterministically using empirical injury risk functions and strain measures, respectively, and compared with those of the target PMHS.
Results: In general, the model exhibited biofidelic kinematic responses (in the Y–Z plane) regarding trajectories (International Organization for Standardization [ISO] ratings: Y = 0.90 ± 0.11, Z = 0.89 ± 0.09), linear resultant velocities (ISO ratings: 0.83 ± 0.07), accelerations (ISO ratings: Y = 0.58 ± 0.11, Z = 0.52 ± 0.12), and angular velocities (ISO ratings: X = 0.48 ± 0.13) but exhibited stiffer leg responses and delayed head responses compared to those of the PMHS. This indicates potential biofidelity issues with the PFEM for regions below the knee and in the neck. The model also demonstrated comparable reaction forces at the buck front-end regions to those from the PMHS tests. The PFEM generally predicted the injuries that the PMHS sustained but overestimated injuries in the ankle and leg regions.
Conclusions: Based on the data considered, the THUMS PFEM was considered to be biofidelic for this pedestrian impact condition and vehicle. Given the capability of the model to reproduce biomechanical responses, it shows potential as a valuable tool for developing novel pedestrian safety systems.
Introduction
As one of the leading causes of motor vehicle–related fatality, pedestrian crashes comprise 22% of all road traffic deaths, which translates to approximately 275,000 deaths per year globally (World Health Organization Citation2013). As protection of pedestrians from injury due to crashes continues to attract worldwide attention, pedestrian finite element models (PFEMs) are viewed as potentially valuable tools for the development of pedestrian safety systems. As one of the most widely used PFEMs, the Total Human Model for Safety (THUMS) pedestrian model has been assessed for biofidelity at both the component level (Iwamoto et al. Citation2002; Maeno and Hasegawa Citation2001; Poulard, Chen, et al. Citation2015) and the full-scale level (Pass, Osth, and Davidsson Citation2015; Poulard et al. Citation2016; Watanabe et al. Citation2012) using a production vehicle. Though pedestrian impact testing and simulation using a production vehicle can represent real-world impact conditions, much of the information about the production vehicle is largely unknown outside the original equipment manufacturer. A simplified and well-characterized vehicle surrogate (i.e., a buck) provides a useful tool to assess the biofidelity of a pedestrian model or dummy (Pipkorn et al. Citation2014).
In addition to concerns regarding fidelity of the vehicle model, evaluating PFEM biofidelity using postmortem human subjects (PMHS) is challenging because the injuries sustained in vehicle–pedestrian collisions may be affected by various factors including, but not limited, to age, pre-impact posture, anthropometry, impact location, as well as collision speed (Chen et al. Citation2015; Watanabe et al. Citation2012). Even for a given impact condition (collision speed, impact location, vehicle geometry, etc.), the PMHS biomechanical response was found to be substantially influenced by pedestrian pre-impact posture and anthropometry (Paas, Osth, and Davidsson Citation2015; Subit et al. Citation2008). Consequently, normalizing responses from full-scale pedestrian experiments cab be challenging. The mass scaling methodology traditionally used for scaling biomechanical response was found to lack sufficiency (Untaroiu et al. Citation2008), and few pedestrian-specific normalization methods for kinematics responses have been published. For those reasons, researchers have scaled or morphed the anthropometry of pedestrian human body models rather than scaling the responses of a PMHS. Poulard et al. (Citation2016) evaluated the benefit of morphed and globally scaled PFEM when compared to generic PFEM by comparing the kinematics against PMHS test results. Both globally scaled and morphed PFEM improved the correlation between the model response and PMHS test results. Pre-impact posture is another variable relevant to accurate simulation of the PMHS test. Though the pre-impact postures of lower extremities have been modified to match with the PMHS tests in previous validation studies (Paas, Osth, and Davidsson Citation2015), torso postures such as spine curvature, upper torso angles, and pelvis angles are rarely considered because they require more sophisticated prepositioning approaches and detailed kinematics information from the PMHS tests.
Recently, the University of Virginia Center for Applied Biomechanics performed PMHS pedestrian impact tests using a generic vehicle buck (Forman et al. Citation2015a, Citation2015b; Tierney et al. Citation2017). The buck replicates the structural stiffness of a production vehicle using engineering materials that can be easily characterized and modeled. The buck utilized in the PMHS tests was based on a sedan-type vehicle, which demonstrated a good pedestrian protection rating (Pipkorn et al. Citation2014). The PMHS in the experimental study were close in anthropometry to a 50th percentile adult male and had an average height of 181.5 ± 3.9 cm and an average weight of 77.4 ± 3.7 kg. A large data set of kinematic and dynamic information for the full-scale pedestrian impact tests was reported, which provides an opportunity to access the biofidelity of the existing PFEM with consideration of pre-impact posture.
The goal of this study was to evaluate the biofidelity of the THUMS PFEM (Ver 4.01) in a whole-body pedestrian impact condition using a well-characterized finite element model of a generic pedestrian buck. In this study, the capability of the THUMS PFEM to reproduce kinematics, including trajectories, linear velocities, angular velocities, and accelerations, was evaluated. Reaction forces at the front-end buck were examined, and injuries predicted by the models were compared with those of the target subjects.
Methods
Simulations conditions
The experimental data set consisted of 3 previously published PMHS pedestrian impact tests using a generic vehicle buck representing a typical compact sedan (Forman et al. Citation2015a, Citation2015b). The subjects were initially positioned in a nominal mid-gait stance with the right foot back and the left foot forward and the subjects were oriented such that they were struck laterally on the subject's right side, targeting the test guidelines described in SAE J2782 (Society of Automotive Engineers Citation2010). During each test, the generic pedestrian buck was accelerated to a target impact velocity of approximately 40 km/h, at which point it contacted the PMHS pedestrian. The biofidelity of the THUMS PFEM was evaluated with respect to data from these full-scale PMHS pedestrian impact tests by reconstructing the test conditions using the LS-DYNA solver (mpp971sR7.1.1, LSTC, Livermore, CA).
THUMS PFEM, scaling, and positioning
Though anthropometries of the subjects were close to a 50th percentile adult male (Table A1, see online supplement), the 50th percentile male THUMS PFEM was uniformly scaled to match the stature and total body weight of each target subject. The scaling process introduced a length scaling factor (λz) that permitted matching of the stature and another scaling factor (λx = λy) in the transverse plane to match the total body mass, as shown in Equations (Equation1(1)
(1) ) and (Equation2
(2)
(2) ). Through this process, the differences in anthropometry between the model and PMHS were mitigated while maintaining good mesh quality of the model.
(1)
(1)
(2)
(2)
In Equations (Equation1(1)
(1) ) and (Equation2
(2)
(2) ), λO, Z is the ratio between PMHS height and THUMS height, and λO, M is the ratio between PMHS mass and THUMS mass.
A 6-step positioning procedure requiring presimulations of the THUMS PFEM was performed to match the pre-impact posture of the scaled THUMS PFEM to that of the PMHS (). Using the Vicon kinematics data extracted at the time of the impact (t = 0), subject-specific pre-impact postures of the model were created rather than using an average posture (Figure A1, see online supplement). Care was taken to match the (Equation1(1)
(1) ) pelvis orientation, (Equation2
(2)
(2) ) knee position (Jani et al. Citation2012), (3) spine curvature (Poulard, Subit, et al. Citation2015), and (4) shrugged posture of the shoulders due to the manner in which the PMHS were supported in the tests (Forman et al. Citation2015a). For step (5), gravity was applied to settle the model on the ground and then step (6) included positioning the arm after binding the arms together. At the end of each presimulation, only the nodal coordinates of the model (excluding the posture apparatus) were exported for the next step. Lastly, the model was positioned based on the head position with respect to the buck in the transverse plane and based on the knee height with respect to the ground in vertical axis following the positioning procedures of the PMHS (Forman et al. Citation2015a). Following positioning of the model, impact simulations were initiated at the time of leg contact at a pre-impact speed of 40 km/h, striking the right-side of the model. Gravity was applied to the THUMS and buck models from the beginning of the simulation. The coefficient of friction between the THUMS model and the vehicle model was 0.3, and it was 0.6 between the feet and ground (Chen et al. Citation2015; Paas, Davidsson, and Brolin Citation2015; Watanabe et al. Citation2012).
Instrumentations
During the experiments, extensive kinematics information from the head to the lower extremities was captured with a 3D optoelectronic motion capture system (Vicon). Accelerations and angular velocities were measured at several anatomical locations (head, T1, pelvis, left and right femurs, and the left and right proximal tibias) using 6-degree-of-freedom sensor packages that included 3 orthogonal accelerometers and 3 orthogonal angular rate sensors. Three orthogonal accelerometers measured linear accelerations of T8 and the left and right distal tibias (LAnkle and RAnkle). The THUMS PFEM was modeled with accelerometers at the same anatomical locations as the PMHS to track the kinematics and equipped with injury criteria measurements like chest band instrumentation to predict injury. Reaction forces in the experiment were measured using the load cells between the frame and the frontal components of the buck (hood edge, grille, upper and lower bumpers) on both the driver side and the passenger side; in the simulation, joints at the same locations were defined to measure the reaction forces. The traces of position, acceleration, angular rates, and reaction forces in the simulation were outputted in the same local coordinate systems as reported in the PMHS tests (). Before the quantitative assessment, simulation data and experimental data were first sampled at the same frequency and digitally filtered using the same filter classes. The reaction force time histories up to 45 ms after leg contact were evaluated, and other kinematics responses of the model were evaluated up to 180 ms. The pre- and postprocessing work was done in LS-PREPOST (Ver 4.1, LSTC) and with scripts written in MATLAB (R2013b; The MathWorks Inc., Natick, MA).
International organization for standardization rating method
To quantitatively assess the correlation between the responses of the THUMS PFEM and PMHS, the International Organization for Standardization (ISO) objective rating method was employed (Barbat et al. Citation2013). This process consists of a cross-correlation method (magnitude coefficient, shape coefficient, phase coefficient) and a corridor method. The rating score was calculated by taking a weighted average of these 4 metrics. This rating method outputs a score ranging from 0 (no correlation) to 1 (perfect match).
Injury prediction
Injury outcomes in the PMHS tests were summarized based on posttest computed tomography scans and autopsy reports (Forman et al. Citation2015a). In the simulations, element erosion/elimination was not implemented but injuries were predicted in postprocessing using both probabilistic and deterministic assessment methods based on empirical injury risk functions and strain measures, respectively; these injury estimates were compared with the actual injuries of the target PMHS. The deterministic method (DM) tracked the maximum principal strain in an element from postprocessing to predict the cortical bone fractures and ligament failures. The thresholds were set to represent a population similar to the PMHS in terms of age (average 65 years old for 3 subjects) based on values extracted from the literature (Bass et al. Citation2007; Golman et al. Citation2014; Meyer et al. Citation2012; Mo et al. Citation2014; Quinn and Winkelstein Citation2007; Snedeker et al. Citation2003; Trajkovski et al. Citation2014; Yasuki and Yamamae Citation2010). Appendix B (see online supplement) provides additional information on both methods.
The probabilistic method (PM) assessed the risk of severe (Abbreviated Injury Scale [AIS] 3+) thoracic injury (Kent and Patrie Citation2005; Kuppa et al. Citation2003) and moderate (AIS 2+) head (Kuppa Citation2004), pelvis (Gunji et al. Citation2012), and lower extremities injuries (Banglmaier et al. Citation1999; Kerrigan et al. Citation2004) using empirical injury risk functions derived from PMHS test data. The age of the subject was not considered for assessing injury risk except for thorax, which includes age as a covariate in the injury risk function. The lateral bending angles (16°) and the shear displacement (14 mm) of the knee were used to assess the risk of a knee ligament rupture (Ivarsson et al. Citation2004; Li et al. Citation2015; Mo et al. Citation2014). The inversion/eversion bending angle and lower tibial axial load were used to predict risk of ankle injury (Funk et al. Citation2002), and the bending angle measured was defined as the rotation angle of the calcaneus relative to the tibia in the coronal plane.
Results
Evaluation of kinematic and kinetic responses
An overall comparison of the PMHS and THUMS PFEM kinematics is provided in , showing the video still captures for the V2371 case as an example. The same information for the other 2 simulations can be found in Appendix D (Figures A6–A11, see online supplement). The trajectories of the head, T1, T8, pelvis, right distal femur (RFemur) and right distal tibia (RAnkle), left distal femur (LFemur) and left distal tibia (LAnkle) in the Y–Z plane were overlaid with those measured with Vicon 3D motion capture in the buck fixed frame (; Figure A12, see online supplement). The head contact times in the simulation were 142, 147, and 144 ms after initial leg contact for the case of V2370, V2371, and V2374, respectively, which were delayed from 3 to 9 ms compared with thehead contact timing of the PMHS.
Figure 3. Pedestrian impact sequences of PMHS and THUMS for test V2371 (posterior view; Forman et al. Citation2015a).
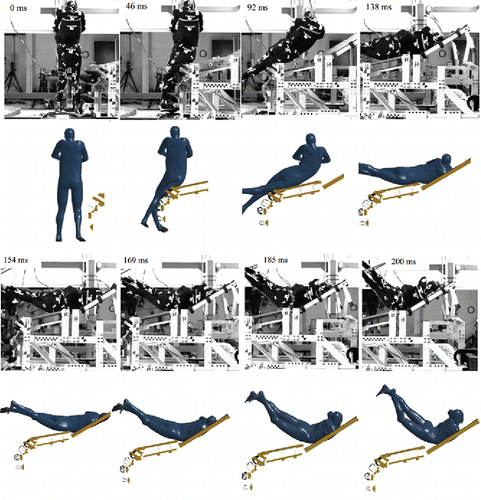
Figure 4. Trajectories in the buck reference frame; markers were displayed at every 30 ms from 0 ms (leg contact) to 120 ms. (The same information for the other 2 simulations can be found in Appendix D, Figure A12.)
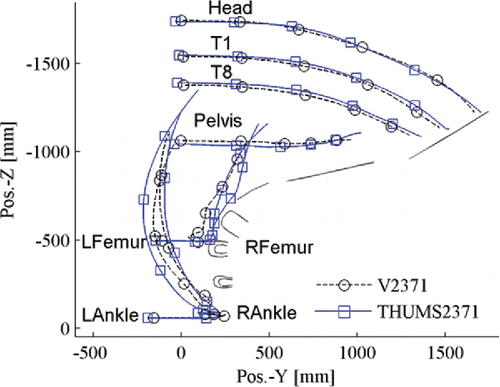
An overall quantitative comparison of the PMHS and THUMS PFEM responses is provided in Appendix D (Table A4, see online supplement). provides the average scores between the responses of the PMHS and the THUMS PFEM. Note that an equal weight was used to calculate the average ISO scores for each location and each test.
Summary of injury prediction
The injury predictions of the model are summarized in , compared with the injuries sustained in the PMHS tests. Please refer to Appendix D for more details.
Table 1. Comparison of the injury reported in experiments and predicted by model.Footnotea
Discussion
In this study, the THUMS PFEM was uniformly scaled to match the height and weight of the target subject, positioned to reproduce a pre-impact posture with the pedestrian struck by a well-characterized pedestrian buck model. The buck model used in the current study was previously evaluated against its mechanical counterparts at the component level by means of impactor tests (Pipkorn et al. Citation2014) and at the full-scale level in terms of the representativeness of car–pedestrian interactions (Takahashi et al. Citation2014). Good correspondence between the buck model and the mechanical buck was generally achieved; some discrepancies in the hood and windshield regions were found.
Instead of evaluating the standard PFEM (50th percentile male model) by comparing with normalized pedestrian experimental responses, we chose to evaluate the PFEM against individual responses by reconstructing the specific experiment scenarios with scaled PFEM representing specific PMHS. The correlation between the responses of the THUMS PFEM and the PMHS could be improved if a morphing technique rather than scaling were employed because the morphed THUMS PFEM has been shown to predict PMHS trajectories better than globally scaled THUMS PFEM (Poulard et al. Citation2016). The anthropometry of PMHS and THUMS PFEM was considered sufficiently similar that the scaling approach used in this study was deemed more efficient, and a good pre-impact posture was achieved based on the scaled THUMS PFEM.
Due to the availability of the limited experimental data, a single vehicle geometry (small sedan) and a single collision speed condition (40 km/h) were investigated in the current study. Different vehicle geometries and collision speeds would potentially generate substantially different kinematics and injury patterns due to the engagement of the various structures of the body. To be useful as a robust evaluation tool, PFEM should demonstrate biofidelic responses with a variety of vehicle types at a variety of collision speeds. Although a limited number of PMHS tests were considered in the current study, the PMHS exhibited consistent responses probably because of their similar anthropometries and the well-controlled test conditions. The capabilities of the THUMS PFEM in reproducing biomechanical responses for different PMHS are also similar, as evidenced by the similar ISO ratings between different cases for each location and each signal type.
In the 3 simulations, the THUMS PFEM generally demonstrated good agreement with the experimental data regarding kinematics under qualitative assessment (). The trajectories of the model and PMHS exhibited very good agreement, resulting in nearly identical upper body trajectories for each location in Y–Z plane (). The noticeable difference occurred in the timing of head contacts; more specifically, the simulation head contact times were delayed. Because the kinematics of the thoracic spine (T1 and T8) are more consistent with the response of the PMHS in terms of timing, the delayed head responses may indicate that the THUMS neck may lack biofidelity and may be softer than these PMHS necks in terms of lateral bending stiffness. However, the THUMS PFEM spine was previously found to be stiffer than the spine of volunteers by comparing with shoulder lateral impact tests (Paas, Davidsson, and Brolin Citation2015). Paas, Osth, and Davidsson (Citation2015) evaluated the THUMS PFEM whole-body kinematics against the PMHS test data and suggested softening the neck slightly in lateral bending. Because boundary conditions in those studies are different from the current study, the lateral bending stiffness of the THUMS PFEM neck or cervical spine needs further investigation. In addition to the neck responses, the trajectories for the leg and left ankle from the simulations differed from those of the PMHS. More specifically, the legs in the simulation were struck further away from the buck, and left ankles in the simulations were temporarily trapped between the lower bumper and the floor, and in some cases the left ankle in the experiments was trapped (see the 46 ms frame in ). Because the knee height of the model was matched to that of the PMHS, these discrepancies were not likely to be caused by the posture difference.
We investigated the influence of the initial contact force between the feet and the ground on pedestrian behavior. A simulation was performed with preloading (settling) the THUMS model under gravity for a certain amount of time (50 ms) before impact. The difference between the simulation results and the current results was minor. Although the biofidelity of the leg and ankle of the model needs further investigation, the differences in these regions may also be due to the fidelity of the buck model. Similar responses were found by Takahashi et al. (Citation2014), in which the same buck model was used to simulate a car–pedestrian lateral impact at 40 km/h using a human finite element (FE) model developed in PAM-CRASH (Engineering System International, Paris, France). The absence of consideration of tissue failure during the simulations in the current study may be a partial reason for the stiffer leg response of the model. Results from a preliminary simulation that considered the ligament failure at the knees showed that failure of the soft tissue reduced the kick-out distance of the legs (Figure A40, see online supplement). Thus, ligament failure would reduce the bending moment sustained by the thigh and leg (Figure A41, see online supplement). Nevertheless, activating tissue failure did not exhibit much effect on the pedestrian upper body kinematics. In this preliminary simulation, the thresholds for cruciate ligament rupture and collateral ligament rupture were respectively chosen as 24 and 28% ultimate strain (Mo et al. Citation2014), but ligament element elimination was not considered in the current study because the failure thresholds of the knee ligaments, which were represented by ultimate strain in the literature, ranges widely (7–60%; Arnoux et al. Citation2008).
The major components of the model responses (Y component for translation, X component for the rotation, and Z component for impact forces) demonstrated the highest ISO rating scores among the 3 components. Trajectories in the coronal plane (Y–Z plane) agreed well with the experimental measurements (Y = 0.90 ± 0.11, Z = 0.89 ± 0.09). Though out-of-plane movements scored poorly (X = 0.41 ± 0.16), the magnitudes of the motions were small both in the simulations and in the experiments. The resultant velocity time histories of the head, T1, T8, and the pelvis relative to the buck derived from displacement outputs in the simulations also matched well with those derived from Vicon motion capture in the experiments (0.83 ± 0.07). The acceleration responses of the model showed poor (R < 0.58) to fair (0.58 < R < 0.8) correlation with the experiment data (X = 0.47 ± 0.12; Y = 0.58 ± 0.11; Z = 0.52 ± 0.12). The angular velocities predicted by the model were not typically consistent with the PMHS responses, and quantitatively showed the lowest overall score among all types of signals assessed (X = 0.48 ± 0.13; Y = 0.34 ± 0.13; Z = 0.35 ± 0.12).
Though the use of a correlation method, such as ISO/TS 18571, facilitated an objective and quantitative evaluation of the model, a certain degree of caution is required when performing biofidelity assessment using an objective rating method. Due to the general shape of the responses depending on its type, the ISO rating scores of trajectories exhibited the highest values among the responses. In addition, the choice of the interval of the signal chosen for evaluation can influence the ISO scores. For example, the reaction force signals in these impact scenarios were truncated just prior to the vehicle sled contacting the pedestrian sled (around 45 ms) to avoid effects of acceleration due to contact vibration, and the majority of the lower limb contact event had already finished by the time of 45 ms. Thus, later signals were not included in the evaluation interval. Otherwise, the rating scores would be skewed and would not provide a quantitative assessment representative of the interested signals. Though the averaged ISO rating scores were provided for each type of signal (), these results should be considered only one of the indicators rather than sole criterion for the biofidelity of the model.
The availability of the buck reaction forces facilitated the biofidelity evaluation of the PFEM. Measured interaction forces were the greatest at the upper bumper. The upper bumper force predicted during the simulation showed good correlation with the force measured in the PMHS tests. The large impact forces at the leading edge of the hood observed during the tests were poorly reproduced by the simulations. The leading edge of the hood experienced forces when it impacted the pelvis and thigh regions. Such discrepancies in kinetics will influence the kinematic and injury outcome of the model. Although evaluation of the human model using the generic buck model eliminated some uncertainties from the vehicle buck model, the characteristics of the current buck model in some local regions—for example, the hood stiffness—may need improvement. Previously, the hood region in the buck model was found to be softer than that of the mechanical buck (Pipkorn et al. Citation2014). The upper bumper was the initial source of contact between the buck and the subject, and good agreement in upper bumper forces between the PMHS and the model (R = 0.81 on average) indicates that this model could be used in the development of the pedestrian to vehicle impact sensing systems.
Both injury prediction methods captured injury outcomes to some extent. Though the probabilistic method was generally consistent with the injuries that the PMHS sustained, it overestimated the risk of head and the ankle injuries and underestimated the risk of pelvic fractures in one case (V2374). The injury prediction for the left-side chest by the model was less consistent with the injury outcomes of the PMHS. Though the model showed good correlation for the linear velocity of the head with that of the PMHS, the model generally predicted higher head injury criterion (HIC) values than did the PMHS. The deterministic method correctly predicted knee ligament ruptures and pelvis and femoral fractures but overestimated tibia fractures and ankle ligament injury and underestimated the number of rib fractures. Consistent with the experiment, some rib fractures with the similar fracture location (rib 2 and rib 3), neck injuries, and lumbar spine injuries were reproduced using the deterministic method. The deterministic method predicts injury further to the more specific location and provides injury references for body regions in which no injury risk functions are available, but it is highly dependent on injury thresholds. Though the choice of injury prediction methodology should be considered based on the goals of the simulation and the expected injury severity, this is beyond the scope of this study and was not investigated.
The current study evaluated the biofidelity of the THUMS PFEM by reconstructing the specific PMHS whole-body pedestrian impact conditions using a well-characterized generic pedestrian buck model. Though the major components of the model responses demonstrated good agreement with the experimental data under qualitative and quantitative assessment, potential biofidelity issues in the regions below the knee and in the neck were also found. Reaction forces in the upper bumper were correctly predicted in the simulations. Based on the THUMS PFEM simulation results, both the probabilistic and deterministic injury prediction methods captured injury outcomes to some extent. Given the capability of the model in reproducing biomechanical responses, the THUMS PFEM could be a valuable tool for developing novel pedestrian safety systems.
Funding
The authors acknowledge Autoliv Research for sponsorship of the research in this article.
Online_Supplement_Appendix.docx
Download MS Word (14.3 MB)Acknowledgments
The opinions expressed here are solely those of the authors. We appreciate Tim Gillispie for his thoughtful and thorough review of this article. The pedestrian buck design used in this project is jointly developed and owned by Honda R&D Co., Ltd., and Autoliv Development AB.
References
- Arnoux PJ, Behr M, Llari M, Thollon L, Brunet C. Injury criteria implementation and evaluation in FE models applications to lower limb segments. Int J Crashworthiness. 2008;13:653–665.
- Banglmaier RF, Dvoracek-Driskna D, Oniang'o TE, Haut RC. Axial Compressive Load Response of the 90 Degrees Flexed Human Tibiofemoral Joint. 1999. SAE Technical Paper No. 99SC08. Warrendale, PA: SAE International.
- Barbat S, Fu Y, Zhan Z, Yang RJ, Gehre C. Objective rating metric for dynamic systems. Paper presented at: International Technical Conference on the Enhanced Safety of Vehicles (ESV); May 27–30, 2013; Seoul, Republic of Korea.
- Bass CR, Lucas SR, Salzar RS, et al. Failure properties of cervical spinal ligaments under fast strain rate deformations. Spine. 2007;32:E7–E13.
- Chen H, Poulard D, Crandall JR, Panzer MB. Pedestrian response with different initial positions during impact with a mid-sized sedan. Paper presented at: 24th International Technical Conference on the Enhanced Safety of Vehicles (ESV); June 8–11, 2015; Gothenburg, Sweden.
- Forman JL, Joodaki H, Forghani A, et al. Biofidelity corridors for whole-body pedestrian impact with a generic buck. Paper presented at: International Research Council on the Biomechanics of Injury (IRCOBI) Conference; September 9–11, 2015a; Lyon, France.
- Forman JL, Joodaki H, Forghani A, et al. Whole-body response for pedestrian impact with a generic sedan buck. Stapp Car Crash J. 2015b;59:401–444.
- Funk JR, Srinivasan S, Crandall JR, et al. The effects of axial preload and dorsiflexion on the tolerance of the ankle/subtalar joint to dynamic inversion and eversion. Stapp Car Crash J. 2002;46:245–265.
- Golman AJ, Danelson KA, Miller LE, Stitzel JD. Injury prediction in a side impact crash using human body model simulation. Accid Anal Prev. 2014;64:1–8.
- Gunji Y, Okamoto M, Takahashi Y. Examination of human body mass influence on pedestrian pelvis injury prediction using a human FE model. Paper presented at: International Research Council on the Biomechanics of Impact (IRCOBI) Conference; September 12–14, 2012; Dublin, Ireland.
- Ivarsson BJ, Lessley DJ, Kerrigan JR, et al. Dynamic response corridors and injury thresholds of the pedestrian lower extremities. Paper presented at: International Research Council on the Biomechanics of Impact (IRCOBI) Conference; September 22–24, 2004; Graz, Austria.
- Iwamoto M, Kisanuki Y, Watanabe I, Furusu K, Miki K, Hasegawa J. Development of a finite element model of the total human model for safety (THUMS) and application to injury reconstruction. Paper presented at: International Research Council on the Biomechanics of Impact (IRCOBI) Conference; September 18–20, 2002; Munich, Germany.
- Jani D, Chawla A, Mukherjee S, Goyal R, Vusirikala N, Jayaraman S. Repositioning the knee joint in human body FE models using a graphics-based technique. Traffic Inj Prev. 2012;13:640–649.
- Kent RW, Patrie J. Chest deflection tolerance to blunt anterior loading is sensitive to age but not to load distribution. Forensic Sci Int. 2005;149(2–3):121–128.
- Kerrigan JR, Drinkwater DC, Kam CY, et al. Tolerance of the human leg and thigh in dynamic latero-medial bending. Int J Crashworthiness. 2004;9:607–623.
- Kuppa S. Injury Criteria for Side Impact Dummies. Washington, DC: NHTSA; 2004. Report No. DOT 67.
- Kuppa S, Eppinger RH, McKoy F, Nguyen T, Pintar FA, Yoganandan N. Development of side impact thoracic injury criteria and their application to the modified Es-2 dummy with rib extensions (Es-2Re). Stapp Car Crash J. 2003;47:189–210.
- Li G, Yang J, Simms C. The influence of gait stance on pedestrian lower limb injury risk. Accid Anal Prev. 2015;85:83–92.
- Maeno T, Hasegawa J. Development of a finite element model of the total human model for safety (THUMS) and application to car–pedestrian impacts. Paper presented at: International Technical Conference on the Enhanced Safety of Vehicles (ESV) Conference; June 4–7, 2001; Amsterdam, The Netherlands.
- Meyer E, Wei F, Button K, Powell J, Haut R. Determination of ligament strain during high ankle sprains due to excessive external foot rotation in sports. Paper presented at: International Research Council on the Biomechanics of Impact (IRCOBI) Conference; September 12–14, 2012; Dublin, Ireland.
- Mo FH, Arnoux PJ, Cesari D, Masson C. Investigation of the injury threshold of knee ligaments by the parametric study of car–pedestrian impact conditions. Saf Sci. 2014;62:58–67.
- Paas R, Davidsson J, Brolin K. Head kinematics and shoulder biomechanics in shoulder impacts similar to pedestrian crashes—a THUMS study. Traffic Inj Prev. 2015;16:498–506.
- Paas R, Osth J, Davidsson J. Which pragmatic finite element human body model scaling technique can most accurately predict head impact conditions in pedestrian–car crashes? Paper presented at: International Research Council on the Biomechanics of Impact (IRCOBI) Conference; September 9–11, 2015; Lyon, France.
- Pipkorn B, Forsberg C, Takahashi Y, et al. Development and component validation of a generic vehicle front buck for pedestrian impact evaluation. Paper presented at: International Research Council on the Biomechanics of Impact (IRCOBI) Conference; September 10–12, 2014; Berlin, Germany.
- Poulard D, Chen H, Crandall JR, Dziewonski T, Pedzisz M, Panzer MB. Component-level biofidelity assessment of morphed pedestrian finite element models. Paper presented at: International Research Council on the Biomechanics of Injury (IRCOBI) Conference; September 9–11, 2015; Lyon, France.
- Poulard D, Chen H, Panzer MB. Geometrical Personalization of Pedestrian Finite Element Models Using Morphing Increases the Biofidelity of Their Impact Kinematics. 2016. SAE Technical Paper No. 2016-01-1506. Warrendale, PA: SAE International.
- Poulard D, Subit DL, Donlon JP, Kent RW. Development of a computational framework to adjust the pre-impact spine posture of a whole-body model based on cadaver tests data. J Biomech. 2015;48:636–643.
- Quinn KP, Winkelstein BA. Cervical facet capsular ligament yield defines the threshold for injury and persistent joint-mediated neck pain. J Biomech. 2007;40:2299–2306.
- Snedeker JG, Muser MH, Walz FH. Assessment of pelvis and upper leg injury risk in car–pedestrian collisions: comparison of accident statistics, impactor tests and a human body finite element model. Stapp Car Crash J. 2003;47:437–457.
- Society of Automotive Engineers, Human Biomechanics and Simulations Standards Steering Committee. Performance Specifications for a Midsize Male Pedestrian Research Dummy. 2010. Surface Vehicle Information Report J2782. Warrendale, PA: SAE International.
- Subit DL, Kerrigan JR, Crandall JR, et al. Pedestrian–vehicle interaction kinematics and injury analysis of four full scale tests. Paper presented at: International Research Council on the Biomechanics of Impact (IRCOBI) Conference; September 17–19, 2008; Bern, Switzerland.
- Takahashi Y, Ikeda M, Asanuma H, et al. Full-scale validation of a generic buck for pedestrian impact simulation. Paper presented at: International Research Council on the Biomechanics of Impact (IRCOBI) Conference; September 10–12, 2014; Berlin, Germany.
- Tierney GJ, Joodaki H, Krosshaug T, Forman J, Crandall JR, Simms CK. Assessment of model-based image-matching for future reconstruction of unhelmeted sport head impact kinematics. Sports Biomech. 2017.
- Trajkovski A, Omerovic S, Hribernik M, Prebil I. Failure properties and damage of cervical spine ligaments, experiments and modeling. J Biomech Eng. 2014;031002-031002-9.
- Untaroiu CD, Shin J, Ivarsson BJ, et al. A study of the pedestrian impact kinematics using finite element dummy models: the corridors and dimensional analysis scaling of upper-body trajectories. Int J Crashworthiness. 2008;13:469–478.
- Watanabe R, Katsuhara T, Miyazaki H, Kitagawa Y, Yasuki T. Research of the relationship of pedestrian injury to collision speed, car-type, impact location and pedestrian sizes using human FE model (THUMS version 4). Stapp Car Crash J. 2012;56:269–321.
- World Health Organization. Global Status Report on Road Safety 2013: Supporting a Decade of Action. Luxembourg: World Health Organization Press; 2013.
- Yasuki T, Yamamae Y. Validation of kinematics and lower extremity injuries estimated by total human model for safety in SUV to pedestrian impact test. J Biomech Sci Eng. 2010;5:340–356.