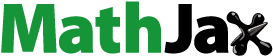
Abstract
This quasi-experimental study investigates the effects of using an electronic development portfolio (PERFLECT) with teacher-guided student self-coaching on the development of students' self-directed learning skills and motivation. In a 12-week program in senior vocational education, students in the PERFLECT group used the portfolio to help self-direct their learning, while the REGULAR group followed the regular educational program. Students in the PERFLECT group reported higher levels of self-direction, intrinsic goal orientation, and self-efficacy than students in the REGULAR group. Using an electronic development portfolio with a student self-coaching protocol constitutes a promising approach to support students' self-directed learning skills and motivation.
Introduction
Competency-based education is widely implemented within vocational education and training (VET). Research on the subject dates back to the 1960s and 1970s (e.g., Grant, Citation1979). Competency-based education is aimed at instructing students to acquire competences (integrations of knowledge, skills, and attitudes) that are derived from actual roles in the workplace or society. Often learning in competency-based curricula occurs by solving real-world problems. This form of education has gained popularity because it is expected to reduce the gap between educational practice and the labor market (Biemans et al., Citation2004) and lead to the development of competencies that can be applied across various contexts (Wesselink et al., Citation2007). In competency-based education students are often given more responsibility for their own learning, for example, by giving them more control over their individual learning trajectories. Such increased control over learning likely motivates students through increased autonomy (e.g., Schnackenberg & Sullivan, Citation2000; Vansteenkiste et al., Citation2008) and gives them the opportunity to develop skills for self-directed learning (SDL; e.g., Knowles, Citation1975).
Support and guidance within SDL
Over the past few decades, the importance of well-developed SDL skills has been emphasized because these skills are deemed to be essential for lifelong learning (e.g., Bolhuis, Citation2003). While SDL is not a clear-cut concept (i.e., many different definitions and closely related concepts exist), most conceptualizations share some form of self-assessment, learning-needs diagnosis and formulation of learning goals, and selection of learning tasks (e.g., Boekaerts & Corno, Citation2005; Paris & Paris, Citation2001; Ziegler et al., Citation2011). These SDL skills do not come naturally; without training, people are notoriously bad at self-assessing their own performance (e.g., Carter & Dunning, Citation2008; Langendyk, Citation2006; Kruger & Dunning, Citation1999) and selecting learning tasks that fit their learning needs (e.g., Azevedo et al., Citation2008; Stone, Citation1994). Fortunately, research shows that the development of SDL skills can be fostered by proper training (e.g., Kostons et al., Citation2012; Roll et al., Citation2011).
While competency-based education can contribute to students’ development of SDL skills, it is essential that training programs also include guidance and support to help the student acquire these skills. This is consistent with sociocognitive learning theories that describe a gradual transition of responsibility for learning from teacher to student (Schunk and Zimmerman, Citation1997; Winne & Hadwin, Citation1998; Zimmerman, Citation1989). However, transfer from teacher-directed to student-directed learning does not occur automatically (Agricola et al., Citation2020).The transition of responsibility for learning is usually supported by instructional scaffolding techniques such as modeling, coaching, and the use of learning tasks that are sequenced from simple to complex. While these techniques are aimed at supporting the development of domain-specific skills, they can also be aimed at supporting the development of SDL skills. This is a process known as “second-order scaffolding” (Van Merriënboer & Kirschner, Citation2018). Second-order scaffolding supports students with self-assessment of performance on learning tasks, formulation of points for improvement (PfIs), and selection of future learning tasks. This support is provided by an intelligent agent (e.g., a teacher or a computer algorithm) that helps to determine the complexity of learning tasks and amount of self-direction that is needed. For example, students can be provided with concrete performance standards to help them with self-assessment, or the number of learning tasks students can choose from can be limited to help them select appropriate future learning tasks. Indeed, recent research shows promising results regarding successfully promoting SDL with intelligent tools (e.g., Bannert et al., Citation2015; Järvelä et al., Citation2015; Lai & Hwang, Citation2016).
Portfolio usage and SDL
Often, competency-based education includes a portfolio aimed at supporting the learning process. These kinds of portfolios are tools that help students document and reflect on their learning process. Research shows that portfolios used to support the learning process can be used successfully to support the acquisition of SDL skills (Alexiou & Paraskeva, Citation2020; Barbera, Citation2009; Cheng & Chau, Citation2013; Kicken et al., Citation2009a). One type of portfolio that is used to support learning is the development portfolio. This kind of portfolio has embedded features that are explicitly designed for second-order scaffolding. Among others, these include overviews of past performance on learning tasks, a preselection of suitable learning tasks, and advice on how to formulate attainable learning goals.
The use of development portfolios has been linked to various positive results regarding SDL outcomes. Among others, it has been associated with improved learning-task selection and improved learning-plan formulation skills (Kicken et al., Citation2009b). The use of development portfolios has also been associated with increased levels of student motivation (Abrami et al., Citation2013). However, effective portfolio use can only be ensured under certain conditions. For example, it is very important that portfolio use is integrated into existing educational activities; it should not be placed in the periphery (e.g., Meyer et al., Citation2010). Furthermore, a portfolio should be used not only as a formative assessment instrument, but also in a summative sense to ensure that students take it seriously (Driessen et al., Citation2005), although it has to be noted that combining purposes may be complex (Heeneman & Driessen, Citation2017). Perhaps reported on the most consistently in the literature is the importance of complementing portfolio use with regular coaching sessions (e.g., Driessen et al., Citation2007; Dornan et al., Citation2003; Dannefer & Henson, Citation2007; Morales et al., Citation2016).
The role of coaching with portfolio usage
Regular coaching sessions are an essential part of the aforementioned second-order scaffolding. In these sessions, students seek to discuss the direction of their learning paths with their coach by attempting to answer the following important feedback questions: “Where am I going?,” “How am I going?,” and “Where to next?” (Hattie & Timperley, Citation2007). The coach helps students to answer these questions by offering them advice on how to effectively self-direct their learning on specific learning tasks. This advice may pertain to self-assessment of performance on learning tasks, formulation of PfIs, or selection of future learning tasks. Coaches can, for example, help students self-assess their performance on learning tasks more accurately by asking them critical questions about their assessment (e.g., “Why do you think you have mastered this skill?”). They can advise students on the quality of formulated PfIs (e.g., “Please make sure to specify how you aim to improve your performance on this learning task”), and they can offer students advice on the appropriateness of selected learning tasks (e.g., “Why do you think this task is right for you at this point in time?”).
While coaching is an essential part of second-order scaffolding, it is a very time- and energy-consuming process. Electronic variants of development portfolios may play a promising role in reducing time and energy expenditure on the side of the coach. These electronic development portfolios can automate certain administrative aspects of paper-based development portfolios (e.g., automatically adding up performance scores), but perhaps more interestingly, they can take on the role of a virtual coach by emulating the role a real-life coach fulfills in coaching sessions. In fact, the available research in this area demonstrates that parts of the regular coaching role can be emulated by intelligent software, such as Metatutor (Azevedo et al., Citation2010) or Atgentschool (Molenaar et al., Citation2011). One way to emulate the teacher’s coaching role is by integrating routine coaching questions (e.g., “What aspects of this learning task have you completed well?”) into a student self-coaching protocol. It is important to investigate whether such a student self-coaching protocol can also effectively emulate the teacher coaching role. In other words: Can an electronic development portfolio with teacher-guided student self-coaching protocol facilitate the development of SDL skills and positively influence students’ motivation?
It is both theoretically and practically urgent to explore how teachers’ workload, due to coaching students, can be alleviated somewhat. As mentioned previously, coaching often places a big burden on teachers, a burden that may be too heavy to bear in light of research on their workload (e.g., Ballet & Kelchtermans, Citation2009; Kim, Citation2019). Using smart technology to lighten that workload may make a big practical difference. On the theoretical side, the field of technology-supported SDL has seen a surge of research focusing on how technology can adaptively support learners’ individual needs (e.g., Chou et al., Citation2018; Molenaar et al., Citation2021; Taub & Azevedo, Citation2019; Su, Citation2020). The current research aims to add to that field by exploring how to optimize students’ preparation for coaching sessions by using a self-coaching protocol.
The following sections describe a quasi-experimental study that is aimed at investigating the effects of using an electronic development portfolio with teacher-guided student self-coaching on the development of students’ SDL skills and motivation. The main theme guiding this study is the reduction of teacher coaching by substituting part of it with self-coaching. Both forms of coaching are aimed at offering some form of second-order scaffolding, as they both support the development of SDL with the use of an e-portfolio. Although we do know that “full” teacher coaching can effectively support aforementioned development, it is not yet clear whether a combination of diminished teacher coaching and self-coaching are able to achieve similar effects. As such, this study is aimed at probing that effect. To do so, the study includes a comparison of students who follow the regular educational program while using the portfolio including teacher-guided student self-coaching (the PERFLECT group) and students who only follow the regular educational program without additional support (the REGULAR group). In the context of senior vocational education, we aim to answer four research questions:
Do students in the PERFLECT group demonstrate gaining more SDL skills compared to students in the REGULAR group?
Do students in the PERFLECT group demonstrate a significant increase of motivation compared to students in the REGULAR group?
How do students’ SDL skills develop in the PERFLECT group during the intervention?
Are students in the PERFLECT group more satisfied about portfolio-supported self-coaching than teacher coaching regarding facilitation of development of SDL skills?
In relation to the first research question, we hypothesize that the PERFLECT group will demonstrate increased SDL skills compared to the REGULAR group. In relation to the second research question, we hypothesize that the PERFLECT group will demonstrate increased motivation compared to the REGULAR group. Pertaining to our third research question, we expect students in the PERFLECT group to demonstrate development of their SDL skills over the intervention period (i.e., we expect an increase in SDL skill scores). Regarding the final research question, we expect students in the PERFLECT group to be more satisfied about teacher coaching than about self-coaching, because teachers can help students with nonroutine questions, while student self-coaching cannot.
Method
Participants
Fifty-four students from two technical programs of a school for senior vocational education in the southern part of the Netherlands participated in our study. The sample included 52 males and 2 females with an average age of 20.0 years (SD = 1.7). They were students of either “middle management engineering” or “middle management functionary building.” All students were in their third or fourth year of these four-year programs. Existing class structures (i.e., no randomization was applied) were used to assign students to the PERFLECT group (n = 25) and the REGULAR group (n = 29). Students in the PERFLECT group used the portfolio and engaged in teacher-guided self-coaching, while the REGULAR group followed the regular educational program without the portfolio and associated teacher-guided self-coaching. The composition of the PERFLECT group was comparable to that of the REGULAR group. Both groups largely consisted of males in similar types of education (both technical). Furthermore, students in both groups had progressed to roughly the same point in their education and shared the same teachers. Four teachers participated in this study.
Context
To facilitate students' development of SDL skills, an electronic development portfolio was designed and implemented (PERFLECT; Beckers et al., Citation2019 ). PERFLECT facilitates students' development of SDL skills with three main functionalities: (a) supporting self-assessment of performance on learning tasks, (b) formulation of points for improvement (PfIs), and (c) selection of future learning tasks.
To self-assess performance on a learning task in PERFLECT, students start by filling out details about the learning task. Following this, students are asked to select assessment criteria they think are suitable for self-assessment of their performance on the learning task. These criteria are derived from “qualification dossiers” that describe all relevant assessment criteria for a certain educational program (e.g., store manager) on three different levels of specificity. At the most abstract level, “core tasks” describe units of assessment very broadly. These core tasks are somewhat similar to competencies. For example, one core task in an educational program for store managers is “You carry out company policy.” At the intermediate level, “working processes” describe all constituents of the core tasks (e.g., “You analyze and interpret sales numbers”). At the most concrete level, “performance criteria” describe all constituents of the working processes (e.g., “You explain the difference between predicted and actual sales numbers”). In the selection of assessment criteria is depicted. This figure shows two core tasks and their associated working processes. The associated working processes are depicted directly under the core tasks. Students can select as many core tasks and working processes for assessment as they deem relevant. This is done by placing a check in the adjacent checkbox. In the working process “You manage article presentations” is selected. Note that students need not select performance criteria for assessment. Performance criteria are automatically generated when students select core tasks and working processes.
Figure 1. A screenshot of the assessment criteria selection page. Here, students select the relevant assessment criteria for their self-assessment. Students can select core tasks and working processes. Performance criteria are automatically retrieved from these choices and shown on the subsequent page (see ).
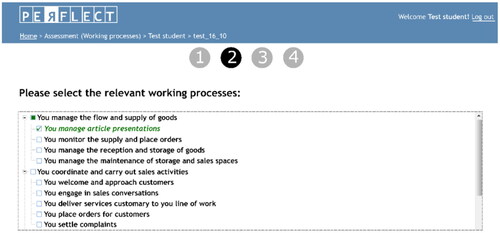
shows the third step of the assessment process. The student is expected to score each individual criterion with either U (unsatisfactory), Q (questionable), S (satisfactory), W (well), or N/A (not applicable). In the final step of this process students are asked to reflect on their performance, by answering eight leading questions (e.g., “What parts of the learning task have you completed well?”). depicts part of the student self-coaching protocol that contains leading questions to help students reflect on their learning-task performance in a structured manner (the first four questions are depicted).
Figure 2. A screenshot of the third step in the self-assessment process. In this step, students score performance criteria based on their previous selection of assessment criteria.
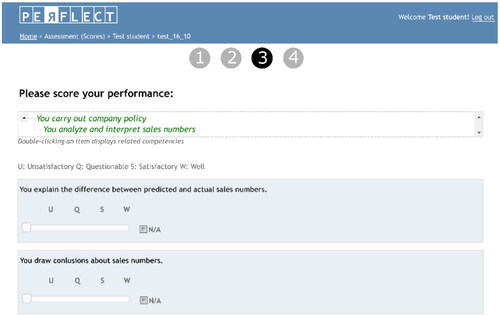
Figure 3. The student self-coaching protocol. This protocol contains leading questions that support students’ reflection on learning-task performance, such as “What parts of the learning task have you completed well?”
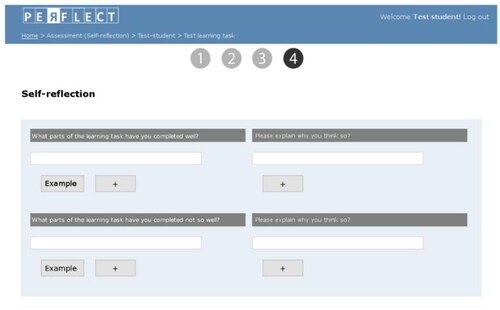
Formulation of PfIs is supported in PERFLECT as a part of the self-assessment. One leading question is specifically concerned with formulating PfIs (“Can you formulate a learning goal to improve your performance on the learning task?”). The answer to this leading question is automatically saved by PERFLECT as a PfI. Saved PfIs are later analyzed and discussed with a teacher, in coaching sessions designed to complement the use of PERFLECT.
Support of selection of future learning tasks is also implemented in the self-assessment in the form of two coaching questions. With these questions, students determine the appropriate difficulty and the level of support needed for the subsequent learning task. These choices are also discussed in the previously mentioned coaching sessions with the teacher.
Finally, all three functionalities are further supported in automatically generated performance overviews. Students and teachers can access overviews based on single learning tasks but also overviews with aggregated assessment information demonstrating progress over time. shows a performance overview with aggregated assessment information over time.
Figure 4. Progress over time for one student of middle management engineering on two core tasks (holistic units of assessment that are comparable to competences). Each line represents one core task. The graph displays various assessment data points that represent an aggregation of self-assessment and teacher assessment scores on a single learning task.
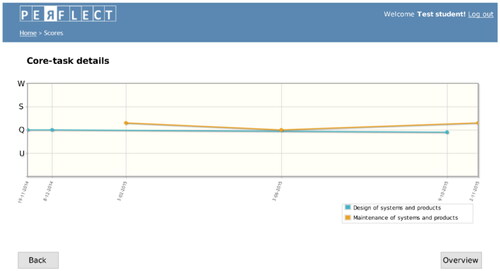
PERFLECT was integrated into the educational routines in several ways. First, students used PERFLECT to assess meaningful learning tasks (i.e., learning tasks were authentic and an integral part of the students’ training). Second, protected time was scheduled within regular lessons to self-assess learning tasks, but also to take part in coaching sessions. Third, PERFLECT was used to store all learning-task-related materials in one place. Finally, PERFLECT was used within the existing technological infrastructure.
Materials and measurement instruments
Coaching protocol
For students in the PERFLECT group a coaching protocol was used to ensure that all coaching sessions with the teacher proceeded in a structured fashion. Students in the REGULAR group did not participate in these sessions. The coaching protocol was designed to support self-assessment of performance on learning tasks, formulation of PfIs, and selection of future learning tasks. Each of the previous three SDL elements is supported by several questions. Self-assessment is supported by three questions (e.g., “Has the student selected the relevant core tasks and working processes?”). Formulation of PfIs is supported by four questions (e.g., “Do the formulated PfIs describe concrete behavior?”). Lastly, selection of future learning tasks is supported by two questions (e.g., “Is the student ready to execute a more difficult learning task?”).
Motivated strategies for learning questionnaire (RQ1 and RQ2)
To measure students’ level of self-directedness and motivation we used four sub-scales of the Motivated Strategies for Learning Questionnaire (MSLQ; Pintrich & de Groot, Citation1990). Specifically, we employed a version that was translated into Dutch and subsequently validated (Van den Boom et al., Citation2007). We used the subscale of Meta-Cognitive Self-Regulation (11 items, α = .71) to measure level of self-directedness. We used the subscales of Intrinsic Goal Orientation (three items, α = .47), Extrinsic Goal Orientation (four items, α = .65) and Self-Efficacy for Learning and Performance (eight items, α = .88) to measure level of motivation. Two items in the Meta-Cognitive Self-Regulation subscale and one item in the Intrinsic Goal Orientation scale were removed from the analysis because they displayed a very low (i.e., <.200) or a negative item-total correlation. Students were asked to indicate their level of agreement with the presented items in the subscales. All answers were recorded on a 7-point Likert scale, ranging from not at all true of me to very true of me.
Self-assessments and teacher assessments (RQ3)
All students’ self-assessments and teachers’ student assessments were logged. We measured the quality of the self-assessments by comparing them with teacher assessments. This comparison entailed determining to what extent students and teachers selected the same performance criteria to score the learning tasks and to what extent students and teachers scored these criteria similarly. To see whether there was improvement over time (i.e., a greater level of agreement between students and teachers) we compared the level of student–teacher agreement for the first half of the assessments with that of the second half of the assessments.
Formulated PfIs (RQ3)
During the self-assessment process, students’ formulated PfIs were also logged. The content of these PfIs was analyzed to see if formulated PfIs included an improvement goal, a method of improvement, and a condition under which improvement should occur. For example, the following PfI contains all three elements: “I need to build electric circuits faster (goal) under time pressure (condition) by making a plan upfront (method).” The presence of each of these elements adds one point to the total PFI quality score; as such, this score ranges from 0 to 3. Each PfI was independently coded by two members of the research team (interrater reliability κ = .85). To see whether there was improvement in quality of formulated PfIs, a linear regression analysis was conducted.
Semistructured interviews (RQ3)
Three semistructured interviews were conducted with the teachers (n = 4) involved in the study, as well as students (n = 7) from the PERFLECT group. The following main questions were explored: “How does using PERFLECT help to self-direct the learning process?” and “How did you experience working with PERFLECT?”
Evaluation questionnaire (RQ4)
We used an evaluation questionnaire to investigate students’ perceptions about both PERFLECT’s ability (including the student self-coaching protocol) and teachers’ ability to support self-assessment of performance on learning tasks (three items, e.g., “PERFLECT makes me reflect more on what aspects of the learning task I performed well”) and formulation of PfIs (one item, “Coaching sessions make me reflect more on what I need to improve about my performance on learning tasks”). Notice that ability to support future task selection was not measured. This was because students had little to no freedom in actually selecting learning tasks (i.e., a predefined lesson plan describing a homogeneous learning path for all students was in place). Students were asked to indicate their level of agreement with the presented items. All answers were recorded on a 5-point Likert scale, ranging from I fully disagree (1) to I fully agree (5).
Procedure
Preceding the intervention, participating students and teachers attended an introductory workshop about working with PERFLECT. For the students, the first part of the workshop was aimed at illustrating the benefits of working with PERFLECT and the second part of the workshop was aimed at instructing students about how to use the portfolio. It was at this time that the pretest (consisting of the MSLQ) was administered. The teachers attended a similar workshop with the addition of an explanation of what was expected from them during the coaching sessions.
The intervention consisted of a 12-week period in which students were asked to assess themselves weekly by filling out the self-assessment along with the student self-coaching protocol in PERFLECT. Teachers were asked to assess students’ performance on learning tasks once every two weeks; these were preferably learning tasks that students had already self-assessed. The information from both the teacher assessment and the student self-assessment was recorded in PERFLECT and discussed in the complementary teacher coaching sessions using the teacher coaching protocol. After each teacher coaching session, teachers were asked to send their students feedback. At the end of the 12-week period the posttest (consisting of the evaluation questionnaire and the MSLQ) was administered. Four weeks after the 12-week period the semistructured interviews were conducted (i.e., week 16).
Results
In the following sections we present student scores on the MSLQ, self-assessments and teacher assessments, formulated points for improvement (PfIs), and the evaluation questionnaire. First, we report MSLQ results to reveal any differences in students’ level of SDL and motivation on the posttest, between the REGULAR group and the PERFLECT group. Next, we present results pertaining to the evaluation questionnaire. Finally, we focus on self-assessment and teacher assessment scores and formulated PfIs to analyze changes over time in the development of SDL.
MSLQ
displays means and standard deviations for the MSLQ subscales of Meta-Cognitive Self-regulation and Self-Efficacy for Learning and Performance, for the pretest and the posttest of both the PERFLECT group and the REGULAR group. Mean scores on the Meta-Cognitive Self-regulation subscale (α = .62) range between 42.21 and 46.41, with a scale maximum of 63 (sum of 9 items, scale 1–7). Mean scores on the Self-Efficacy for Learning and Performance subscale (α = .85) range between 42.12 and 45.20, with a scale maximum of 56 (sum of 8 items, scale 1–7).
Table 1. Means and standard deviations for the MSLQ subscales of Meta-Cognitive Self-Regulation, Intrinsic Goal Orientation, Extrinsic Goal Orientation, and Self-Efficacy for Learning and Performance.
Analysis of covariance (ANCOVA) demonstrates that there was a significant effect of condition on posttest scores, after controlling for pretest scores on the MSLQ subscale of Meta-Cognitive Self-Regulation, F(1, 42) = 6.78, p = .013, = .145. The adjusted means of the PERFLECT group (M = 46.41, SE = 1.05) and the REGULAR group (M = 42.68, SE = 0.98) reveal that the PERFLECT group scored significantly higher on the posttest than the REGULAR group did. There was also a significant effect of condition on posttest scores, after controlling for pretest scores on the MSLQ subscale of Intrinsic Goal Orientation, F(1, 46) = 4.68, p = .036,
= .096. The adjusted means of the PERFLECT group (M = 12.40, SE = 0.29) and the REGULAR group (M = 11.50, SE = 0.29) reveal that the PERFLECT group scored significantly higher on the posttest than the REGULAR group did. There was no significant effect of condition on posttest scores, after controlling for pretest scores on the MSLQ subscale of Extrinsic Goal Orientation, F(1, 43) = 2.46, p = .125. Finally, ANCOVA also demonstrates that there was a significant effect of condition on posttest scores, after controlling for pretest scores on the MSLQ subscale of Self-Efficacy for Learning and Performance, F(1, 42) = 4.55, p = .039,
= .102. The adjusted means of the PERFLECT group (M = 45.20, SE = 1.00) and the REGULAR group (M = 42.12, SE = 1.03) reveal that the PERFLECT group scored significantly higher on the posttest than the REGULAR group did.
Self-assessments and teacher assessments
On average, students self-assessed between three and four learning tasks (M = 3.2, SD = 1.6) over the 12-week period. Their teachers assessed a little less than two learning tasks per student (M = 1.7, SD = 1.7). In total there were 29 learning tasks that were assessed by both teachers and students (note that some learning tasks were assessed by teachers but not by students and vice versa). When divided over two halves, the first half of the assessments includes 14 assessments in which students were assessed by their teacher for the first time. The second half includes 15 assessments in which students were assessed a second or a third time by their teacher.
displays agreement percentages between students’ self-assessments and those of their teachers on scored performance criteria for both time periods. In period 1, students and teachers scored criteria identically in 6% of the cases (i.e., the bold-only diagonal in ). In period 2, they scored criteria identically in 16% of the cases. This is a significant increase of 10 percentage points, χ2(1, 482) = 13.301, p = .000. In period 1, both students and teachers scored criteria as not applicable or did not score them at all in 18% of the cases (i.e., the bold italic number in ). In period 2, they selected not applicable or did not score them at all in 7% of the cases. This is a significant decrease of 11 percentage points, χ2(1, 482) = 12.403, p = .000. In period 1, students and teachers selected different criteria for most of the cases (69%, i.e., all non-boldfaced italic numbers in ). In period 2, they selected different criteria for 67% of the cases. The difference of 2 percentage points between period 1 and period 2 is not significant, χ2(1, 482) = .259, p = .611. In period 1, students overestimate their performance in 4% of the cases (i.e., the sum of the underlined numbers below the bold italic diagonal in ). In period 2, this is 5%; there is no significant difference between the two periods for overestimation percentage, χ2(1, 482) = 0.363, p = .547. Finally, students underestimate their performance in 5% of the cases in the first period (i.e., the sum of the underlined numbers above the bold italic diagonal in ). This was 5% in the second period; there is also no significant difference between the two periods for underestimation, χ2(1, 482) = 0.001, p = .981.
Table 2. Students’ self-assessment scores versus teacher assessment scores in period 1 and period 2 (as percentage of total).
Formulated PFIs
On average, students formulated a little over three PfIs (M = 3.3, SD = 1.6) in the 12-week period. After subtracting meaningless PfIs such as “N/A” and “Nothing,” students have formulated a little less than three meaningful PfIs (M = 2.7, SD = 1.5), which is about one PfI for every assessed learning task. The quality of formulated PfIs varied considerably, ranging from very broad keywords such as “Planning” to rather specific descriptions such as “I have to practice more with the software program ‘Solidworks’ to gain better control over it.”
In we present repeated measures of the quality and composition of the meaningfully formulated PfIs. For every trial (i.e., each instance a PfI is formulated) we report the number of formulated PfIs, composition scores (i.e., the percentage of PfIs that include goals, methods and conditions), and the average quality score of the formulated PfIs. Over time there is a substantial decline in the number of formulated PfIs. Although 20 PfIs were formulated in the first trial, this number drops to 3 in the last trial. Looking at the composition of formulated PfIs across trials, it is evident that most of these points include a method (a weighted average of 81%), much less include a goal (a weighted average of 34%), and even less include a condition (a weighted average of 15%). Quality of points for improvement can be described with the following regression equation: 0.991 + 0.102x (where x = trial number).
Table 3. The quality of students’ formulated PfIs over time/trials.
Semistructured interviews
The semistructured interviews explored two main questions. First, students and teachers were asked how using PERFLECT helped to self-direct the learning process. Generally speaking, teachers said that PERFLECT has helped them explicate previously implicit topics. Using PERFLECT helped teachers to discuss performance criteria out in the open, providing more insight into the students’ thought process, as captured by the following quote: “Actually, I liked experiencing their different point of view. I can learn from that. I liked it and I was curious as to what the students had written down” (T1). Furthermore, teachers pointed out that PERFLECT has helped them explicate discrepancies between their judgments of students’ performance and students’ own judgments of their performance. Confronting these discrepancies was seen as productive:
You had a kind of sudden awareness of how things were experienced by the students. When I assessed one their works, often we would not be on the same page. Then you see their opinion, it gives you something to reflect upon. (T2)
Second, teachers and students were asked how they experienced working with PERFLECT. Most of the conversation about the experiences students had with working with PERFLECT was geared toward points for improvement. The overall theme that emerged from the conversations with the students is centered around the static nature of PERFLECT, which did not allow for much autonomy. Four points of improvement were raised. First, students described the importance of integrating PERFLECT in their existing infrastructure. This was mainly pointed toward the software side (including systems such as N@tschool), but also toward the fact that students and teachers agree that PERFLECT would do better in a workplace setting where students experience authentic learning tasks. “It does not really fit. The only real place where it could fit, would be during the internships” (S2).
Teachers agreed that PERFLECT could have fit better into the organization. Especially, the rate of self-assessing performance on learning tasks was perceived as too high and sometimes trivial, because often there was not much to be assessed when students engaged in larger learning tasks.
Sometimes the rigidity of the system was not appreciated (e.g., not being able to skip filling out certain fields). At those times, students wanted to skip what felt like a pointless exercise to them. “Sometimes you just have fill out certain fields just to fill them out, that should not have to happen!” (S3).
Finally, students would appreciate less repetitiveness in the process. They claim that the tedious unchanging series of events led them to take the whole process less seriously.
Evaluation questionnaire
In we present student evaluation scores of both PERFLECT’s and teachers’ ability to facilitate the development of SDL skills. Only students in the PERFLECT group completed this questionnaire, because the REGULAR group did not work with PERFLECT, nor did they participate in the intervention coaching sessions with their teacher.
Table 4. Evaluation scores of students’ satisfaction with self-coaching and teacher coaching with respect to ability to facilitate development of SDL skills.
We present evaluation scores for self-assessment of performance (measured with three items) and formulation of PfIs (measured with one item). Individual item means range from 3.5 to 3.9, with a maximum of 5. Results show that students appreciated both PERFLECT’s ability to facilitate self-assessment of performance (M = 11.0, SD = 2.2, α = .87) and their teachers’ ability to do so (M = 10.8, SD = 2.4, α = .88). The evaluation scores for PERFLECT and the teachers did not differ significantly, t(23) = 0.266, p = .793. Additionally, students appreciated PERFLECT’s ability to facilitate the formulation of PfIs (M = 3.9, SD = 0.7), as well as their teachers’ ability to do so (M = 3.8, SD = 0.9). These evaluation scores also did not differ significantly, t(23) = −0.723, p = .477.
Discussion and conclusion
This study investigated the effects of using an electronic development portfolio with teacher-guided student self-coaching on the development of SDL skills and motivation. Regarding our first two research questions, we hypothesized that students who used PERFLECT with teacher-guided student self-coaching would demonstrate gaining more SDL skills and a significant increase of motivation compared to their peers who did not use PERFLECT. These hypotheses are confirmed. Students who used PERFLECT with teacher-guided student self-coaching reported gaining SDL skills as measured by the Meta-Cognitive Self-Regulation Scale in the MSLQ, while the other students did not. Regarding our second hypothesis, the PERFLECT students reported significantly increased intrinsic goal orientation and increased self-efficacy beliefs, compared to their peers who did not use PERFLECT. Interestingly, differences between the PERFLECT group and the REGULAR group were observed for intrinsic goal orientation, but not for extrinsic goal orientation. The most logical explanation for the increase in intrinsic goal orientation seems to be that students were given quite a lot of autonomy over the topic of their self-assessments and the discussion in subsequent coaching sessions. The absence of differences between the PERFLECT group and the REGULAR group for extrinsic goal orientation, might be explained by our attempts to avoid placing extra demands on students and overly structuring the PERFLECT workflow during our study.
Concerning our third hypothesis, we expected that PERFLECT students would display improvement of their SDL skills over time. This hypothesis is partly confirmed. Over time, students and teachers more often chose to score identical performance criteria, indicating that they increasingly perceived the same criteria as being important for assessment of the learning task. This might have to do with the fact that students and teachers claim that they explicitly discussed the students’ performance on learning tasks in the study period. Moreover, over time, they also agree more often on how these criteria should be scored. This suggests that students and teachers move toward each other in terms of scoring performance criteria when the same criteria are chosen. However, more often than not students and teachers disagree on what criteria should be used to assess performance on learning tasks. While this finding is interesting, it is unsurprising, because previous research already suggests that students need a lot of support with selection of relevant criteria for performance assessment (e.g., Fastré et al., Citation2014). Interestingly, occurrences of under- and overestimation of performance constitute a minority in our study. This is remarkable, because it is incongruent with the vast body of literature reporting student overconfidence as a serious problem (e.g., De Bruin & van Gog, Citation2012). This apparent lack of overconfidence might have occurred due to the nature of the self-assessments in this study. Most studies reporting overconfidence as a problem often measure it on a micro scale with relatively objective measures (e.g., by comparing students’ estimations of how many items they can remember correctly vs. how many items they have actually remembered correctly), whereas in this study overconfidence was measured with more subjective, holistic measures. This may have influenced the level of overconfidence.
In terms of the composition of the points for improvement (PfIs) that students formulate, students almost always include how they want to improve their performance. However, in the majority of cases they do not include what they want to improve. Moreover, hardly any PfI includes the conditions under which better performance should be achieved. Thus, while in general students improve their ability to formulate PfIs, they can greatly profit from coaching aimed at including what they want to improve and under which conditions they want to improve it, and not so much how they plan to do it.
Lastly, we expected students to be more satisfied about teacher coaching than self-coaching, regarding facilitation of SDL. This hypothesis was not confirmed. In this study, students were satisfied with both forms of coaching. Moreover, no difference was found in students’ satisfaction with the two forms of coaching. We expected a difference in satisfaction because student self-coaching was designed to handle routine aspects of coaching, so that teacher coaching could help students find answers to nonroutine questions. Possibly, teacher coaching also had to focus on routine aspects of coaching. Effectively developing a self-coaching routine takes time, which was limited in this study. Likely, teachers spent time supporting students’ internalization of the self-coaching routine, limiting the available time to focus on nonroutine aspects of coaching. However, self-coaching should probably be designed in such a way that students do not experience it as a routine; this might impact their motivation to use it in a productive manner.
Some limitations apply to our study. We employed a nonequivalent groups design (i.e., the existing class structures were used and the REGULAR group only participated in pre- and posttests). While this kind of design ensures high ecological validity, effects may be less attributable to the intervention. To reduce the risk of confounding due to the possibility of unequal groups, we took steps to minimize the risk. First, time on task was kept equal for the PERFLECT and the REGULAR group, by organizing the coaching sessions during class time. As such, the PERFLECT group did not receive more instruction than the REGULAR group and score differences between both groups cannot be attributed to differences in time spent on task. Second, to minimize the influence of pre-existing differences between the PERFLECT group and REGULAR group we used ANCOVA, which adjusts the treatment effect for pre-existing differences.
This study aimed to improve students’ SDL skills. It is well known that improving these skills takes time. To ensure robust effects, longer study periods are needed. Furthermore, SDL is a very broad construct that can be measured in very different ways. To ensure that our measurement correctly reflects the broad spectrum that SDL encompasses, it is necessary to compare results from our measures with a broad set of other measures. Finally, at this point in time it is hard to differentiate effects of the development portfolio from those of the coaching sessions. We suggest that future research focus on longitudinal study designs, employing additional SDL measures and adopting research designs that make it possible to differentiate effects of development portfolios from coaching sessions.
In this study we compared a condition of diminished teacher coaching with an added self-coaching condition to a condition with no teacher coaching. It might be argued that results of this study are predictable, because it is widely acknowledged that teacher coaching is essential to supporting the development of SDL. This argument would deny the influence of amount of teacher coaching and effectively restrict the influence of teacher coaching to a dichotomous nature. However, the literature suggests that the amount of coaching is an important factor in supporting the development of SDL (e.g., Kicken et al., Citation2009b). Our study is focused on substituting a portion of teacher coaching with self-coaching, effectively reducing the amount of teacher coaching. As such, we argue that the results in this study are less predictable than might be assumed and thus have added value. Exploring the nature of the relationship between the amount of coaching provided (by a teacher or another intelligent agent) and SDL gains achieved seems to be very practically relevant. If it were clear how much SDL gain is to be expected with a certain amount of coaching, this could inform policy regarding said coaching. If teachers’ resources are directed so that they are fit for purpose (e.g., expending a smaller amount of teacher coaching when smaller gains in SDL are sufficient, or when another intelligent agent can assume a part of the coaching role), this may help to alleviate teachers’ workload.
An interesting line of future research is situated in the field of metacognition and is concerned with judgments of learning (JOLs). These JOLs comprise students’ judgments about how much is learned from previously performed learning tasks. Koriat (Citation1997) states that at the heart of these JOLs lie several performance cues indirectly related to the strength of the memory trace of the performed learning tasks. Some cues are more reliable than others in predicting actual performance. Aspecific cues (e.g., JOLs based on a person’s belief about his general ability to execute similar learning tasks) are less effective than specific, performance-related cues (e.g., JOLs based on the use of objective performance standards) in helping predict how much is actually learned. In order to improve students’ self-assessment skills, future research could focus on integrating performance related cues into an e-portfolio.
Another valuable line of future inquiry is that of learning analytics (e.g., Van der Schaaf et al., Citation2017; Viberg et al., Citation2020). Through learning analytics large amounts of user-produced data can be transformed to provide useful personal learning opportunities. The system can, for example, analyze commonly made mistakes in the user-provided answers and offer suggestions for improvement based on input from other users. Applied to electronic development portfolios, it would be interesting to investigate whether learning analytics can also be used to scaffold students’ SDL skills.
In conclusion, the use of an electronic development portfolio with a student self-coaching protocol and teacher-guided student self-coaching is a promising approach to facilitate students’ development of SDL skills. The use of this approach leads to increased student levels of self-directedness and motivation. Moreover, students’ self-assessment accuracy improves, as well as the quality of their formulated PfIs. However, students must be supported with selecting suitable criteria for self-assessment. Using performance-related assessment cues and learning analytics, the use of electronic development portfolios can likely be made even more effective.
Disclosure statement
The authors report no conflict of interest.
Data availability statement
The data that support the findings of this study are available from the corresponding author, JB, upon reasonable request.
Additional information
Funding
Notes on contributors
Jorrick Beckers
Jorrick Beckers is an assistant professor at the Open University of the Netherlands. His research interests include self-directed learning and electronic development portfolios.
Diana Dolmans
Diana Dolmans is a full professor of Innovative Learning Arrangements at Maastricht University. She is the scientific director of the Dutch Interuniversity Center for Educational Sciences (ICO). Her research focuses on the key success factors of innovative curricula in higher education.
Jeroen van Merriënboer
Jeroen van Merriënboer is a full professor of Learning and Instruction at Maastricht University. He is the research program director of the Graduate School of Health Professions Education of Maastricht University. He developed the acclaimed 4C/ID instructional model.
References
- Abrami, P. C., Venkatesh, V., Meyer, E. J., & Wade, C. A. (2013). Using electronic portfolios to foster literacy and self-regulated learning skills in elementary students. Journal of Educational Psychology, 105(4), 1188–1209. https://doi.org/10.1037/a0032448
- Agricola, B. T., van der Schaaf, M. F., Prins, F. J., & van Tartwijk, J. (2020). Shifting Patterns in Co-regulation, Feedback Perception, and Motivation During Research Supervision Meetings. Scandinavian Journal of Educational Research, 64(7), 1030–1051. https://doi.org/10.1080/00313831.2019.1640283
- Alexiou, A., & Paraskeva, F. (2020). Being a student in the social media era: Exploring educational affordances of an ePortfolio for managing academic performance. The International Journal of Information and Learning Technology, 37(4), 121–138. https://doi.org/10.1108/IJILT-12-2019-0120
- Azevedo, R., Johnson, A., Chauncey, A., & Burkett, C. (2010). Self-regulated learning with metatutor: Advancing the science of learning with metacognitive tools. In M. S. Khine & I. M. Saleh (Eds.) New science of learning: Computers, cognition, and collaboration in education (pp. 225–247). Springer.
- Azevedo, R., Moos, D. C., Greene, J. A., Winters, F. I., & Cromley, J. G. (2008). Why is externally-facilitated regulated learning more effective than self-regulated learning with hypermedia? Educational Technology Research and Development, 56(1), 45–72. https://doi.org/10.1007/s11423-007-9067-0
- Ballet, K., & Kelchtermans, G. (2009). Struggling with workload: Primary teachers’ experience of intensification. Teaching and Teacher Education, 25(8), 1150–1157. https://doi.org/10.1016/j.tate.2009.02.012
- Bannert, M., Sonnenberg, C., Mengelkamp, C., & Pieger, E. (2015). Short- and long-term effects of students’ self-directed metacognitive prompts on navigation behavior and learning performance. Computers in Human Behavior, 52, 293–306. https://doi.org/10.1016/j.chb.2015.05.038
- Barbera, E. (2009). Mutual feedback in e-portfolio assessment: An approach to the netfolio system. British Journal of Educational Technology, 40(2), 342–357. https://doi.org/10.1111/j.1467-8535.2007.00803.x
- Beckers, J., Dolmans, D. H., & van Merriënboer, J. J. (2019). PERFLECT: Design and evaluation of an electronic development portfolio aimed at supporting self-directed learning. TechTrends, 63(4), 420–427. https://doi.org/10.1007/s11528-018-0354-x
- Biemans, H., Nieuwenhuis, L., Poell, R., Mulder, M., & Wesselink, R. (2004). Competence-based vet in the Netherlands: Background and pitfalls. Journal of Vocational Education & Training, 56(4), 523–538. https://doi.org/10.1080/13636820400200268
- Boekaerts, M., & Corno, L. (2005). Self-regulation in the classroom: A perspective on assessment and intervention. Applied Psychology, 54(2), 199–231. https://doi.org/10.1111/j.1464-0597.2005.00205.x
- Bolhuis, S. (2003). Towards process-oriented teaching for self-directed lifelong learning: A multidimensional perspective. Learning and Instruction, 13(3), 327–347. https://doi.org/10.1016/S0959-4752(02)00008-7
- Carter, T. J., & Dunning, D. (2008). Faulty self-assessment: Why evaluating one’s own competence is an intrinsically difficult task. Social and Personality Psychology Compass, 2(1), 346–360. https://doi.org/10.1111/j.1751-9004.2007.00031.x
- Chou, C. Y., Lai, K. R., Chao, P. Y., Tseng, S. F., & Liao, T. Y. (2018). A negotiation-based adaptive learning system for regulating help-seeking behaviors. Computers & Education, 126, 115–128. https://doi.org/10.1016/j.compedu.2018.07.010
- Cheng, G., & Chau, J. (2013). A study of the effects of goal orientation on the reflective ability of electronic portfolio users. The Internet and Higher Education, 16, 51–56. https://doi.org/10.1016/j.iheduc.2012.01.003
- Dannefer, E. F., & Henson, L. C. (2007). The portfolio approach to competency-based assessment at the Cleveland Clinic Lerner College of Medicine. Academic Medicine: Journal of the Association of American Medical Colleges, 82(5), 493–502. https://doi.org/10.1097/ACM.0b013e31803ead30
- De Bruin, A. B. H., & van Gog, T. (2012). Improving self-monitoring and self-regulation: From cognitive psychology to the classroom. Learning and Instruction, 22(4), 245–252. https://doi.org/10.1016/j.learninstruc.2012.01.003
- Dornan, T., Maredia, N., Hosie, L., Lee, C., & Stopford, A. (2003). A web-based presentation of an undergraduate clinical skills curriculum. Medical Education, 37(6), 500–508. https://doi.org/10.1046/j.1365-2923.2003.01531.x
- Driessen, E. W., Van Tartwijk, J., Overeem, K., Vermunt, J. D., & Van der Vleuten, C. P. M. (2005). Conditions for successful reflective use of portfolios in undergraduate medical education. Medical Education, 39(12), 1230–1235. https://doi.org/10.1111/j.1365-2929.2005.02337.x
- Driessen, E. W., van Tartwijk, J., van der Vleuten, C., & Wass, V. (2007). Portfolios in medical education: why do they meet with mixed success? A systematic review. Medical Education, 41(12), 1224–1233. https://doi.org/10.1111/j.1365-2923.2007.02944.x
- Fastré, G. M., van der Klink, M. R., Amsing-Smit, P., & van Merriënboer, J. J. (2014). Assessment criteria for competency-based education: A study in nursing education. Instructional Science, 42(6), 971–994. https://doi.org/10.1007/s11251-014-9326-5
- Grant, G. (1979). On competence: A critical analysis of competence-based reforms in higher education. Jossey-Bass.
- Hattie, J., & Timperley, H. (2007). The power of feedback. Review of Educational Research, 77(1), 81–112. https://doi.org/10.3102/003465430298487
- Heeneman, S., & Driessen, E. W. (2017). The use of a portfolio in postgraduate medical education–reflect, assess and account, one for each or all in one? GMS Journal for Medical Education, 34(5), 1–12. https://doi.org/10.3205/zma001134
- Järvelä, S., Kirschner, P. A., Panadero, E., Malmberg, J., Phielix, C., Jaspers, J., Koivuniemi, M., & Järvenoja, H. (2015). Enhancing socially shared regulation in collaborative learning groups: Designing for cscl regulation tools. Educational Technology Research and Development, 63(1), 125–142. https://doi.org/10.1007/s11423-014-9358-1
- Kicken, W., Brand-Gruwel, S., van Merriënboer, J. J. G., & Slot, W. (2009a). Design and evaluation of a development portfolio: How to improve students’ self-directed learning skills. Instructional Science, 37(5), 453–473. https://doi.org/10.1007/s11251-008-9058-5
- Kicken, W., Brand-Gruwel, S., van Merriënboer, J. J. G., & Slot, W. (2009b). The effects of portfolio-based advice on the development of self-directed learning skills in secondary vocational education. Educational Technology Research and Development, 57(4), 439–460. https://doi.org/10.1007/s11423-009-9111-3
- Kim, K. N. (2019). Teachers’ administrative workload crowding out instructional activities. Asia Pacific Journal of Education, 39(1), 31–49. https://doi.org/10.1080/02188791.2019.1572592
- Knowles, M. S. (1975). Self-directed learning: A guide for learners and teachers. Cambridge Adult Education.
- Koriat, A. (1997). Monitoring one’s own knowledge during study: A cue-utilization approach to judgments of learning. Journal of Experimental Psychology: General, 126(4), 349–370. https://doi.org/10.1037/0096-3445.126.4.349
- Kostons, D., Van Gog, T., & Paas, F. (2012). Training self-assessment and task-selection skills: A cognitive approach to improving self-regulated learning. Learning and Instruction, 22(2), 121–132. https://doi.org/10.1016/j.learninstruc.2011.08.004
- Kruger, J., & Dunning, D. (1999). Unskilled and unaware of it: How difficulties in recognizing one’s own incompetence lead to inflated self-assessments. Journal of Personality and Social Psychology, 77(6), 1121–1134. https://doi.org/10.1037/0022-3514.77.6.1121
- Lai, C.-L., & Hwang, G.-J. (2016). A self-regulated flipped classroom approach to improving students’ learning performance in a mathematics course. Computers & Education, 100, 126–140. https://doi.org/10.1016/j.compedu.2016.05.006
- Langendyk, V. (2006). Not knowing that they do not know: self-assessment accuracy of third-year medical students. Medical Education, 40(2), 173–179. https://doi.org/10.1111/j.1365-2929.2005.02372.x
- Meyer, E. J., Abrami, P. C., Wade, C. A., Aslan, O., & Deault, L. (2010). Improving literacy and metacognition with electronic portfolios: teaching and learning with ePEARL. Computers & Education, 55(1), 84–91. https://doi.org/10.1016/j.compedu.2009.12.005
- Molenaar, I., van Boxtel, C., Sleegers, P., & Roda, C. (2011). Attention management for self-regulated learning: AtGentSchool. In C. Roda (Ed.), Human attention in digital environments. (pp. 259–280). Cambridge University Press.
- Molenaar, I., Horvers, A., & Baker, R. S. (2021). What can moment-by-moment learning curves tell about students’ self-regulated learning? Learning and Instruction, 72, 101206. https://doi.org/10.1016/j.learninstruc.2019.05.003
- Morales, L., Soler-Domínguez, A., & Tarkovska, V. (2016). Self-regulated learning and the role of ePortfolios in business studies. Education and Information Technologies, 21(6), 1733–1751. https://doi.org/10.1007/s10639-015-9415-3
- Paris, S. G., & Paris, A. H. (2001). Classroom applications of research on self-regulated learning. Educational Psychologist, 36(2), 89–101. https://doi.org/10.1207/S15326985EP3602_4
- Pintrich, P. R., & de Groot, E. V. (1990). Motivational and self-regulated learning components of classroom academic-performance. Journal of Educational Psychology, 82(1), 33–40. https://doi.org/10.1037/0022-0663.82.1.33
- Roll, I., Aleven, V., McLaren, B. M., & Koedinger, K. R. (2011). Metacognitive practice makes perfect: Improving students’ self-assessment skills with an intelligent tutoring system. In G. Biswas, S. Bull, J. Kay, & A. Mitrovic (Eds.), Artificial intelligence in education. (p. 288–295). Springer.
- Schnackenberg, H. L., & Sullivan, H. J. (2000). Learner control over full and lean computer-based instruction under differing ability levels. Educational Technology Research and Development, 48(2), 19–35. https://doi.org/10.1007/BF02313399
- Schunk, D. H., & Zimmerman, B. J. (1997). Social origins of self-regulatory competence. Educational Psychologist, 32(4), 195–208. https://doi.org/10.1207/s15326985ep3204_1
- Stone, D. N. (1994). Overconfidence in initial self-efficacy judgments: Effects on decision processes and performance. Organizational Behavior and Human Decision Processes, 59(3), 452–474. https://doi.org/10.1006/obhd.1994.1069
- Su, J. M. (2020). A rule‐based self‐regulated learning assistance scheme to facilitate personalized learning with adaptive scaffoldings: A case study for learning computer software. Computer Applications in Engineering Education, 28(3), 536–555. https://doi.org/10.1002/cae.22222
- Taub, M., & Azevedo, R. (2019). How does prior knowledge influence eye fixations and sequences of cognitive and metacognitive SRL processes during learning with an Intelligent Tutoring System? International Journal of Artificial Intelligence in Education, 29(1), 1–28. https://doi.org/10.1007/s40593-018-0165-4
- Van den Boom, G., Paas, F., & van Merrienboer, J. J. G. (2007). Effects of elicited reflections combined with tutor or peer feedback on self-regulated learning and learning outcomes. Learning and Instruction, 17(5), 532–548. https://doi.org/10.1016/j.learninstruc.2007.09.003
- Van der Schaaf, M., Donkers, J., Slof, B., Moonen-van Loon, J., van Tartwijk, J., Driessen, E., Badii, A., Serban, O., & Ten Cate, O. (2017). Improving workplace-based assessment and feedback by an E-portfolio enhanced with learning analytics. Educational Technology Research and Development, 65(2), 359–380. https://doi.org/10.1007/s11423-016-9496-8
- Van Merriënboer, J. J. G., & Kirschner, P. A. (2018). Ten steps to complex learning. Routledge.
- Vansteenkiste, M., Ryan, R., & Deci, E. (2008). Self-determination theory and the explanatory role of psychological needs in human well-being. In L. Bruni, F. Comim, & M. Pugno (Eds.), Capabilities and happiness. (pp. 187–223). Oxford University Press.
- Viberg, O., Wasson, B., & Kukulska-Hulme, A. (2020). Mobile-assisted language learning through learning analytics for self-regulated learning (MALLAS): A conceptual framework. Australasian Journal of Educational Technology, 36(6), 34–52. https://doi.org/10.14742/ajet.6494
- Wesselink, R., Biemans, H. J., Mulder, M., & Van den Elsen, E. R. (2007). Competence-based vet as seen by Dutch researchers. European Journal of Vocational Training, 40(1), 38–51. https://doi.org/10.1080/13636820400200268
- Winne, P. H., & Hadwin, A. F. (1998). Studying as self-regulated learning. In D. J. Hacker & J. Dunlosky (Eds.), Metacognition in educational theory and practice. The educational psychology series (pp. 277–304). Erlbaum Associates Publishers.
- Ziegler, A., Stoeger, H., & Grassinger, R. (2011). Actiotope model and self-regulated learning. Psychological Test and Assessment Modeling, 53(1), 161–179.
- Zimmerman, B. J. (1989). A social cognitive view of self-regulated academic learning. Journal of Educational Psychology, 81(3), 329–339. https://doi.org/10.1037/0022-0663.81.3.329