Abstract
The Common European Framework of Reference (CEFR; CitationCouncil of Europe, 2001) provides a competency model that is increasingly used as a point of reference to compare language examinations. Nevertheless, aligning examinations to the CEFR proficiency levels remains a challenge. In this article, we propose a new, level-centered approach to designing and aligning writing tasks in line with the CEFR levels. Much work has been done on assessing writing via tasks spanning over several levels of proficiency but little research on a level-specific approach, where one task targets one specific proficiency level. In our study, situated in a large-scale assessment project where such a level-specific approach was employed, we investigate the influence of the design factors tasks, assessment criteria, raters, and student proficiency on the variability of ratings, using descriptive statistics, generalizability theory, and multifaceted Rasch modeling. Results show that the level-specific approach yields plausible inferences about task difficulty, rater harshness, rating criteria difficulty, and student distribution. Moreover, Rasch analyses show a high level of consistency between a priori task classifications in terms of CEFR levels and empirical task difficulty estimates. This allows for a test-centered approach to standard setting by suggesting empirically grounded cut-scores in line with the CEFR proficiency levels targeted by the tasks.
INTRODUCTION
Since its publication in 2001, the Common European Framework of Reference (CEFR; CitationCouncil of Europe 2001) has increasingly become a key reference document for language test developers who seek to gain widespread acceptance for their tests within Europe. The CEFR represents a synthesis of key aspects about second and foreign language learning, teaching, and assessment. It primarily serves as a consciousness-raising device for anyone working in these areas and as an instrument for the self-assessment of language ability via calibrated scales. In other words, it is not a how-to guide for developing language tests even though it can serve as a basis for such endeavors (see, e.g., CitationAlderson & Huhta, 2005, or CitationNorth, 2004). As a result, many test developers are unsure about how to use the information in the CEFR to design tests that are aligned with the CEFR, both in philosophy and practice. This article is situated within this broader context and aims to provide insight into the question how writing tasks can be aligned with the CEFR levels.
Of importance, writing ability is usually measured by using open tasks that can elicit a range of written responses. These, in turn, are generally scored by trained raters using a rating scale that covers several bands or levels of proficiency; we call this approach a multilevel approach. However, if one needs to determine whether a student has reached one specific level, it is worth exploring an approach in which tasks are used that are each targeted at one specific level; the written responses of the students are then assessed by having trained raters assign a fail/pass rating using level-specific rating instruments; we call this a level-specific approach.
This is the task development and rating approach that is taken in this study. Specifically the level-specific rating approach constitutes a rather novel approach, especially within the context of test development in line with the CEFR levels. The alignment process described in the Manual for Relating Examinations to the CEFR (CitationCouncil of Europe, 2009) encompasses several steps, one of which is the “specification” stage focusing on task characterization as a prerequisite for the formal standard setting itself. Although this stage focuses on tasks, the standard setting methods suggested in the Manual suitable for the skill of writing are examinee-centered (CitationCouncil of Europe, 2009, Chapter 6). We therefore aim to make a contribution to the literature by describing key development, implementation, and scoring facets of a test-centered approach to linking level-specific writing tasks to the CEFR.
To address this aim, we first analyze the effect of the level-specific design factors (i.e., tasks, rating criteria, raters, and students) on the variability of the ratings of students' written responses. We then investigate whether the analyses in particular suggest empirically grounded cut-scores that are in alignment with the targeted CEFR proficiency levels of the tasks.
We have organized this article as follows. In the first main section, we present a literature review focused on the significance of the CEFR for language testing and cut-core setting, on different approaches to assessing writing, on research into difficulty-determining task characteristics, and on the influence of rating approaches and rater training on rating variability. In the second main section we describe the broader background of our study, delineating the large-scale assessment project within which our study is situated and providing details on the development of the writing construct specification and the task development. In the Methodology section, we describe the design of the study reported here, as well as the rating procedure used for data collection; this is followed by the research questions and the statistical analyses we conducted with a specific emphasis on g-theory and multifaceted Rasch analysis. We then present the empirical results of our research in the fourth main section and conclude the article with a discussion of these results in the context of task development for large-scale writing assessment linked to the CEFR.
LITERATURE REVIEW
Test Development and Setting Cut-Scores in Line With the CEFR
The overall aim of the CEFR as a tool of the Council of Europe language policy is to stipulate reflection, communication, and discussion amongst practitioners in the fields of language learning, teaching and assessment (CitationCouncil of Europe, 2001, p. 1). The CEFR is intended “to
• | promote and facilitate co-operation among educational institutions in different countries; | ||||
• | provide a sound basis for the mutual recognition of language qualifications; | ||||
• | assist learners, teachers, course designers, examining bodies and educational administrators to situate and co-ordinate their efforts” (CitationCouncil of Europe, 2001, p. 5f). |
To achieve these aims, the CEFR provides a descriptive scheme of activities, skills, knowledge, and quality of language “in order to use a language for communication … effectively” (CitationCouncil of Europe, 2001, p. 1). These categories are defined by proficiency level descriptors in six levels of emerging communicative language ability, ranging from the basic user stage (Levels A1 and A2) via the independent user stage (Levels B1 and B2) to the proficient user stage (Levels C1 and C2).
Even though the CEFR has become a key reference document in the area of language tests and examinations, best practices for its use are heavily debated amongst scholars. Of importance, the CEFR is a “descriptive framework, not a set of suggestions, recommendations, or guidelines” (CitationMorrow, 2004, p. 7). Some researchers, such as CitationNorth (2004), regard the framework as a “practical, accessible tool that can be used to relate course, assessment, and examination content to the CEF categories and levels” (CitationNorth, 2004, p. 77). North suggested “studying relevant CEF scales, stating what is and what is not assessed, and what level of proficiency is expected” (p. 78) as a basis for relating examinations to the CEFR. Other researchers, however, take a more critical stance. CitationWeir (2005), for instance, came to the conclusion that “the CEFR is not sufficiently comprehensive, coherent or transparent for uncritical use in language testing” (p. 281), as it does not “address at different levels of proficiency the components of validity” (p. 284), such as describing contextual variables or defining theory-based language processes. Weir's contentions are to a certain degree in line with the outcomes of the Dutch CEFR construct project (CitationAlderson et al., 2006), which reports shortcomings in the CEFR as a descriptive instrument. To overcome some limitations of the CEFR for test development practices, this group developed the Dutch Grid, a task classification scheme designed to assist in the characterization of reading and listening comprehension tests. In the context of using the CEFR for assessing writing, CitationHarsch (2007) came to the conclusion that the CEFR scales are too coarse, vague, and at times incoherent to be directly used for the development of writing tasks or rating scales. To overcome the specific limitations of the CEFR for developing writing assessment instruments, a grid for characterizing writing tasks, the CEFR Grid for the Analysis of Writing Tasks, was developed by members of the Association of Language Testers in Europe on behalf of the CitationCouncil of Europe (2008). Its main aim is to “analyse test task content and other attributes, facilitating comparison and review” (http://www.alte.org/projects/grids.php) to facilitate the “specification” stage when aligning tests to the CEFR.
The procedure of aligning examinations to the CEFR is a complex endeavor. Its core component is known as standard-setting (e.g., Cizek, 2001; CitationZieky & Perie, 2006), and it involves setting cut-scores on the examination's proficiency scale in correspondence to the CEFR levels. Due to the growing importance of setting defensible cut-scores for reporting purposes in Europe, the CitationCouncil of Europe (2009) has published the Manual for Relating Language Examinations to the CEFR, based on an earlier pilot version (CitationCouncil of Europe, 2003). The Manual provides a comprehensive overview over basic considerations and possible steps to align language examinations; it is accompanied by several reference supplements addressing more technical issues. As far as the alignment of writing tasks is concerned, the Manual suggests specifying the tasks by using, for instance, the aforementioned Grid, followed by formal standard-setting methods. In the field of writing tasks, examinee-centered standard-setting methods are suggested, using examinees' responses to align the writing test to the CEFR levels (CitationCouncil of Europe, 2009, p. 44ff). If one, however, wants to link the writing tasks themselves directly to the CEFR levels, in accordance with test-centered standard-setting methods usually chosen to link tests targeting reading or listening comprehension to the CEFR levels, the Manual does not suggest a suitable test-centered standard-setting method for writing tests. We therefore investigate the feasibility of applying a test-centered standard-setting approach to align level-specific writing tasks directly to their targeted CEFR levels. Although this article does not focus on the actual formal standard-setting procedure, its aim is to explore how far the results of our analyses can contribute toward underpinning the alignment of writing tasks to the CEFR with empirically grounded cut-scores that can be used as a supplementary data source for standard-setting procedures.
Approaches to Assessing Writing
The prototypical task design for assessing writing in large-scale assessment contexts consists of using tasks of approximately equal difficulty that are designed to elicit a wide range of written responses, which can then be rated by trained raters with a rating scale covering performance criteria across multiple proficiency levels (see, e.g., Hamp-Lyons & Kroll, 1998, or CitationWeigle, 1999, Citation2002). Such a multilevel approach was used, for example, in the Study of German and English Language Proficiency (Deutsch-Englisch Schülerleistungen International; DESI; CitationBeck & Klieme, 2007; CitationHarsch, Neumann, Lehmann, & Schröder, 2007). In that context, the approach was justifiable by the test purpose, which was to report the proficiency distribution of all ninth graders in Germany based on curricular expectations.
If the purpose of an assessment is, however, to report whether students have reached a specific level of attainment or a specific performance standard within a broader framework of proficiency, a different approach to assessing writing could be worth pursuing, that is, the aforementioned level-specific approach, whereby one task operationalizes one specific level, as it is the traditional approach for assessing receptive skills. Examples of a level-specific task approach can be found in international English proficiency tests offered for example by Trinity, Pearson, or Cambridge Assessment. In the case of the Cambridge ESOL General English suite of exams (CitationTaylor & Jones, 2006), for instance, different exams target five different proficiency levels; however, the written responses are assessed via different multiband (or multilevel) rating scales. Although the tasks can be characterized as level-specific (see CitationShaw & Weir, 2007, for a detailed account of how tasks of the different exam levels operationalize CEFR levels), the exam-specific rating scales cover six finer proficiency bands (“multilevels” from 0 to 5) within each of the five exam levels. As a result, each band of the rating scales has a meaning only in relation to the targeted exam level. It is stated that “candidates who fully satisfy the band 3 descriptor will demonstrate an adequate performance of writing at [the exam] level” (CitationCambridge ESOL, n.d., p. 33). We could therefore interpret that ratings at or above band 3 constitute a “pass” and ratings below band 3 a “fail.” To link the various rating bands across the five exam levels, Cambridge ESOL has recently completed a long-term project to develop a Common Scale for Writing covering the five upper CEFR levels (cf. CitationHawkey & Shaw, 2005, CitationShaw & Weir, 2007). However, it remains unclear how the finer bands of the exam-specific rating scales can be interpreted with reference to the levels of this Common Scale and to the CEFR proficiency levels; to be more specific, could a band 5 rating, for instance, in the CAE be interpreted as the candidate having shown a writing performance beyond CEFR Level C1? Although this issue is addressed for the overall grade (cf. the information on the CAE certificate at http://www.cambridgeesol.org/assets/pdf/cae_statement_of_results.pdf, where grade A in the CAE is interpreted as having shown performance at Level C2), it is not addressed for reporting a profile for the different skills covered in the exam. Thus, it seems difficult to transparently trace how multiband ratings of written performances in this suite of exams could lead to the assessment of a candidate's writing proficiency in terms of CEFR levels. Although such level-specific exams are aimed to assess and report individuals' English proficiency with a focus on one proficiency level, they seem not to be suitable for a large-scale context, where the aim is to screen a population spanning several proficiency levels. Here, instruments are needed which can account for a range of abilities in the sample tested, and the results of the assessment have to be generalisable for the population.
Another relevant example of assessing writing by using level-specific tasks and level-specific rating criteria is the one taken by the Australian Certificates in Spoken and Written English, an achievement test for adult migrants. Tasks are developed by teachers to operationalize four different levels of attainment. Written learner productions are then assessed by teachers using performance criteria that describe demanded or expected features for each of the four levels; the criteria are assessed by giving binary judgments as to whether or not learner texts show the demanded features (CitationBrindley, 2000, Citation2001). Although this approach to assessing writing at a specific level is promising, it holds certain constraints: The Australian assessment (CitationBrindley, 2000) focuses on individual achievement, whereby teachers give individual feedback to their learners. The task development is not standardized, and neither are the administration and assessment procedures. The relationship between the targeted four levels of attainment and the task specifications targeting each level remains somewhat unclear. For a large-scale proficiency assessment, the focus is less on individual achievement and more on gaining generalizable data for the targeted population. Therefore, standardized procedures for task development, administration, and assessment are needed. Specifically, the relationship between the targeted proficiency level and the task characteristic, as well as the relationship between proficiency levels and rating scale levels need to be made transparent. The current study therefore aims to make a significant contribution toward researching the level-specific approach for tasks and rating scales in a large-scale assessment context.
Task-Difficulty Characteristics
When using a level-specific task approach, research on task-difficulty characteristics may help to shed light on information processing and cognitive demands. Influential work in this field, mainly situated in pedagogical, second language acquisition contexts, is reported by, for example, CitationBrown, Anderson, Shillcock, and Yule (1984); CitationPrabhu (1987); CitationRobinson (2001); CitationSkehan (1998); and CitationSkehan and Foster (2001). The results of these studies point toward a progression of difficulty from simple tasks, where all information necessary to solve the task is given, to more complex ones, where the prompt requires the explanation of abstract concepts or the development of an argument; the number of elements to address also increases task difficulty, as does the amount of processing and reasoning required to solve the task. Task difficulty decreases with an increasing level of familiarity and prior knowledge of topics, tasks, and cognitive operations demanded; furthermore, difficulty decreases with the amount of information given and the degree of precision—the more precise, the easier the task.
CitationSkehan and Foster (2001) claimed in their Limited Attention Capacity Model (LACM) that an increase in task complexity would strain the limited attention capacity of learners, who would thus shift their focus on form and produce linguistically less complex output with more errors. CitationRobinson (2001) proposed the Triadic Componential Framework, expanding the LACM and claiming that as learners possess different attentional resource pools, an increase in task complexity would not necessarily lead to a decrease in their linguistic output.
When looking at studies that tried to empirically put these models into operation, the results are rather inconsistent. CitationKuiken and Vedder (2007), for example, found it difficult to operationalize the variables of the models in their study of task complexity and linguistic performance (p. 265), and they came to the conclusion that it was not possible to establish interactional effects between task complexity and proficiency level (p. 276). An example of the application of the LACM in a testing context is the study by CitationIwashita, McNamara, and Elder (2001) on speaking tasks in the ETS oral test. They found a “lack of consonance” between their results and SLA research findings and came to the conclusion that the “differences between testing and pedagogical contexts are so great as to alter the cognitive focus of the task” (p. 430). This seems further corroborated by CitationNorris, Brown, Hudson, and Bonk (2002), who reported inconsistent results when operationalizing Skehan's model.
The aforementioned studies seem to indicate that it is difficult to apply task characteristics found in pedagogical contexts to test contexts; nevertheless, these characteristics can help to underpin test constructs and the level descriptors in the CEFR with empirical research. Although a direct alignment of these characteristics to specific CEFR levels is not the aim of the study reported here, it would remain a challenging desideratum for further research. In this context, issues of congruence between the level specificity of test tasks and the functional orientation of the CEFR would need to be explored in more depth. As a starting point, one could select relevant CEFR descriptors focusing on “communicative activities” (Chapter 4), extract relevant functional concepts and task characteristics and translate these concepts and characteristics into test specifications. Rating criteria and scales could also be based on relevant CEFR descriptors targeting “linguistic competences” as described in CEFR Chapter 5. This is the approach we chose, which is described in more detail next. It is worth mentioning here that the CEFR differentiates between “quality” and “quantity,” as, for example, CitationDe Jong (2004) or CitationHulstijn (2007) stated: Quantity refers to what learners can do in terms of functional skills as for instance demanded by a task, whereas quality refers to how well learners perform in terms of effective and efficient use of language skills. Bearing this in mind, it seems reasonable in a large-scale assessment to offer test tasks targeting a span of proficiency levels, and assessment criteria covering both, task quantity and performance quality.
Variability of Ratings
There exists ample research into the influence of assessment criteria and raters on the variability of ratings, yet it is mainly situated in contexts where multilevel rating approaches were used (see, e.g., CitationEckes, 2005, Citation2008; CitationHamp-Lyons & Kroll, 1996; CitationKnoch, 2009; CitationLumley, 2002, Citation2005; or CitationWeigle, 1999, Citation2002). A general distinction is made between impressionistic holistic and detailed analytic assessment criteria, whereby the latter are reported to account for shortcomings of the former, such as impressionistic ratings of surface features (CitationHamp-Lyons & Kroll, 1996), halo-effects (CitationKnoch, 2009), or imprecise wording of the criteria (CitationWeigle, 2002). Therefore, an analytic approach using detailed rating criteria which account for the complexity of writing is often favored (e.g., CitationHamp-Lyons & Kroll, 1996).
There is also substantial research on the effect of rater characteristics on the variability of their assigned ratings, yet it is, again, predominantly situated in multilevel approaches and does not focus on the particularities of level-specific ratings. For the traditional multilevel approach, critical characteristics include raters' background, experience, expectations, and their preferred rating styles (see, e.g., CitationEckes, 2005, Citation2008; CitationLumley, 2002, Citation2005; Lumley & McNamara, 1995; CitationWeigle, 1998, Citation2002).
Although these studies can help determine which facets have to be explored and controlled in a level-specific context, there nevertheless exist gaps in the literature as far as the level-specific approach to assessing writing is concerned: There is a lack of research on the effects of holistic and analytic criteria on the variability of level-specific ratings as well as on the effects of rater characteristics. With regards to these areas, two of the scarce studies can be found in the aforementioned Australian context. CitationSmith (2000) reported that although raters may show acceptable consistency on an overall level, this might well “obscure differences at the level of individual performance criteria” (p. 174). These findings are corroborated by a study conducted by CitationBrindley (2001), who concluded that the main sources of variance in his study were the terminology used in the performance criteria, and the writing tasks, which had been developed by individual teachers rather than in a standardized process. Both studies revealed a need for rater training, which can help lessen the unintended variance to a certain extent (e.g., CitationLumley, 2002). Therefore, all of the known characteristics that drive rating performance should be addressed during rater training, regardless of which approach is chosen.
Bearing in mind the peculiarities of the level-specific approach, where tasks and criteria focus on one level only, and raters have to rate via a fail/pass judgment, an analytic approach seems vital to gain insight into what aspects raters are actually rating on the descriptor level and to ensure rater reliability. It is this aspect of ensuring reliability that justifies the analytic approach even if the assessment purpose is to report one global proficiency score.
The review of the research literature thus reveals a gap in the area of level-specific approaches to assessing writing. There is, to our knowledge, no large-scale study reporting on how a level-specific rating approach affects the variability of ratings. Our article aims to explore facets known from other contexts to influence rating variability, namely, tasks, assessment criteria, raters, and student ability, within a level-specific approach in a large-scale assessment study. Because the in-depth analysis of different rater characteristics and their effects on rating variability is beyond the scope of this article, we intend to control rater characteristics as far as possible via selecting raters with similar background and via extensive training. The purpose is to ensure reliable ratings because they form the basis of inferences about task quality and difficulty estimates.
Moreover, to our knowledge, there are no reports on test-centered methods for linking level-specific writing tasks to specific CEFR levels. Our article thus makes an important contribution to test-centered standard-setting methods by exploring how far a priori task CEFR level classifications correspond with empirical task difficulty estimates.
BACKGROUND
To contextualize the study we are reporting in this article, we first need to delineate the broader context of the large-scale assessment project within which our study is situated. More specifically, we need to explain the writing construct and the writing task development, because these aspects form the basis of the a priori task classifications in terms of targeted CEFR levels, and thus the backdrop for our empirical analyses of possible cut-score suggestions in line with the CEFR levels. Following the background explained in this section, the Methodology section then describes the actual study within which our data were collected.
Evaluation of Educational Standards in Germany
The broader background of the study reported in this article is a large-scale standards-based assessment project in Germany. There, the CEFR is currently used by the Institute for Educational Progress (Institut zur Qualitätsentwicklung im Bildungswesen) to develop large item pools to test reading comprehension, listening comprehension, and writing for English as a first foreign language. These tests are used to evaluate the German National Educational Standards (NES), which were commissioned in 2003 by the Standing Conference of the Ministers of Education (Kultusministerkonferenz). For a comprehensive description of the overall test development process generally, the task development processes for specific competences apart from writing, the key documents that guided these processes, and the political context within which all of this work is embedded, we refer the reader to CitationRupp, Vock, Harsch, and Köller (2008).
For the purpose of this article, it is important to note that the NES target students in two different school tracks, one leading to a basic school leaving exam at the end of Grade 9 (Hauptschulabschluss [HSA]) and the other leading to a secondary school qualification at a higher level at the end of Grade 10 (Mittlerer Schulabschluss [MSA]), qualifying either for vocational training or further secondary school. The NES for the first foreign language are based on the CEFR and adopt its model of communicative competence (see ).
TABLE 1 Model of Communicative Competencies in the NES
More specifically, the NES use selected descriptors from the CEFR proficiency scales to describe student competencies at Level A2 for the HSA and at Level B1/B1+ for the MSA. gives an overview over the description of writing competencies in the NES targeting the MSA (CEFR Level B1).
TABLE 2 Overview Over the National Educational Standards Writing Competency Descriptors for the Mittlerer Schulabschluss
Although the NES target CEFR Levels A2 and B1, their evaluation is situated within a broader range of CEFR levels to be able to report also on students' proficiency above or below the NES. The main operational purpose of the standards-based writing tests is thus to report students' writing proficiency in English as first foreign language in terms of the five lower CEFR levels (i.e., Levels A1 to C1). No tasks targeted at proficiency level C2 were included in the standards-based assessment, because Level C1 already represents the level of expected English proficiency for entering university in Germany and only very few students, if any, were expected to be at Level C2 in the MSA sample.
Given this context, it was decided that multilevel tasks were not desirable for several reasons. First, similar to the Cambridge ESOL exams (cf. CitationShaw & Weir 2007), task demands for different CEFR levels needed to be operationalized as precisely as possible, which would have been more difficult with multilevel tasks that spanned a wide range of proficiency levels. Second, we found in preliminary studies that it can be challenging to train raters to use different rating scales for tasks targeting different proficiency levels reliably. Third, in contrast to DESI, the writing tasks needed to be administered to students with a wider range of proficiencies, making it challenging to write tasks that could elicit responses across a broad range of proficiency levels. We therefore adopted a level-specific approach for our study, similar to the approach mentioned in CitationBrindley (2000, Citation2001) yet for a large-scale proficiency assessment in alignment with the CEFR. Writing tasks and rating scales were targeted to a specific CEFR level so that a successful student response is evidence of proficiency at the specific CEFR level that is targeted by the task. Consequently, it was most advisable to apply a test-centered standard-setting procedure to align the level-specific writing tasks directly to their targeted CEFR levels.
Writing Task Development
The writing tasks and the associated rating scales were developed by teachers who were nominated by the Kultusministerkonferenz in 2005, representing the federal school system in Germany. The teachers received comprehensive training by an internationally renowned expert in the knowledge and skills of professional task development for standards-based language assessment over a period of 2 years for a total amount of 252 hr of training time. In addition, the item development process was reviewed by an international expert team, which met regularly in Berlin.
Because the CEFR and the NES constituted the larger framework of assessment, the model of writing used in these documents was taken as the starting point. According to the CEFR and NES, writing is a productive and interactive activity whereby authentic communicative tasks are carried out. Completing such tasks requires engagement in communicative language activities and the operation of meta-cognitive communication strategies (for an analysis of the writing construct in the CEFR, see CitationHarsch, 2007). This model served as the backdrop for the development of test specifications in the Institut zur Qualitätsentwicklung im Bildungswesen project. Due to the perceived shortcomings of the CEFR to provide for test specifications, which we outlined in the literature review, the following procedural approach was implemented, based on CitationNorth's (2004) recommendations. First, the NES descriptors as well as CEFR level descriptors for Levels A1 to C1 from 13 different CEFR scales relevant for writing (CitationCouncil of Europe, 2001, pp. 61f, 83f, 110–118, 125) were collected. Appendix A illustrates selected CEFR writing descriptors for Level B1. All descriptors were then analyzed in terms of their content and terminology and subsequently condensed and refined to serve as a basis for developing a construct definition and test specifications. Specifically, redundant concepts were omitted; terminology was revised to derive at coherent descriptions of the specific purpose; vague or too general statements were revised to be more concrete; and writing purposes, text types, and communicative activities relevant in our context were supplemented. Appendix B illustrates this process for the development of the specific purpose at Level B1.
In addition to the analysis of the descriptors in the CEFR and NES, the task specifications also drew on research on writing in English as a foreign language, whose key results are sketched out only coarsely in the CEFR. For example, CitationHayes and Flower (1980) identified key recursive processes in writing thereby shifting the traditional focus on form toward a focus on writing as a social action. Recent research similarly suggests that it is also necessary to consider relevant facets such as task environment, individual factors, and the social context of the writing activity when developing writing tasks and rating student responses (e.g., CitationGrabe & Kaplan, 1996; CitationHayes, 1996; CitationShaw & Weir, 2007; CitationWeigle, 1999, Citation2002).
As a consequence, the writing purpose, the addressees of the text, and the social context of the writing activity were varied systematically across tasks and clearly stated in the task prompts. In alignment with the level-specific approach, task demands, expected content, structural and linguistic features as well as the time allotted to answer a task were systematically varied across the targeted CEFR levels. In other words, the complexity of the required speech acts; text types; linguistic structures; and the organizational, strategic, and cognitive expectations increased consistently for tasks from Levels A1 to C1. A similar approach is described for the Cambridge ESOL suite (Chapter 4 in CitationShaw & Weir 2007).
One practical implication of the level-specific approach is that tasks are much more constrained at lower CEFR levels and much more open at higher CEFR levels. At Level A1, for example, a task might consist of filling in a simple information sheet at a hotel reception and would not require organizing a text into coherent paragraphs. Thus, students are not able to display higher levels of proficiency for such a task even if they are highly proficient. In contrast, tasks at higher CEFR levels such as B2 require more elaborated responses such as writing an opinion on a topic of general interest. Successful student responses at this level include evidence of various methodological competencies such as the logical development of ideas and the coherent structuring of these ideas in a longer text.
To aid the task developers considering these design characteristics during the development process, they were asked to classify their tasks according to a variety of relevant criteria for language test development adapted from the Dutch Grid (CitationAlderson et al., 2006) as well as the CEFR Grid for Writing Tasks (CitationCouncil of Europe, 2008). To address the ambiguous terminology previously mentioned in the literature review, the developers worked in teams and based the classification on consensus ratings, using templates created for this purpose. A total of 86 prototype writing tasks were created, out of which 19 were included in the study of this article. All tasks were a priori classified in terms of their targeted CEFR level by the task developers, again based on consensus ratings. summarizes the task specifications in terms of the level-specific purposes, text types, task characteristics, textual, and linguistic expectations for three of the five CEFR levels. These specifications result from two workshops with all developers to train interpretation and application.
TABLE 3 Test Specifications
METHODOLOGY
The data for the study we report in this article were collected during a large-scale field trial of the standards-based tests for reading, listening, and writing that took place between April and May 2007. Although the broader purpose of the field trial was to obtain empirical information about the operating characteristics of the tests and to establish three separate one-dimensional proficiency scales for reading, listening, and writing, the focus of this article is on the writing data to investigate whether the level-specific approach allows for reliable inferences about the quality of the writing tasks and to assess how far the a priori classified tasks in terms of their targeted CEFR levels match the empirical task difficulties. Because the empirical difficulty of a task is the result of the complex interactions between the task, the rating criteria, the raters, and the students' proficiency distribution (see CitationWeigle, 1999, for multilevel approaches to assessing writing), we need to analyze the data by taking these design factors into consideration, the more so because in our context these aspects are restricted to specific levels.
To address these aims, the design of the study, the specific research questions, and the statistical analyses have to be attuned to one another. Therefore, we first describe relevant facets of the design of the writing field trial, that is, the task samples, the rating approach, the rater training, and the rating design. Based on this, we address the specific research questions and describe the statistical analyses we conducted.
Design of Study and Data Collection
Sampling issues
For the field trial, a representative national random sample of 2,065 students from all 16 federal states in Germany was selected. The students had undergone 8 to 10 years of schooling and were between 15 and 18 years of age. Most students were native German speakers. The students came from the HSA and MSA school tracks targeted by the NES: There were 791 students in the HSA sample (approximately balanced by grade with 383 in Grade 8 and 408 in Grade 9) and 1,274 students in the MSA sample (approximately balanced by grade with 629 in Grade 9 and 645 in Grade 10).
Taken together, the students responded to 19 writing tasks that covered levels A1 to C1 of the CEFR. Due to time constraints and the cognitive demands of the writing tasks, however, each student responded only to two, three, or four writing tasks, depending on the CEFR level that the tasks targeted. The writing tasks were administered in 13 different test booklets within a complex rotation design across the two samples, which is also known as a matrix-sampling or balanced incomplete block design (e.g., CitationFrey, Hartig, & Rupp, 2009; CitationMislevy & Rupp, 2009; Citationvon Davier, Sinharay, Oranje, & Beaton, 2006). The different booklets in the two sample designs were linked by common writing tasks, so-called anchor tasks. These were included to allow for an investigation of whether the two samples could be calibrated together on one common proficiency scale.
Rating Approach and Rater Training
The construction of the rating scale was based on the analysis and adaptation of preexisting rating scales along with the creation of descriptors suitable for the proficiency level targeted by each writing task. The development of the rating scale was grounded in the following key documents:
1. | Relevant illustrative scales from the CEFR (sections starting at p. 61 and p. 82 for written production and interaction as well as p. 109 for language competencies) | ||||
2. | The Written Assessment Criteria Grid from the Manual to the CEFR (CitationCouncil of Europe, 2003, p. 82) | ||||
3. | Rating scales from the DESI project (CitationHarsch, 2007) | ||||
4. | Rating scales from the Hungarian project Into Europe (CitationTankó, 2005) |
Similar to the developmental process of the test specifications described in the previous background section, rating scale descriptors were analyzed for content, terminology, coherence, and consistency. Based on this, level-specific descriptors were constructed for the following four assessment criteria: task fulfillment, organization, grammar, and vocabulary. The initial draft was pretrialed and revised in an iterative process by the task developers and reviewed by an international expert team. Moreover, a set of initial benchmark texts was selected from the pretrials to illustrate prototypical features of student responses for each assessment criterion at each proficiency level. During rater training seminars for the study of this paper, which are described in more detail next, the rating scales were revised once more and further benchmark texts were added. The final version of the rating scale was validated by a team of teachers and international researchers in the field of writing assessment (for details, see CitationHarsch, 2010). An illustration of the scale for Level B1 is provided in Appendix C.
The rating approach chosen was an analytic one, whereby the detailed analyses of the students' responses formed the basis for a final overall score to account for the above discussed higher reliability of such a detailed rating procedure (CitationKnoch, 2009). First, four analytic ratings of the four mentioned criteria were given. In line with the level-specific task design approach, each student response was rated on a two-point rating scale (“below pass” = not reaching the targeted level of performance, “pass” = reaching the targeted level of performance). To derive at reliable analytic ratings for the criteria, each single descriptor was first rated on the 2-point scale. These descriptor ratings formed the basis of the four analytic criteria ratings. Finally, based on the analysis of the student text, an overall grade was assigned in line with the test purpose, that is, to report one proficiency score.
The rater training took place between July and August 2007. We aimed to account for the known effects of rater characteristics by choosing raters with comparable educational backgrounds; by providing enough rating practice to account for different levels of experience and to allow for an in-depth familiarization with the procedures; by encouraging discussions of key task characteristics, assessment instruments, and sample students' responses to derive at a common level of expected performance; and by actively engaging raters in evaluating the adequacy and applicability of benchmark texts and rating scales, which they were allowed to revise where necessary.
In cooperation with the Data Processing Center in Hamburg, 13 graduate students from the University of Hamburg (English as a Foreign Language program) were trained on the level-specific rating approach. Their functional level of proficiency in English was established by an entrance test that included an assessment of their writing ability. The raters were between 25 and 33 years old and all had prior experience in either teaching English or marking essays. During the intensive training sessions (two 1-week seminars and six additional 1-day sessions over a period of 8 weeks), the raters were familiarized with the previously described rating instruments and procedures. Throughout the training period they rated sample student responses. These ratings were analyzed by the facilitator to control rater reliability, guide the training process, and further revise the assessment instruments. To describe this process further would go beyond the scope of this article. For a detailed account, See CitationHarsch & Martin (2011).
Rating Design for Field Trial
The field trial responses were rated between September and October 2007. To analyze the effects of the design factors (tasks, rating criteria, raters, student proficiencies), the two following rating designs were used. The first rating design, the so-called multiple marking design, involved all raters, in groups of four, independently rating the same set of selected student responses within one group. For this, 30 responses from each of the 13 booklets were randomly chosen and allocated to the rater groups in a Youden square design (CitationPreece, 1990; see CitationFrey et al., 2009). The Youden square design is a particular form of an incomplete block design that in our case ensured a linkage of ratings across all booklets and an even distribution of rater combinations across booklets. The resulting linkage of students, tasks, and raters allowed us to perform variance component analyses motivated by g-theory, as described next.
The second design, the so-called single marking design, allocated all student responses randomly to all raters, with each rater rating an equal number of responses and each response being rated once. This design allowed controlling for systematic rater effects by ensuring an approximately balanced allocation of student responses across tasks to different raters.
The multifaceted Rasch analyses, which are described next, are based on the combined data from the two rating designs to ensure a sufficiently strong linkage between tasks, raters, and students.
Data Analysis
Given the previous considerations about quality control in rating procedures and the lack of a strong research base for level-specific approaches to assessing writing proficiency, the primary objective of the study we report is on establishing the psychometric qualities of the writing tasks using the ratings from the field trial. This is critical because the ratings form the basis of inferences about task difficulty estimates, raters' performance, and students' proficiency estimates. If the rating quality is poor vis-à-vis the design characteristics used in the field trial, the defensibility of any resulting narratives about the difficulty of the writing tasks and their alignment to the CEFR levels is compromised.
In more specific terms, the two research questions for this study based on the primary objective are as follows:
-
RQ1: What are the relative contributions of each of the design factors (tasks, criteria, raters, students) to the overall variability in the ratings of the HSA and MSA student samples?
-
RQ2: Based on the analyses in RQ1, how closely do empirical estimates of task difficulty and a priori estimates of task difficulty by task developers align? Is it possible to arrive at empirically grounded cut-scores in alignment with the CEFR using suitable statistical analyses?
We answer both research questions separately for students from the HSA and MSA school tracks. We do this primarily because preliminary calibrations with the writing data, which are not the main focus of this article, as well as related data on reading and listening proficiency tests have suggested that a separate calibration leads to more reliable and defensible interpretations. This decision was also partly politically motivated by the need for consistent and defensible reporting strategies across reading, listening, and writing proficiency tests.
To answer the first research question, we use descriptive statistics of the rating data as well as variance components analyses grounded in generalizability theory (g-theory; e.g., CitationBrennan, 2001, Citation2007), which decomposes the overall variation in ratings according to the relative contribution of each of the design factors listed previously. To answer the second question, we take into account the interactional effects of our design facets on the variability of the ratings via multifaceted Rasch modeling (e.g., CitationCongdon & McQueen, 2000; CitationEckes, 2005; see CitationBriggs & Wilson, 2007). This represents a parametric latent-variable approach that goes beyond identifying the influence of individual design factors to statistically correct for potential biases in the resulting estimates of task and criteria difficulty, rater performance, and student proficiency. Utilizing descriptive statistics, g-theory analyses, and multifaceted Rasch model analyses en concerto helps to triangulate the empirical evidence for the rating quality and to illustrate the different kinds of inferences supported by each analytic approach.
Generalizability Theory
G-Theory is an extension of classical test theory (e.g., CitationKline, 2005; CitationLord & Novick, 1968; CitationWainer & Thissen, 2001), which is concerned with identifying the influence that different design facets have on the magnitude of measurement error in assessment procedures. On one hand, this reduces the amount of unexplained measurement error and, on the other hand, it allows researchers to determine the number of levels by which each design facet should be increased or decreased to attain a desired level of precision for relative or absolute comparisons of students. Because g-theory models are based on variance components, they can provide information about relative contributions of each design facet to measurement error, but they do not allow for an adjustment of task difficulty, rater severity, or student proficiency estimates due to the influence of other design facets.
The types of information that can be culled from a g-theory analysis depend on the design with which the data were collected. The simplest design is a fully crossed balanced design in which an identical number of experimental units (i.e., student responses in our case) are randomly assigned to each combination of the design facets and the outcome variable of interest (i.e., the rating). Specifically, for our context, the four design facets of interest from a g-theory perspective are tasks, rating criteria, raters, and students.
Because the Youden Square design just described results in a fully crossed balanced design such that the same four raters rate each student response to each task on all five criteria, the g-theory model for analyzing the variation in the ratings has the following form:
The subscripted model coefficients denote the effects of interest with the last term representing the confounded effect for error and the four-way interaction; s denotes students, t denotes tasks, c denotes criteria, and r denotes raters. That is, the model in EquationEquation 1 contains four main effects, six two-way interaction effects, four three-way interaction effects, and one error term that is equivalent to the four-way interaction term due to the fact that there is only one observation per cell at that level. The estimation of variance component can follow different approaches. Because the response data in our case are dichotomous (below pass / pass), the assumption of a normally distributed rating is not justified. Consequently, the variance component point estimates were estimated by equating observed and expected mean squares and interpreted descriptively, not inferentially. The estimation was done using the program GENOVA (CitationCrick & Brennan, 1983), which provided the estimates and the standard errors of the estimates.
We performed the g-theory analyses using the model in EquationEquation 1 separately for each of the 13 Youden Square booklets in the multiple-marking study. We then aggregated the resulting variance components across the 13 booklets as described in CitationChiu and Wolfe (2002). The proportion of ratings in each booklet was used as the weight, which varied across booklets as a different number of tasks were included in each booklet due to different difficulty levels of the tasks in each booklet.
Multifaceted Rasch Modeling
Generally speaking, the main focus of multifaceted modeling is to use a latent-variable measurement model to adjust the proficiency estimates of the students due to the design facets that have given rise to their variability. Recall that the writing tasks were designed to capture variation in student proficiencies across a single proficiency scale that was supposed to be used for reporting purposes. Because reading and listening comprehension tasks had been successfully scaled with a Rasch model from item response theory (e.g., Citationde Ayala, 2009; CitationEmbretson & Reise, 2000), and because the available sample size per task prohibited a reliable estimation of more complex models, we used a multifaceted Rasch model with a single proficiency variable (e.g., CitationEckes, 2005). Our model included task, rater, and rating criterion as separate facets in addition to the student proficiency variable:
RESULTS
In this section we describe and synthesize key results from the descriptive analyses, the g-theory analyses, and the multifaceted Rasch analyses.
Descriptive Summary Statistics
Apart from data that were missing by design due to the complex booklet design that was used in the assessment overall, some student responses were not legible, some students produced so little text that it could not be assessed, and some students did not respond to their assigned tasks. All of these cases led to missing ratings, which accounted for 24.8% of the ratings in the HSA sample but only for 5.0% of the ratings in the MSA design. These magnitudes reflect the different mean achievement levels in these samples as well as a poorer match of the assigned tasks to the students in the HSA sample, who found some tasks at CEFR levels B1 and B2 too challenging and opted to skip them. As these missing data can be interpreted as unsuccessful attempts at the tasks, they were coded as “below pass.”
We first address the design factor raters. shows the percentage of students who received a pass on the global rating for each of the 19 tasks by each of the 13 raters. We show the global rating here to conserve space. also presents the expected a priori CEFR levels as provided by the task developers before the data were collected. As shows, the variation among the raters in terms of marginal percentages correct across all tasks within a particular student sample is relatively small with a few exceptions such as Rater 1 and Rater 2 in the MSA design, who gave, on average, much higher ratings than the other raters. The average ratings for each sample across all tasks and raters (i.e., .37 for the HSA sample and .63 for the MSA sample) reflect the expected proficiency difference of students in the two samples.
TABLE 4 Percentage of Student Responses Classified as “Pass” by Sample, Task, and Rater
On the task side, tasks that were classified into lower CEFR proficiency levels had higher marginal “pass” proportions across raters and vice versa; the only exception is Task 12 in the MSA sample, the empirical characteristics of which are more similar to tasks at Level A2. Despite this desirable ordering of the tasks, Tasks 13, 17, and 19 did not function very well in the HSA sample, whereas Tasks 7, 11, and 18 did not function well in the MSA sample from a discriminatory perspective. With the exception of Task 7, which was too easy in the MSA sample (i.e., % “pass” = .99), the other five tasks were too difficult in their respective samples (i.e., % “pass” ≤ .11), thus providing little discriminatory information about students.
Results From G-Theory Analysis
Note that the first six booklets were administered to students in the HSA sample, whereas the remaining seven booklets were administered to students in the MSA sample; the first and sixth booklets were administered in both designs as anchor booklets. shows the aggregated variance components for these two student samples.
TABLE 5 Aggregated Variance Components Estimates for HSA and MSA Samples
Notably, the absolute values of the variance components are small because the ratings are dichotomous. However, the percentage of total variance in the ratings accounted for each component shows that the writing tasks functioned differently across the two samples.
On the one hand, it can be seen that the majority of variation of ratings in either sample is due to differences between students (24.3% and 8.4%, respectively), differences between tasks (9.0% and 37.3%, respectively), and the interaction between students and tasks (17.2% and 11.4%, respectively). However, the relative amount of variance explained by the main effect differs between the two samples. In the HSA sample, the amount of variation in the ratings that is due to students is almost three times as large as the amount of variation due to tasks. In the MSA sample, this effect is reversed and the amount of variation due to tasks is four times larger than the amount of variation due to students in this sample. As we see next, this difference is also captured in the results of the multifaceted Rasch modeling. Similar to CitationChiu and Wolfe (2002), the aggregated effects combine substantial variation across booklets highlighting the importance of comparing all available data instead of using just one particular booklet, for example.
In addition to the effects just discussed, we can also note that the amounts of unexplained variation are similar in both samples (25.2% and 19.0%, respectively) and that the main effects of different raters are negligible (1.2% and 2.2%, respectively). Among the interaction effects, the effect of the interaction between students, tasks, and raters is the second-largest one in both samples (8.6% and 7.1%, respectively). This shows that, on average, there are differences in mean ratings that are attributable to the fact that different raters are assigned to different student responses from different booklets, and tasks within a booklet are of different difficulty.
Results of Multifaceted Rasch Modeling
The previous analyses suggest that there will probably be a large variation in task difficulty and student proficiency estimates but only small variation in rater severity. To investigate this hypothesis using a suitable model, we first computed the deviance values between all nested models for both the HSA and MSA samples, including models with different main effects only and models with different main and two-way interaction effects. All deviance comparisons were statistically significant at the α = .001 level so that we do not present numerical details to conserve space.
Consequently, the final model chosen for reporting purposes was the most complex model with all main and two-way interaction effects for the design facets of tasks, raters, and rating criteria as well as a single latent proficiency variable as shown in EquationEquation 2. To illustrate the structure of this model graphically, shows the Wright-map from ACER ConQuest for this model for the MSA sample. It contains the writing proficiency estimates of the students in the very left column along with the parameter estimates for all model effects in the columns to the right of it. Note that we have replaced the numerical codes for the rating criteria with letters (F = task fulfillment, O = organization, V = vocabulary, G = grammar, H = overall) in the main effect and two-way interaction effects panels and that we have replaced the numerical task IDs with the a priori CEFR classification (A1 − C1) of the writing tasks by the task developers in the task main effect panel. Note further that the figure only shows some parameter estimates for the two-way interaction effects due to space limitations; however, the remaining parameter estimates are located in the visible clusters so that the boundaries are well represented.
FIGURE 1 Wright-map for multifaceted Rasch analysis of Mittlerer Schulabschluss sample data (from CitationRupp & Porsch, 2010, p. 60. Reprinted with permission).
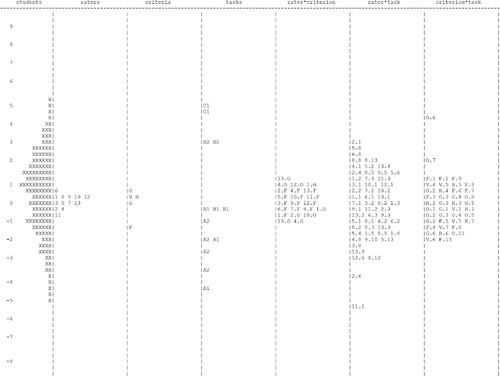
Due to the nature of the model used, all parameter estimates on a common scale and the resulting student proficiency estimates are conditioned on the remaining effects in the model, which, most important, removes any systematic differences due to rater severity. Overall, shows how this analysis captures the essential features observed earlier through the g-theory analyses. Specifically, we can see that there is a reasonably large amount of variation in the student proficiency estimates supporting similar analyses of reading and listening comprehension tasks that show a large degree of inter-individual proficiency differences for the population tested. Moreover, the rater variance is relatively small compared to the other effects showing that raters performed, on average, very similarly. The students also received similar average scores per rating criteria with task fulfillment being the easiest criterion to score highly on and the global rating being an approximate average of the other criteria. Finally, the reliability of the writing scales for making individual norm-referenced decisions is moderate for the HSA sample at .73 and high for the MSA sample at .89; consequently, the reliability for norm-referenced decisions at aggregate levels (e.g., schools, school districts) will be higher. Reliabilities are the expected a posteriori / plausible value reliability estimates available through ACER ConQuest 2.0 (see CitationAdams, 2006).
There are two obvious problems with this model that remain nevertheless. The first one concerns the large “gap” on the proficiency scale that is visible between the B1 and the B2 tasks. Clearly, less information about students in this range of the scale is available under this model than for students in other ranges. The second problem is not directly visible from the map as it concerns data-model fit at the item level. To characterize item fit we use infit and outfit measures and cut-off values of .9 and 1.1 for both in alignment with conventional large-scale assessment practice, which are reasonably conservative for a scenario with approximately n = 400 responses per writing task (e.g., CitationAdams & Wu, 2009). Most fit statistics for main effect parameters and interaction effect parameters are outside of their respective confidence intervals as computed in ACER ConQuest 2.0 (see CitationEckes, 2005, p. 210, for a discussion of alternatively suggested cutoff values) showing that some model effects are over- or underfitting. Specifically, raters for both samples show more variation in their ratings than expected (i.e., they underfit the model) even though the effect is relatively more pronounced for the HSA sample. These effects are not necessarily uncommon, however, as even the ACER ConQuest 2.0 manual shows similar parameter values for an illustrative multifaceted analysis of rater severity with multiple rating criteria (pp. 49−50). Due to the limited available sample size per rating per task and the desire to keep the writing assessment results in line with the reading and listening comprehension results, it was eventually decided to use the current results only cautiously, for refining the efficaciousness of the test development, rater training, and operational scoring process.
DISCUSSION
We first discuss the design factors task difficulty, difficulty of rating criteria, rater severity, and student proficiency, in relation to our first research question. We also address the question how far the a priori classifications match the empirical task difficulties. Based on this, we answer the question whether our analyses suggest empirical cut-scores in line with the targeted CEFR proficiency levels.
Task Difficulty
As anticipated, the relative pass rates decrease with the level of the tasks in both the HSA and the MSA sample. In other words, it is relatively easier to get a pass in a lower level task than it is in a higher level task. Rasch analyses confirmed the assumed differences between task difficulties reflecting the task design. Descriptive statistics and Rasch analyses showed that the intended a priori task difficulty classification in terms of CEFR levels correspond to a high degree with the empirical task difficulty estimates as the tasks cluster accordingly along the scale. Apart from one task in each sample, which was preclassified as A1 but is empirically estimated closer to other preclassified A2 tasks and should thus be reclassified,Footnote 1 all remaining tasks appear in the anticipated order of difficulty, which suggests that these tasks seem to function as intended. These findings were corroborated by the relative variance components from the g-theory analyses as well. Thus, there is some empirical evidence that a core set of tasks functioned as intended. At the same time, however, descriptive analyses and the Rasch-model analyses suggested that the task administration should be redesigned to match the students' proficiency levels better, as tasks of the highest or lowest level—specifically, tasks targeting Level B2 in the HSA sample and Levels A1 and C1 in the MSA sample—did not discriminate well in this study.
Rating Criteria Difficulty
The second design factor we discuss concerns the rating criteria. G-theory analyses showed a negligible influence of the rating criteria on overall rating variation. This is, in part, supported by the Rasch analyses. With regards to the relative difficulty of the five criteria, the analytic criterion task fulfillment proved to be the easiest. This was anticipated as the task instructions clearly state the expected content, addressee and communicative purpose. The thresholds of the remaining criteria are located close to each other on the Rasch scale, indicating similar difficulties. This means that the global rating would probably be sufficient for operational calibration purposes. This also confirms that treating the ratings jointly as one design facet, rather than performing a multidimensional multifaceted Rasch analysis with highly correlated and, therefore, essentially redundant latent variable dimensions was sufficient for these data. However, because the global rating is based on detailed analyses of student responses, we cannot conclude that one holistic, impressionistic rating instead of the analytic approach would have led to the same effects.
Rater Severity
Descriptive statistics showed that raters themselves displayed a relatively small degree of variation across all levels and tasks on average, which indicates an effective rater training to some extent. This conclusion is supported by g-theory, which shows that the main effect of different raters is negligible (1.2% and 2.2%, respectively), and by the Rasch analysis, which shows that there is a relatively small rater effect, making the adjustment in the student proficiency estimates due to rater severity relatively mild. Thus, we conclude that the rater training and the accompanying seminars in which raters could select and justify benchmark texts and revise conspicuous rating scale descriptors were very effective in producing raters who have a similar understanding of the different levels and criteria. Moreover, the results suggest that our approach to use descriptor-ratings as the basis for criteria ratings (see CitationHarsch & Martin, 2011) leads to reliable overall ratings.
Student Proficiency
The multifaceted Rasch analysis showed a plausible level of variance among the students in each sample and the descriptive statistics and g-theory analyses supported a higher average proficiency of the MSA sample compared to the HSA sample. The analysis of the reading and listening comprehension tests provided comparable degrees of variance, thus corroborating the findings for the writing scales.
Empirical Cut-Score Suggestions
Rasch model analyses suggested regions where cut-scores could be empirically set. Although this is true for both student samples, potential regions are more clearly separated in the upper range of the proficiency continuum. That is, tasks targeting Level B1 in the HSA sample and tasks targeting Levels B2 and C1 in the MSA sample are more easily empirically distinguishable from tasks at Levels A1 and A2 taken together than, for example, tasks at Level A2 from tasks at Level B1 or tasks at Level A1 from tasks at Level A2. Unfortunately, some ambiguity about cut-score boundaries thus remains for tasks in the lower ranges of the proficiency continuum, which are critical for standards-based reporting. In addition, there is a significant gap for students in the range of B1 and B2 on the proficiency scale for the MSA sample. Thus, although our analyses cannot provide unambiguous cut-score suggestions across the whole scale, they nevertheless suggest regions in which cut-scores could be set. More important, they show that the a priori task classifications align very well with their empirical difficulty estimations, implying that a test-centered approach to aligning level-specific tasks to the CEFR Levels A1 to B2 seems to be an empirically defensible basis, upon which consensus-based approaches can be used to confirm the CEFR levels of the tasks and to set cut-scores (see next).
CONCLUSION
In this article we have analyzed rating data from a large-scale standards-based writing assessment study in Germany. The tasks were developed as level-specific tasks with reference to the lower five proficiency levels of the CEFR, which is the primary document upon which the German Educational Standards are based. Our analyses of the data with descriptive statistics, g-theory, and multifaceted Rasch modeling provide a consistent narrative about the quality of the tasks, the assessment instruments, and the rater training as well as the hierarchical order of task difficulties and distribution of student proficiencies. The level-specific task and rating approach allows for a transparent assessment, from designing tasks to rating performances to reporting on CEFR levels, an advantage to other, multilevel approaches (multilevel either in their task or rating design).
In relation to the design factors criteria and raters, our analyses suggest that the rater training and the application of the detailed rating scale and benchmark texts effectively eliminated many differences between how the raters utilized the rating criteria across the different tasks, which speaks positively to the rater training and the assessment instruments in general. It was indispensable to select and train raters appropriately and to continually revise rating scales on the basis of incoming data from pretrials conducted between workshops. This also allowed for the careful selection of benchmark texts, which can be used for detailed discussions and comments so that raters can interpret and apply the rating levels and the detailed analytic criteria in a comparable way. This is pivotal to gain reliable and valid ratings which form the basis of inferences of task difficulty and proficiency estimates. On the basis of our findings, we suggest an integrative, iterative, and data-driven approach to assessment design and rater training for standards-based writing assessment (see CitationHarsch & Martin, 2011).
Although providing analytic ratings did not add much informative value as far as the scaling of the data is concerned, it was nonetheless the detailed analytic approach that ensured the high consistency of the overall rater performance. This is why we would recommend such a detailed approach even if only one overall score is to be reported.
We would argue that the level-specific tasks were generally designed appropriately for a coarse differentiation of performance in that they seemed to elicit ranges of student responses that could be used to distinguish target-level performance from below-target-level performance. However, some of the tasks were somewhat too easy or too difficult for the student samples, suggesting that some tasks need to be better matched to the student samples based on the lessons learned in this study.
Our analyses showed one possible way to derive reliable task difficulty estimates, the hierarchical order of which showed a high level of correspondence with the targeted difficulty level. Based on these findings, we could suggest possible regions where cut-scores in alignment with the CEFR proficiency levels could be set. This is the basis to set cut-scores operationally, using test-centered, consensus-based standard-setting procedures whereby the judges rate the tasks in terms of their targeted CEFR level, based on an analysis of task demands and task characteristics. This in turn represents the validation of the a priori CEFR-level ratings by the test developers. The empirical results presented in this study are currently combined with results from such formal, consensus-based standard-setting procedures (see CitationHarsch, Pant, & Köller, 2010) using the Bookmark method and a novel adaptation of it (e.g., CitationCizek et al., 2004; CitationZieky & Perie, 2006), whereby the representativeness of the tasks in terms of their targeted and preclassified CEFR level was judged to confirm the targeted level or revise the task. As for future research in this area, an examinee-centered standard-setting method is also planned to link the rating scale and the benchmark texts to the CEFR levels by using expert ratings and the assessment grid suggested in the Manual (CitationCouncil of Europe, 2009, p. 187).
Furthermore, modern methods from latent class analysis that statistically determine cut-scores (CitationHenson & Templin, 2008; CitationRupp, Templin, & Henson, 2010) could be used in the future to provide additional empirical data that can complement the consensual approaches.
Notes
1All writing tasks were reanalysed by a team of experts during the formal standard-setting procedure; this analysis of task demands and task characteristics resulted in reclassifying particularly those two tasks that seemingly had been misclassified; they were reclassified at CEFR Level A2, in line with their empirically estimated level.
REFERENCES
- Adams , R. J. 2006 . Reliability as a measurement design effect . Studies in Educational Evaluation , 31 : 162 – 172 .
- Adams , R. J. and Wu , M. L. 2009 . The construction and implementation of user-defined fit tests for use with marginal maximum likelihood estimation and generalized item response models . Journal of Applied Measurement , 10 : 355 – 370 .
- Alderson , J. C. , Figueras , N. , Kuijper , H. , Nold , G. , Takala , S. and Tardieu , C. 2006 . Analysing tests of reading and listening in relation to the common European framework of reference: The experience of the Dutch CEFR construct project . Language Assessment Quarterly , 3 : 3 – 30 .
- Alderson , J. C. and Huhta , A. 2005 . The development of a suite of computer-based diagnostic tests based on the Common European Framework . Language Testing , 22 : 301 – 320 .
- Beck , B. and Klieme , E. , eds. 2007 . Sprachliche Kompetenzen – Konzepte und Messungen. DESI Studie [Linguistic competences—Constructs and their measurement. DESI –Study] , Weinheim, , Germany : Beltz .
- Brennan , R. L. 2001 . Generalizability theory , New York, NY : Springer .
- Brennan , R. L. 2007 . An NCME instructional module on generalizability theory items . Instructional Topics in Educational Measurement , : 225 – 232 .
- Briggs , D. C. and Wilson , M. 2007 . Generalizability in item response modeling . Journal of Educational Measurement , 44 : 131 – 155 .
- Brindley , G. 2000 . “ Task difficulty and task generalizability in competency-based writing assessment ” . In Studies in immigrant English language assessment , Edited by: Brindley , G. Vol. 1 , 125 – 157 . Sydney, , Australia : National Centre for English Language Teaching and Research, Macquarie University .
- Brindley , G. 2001 . “ Investigating rater consistency in competency-based language assessment ” . In Studies in immigrant English language assessment , Edited by: Brindley , G. and Burrows , C. Vol. 2 , 59 – 80 . Sydney, , Australia : National Centre for English Language Teaching and Research, Macquarie University .
- Brown , G. , Anderson , A. , Shillcock , R. and Yule , G. 1984 . Teaching talk: Strategies for production and assessment , Cambridge, , UK : Cambridge University Press .
- Cambridge ESOL: Handbook for teachers. (n.d.) Cambridge, UK: University of Cambridge. https://www.teachers.cambridgeesol.org/ts/digitalAssets/109740_cae_hb_dec08.pdf (https://www.teachers.cambridgeesol.org/ts/digitalAssets/109740_cae_hb_dec08.pdf)
- Chiu , C. W. T. and Wolfe , E. W. 2002 . A method for analyzing sparse data matrices in the generalizability theory framework . Applied Psychological Measurement , 26 ( 3 ) : 321 – 338 .
- Cizek , G. J. , Bunch , M. B. and Koons , H. 2004 . Setting performance standards: Contemporary methods . Educational Measurement: Issues and Practice , 23 ( 4 ) : 31 – 50 .
- Congdon , P. J. and McQueen , J. 2000 . The stability of rater severity in large-scale assessment programs . Journal of Educational Measurement , 37 : 163 – 178 .
- Council of Europe. (2001). Common European reference framework for languages. Strasbourg, France: Author. http://www.coe.int/T/DG4/Portfolio/?L=E&M=/documents_intro/common_framework.html (http://www.coe.int/T/DG4/Portfolio/?L=E&M=/documents_intro/common_framework.html)
- Council of Europe. (2003). Relating language examinations to the Common European Framework of Reference for Languages: Learning, Teaching, Assessment (CEF). Manual, preliminary pilot version. Strasbourg, France: Author. http://www.coe.int/T/DG4/Portfolio/?L=E&M=/documents_intro/Manual.html (http://www.coe.int/T/DG4/Portfolio/?L=E&M=/documents_intro/Manual.html)
- Council of Europe. (2008). The CEFR Grid for Writing Tasks. Strasbourg, France: Author. http://www.coe.int/T/DG4/Portfolio/documents/CEFRWritingGridv3_1_presentation.doc (http://www.coe.int/T/DG4/Portfolio/documents/CEFRWritingGridv3_1_presentation.doc)
- Council of Europe (2009). Manual for Relating Language Examinations to the Common European Framework of Reference for Languages (CEFR). Strasbourg, France: Author. http://www.coe.int/t/dg4/linguistic/Manuel1_EN.asp (http://www.coe.int/t/dg4/linguistic/Manuel1_EN.asp)
- Crick , J. E. and Brennan , R. L. 1983 . Manual for GENOVA: A generalized analysis of variance system (ACT Technical Bulletin No. 43) , Iowa City : American College Testing .
- de Ayala , R. J. 2009 . The theory and practice of item response theory , New York, NY : Guilford .
- De Jong , J. H. What is the role of the Common European Framework of Reference for Languages: Learning, teaching, assessment? . Paper presented at the EALTA conference . May , Kranjska Gora, Slovenia.
- Dutch Grid http://www.lancs.ac.uk/fss/projects/grid/grid.php (http://www.lancs.ac.uk/fss/projects/grid/grid.php)
- Eckes , T. 2005 . Examining rater effects in TestDaF writing and speaking performance assessments: A many-facet Rasch analysis . Language Assessment Quarterly , 2 : 197 – 221 .
- Eckes , T. 2008 . Rater types in writing performance assessments: A classification approach to rater variability . Language Testing , 25 : 155 – 185 .
- Embretson , S. E. and Reise , S. P. 2000 . Item response theory for psychologists , Mahwah, NJ : Erlbaum .
- Frey , A. , Hartig , J. and Rupp , A. A. 2009 . An NCME instructional module on booklet designs in large-scale assessments of student achievement: Theory and practice . Educational Measurement: Issues and Practice , 28 ( 3 ) : 39 – 53 .
- Grabe , W. and Kaplan , R. B. 1996 . Theory & practice of writing: An applied linguistic perspective , Harlow, , England : Pearson Education .
- Hamp-Lyons , L. and Kroll , B. 1996 . Issues in ESL Writing Assessment: An overview . College ESL , 6 ( 1 ) : 52 – 72 .
- Harsch , C. 2007 . Der gemeinsame europäische Referenzrahmen für Sprachen: Leistung und Grenzen [The Common European Framework of Reference for Languages: Strengths and limitations] , Saarbrücken, , Germany : VDM .
- Harsch , C. 2010 . “ Schreibbewertung im Zuge der Normierung der KMK–Bildungsstandards: Der “niveauspezifische Ansatz” und ausgewählte Schritte zu seiner Validierung [Writing assessment in the context of evaluating the German educational standards: The level-specific approach and steps towards its validation] ” . In Fremdsprachliches Handeln beobachten, messen und evaluieren: Neue methodische Ansätze der Kompetenzforschung und Videographie , Edited by: Aguado , K. , Vollmer , H. and Schramm , K. 99 – 117 . Frankfurt, , Germany : Lang .
- Harsch , C. and Martin , G. 2011 . Coding the descriptors-a data–driven approach to improving rating validity , Manuscript submitted for publication .
- Harsch , C. , Neumann , A. , Lehmann , R. and Schröder , K. 2007 . “ Schreibfähigkeit [Writing proficiency] ” . In Sprachliche Kompetenzen: Konzepte und Messungen in der DESI–Studie , Edited by: Beck , B. and Klieme , E. 42 – 62 . Weinheim, , Germany : Beltz .
- Harsch , C. , Pant , A. and Köller , O. , eds. 2010 . Calibrating Standards-based Assessment Tasks for English as a First Foreign Language—Standard-setting procedures in Germany , Münster, , Germany : Waxmann .
- Hawkey , R. A. and Shaw , S.D. 2005 . The Common Scale for Writing project: Implications for the comparison of IELTS band scores and Main Suite exam levels . Research Notes , 19 : 19 – 24 .
- Hayes , J. R. 1996 . “ A new framework for understanding cognition and affect in writing ” . In The science of writing. Theories, methods, individual differences and applications , Edited by: Levy , C. M. and Ransdell , S. 1 – 27 . Hillsdale, NJ : Erlbaum .
- Hayes , J. R. and Flower , S. L. 1980 . “ Identifying the organization of writing processes ” . In Cognitive processes in writing , Edited by: Gregg , L. W. and Steinberg , E. R. 31 – 50 . Hillsdale, NJ : Erlbaum .
- Henson , R. and Templin , J. Implementation of standards setting for an algebra two end-of-course exam using cognitive diagnosis . Paper presented at the annual meeting of the American Educational Research Association . March , New York.
- Hulstijn , J. H. 2007 . The shaky ground beneath the CEFR: Quantitative and qualitative dimensions of language proficiency . The Modern Language Journal , 91 : 663 – 667 .
- Iwashita , N. , McNamara , T. and Elder , C. 2001 . Can we predict task difficulty in an oral proficiency test? Exploring the potential of an information-processing approach to task design . Language Learning , 51 : 401 – 436 .
- Kline , T. J. B. 2005 . “ Classical test theory: Assumptions, equations, limitations, and item analyses ” . In Psychological testing: A practical approach to design and evaluation , Edited by: Kline , T. J. B. 91 – 105 . Thousand Oaks, CA : Sage .
- Knoch , U. 2009 . Diagnostic assessment of writing: A comparison of two rating scales . Language Testing , 26 : 275 – 304 .
- Kuiken , F. and Vedder , I. 2007 . Task complexity and measures of linguistics performance in L2 writing . IRAL , 45 : 261 – 284 .
- Lord , F. M. and Novick , M. R. 1968 . Statistical theories of mental test scores , Boston, MA : Addison-Wesley .
- Lumley , T. 2002 . Assessment criteria in a large-scale writing test: what do they really mean to the raters? . Language Testing , 19 : 246 – 276 .
- Lumley , T. 2005 . Assessing second language writing: the rater's perspective , Frankfurt, , Germany : Lang .
- Mislevy , J. and Rupp , A. A. 2009 . Using item response theory in survey research: Accommodating complex assessment and sampling designs , Manuscript submitted for publication .
- Morrow , K. 2004 . “ Background to the CEF ” . In Insights from the Common European Framework , Edited by: Morrow , K. 3 – 11 . Oxford, , UK : Oxford University Press .
- Norris , J. M. , Brown , J. D. , Hudson , T. D. and Bonk , W. 2002 . Examinee abilities and task difficulty in task-based second language performance assessment . Language Testing , 19 : 395 – 418 .
- North , B. 2004 . “ Relating assessments, examinations, and courses to the CEF ” . In Insights from the Common European Framework , Edited by: Morrow , K. 77 – 90 . Oxford, , UK : Oxford University Press .
- Prabhu , N. S. 1987 . Second language pedagogy , Oxford, , UK : Oxford University Press .
- Preece , D. A. 1990 . Fifty years of Youden squares: A review . Bulletin of the Institute of Mathematics and Its Applications , 26 : 65 – 75 .
- Robinson , P. 2001 . “ Task complexity, cognitive resources, and syllabus design: A triadic framework for examining task influences on SLA ” . In Cognition and second language instruction , Edited by: Robinson , P. 287 – 318 . Cambridge, , UK : Cambridge University Press .
- Rupp , A. A. and Porsch , R. 2010 . “ Standard-setting item pool ” . In Calibrating standards-based assessment tasks for English as a First Foreign Language—Standard-setting procedures in Germany , Edited by: Harsch , C. , Pant , H. A. and Köller , O. 39 – 60 . Münster, , Germany : Waxmann .
- Rupp , A. A. , Templin , J. and Henson , R. 2010 . “ Diagnostic measurement: Theory ” . In methods, and applications , New York, NY : Guilford .
- Rupp , A. A. , Vock , M. , Harsch , C. and Köller , O. 2008 . Developing standards-based assessment items for English as a first foreign language: Context, processes and outcomes in Germany , Münster, , Germany : Waxmann .
- Shaw , S. and Weir , C. J. 2007 . Examining writing in a second language. Studies in Language Testing 26 , Cambridge, , UK : Cambridge University Press and Cambridge ESOL .
- Skehan , P. 1998 . A cognitive approach to language learning , Oxford, , UK : Oxford University Press .
- Skehan , P. and Foster , P. 2001 . “ Cognition and tasks ” . In Cognition and second language instruction , Edited by: Robinson , P. 183 – 205 . Cambridge, , UK : Cambridge University Press .
- Smith , D. 2000 . “ Rater judgments in the direct assessment of competency-based second language writing ability ” . In Studies in immigrant English language assessment , Edited by: Brindley , G. Vol. 1 , 159 – 189 . Sydney, , Australia : National Centre for English Language Teaching and Research, Macquarie University .
- Tankó , G. 2005 . Into Europe: Prepare for modern English exams. The writing handbook , Budapest, , Hungary : Teleki László Foundation .
- Taylor , L. and Jones , N. 2006 . “ Cambridge ESOL exams and the Common European Framework of Reference (CEFR) ” . In Research Notes, 24 , 2 – 5 . Cambridge, , UK : Cambridge ESOL .
- von Davier , M. , Sinharay , S. , Oranje , A. and Beaton , A. 2006 . “ The statistical procedures used in National Assessment of Educational Progress: Recent developments and future directions ” . In Handbook of statistics (Vol. 26): Psychometrics , Edited by: Rao , C. R. and Sinharay , S. 1039 – 1056 . Amsterdam, , the Netherlands : Elsevier .
- Wainer , H. and Thissen , D . 2001 . “ True score theory: The traditional method ” . In Test scoring , Edited by: Thissen , D. and Wainer , H. 23 – 72 . Hillsdale, NJ : Erlbaum . InEds.pp.
- Weigle , S. C. 1998 . Using FACETS to model rater training effects . Language Testing , 15 : 264 – 288 .
- Weigle , S. C. 1999 . Investigating rater / prompt interactions in writing assessment: Quantitative and qualitative approaches . Assessing Writing , 6 : 145 – 178 .
- Weigle , S. C. 2002 . Assessing writing , Cambridge, , UK : Cambridge University Press .
- Weir , C. J. 2005 . Limitations of the Common European Framework for developing comparable examinations and tests . Language Testing , 22 : 281 – 300 .
- Wu , M. L. , Adams , R. J. and Wilson , M. R. 1998 . ACER ConQuest: Generalised item response modeling software , Melbourne : The Australian Council for Educational Research Limited . [Computer program]
- Zieky , M. and Perie , M. 2006 . A primer on setting cut scores on tests of educational achievement , Princeton, NJ : Educational Testing Service .
APPENDIX B