Abstract
The purpose of this article is to provide an overview and practical guide to occupational health professionals concerning the derivation and use of dose estimates in risk assessment for development of occupational exposure limits (OELs) for inhaled substances. Dosimetry is the study and practice of measuring or estimating the internal dose of a substance in individuals or a population. Dosimetry thus provides an essential link to understanding the relationship between an external exposure and a biological response. Use of dosimetry principles and tools can improve the accuracy of risk assessment, and reduce the uncertainty, by providing reliable estimates of the internal dose at the target tissue. This is accomplished through specific measurement data or predictive models, when available, or the use of basic dosimetry principles for broad classes of materials. Accurate dose estimation is essential not only for dose-response assessment, but also for interspecies extrapolation and for risk characterization at given exposures. Inhalation dosimetry is the focus of this paper since it is a major route of exposure in the workplace. Practical examples of dose estimation and OEL derivation are provided for inhaled gases and particulates.
INTRODUCTION: BASIC CONCEPTS OF DOSIMETRY
The dose of a toxicant is the amount (e.g., mg or mg/kg) of that substance that enters the body from exposure by any route. For example, occupational exposure to an airborne substance at a given average airborne concentration (e.g., mg/m3) during the workday would result in a certain inhaled dose, depending on various factors including properties of the substance (e.g., airborne particle size and shape) and of the individual (e.g., exertion level, breathing patterns, and respiratory tract morphology and physiology). Some fraction of the inhaled dose deposits in the respiratory tract and may also be absorbed and transported systemically (which could affect other organs) and may be retained in the body or cleared. The target tissue dose of a toxin is the amount of the total internal dose that reaches a specific tissue and is associated with adverse biological response. Dosimetry study and practice involves determining the amount, rate, and distribution of a substance in the body. Dosimetry models and methods are used in risk assessment in various applications ().
Table 1 Uses of Dosimetry Models and Methods in Quantitative Risk Assessment
One of the most common uses of dosimetry methods in risk assessment involves estimating the internal dose associated with an adverse health effect in animals or humans. (This associated internal dose has also been called a critical effect level, the effective dose, or simply an effect level). Alternatively, the external exposure or the administered dose may be used as an effect level, although the internal dose (measured, or estimated in a validated dosimetry model) may be more predictive of the adverse health outcome. Dosimetry models also enable science-based extrapolation of dose across species since for most substances, human health effects data are not available and animal toxicology study data are used to identify an effect level.
The effect level used in a health risk assessment is often called the point of departure (POD), since it is the point on the dose-response curve which is adjusted to estimate a lower dose (and associated risk) in the derivation of health-based exposure limits. Examples of effect level estimates include the no observed adverse effect level (NOAEL) or the lowest observed adverse effect level (LOAEL) as reported in an animal study, or the benchmark dose (BMD), which is the dose associated with a specified risk (e.g., 10%) of an adverse health effect (or benchmark response) as estimated from modeling the dose-response relationship.(Citation1–Citation3) These effect levels can be estimated for either cancer or noncancer endpoints.
Once the POD is identified, dosimetry models and methods are often used to adjust the POD, which may include normalization of the dose across species (e.g., scaling the parameters affecting internal dose in animals and in humans), temporal extrapolation of the effect level (e.g., as observed in a subchronic animal study to a human working lifetime exposure), or extending the dose-response relationship (e.g., given an understanding of the factors that influence human variability in dose given exposure).
In the case of an inhaled toxicant, the critical parameters for processes that determine the inhaled dose include the portal of entry parameters such as the airway architecture, ventilation rate, and diffusion rate across lung tissues, as well as systemic parameters such as blood flow, metabolism, and elimination rates. The physicochemical properties of the inhaled agent interact dynamically with these parameters and are further influenced by exposure concentration, duration, and frequency. Dosimetry models describe the kinetic or physicochemical processes by using differential equations that are integrated over time to predict internal dose of the toxicant to the respiratory tract and/or internal organs. Several extensive reviews are available for dosimetry modeling of inhaled particles(Citation4–Citation9) and gas uptake.(Citation4,Citation5,Citation10–Citation16) The degree of detail or sophistication in the dosimetry estimation depends on the level of data available and the purpose of the risk assessment (e.g., screening assessment or full risk characterization).
Through the dose-response relationship, dose estimation is an essential component in quantitative risk assessment. In this paper, several practical examples of dose estimation and OEL derivation are provided for inhaled gases and particulates. Key points of emphasis include the following.
Dosimetry approaches can improve the accuracy and reduce the uncertainty of the internal dose estimates used to derive OELs.
Dosimetric adjustments are used to better account for our understanding of toxicokinetics as reflected in interspecies differences, dose rate effects, and population variability.
A variety of dosimetry approaches are available for application to OEL setting, and the tools to implement these approaches are becoming more accessible for routine use, as evidenced through currently published OELs.
HIERARCHICAL MODEL SELECTION CRITERIA
The selection of the dosimetry model to use in a particular risk assessment depends on the goals of the risk assessment (e.g., screening vs. full risk characterization), the degree of understanding about the biological mode of action (MOA),(Citation17) and the level of detail and specificity of the data available. Biological MOA is defined as a sequence of key events and processes, starting with interaction of an agent with a cell, proceeding through functional and anatomical changes, and resulting in toxicity or cancer.(Citation17) In a hierarchy of models (), as the complexity of the models increases, so do the specific data needs; but the benefits include greater precision in the dose estimates and reduction of uncertainty. Default dosimetry models are simpler and require less data, but are associated with greater uncertainty, resulting in less information for decision making. If animal data are used, dosimetry concepts are used to estimate the equivalent dose in humans. An example of a commonly used simple interspecies dosimetric adjustment is that based on body weight scaling (allometry) or body weight to a power.(Citation18–Citation20) To account for metabolic differences across species (related to physiological time) to estimate kinetically-equivalent concentrations for carcinogen risk assessment, body weight to the 3/4 power has been used as a standard default dosimetry adjustment.(Citation17,Citation19–Citation21)
Table 2 Hierarchy of Dosimetry Models and Methods. Ordered from Simpler, Less Specific Approaches to More Complex, Chemical-Specific Approaches.
The selection of dose metric (i.e., the measure of the dose) is another important consideration, and the best dose metrics are those that are most closely associated with the MOA determining the adverse response in the target tissue. A useful dose metric will have sufficient detail to accurately describe the adverse health effect at the duration of exposure for which the dose-response relationship is derived.(Citation4,Citation22,Citation23) For example, peak concentration may be the best predictor of an acute irritant effect, whereas total integrated dose (over a period of time) of a biopersistent substance may better predict its chronic effect. It is also possible that the best dose metric could vary for the same substance that causes more than one adverse effect, depending on the MOA. For example, pulmonary inflammation and granulomas are associated with the mass, surface area, or volume dose of carbon nanotubes,(Citation24,Citation25) whereas abnormal cell division may be better predicted by the number of individual nanostructures of certain dimension that are able to act as microtubules in dividing cells.(Citation26)
When animal data are used for the risk assessment, the PODANIMAL is extrapolated to a human equivalent concentration (PODHEC) to account for interspecies differences that influence the internal dose by applying, for example, a dosimetric adjustment factor (DAF) (see the sections on Calculating the DAF for Particles and Calculating the DAF for Gases and Vapors). The DAF can range from rudimentary algorithms with a minimal number of parameters that accommodate sparse databases, to more sophisticated biologically-based dose-response (BBDR) model structures with detailed mechanistic descriptions of tissue responses.(Citation4,Citation23,Citation27) In addition to the DAF, uncertainty factors are often employed to address uncertainty or variability in required extrapolations. For example, in the case of a noncarcinogen, the PODHEC is often adjusted by uncertainty factors (UFs) that account for variability and uncertainty in its estimation.(Citation28) These UFs include factors for toxicokinetics (influencing dose) and toxicodynamics (influencing response).(Citation29) A physiologically based pharmacokinetic (PBPK) or BBDR model, if available, could replace or reduce some of these factors.(Citation4,Citation6,Citation30)
Considerations in selection of an optimal vs. default model structure include whether the model utilizes chemical- and species-specific mechanistic information or rather relies on categorical, empirical parameters for key determinants such as ventilation rates or mass transfer coefficient to respiratory tract tissues. Default model structures provide a limited description of mechanistic determinants of toxicant disposition and interaction with target tissue eliciting a critical response. Categorical or default values may be used for chemical and species parameters, and the dose metric is at a generic level of detail. Examples of default dosimetry methods include the general reference concentration (RfC) categorical methods.(Citation4,Citation5) In contrast, optimal or preferred model structures describe all significant mechanistic determinants of toxicant disposition and interaction with the target tissue eliciting a critical response; the model parameters are chemical- and species-specific; and the dose metric is described with detail at the level of toxicity at the target organ.(Citation4,Citation23,Citation27) PBPK or BBDR models are examples of optimal model structures (when the available data permit their use) compared to default methods (for more data-poor substances and exposure scenarios) ().
The RfC methods introduced rudimentary models for the default DAF that relied on predominantly empirical descriptions of particle deposition and gas uptake, but nonetheless also represented reduced forms consistent with more sophisticated, detailed structures.(Citation4,Citation5) The mass transfer coefficient used in the RfC methods is analogous to that used in computational fluid dynamic (CFD) and single-path mass transfer models of ozone and formaldehyde uptake,(Citation11,Citation31–Citation33) and the inhalability adjustments and fractional deposition algorithms for particles are analogous to those used in the respiratory tract model of the International Commission on Radiological Protection (ICRP) and multiple-path particle dosimetry (MPPD) models (see the section on Respiratory Tract Models for Particles). What distinguishes these models is the degree of detail and data underlying different descriptions (e.g., delineation of bronchioles and interstitial compartments in the ICRP model, localized gas flux estimates within the upper respiratory tract (URT) for formaldehyde uptake in CFD models).
Some dosimetry models have been developed for wider use and provide user-friendly interfaces (e.g., MPPD animal and human models for deposition and clearance of inhaled particles in the respiratory tract).(Citation34) Other dosimetry models may require more expertise in toxicokinetics and computer modeling such as PBPK or CFD models.(Citation10,Citation15,Citation16,Citation35) Some models employ hybrid CFD-PBPK structures(Citation36) to best capture behavior of gas uptake due to the combined influences of tissue metabolism and airway architecture. PBPK models have been used to improve dosimetry for risk analysis of numerous chemicals, e.g., PBPK models of trichloroethylene,(Citation37) glycol ethers,(Citation38–Citation41) or methyl iodide.(Citation42,Citation43) These PBPK models were used to estimate internal dose and to estimate safe exposure levels or OELs based on, respectively: the sum of the parent compound (trichloroethylene) and metabolite concentration in blood; the average daily area under the blood concentration of metabolites and time curve (AUC) (glycol ethers); and separate dose estimates based on MOA for different endpoints extrapolated from animal data, including maximum concentration of methyl iodide in the brain for neurotoxic effects and fetal blood iodide AUC for developmental effects.
Interaction between a toxic agent and the biological system is complex, and it is preferable to have a comprehensive model that incorporates the mechanisms of chemical disposition, toxicant-target interactions, and tissue responses to describe the toxicity induced by an inhaled substance. Unfortunately, the data to construct such comprehensive models do not exist for most chemicals, in which cases, the more categorical or default approaches are typically used.
AGENT-SPECIFIC DOSIMETRY AND MODEL SELECTION
The principal physicochemical property of an inhaled substance that determines the probability that it will deposit somewhere in the respiratory tract is its physical state, whether the substance is a particulate (i.e., a solid particle or fiber or a liquid aerosol) or it is in a gaseous state (gas or vapor). Once deposited, the internal dose over time will depend on the extent to which the substance is cleared or whether it is retained in the respiratory tract or translocates to another tissue or organ. The main regions of the respiratory tract include: extrathoracic (nasal, pharyngeal, laryngeal), tracheobronchial (airways), and pulmonary or alveolar (gas exchange) (). These regions are also where adverse responses associated with the target tissue dose have been observed (which can be quantified in dose-response modeling for risk assessment). These regions also correspond to the inhalable, thoracic, and respirable particle size fractions for airborne sampling [ACGIH, Appendix C(Citation44)]. Inhalable refers to the fraction of airborne particulate matter that is hazardous when deposited anywhere in the respiratory tract; thoracic is the airborne fraction that is hazardous when deposited in the lung airways (tracheobronchial region) and gas-exchange (pulmonary) regions; and respirable is the airborne fraction that is hazardous when deposited in the gas-exchange (pulmonary) region of the respiratory tract. These concepts, and their implications for risk assessment, are discussed in more detail below for particles and fibers and for gases and vapors.
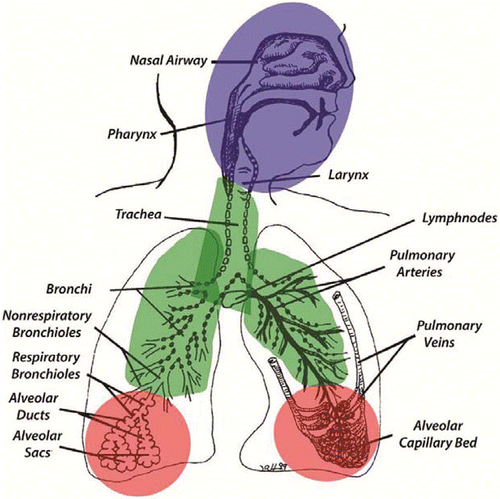
Particles and Fibers
Deposition Mechanisms
The deposition of inhaled substances in the human respiratory tract depends on the aerodynamic diameter for particles larger than approximately 300–500 nm in diameter or on the diffusion diameter and density for smaller particles (including nanoparticles) (). Aerodynamic equivalent diameter is the diameter of a standard-density (1 g/cm3) sphere having the same terminal velocity when settling under gravity as the particle under consideration.(Citation7,Citation45–Citation47) Diffusion equivalent diameter is diameter of a sphere with the same thermal or Brownian diffusivity as the particle under consideration.(Citation7,Citation45–Citation47) The main deposition mechanisms are impaction, sedimentation, and interception for particles with aerodynamic diameters greater than approximately 500 nm, whereas diffusion is the predominant deposition mechanism for smaller particles ().(Citation7,Citation45–Citation47) These competing deposition mechanisms result in minimal deposition efficiency at approximately 500 nm (). For nonspherical particles such as fibers, shape and orientation can be additional factors influencing deposition.(Citation47–Citation49)
Respiratory tract deposition models take these properties into account to predict the deposited dose in each region. In addition to the particle properties, individual factors such as age, gender, breathing pattern (e.g., nasal only or oronasal), and activity level (e.g., resting or exercising) can also influence the ventilation rate and thereby particle deposition in the respiratory tract. Deposition models can also account for these factors(Citation4,Citation7,Citation34) and some consider other sources of inter-individual variability (e.g., lung morphology or clearance rate differences in healthy populations) (see the section on Respiratory Tract Models for Particles).
Clearance and Retention Mechanisms
Clearance of a deposited dose depends on the initial site of deposition within the respiratory tract, physicochemical properties such as solubility, and the time since deposition. Soluble particles dissolve in alveolar lining fluid and enter the blood or lymph directly.(Citation7,Citation8,Citation50) The rates of dissolution and transfer of soluble particles into blood depend primarily on the physicochemical properties of the material, and thus do not differ widely across species.(Citation8) Clearance rates of poorly soluble particles, however, can differ among species due to differences in the rates of mucociliary transport in the conducting airways and of macrophage-mediated clearance from the alveolar region.(Citation51) Poorly soluble particles that deposit in the bronchial region are cleared mainly by cilia that line the airways and move mucous and other fluids toward the mouth, carrying any particles or other exogenous materials along. Particles that deposit in the pulmonary region are cleared by alveolar macrophages that phagocytose (engulf) particles, where they are dissolved or are transported to the tracheobronchial region and cleared by the mucociliary escalator.(Citation50)
Retention refers to the temporal distribution of uncleared particles in the respiratory tract.(Citation52) In humans, two distinct phases of particle retention have been observed. The first phase is thought to represent mucociliary clearance of particles depositing in the tracheobronchial region and is complete within approximately 24 hr, although a particle size-dependent slow clearance fraction has also been demonstrated.(Citation7,Citation53) The second phase—described by retention half-times from approximately 30 to several hundred days—is considered to represent particle clearance within the alveoli (air sacs) and interstitium (connective tissue separating the alveoli) of the pulmonary region.(Citation7) The range of retention half-times in the second phase may represent macrophage-mediated clearance to the mucociliary escalator (for the shorter half-times), while the longer half-times may represent particles that escape alveolar macrophages and enter the interstitium. Retention of inhaled particles is increased when clearance is slower, as observed in some workers (retired coal miners) for whom the clearance rate was shown to be several times slower than that in healthy adults without occupational dust exposure.(Citation54,Citation55) Clearance is also reduced among individuals with some diseases such as chronic obstructive pulmonary disease (including that resulting from occupational dust exposure) or among smokers; and ICRP recommends reducing the clearance rate by a factor of two when estimating the retained particle dose among individuals with COPD.(Citation7)
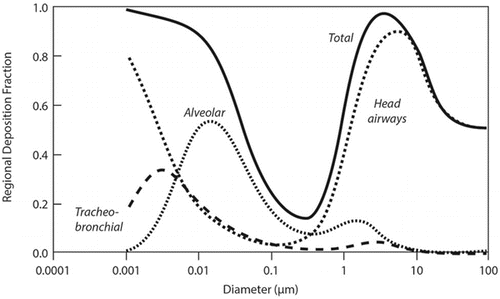
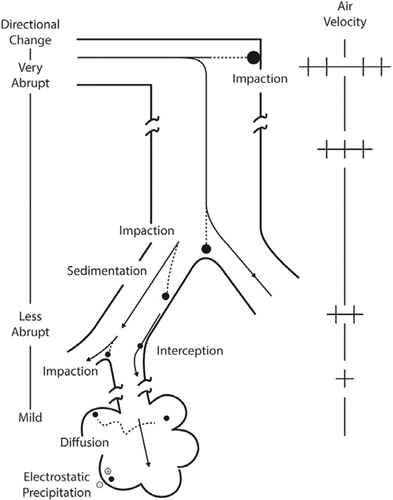
Interspecies Comparison of Clearance and Retention
Similar clearance pathways exist in humans and laboratory animals, although the relative importance of these pathways may vary.(Citation56) Normal pulmonary clearance of particles is approximately 10 times faster in rats than in humans.(Citation57,Citation58) Reasons may include differences in the particle deposition fraction and pattern within the respiratory tract regions, the number of respiratory bronchioles and clearance pathway length, and the alveolar macrophage number and mobility.(Citation59) The location of dust retention in the lungs also differs between rats and primates, with a greater proportion of poorly soluble particles retained within macrophages in alveolar ducts and lumen in rats vs. within the pulmonary interstitium of monkeys and humans.(Citation60,Citation61) When the rat lung dose is sufficient to cause overloading of lung clearance,(Citation62) particle transport to the interstitium increases.(Citation63)
Studies in humans have shown that first-order clearance models underpredict the retained lung dose of particles in individuals with either high exposures (coal miners in the U.S.A. and U.K.)(Citation64) or low exposures (nuclear workers exposed to low levels of radioactive particles in France and the U.S.A.).(Citation65,Citation66) These and other studies (including those showing a slow particle clearance component in bronchial airways) have led to proposed revisions to the ICRP model.(Citation67) In evaluations of several human lung clearance models, a higher-order clearance model that includes sequestration-interstitialization of particles has been shown to best predict the long-term particle retention in humans.(Citation65,Citation66,Citation68–Citation70) The rat-based overload model (i.e., first-order clearance at low exposure and dose-dependent decline in clearance at high exposure) underpredicted human lung burden at low exposure and overpredicted the lung burden at high exposures.(Citation64,Citation68) Because of the faster normal clearance in rats, only at doses that overload lung clearance does the rat achieve lung burdens that are comparable to those observed in workers in dusty jobs (e.g., coal miners).(Citation71,Citation72) Species differences that result in different target tissue doses given exposure are important to consider when extrapolating animal data to humans for risk assessment. Such factors can be taken into account by using validated dosimetry models in order to estimate species-equivalent doses.
Respiratory Tract Models for Particles
One of the earlier models to estimate the dose of inhaled particles in the respiratory tract across species is the U.S. EPA model of the regional deposited dose (RDD) in several animal species and humans.(Citation4) The RDDs can be calculated for the extrathoracic (head/nasal), tracheobronchial, pulmonary, or total respiratory tract depending on the region(s) relevant to the adverse health outcomes. The RDD ratio (RDDR) is used to adjust the animal deposited dose to a human-equivalent concentration for use in a risk assessment. The RDDR software does not provide estimation of particle clearance or retention. Use of the RDDR software has decreased over time with the development of alternative software tools such as the Multiple Path Particle Dosimetry (MPPD) model for humans and rats.(Citation34,Citation73) Nevertheless, many risk assessments are based on POD adjustments derived using this method, especially for other laboratory animal species such as mice and guinea pigs.
The MPPD model is a widely-used dosimetry software that is freely available.(Citation34,Citation73) The MPPD model includes both human and rat respiratory tract models of the deposition and clearance of spherical particles.(Citation74,Citation75) The human model options include several deposition models and the ICRP clearance model.(Citation7) Recently, the lung geometry for the non-human primate was also published.(Citation76) Typical input parameters include particle characteristics (e.g., aerodynamic size distribution parameters and density), breathing frequency and pattern, and exposure concentration and duration. Total, regional, and airway-specific lung doses (e.g., individual lung lobes) are predicted as a function of particle properties and breathing parameters. Tutorials are provided with the MPPD software.
An evaluation of several human lung deposition models showed similar predictions of the total respiratory tract deposition fraction, as well as the deposition fractions in the tracheobronchial and alveolar regions.(Citation75) However, in the models that include information about inter-individual variability in airway morphology, a three-fold difference in airway deposition fraction estimates was observed.(Citation75) The most appropriate model to select depends on both the data available and the purpose for the predictions.
Respiratory Tract Models for Nano-Scale Particles
In general, the deposition efficiency of spherical nanoparticles in the respiratory tract of humans and rats is reasonably well understood.(Citation77) The pulmonary (alveolar) region is a major deposition site, reaching approximately 50% deposition for nanoparticles of 10–20 nm diameter (), while the smaller nanoparticles deposit to a greater extent in the tracheobronchial and head airways.(Citation7) The total respiratory tract deposition efficiency of nanoparticles increases as the particle diameter decreases, exceeding 90% for the smallest nanoparticles ().(Citation7) Improved predictions of nanoparticle deposition efficiency in the human respiratory tract models are achieved by accounting for particle-specific axial diffusion and dispersion effects during transport.(Citation78) These model refinements have been included in a recent version (2.11) of the MPPD model.(Citation34)
The clearance and retention of deposited nanoparticles are less well known, although animal studies have provided some insights. In rats, the long-term pulmonary clearance of nan-oparticles was similar to that of other poorly-soluble, micro-meter-diameter particles, and particles in both size categories were retained in the rat lungs at 6 months.(Citation79,Citation80) However, some nanoparticles can escape alveolar macrophage phagocytosis to a greater extent,(Citation81) allowing for increased access of nanoparticles to the lung interstitium and possibly to the pleura, as observed in mice exposed to single-wall carbon nanotubes.(Citation24) Nanoparticles can readily pass into cells through diffusion or adhesive interactions rather than through endocytotic processes as for larger particles.(Citation82,Citation83) Being similar in size to proteins, nanoparticles can bind with proteins in lung surfactant, which may facilitate their translocation across the air-blood barrier to systemic circulation.(Citation84) The percentage of nanoparticles that translocate from the lungs to other organs (liver, spleen, kidneys, heart, brain) is relatively small (e.g., <1%),(Citation85) although translocation rates depend on both particle size and surface properties–with more rapid translocation (within hours) for carbon than iridium and for smaller than larger nanoparticles.(Citation85–Citation87) PBPK models are beginning to be developed to describe the clearance and translocation of nanoparticles from rodent lungs,(Citation88, 178) although long-term kinetics data are still limited.
In a pathway previously shown for viruses, inhaled nanoparticles including metals have been shown to translocate to the brain via the olfactory nerve in rats.(Citation89–Citation92) Deposition patterns of microscale and nanoscale particles have been evaluated in a human nasal model.(Citation93) In order to estimate the fraction of inhaled particles available for olfactory transport, models been developed to describe the deposition efficiency of nanoparticles in the nasal olfactory region.(Citation94) These revisions are being implemented in the rat model of MPPD.(Citation34)
Respiratory Tract Models for Fibers
A user-friendly model and accompanying software are not yet available for estimating deposited dose and retention of inhaled nonspherical particles in the respiratory tract, these are currently under development as an extension to MPPD. Simplified methods to estimate the fiber deposition fraction in the respiratory tract involve calculating the spherical equivalent diameter of the fiber.(Citation95) Some mathematical models for inhaled fibers have been published that adequately describe the experimental data on fiber deposition and clearance in the respiratory tract.(Citation49,Citation96,Citation97) Orientation of the fiber influences its behavior in air, and can change with air flow (e.g., turbulence). Most available models describe fiber dimensions as cylinders, but additional complexity to best describe the aerodynamics and clearance may arise from the irregular structures of many airborne fibers (i.e., departing from straight cylinder geometry), including chrysotile asbestos and carbon nanotubes.
Earlier models for deposition of fibers in the human respiratory tract developed by Asgharian and Yu(Citation98–Citation100) have been refined to provide further developments such as more realistic mathematical descriptions of lung morphology (e.g., multi-path airway branching in the rat) as well as the fiber orientation in air which influences deposition efficiency.(Citation59,Citation96,Citation101–Citation103) These enhancements permit region-specific estimates of dose, and the rat model indicates hot-spots of fiber deposition in the bifurcations of the airways. Dose-dependent overloading of pulmonary clearance in rats is also taken into account in these models. Fibers that are cleared slowly have a higher probability of being taken up in epithelial cells, and also of being translocated to the mesothelial tissue lining the lungs (target tissue for mesothelioma).
Clearance of fibers from the respiratory tract is influenced by fiber diameter and solubility.(Citation104) Models of fiber cle-arance from the respiratory tract in rats have been developed;(Citation101,Citation103,Citation104) and similar model structures are now addressing fiber deposition and clearance in humans.(Citation49,Citation102) The latter model shows different regional dose estimates by fiber properties and breathing patterns.(Citation49,Citation97)
Calculating the DAF for Particles
As discussed in the section Introduction: Basic Concepts of Dosimetry, an animal exposure associated with an adverse health effect (e.g., POD) is extrapolated to a human-equivalent concentration (HEC) by applying a dosimetric adjustment factor (DAF)(Citation4,Citation6) to account for differences in the factors that influence the internal dose in each species. That is:
(1)
A simple example of deriving a DAF and PODHEC is illustrated here for a respirable toxicant for which the relevant dose metric is assumed to be the average daily deposited lung dose (e.g., mass of soluble particles) based on the U.S. EPA default method.(Citation4) Particle number or surface area could be substituted for mass if the MOA suggests this is a better metric.
The first step is to adjust the rat NOAEL (or other POD) to account for differences between the experimental regimen and the human exposure pattern (e.g., occupational). Assuming the rat exposure was 6 hr/d, 5 d/wk, an adjustment is needed only for the hours per day (to be equivalent to 8 hr/d 5 d/wk workplace exposure):
(2)
The NOAELADJ in this example is used as the PODANIMAL in EquationEquation (1)(1) . The human-equivalent NOAEL (i.e., PODHEC) is then estimated by adjusting for the differences in the rat and human ventilation rate (VE), the particle size-specific deposition fraction (DF) (e.g., mass in the pulmonary region of the respiratory tract), and a normalizing factor (NF) such as the surface area of the respiratory tract target tissue in the URT, tracheobronchial (TB) and/or pulmonary (PU) region,(Citation4,Citation6) as follows:
(3)
Ventilation rates for rats can be calculated from an allometric formula with species-specific parameters, given the body weight (e.g., Tables 4–6 in U.S. EPA(Citation4)). For example, it would be 2.1 L/min for a 300 g rat. The human ventilation rate (corresponding to the reference worker value of 9.6 m3/8-hr d) is 20 L/min.(Citation7) The deposition fraction in the full respiratory tract or target region can be estimated from a dosimetry model (e.g., MPPD)(Citation34,Citation73) by providing the input parameters for the airborne size distribution (e.g., mass median aerodynamic diameter and geometric standard deviation) and the density. (Alternatively, the EPA RDDR software(Citation4) can be used to calculate a single regional deposited dose ratio (RDDR), which incorporates the three factors applied to the NOAELADJ in (EquationEquation 3(3) )).
For example, the pulmonary mass deposition fraction for particles with MMAD of 1 μm, geometric standard deviation (GSD) of 2 μm, and density of 1 g/ml, is estimated to be 0.095 in humans and 0.056 in rats (these and other respiratory tract region deposition fractions are shown in ). The normalizing factor may be selected to account for the species differences, for example, in the alveolar epithelial cell surface area (of 102 and 0.4 m2, respectively, in humans and rats) to normalize the dose of respirable particles that deposit in the pulmonary region. Thus, assuming, for example, an animal NOAEL of 1 mg/m3, the PODHEC is calculated as:
To derive the OEL, the PODHEC would be divided by appropriate uncertainty and variability factors (e.g., factors of 10 or 3.3) (see Dankovic et al.(Citation28)). The toxicokinetic component of the interspecies uncertainty factor may not be needed if the DAF takes sufficient account of the relevant dose adjustment (e.g., an acute effect that depends on the regional deposited dose). However, it should be noted that in this example, the clearance and retention differences in animals and humans have not been taken into account, which would be relevant for chronic health effects associated with respirable poorly soluble particles. Because pulmonary clearance is slower in humans than rats (see the section on Interspecies Comparison of Clearance and Retention), the human-equivalent concentration of poorly-soluble particles would be lower given long-term exposure (e.g., working lifetime). In that case, a human lung dosimetry model (e.g., ICRP;(Citation7) ARA(Citation34)) can be used to estimate directly the exposure concentration (e.g., 8-hr time-weighted average, TWA) associated with a human-equivalent retained lung dose.
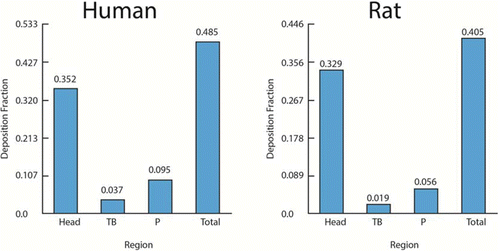
Gases
Gas Uptake Factors
The major factors influencing the uptake and absorption of gases in the respiratory tract include convection, diffusion, dissolution, and chemical reactions.(Citation4,Citation5,Citation11,Citation27,Citation30) Convection induced by a pressure gradient during chest expansion causes the bulk movement of an inhaled gas in the respiratory tract. Molecular diffusion accompanies convection due to local concentration gradients. Solubility and reactivity are the two major properties of gases that influence their biological interaction with the respiratory tract.(Citation4,Citation5,Citation23,Citation27,Citation30) Absorption of gases results in their removal from the airway lumen and affects the concentration gradients in tissues at the site of deposition, blood circulation, and systemic organs. Chemical reactions in the respiratory tract tissues can increase absorption by acting as a sink to drive the concentration gradient. Reactivity includes both the propensity for dissociation of the parent gas in the tissue (e.g., hydrolysis) or its ability to react either spontaneously or via enzymatic reaction in the respiratory tract tissues. Systemic metabolism can also drive the concentration gradient for gases that are removed from the respiratory tract by blood perfusion. Thus, the disposition of gases in the body is determined by their rate of transfer from the airstream to the tissues, the capacity of the tissues (whether respiratory tract or systemic tissues) to retain the material, and the rate of elimination of the parent or metabolite(s) by chemical reaction, exhalation, metabolism and excretion.
Gas Categories
The U.S. EPA gas categorization scheme describes the different modeling structures that might be needed to arrive at dose estimates for different gases or vapors ().(Citation4,Citation5) The goal of these models is a description of the dosimetry of inhaled gases that is commensurate with the available dosimetry data, the physicochemical properties of the gas, the nature and location of the toxicity, and the level of detail regarding the MOA.(Citation23,Citation27) However, it should be recognized that the gas category scheme represents a way to select specific model components from a continuum and that the same broad model structure could be applied to all categories. The framework has motivated many of the modeling efforts described herein for reactive gases in the URT.(Citation105)
Table 3 Gas Categories and Characteristics(Citation4,Citation11)
Category 1 gases (e.g., chlorine, formaldehyde, vinyl acetate) are either water soluble or reactive, and are thus scrubbed out of the inhaled air primarily in the extrathoracic (ET) region at low exposure concentrations. Such gases typically exhibit a proximal to distal penetration and toxicity profile with increasing exposure concentration.(Citation4,Citation11,Citation23,Citation27) High levels of deposition in discrete regions of the nose combined with high reactivity leads to the potential for localized tissue damage. Only a small percentage of a Category 1 gas penetrates beyond the ET at low concentrations typical of ambient exposures, with this penetration greatest during exercise when ventilation rates are the highest.(Citation4,Citation106) Potential high exposures could occur with emergency situations (e.g., fires/explosions that involved release of reactive gases and vapors) and could result in increased penetration to the lower respiratory tract resulting in tissue damage and subsequent clinical effects. Exposure limits based on preventing such acute health effects include the Immediately Dangerous to Life and Health (IDLH) or Acute Exposure Guideline Levels (AEGL) values.
Category 2 gases are intermediate in reactivity and water solubility, which allows them to penetrate more readily beyond the ET and into the bronchi, and to a lesser extent the PU region. Some gases have the potential to accumulate in blood and thus have systemic as well as local effects, or may also deliver the toxicant back to the airway tissues from the endothelial side of the respiratory/circulatory tissues, where it may re-interact with respiratory tract tissues and/or be exhaled.(Citation4,Citation23,Citation27) While not as reactive as Category 1 gases, Category 2 gases such as ozone can still attack cellular constituents in the respiratory epithelium. Their potential to produce damage can be enhanced because they penetrate deeper into the airways where the protective mucus layer is thinner.(Citation4,Citation107) However, mucus is not always effective as a protective barrier since toxic reaction products can be formed in mucus and these can penetrate to underlying epithelial tissue.(Citation108)
Category 3 gases, such as the chlorinated solvents chloroform and trichloroethylene, have limited reactivity in the respiratory epithelium and are generally water insoluble. They are not scrubbed out in the conducting airways but instead readily penetrate to the PU region where they are available to be absorbed into the systemic circulation. The site of toxicity is typically distal to the respiratory tract, although metabolism in airway tissues can lead to portal-of-entry effects.
As mentioned, the chemical characteristics represent a continuum, and these categories are used as a convenience to guide the choice of model structures for use in risk assessment. In particular, distinguishing between Category 2 and Category 3 gases can be challenging. For example, metabolism (a listed characteristic of Category 2 gases) () can also occur to some extent for many of the Category 3 compounds, so that the choice of a model structure in this case will largely depend on the available database to characterize the MOA and the relative contribution of tissue reactions including metabolism to an accurate description of dose
Calculating the DAF for Gases and Vapors
The general concept of adjusting the animal exposure level associated effect (POD) by the factors that influence the internal dose in humans is analogous to that described for inhaled particles (see the section on Calculating the DAF for Particles). For gases and vapors, a simple default DAF is the regional gas deposition ratio (RGDRr). The RGDRr is the ratio of regional gas dose in laboratory animal species to that of humans for region (r) of interest for the toxic effect, i.e., (RGDr)ANIMAL/(RGDr)HUMAN.
(4) where NOAELADJ is defined in EquationEquation (2)
(2) . The type of information needed to calculate an RGDRr includes: the target respiratory tract region(s), physicochemical properties of the gas such as its water solubility and tissue reactivity, species-specific surface areas of the respiratory tract regions of interest, ventilation rates, and the mass transfer coefficient or the blood:air partition coefficient.
Derivation of the default analytical solutions for calculation of the RGDRr are provided in detail in the U.S. EPA methods;(Citation4, Citation109) and the default algorithms for Category 1 and 3 gases provided briefly herein for a single respiratory tract region of a Category 1 gas and for Category 3 gases to provide context for some of the concepts discussed earlier.
As for inhaled particles (see the section on Calculating the DAF for Particles), calculations are made to adjust human occupational to environmental exposures, which include adjustment for an 8 vs. 24-hr day and a 45-year working lifetime vs. a full lifetime (75 or 80 years have been used). Breathing rates to reflect differences in activity level during the day are also typically adjusted as needed. These can be simple arithmetic adjustments or may be done within a dosimetry model.
RGDR for Category 1 Gases
As mentioned earlier, uptake of a gas is dictated by its mass transfer coefficient, consisting of a gas-phase component and tissue-phase component.(Citation4,Citation5,Citation11) Realistic gas uptake models must account for coupled vapor exchange dynamics between the air and tissue phases.(Citation15) In the case of Category 1 gases, description of dose delivered to the tissue in a given region must account for the scrubbing of the gas out of the airstream as it travels through the nose (proximal) to alveolar (distal) airway. The scrubbing is caused by uptake of the gas into the tissue, for example by reactions such as metabolism in the tissue. If the rate of metabolism is fast, as is assumed for reactive gases, then the gas-phase component dictates the overall mass transfer. The amount of gas traversing the gas-phase in the airway of a given region in the respiratory tract is balanced by the mass absorbed at the gas:tissue interface.
From this derivation it can be appreciated that the CFD or single-path mass transfer models are preferred to more accurately describe the species-specific anatomical influences on airflow delivery (mass transfer) and PBPK models for tissue kinetics. A recent analysis of the uptake of reactive gases in different species using CFD models developed after the 1994 RfC methods by the U.S. EPA concluded that the RGDRr for category 1 gases is approximately 1 in most cases.(Citation5)
RGDR for Category 3 Gases.
To adjust for the extrarespiratory dose of category 3 gases in humans, the default RGDR is calculated as the ratio of the blood:gas (air) partition coefficients (Hb/g) in animals and humans, as shown:
(5)
The underlying model structure is that of a ventilation:perfusion model in which the blood:gas partition coefficient is used to modulate the rate of transfer from the alveolar region to the blood. A value of 1 is used as the default RGDR value if the ratio of partition coefficients is greater than 1 or if these partition coefficients are not known. This default was chosen as a protective approach to extrapolate across species,(Citation4) and ensures that the human-equivalent exposure concentration is less than that for the laboratory animal. Blood:air partition coefficients are available in various references (e.g., Gargas et al.(Citation110)).
The use of a constant value (e.g., 1) for the ratio of blood:air partition coefficients in animals and humans is based on the assumption of periodic (i.e., the same pattern week by week) kinetics. This assumption may be reasonable for chronic, continuous exposure to volatile gases (e.g., 24 h/d), although it would not be an adequate assumption for estimating the internal body dose given occupational exposures (e.g., 8-hr/d and 40 hr/wk) if those exposures do not result in steady-state tissue concentrations (i.e., due to an equilibrium between gas uptake and elimination). Blood:air partition coefficients are available in various references (e.g., Gargas et al.(Citation110)). Alternative methods to the use of various default assumptions may include biologically-based algorithms and quantitative structure activity relationships.(Citation111)
AGENT-SPECIFIC EXAMPLES
The process of considering the type of dosimetry calculations needed for a given substance includes basic considerations of its physicochemical properties, the observed site of toxicity, and the route of exposure. This information is typically obtained from literatures searches and other sources (e.g., material safety data sheets or other information from the manufacturer), as well as evaluation of the conditions of use of the specific substance. The purpose of the risk assessment and the amount of data available (e.g., concerning mode of action) are other important considerations in determining whether a simple default approach or a more biologically-based mechanistic model is needed to estimate the dose in humans. Some examples of dosimetry models applied in deriving OELs for inhaled particles and gases are provided in this section.
Working Lifetime Lung Dose Estimation for Poorly Soluble Particles
Poorly soluble particles can cause adverse lung effects that are associated with their biopersistence in lung tissue at sites of particle deposition (e.g., airway bifurcations, alveoli) or translocation (interstitium or pleura). Thus, the relevant dose duration metric is the retained dose in the lungs over a full (45-year) working lifetime (vs. average daily dose as described in the section on Calculating the DAF for Particles). The dose metric of total particle surface area of retained particles in the lungs has been shown to be associated with the MOA evidence (secondary genotoxicity via persistent inflammation) for the adverse lung responses (pulmonary inflammation and cancer).(Citation72) This dose metric describes well the dose-response relationships for the category of low toxicity poorly soluble particles despite differences in particles size, chemical composition, and crystal structure. Based on these findings, a quantitative risk assessment of fine and ultrafine titanium dioxide (TiO2) was conducted to estimate the working lifetime dose of TiO2 in the alveolar region of the lungs that was equivalent to a POD estimated from rat dose-response data.(Citation72)
The key steps in the TiO2 risk assessment are highlighted in . Statistical models were fit to the rat dose-response data from subchronic and chronic inhalation studies. The rat lung dose of TiO2 was measured in these studies (as retained particle mass), and thus no dosimetry modeling was needed for the rat data. The particle surface area lung dose was estimated based on measurements of the specific surface area (i.e., surface area per unit mass). The PODANIMAL was the benchmark dose lower confidence limit (BMDL), which is the 95% lower confidence limit estimate of the BMD. In this example, the BMD and BMDL are estimates of the retained lung dose associated with a 1/1000 excess risk of lung cancer based on the weighted average of three nonlinear models. The PODANIMAL was normalized to a human-equivalent lung dose by adjusting for differences in alveolar epithelial surface area (as shown in the section on Calculating the DAF for Particles). The total surface area dose of retained particles in human lungs was converted back to the retained particle mass lung dose (again using the specific surface area of fine or ultrafine TiO2). This was done in order to utilize the human lung dosimetry models (for which the input air concentrations and predicted lung dose outputs are mass-based) to estimate the working lifetime average concentration that would result in the retained lung burden. The MPPD 2.0 human deposition model,(Citation73) which uses the ICRP clearance model,(Citation7) and the interstitial-sequestration model were used to estimate the working lifetime concentrations.(Citation68) The estimates from these models differed by a factor of 2–3 (with the interstitial-sequestration model predicting lower airborne concentrations associated with the working lifetime retained lung burden).
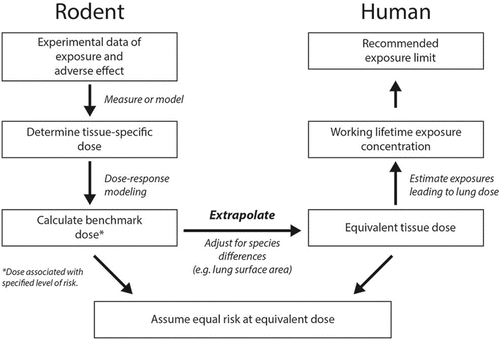
PBPK Model for Systemic Effects of Category 3 Gases: Example of Methylene Chloride
The derivation of the Occupational Safety and Health Administration (OSHA) Permissible Exposure Limit (PEL) for methylene chloride(Citation112) provides a useful example of considerations involved in using internal dosimetry of a Category 3 gas to derive an OEL. In January, 1997, OSHA released a Final Rule reducing the allowable 8-hr TWA exposure to methylene chloride from 500 ppm to 25 ppm. OSHA estimated that this change would reduce the working lifetime cancer risk from 126 to 3.62 excess cancers per 1000 workers. OSHA arrived at these risk estimates using PBPK modeling to derive target tissue dose estimates for lung tumors in mice; derived a cancer potency using the linearized multistage dose-response model with inhaled dose; and then computed the air concentrations-associated risks for exposed workers.(Citation112)
OSHA supported use of PBPK modeling in the methylene chloride rule making based on considerations including the following.(Citation112)
The major pathway for metabolism must be well-described in multiple species and relevant minor pathways must also be described; in the model, metabolism must be adequately described.
The proposed mechanism of action must be plausible and well supported by empirical data.
The putative carcinogenic pathway must contain plausible proximate carcinogens and these pathways must have been measured in test animals (in addition, the kinetic description of that pathway in humans should be supported by (at a minimum) in vitro data; and contributions of other pathways to carcinogenesis adequately modeled or ruled out).
A correlative relationship between the dose surrogate (tissue dose) and tumor response in animals must be demonstrated.
Chemical-specific biochemical parameters must have been measured experimentally, especially those to which the model results were most sensitive.
The models (for test species and human) must have been validated with data not used in model development; the human data must be sufficient to assess uncertainty and variability.
The PBPK model and approach to model application used by OSHA(Citation112) in their quantitative risk assessment () were based largely on earlier methylene chloride modeling efforts by Clewell and colleagues.(Citation113) While this quantitative risk assessment is now over 15 years old, the types of evidence considered and the model development, evaluation, and application processes continue to be highly relevant. PBPK modeling of methylene chloride and its application to quantitative risk assessment remain active areas of scientific endeavor.(Citation114,Citation115)
![Figure 6 Process for OSHA methylene chloride PEL development and risk estimate.(Citation112) In developing their final rule, OSHA used Bayesian analysis to fit their model to multiple pharmacokinetic data sets for mice and humans to arrive at estimates for posterior distributions of PBPK model parameter values. Using these posterior parameter distributions, estimates of the dose surrogates (production of metabolites via the glutathione-S-transferase [GST] pathway in the lung) produced in the key mouse bioassay were computed. OSHA conducted analyses using the human PBPK model with a baseline set of parameters for the GST pathway derived from the mouse values via allometric scaling and an alternative human GST pathway parameter set derived by incorporating human in vitro metabolism data using the parallelogram approach (as described in Reitz et al.(Citation169)). The mouse lung dose surrogates were used as inputs to derive the parameters for the linearized multistage cancer dose-response relationship. The human lung dose surrogates for the new PEL were then computed using the human PBPK model, and working lifetime cancer estimates derived from the 95th percent upper confidence limit of the baseline and alternative dose surrogates.](/cms/asset/35b61441-845d-4a6e-a36b-4638bd422024/uoeh_a_1060328_f0006_oc.gif)
Other proposed occupational exposure limits (OEL) or guidance values have been developed using PBPK modeling. These examples demonstrate the use of PBPK modeling to extrapolate from one population effect level to another (trichloroethylene),(Citation37) for interspecies extrapolation and uncertainty factor refinement (glycol ethers),(Citation40) and discriminating among multiple potential “key” effects under different exposure scenarios (methyl iodide).(Citation43) Additional information on these three examples is provided in section S1 of the online supplemental material. Guidance for the application of such models for developing exposure guidance that is also applicable for occupational scenarios is available.(Citation10)
Hybrid Computational Fluid Dynamics (CFD)-PBPK for Category 2 Gases
Category 2 gases and vapors are defined as being “moderately water soluble and rapidly reversibly reactive or moderately to slowly irreversibly metabolized in respiratory tract tissue.”(Citation4) For such substances, respiratory absorption kinetics are quite complicated in large part because the possibility exists that fractional absorption will change with inspired concentration.(Citation116) This may result from saturation of metabolic pathways and/or depletion of tissue substrates (e.g., glutathione) at high exposure concentrations. This phenomenon has been demonstrated for many vapors.(Citation117–Citation119) For example, due to saturation of local metabolism, URT uptake efficiency for styrene in the mouse (at flow rates approximating the minute ventilation) ranges between 44% to 10% at inspired concentrations of 5 and 200 ppm, respectively.(Citation117) Vapors that are metabolized in the respiratory tract are typically metabolized in the liver as well. Due to saturation of nasal metabolism the fraction of inspired vapor that is metabolized in the respiratory tract versus the liver differs widely with inspired concentrations.(Citation120) Such nonlinear behavior complicates quantitative risk assessment as fractional absorption in the nose and fractional penetration to the lungs at high concentrations used in inhalation toxicity studies may differ substantially from that at the OEL. A benefit of dosimetry modeling is that such nonlinear behavior can be captured and incorporated into the estimation of internal dose during inhalation, thus reducing uncertainty in human risk evaluation.
Two approaches have been used for detailed modeling of Category 2 gases and vapors: a detailed computational fluid dynamics (CFD) approach and a hybrid CFD-physiologically based pharmacokinetic approach (CFD-PBPK). CFD models are extraordinarily useful in understanding local fluxes of vapor into specific anatomical sites and, consequently, are well suited to understand the role of local dose delivery relative to regional injury. The nasal dosimetry modeling of hydrogen sulfide provides an example of detailed local dose predictions via a CFD approach for a Category 2 gas.(Citation121,Citation122) Hybrid CFD-PBPK models typically provide more anatomically defined detail to the tissue phases, allowing for uneven distribution of enzymatic activity between epithelial and submucosal tissues and between respiratory or olfactory epithelium or the nose. These models also allow for differential blood flow throughout the respiratory tract, and by coupling the respiratory tract to the whole body allow for modelling the systemic absorption and redistribution of vapor.(Citation123,Citation124) This approach has been used to describe nasal dosimetry of acetic acid, ethyl acrylate and diacetyl(Citation125–Citation127) and upper- and lower-airway dosimetry of styrene and diacetyl.(Citation120,Citation123,Citation124,Citation128)
Styrene provides an example of a hybrid CFD-PBPK approach. Inhaled styrene is tumorigenic in rodents; and styrene oxide, a cytochrome P450 generated metabolite, has been proposed to be critically involved in the cancer mode of action.(Citation123) A CFD-PBPK hybrid model was developed to estimate dosimetry of styrene and styrene oxide in the bronchiolar airways of the mouse, rat and human ().(Citation123) The nose, conducting airways, terminal bronchioles, and alveoli were each treated individually with each including mucus, epithelium, and submucosa layers (). Vapor was allowed to transfer from air into tissue (and vice versa) based on air phase mass transfer coefficients, and within tissue allowed to diffuse based on its molecular diffusivity. Cytochrome P450 activities were assigned to each tissue based on direct measurements; the model also included phase II detoxification pathways in each tissue. The model was validated against ten independent data sets ranging from close-chamber uptake to tissue measurements of styrene and styrene oxide following styrene exposure. Tissue levels of styrene oxide were then used as an internal dosimeter to interpret and extrapolate animal toxicity data to humans. An advantage of this modeling approach is that the tissue metabolic parameters were measured by direct experimentation and incorporated into specific tissues (e.g., epithelia) in specific locations (e.g., bronchioles). This approach enhances confidence in the predictions for metabolite levels. Moreover, by including the differing metabolism rates in the rodent versus the human, the model reduces uncertainty in extrapolating animal data to humans. A disadvantage of this approach is that it does not provide anatomical detail with respect to local tissue doses within a given airway.
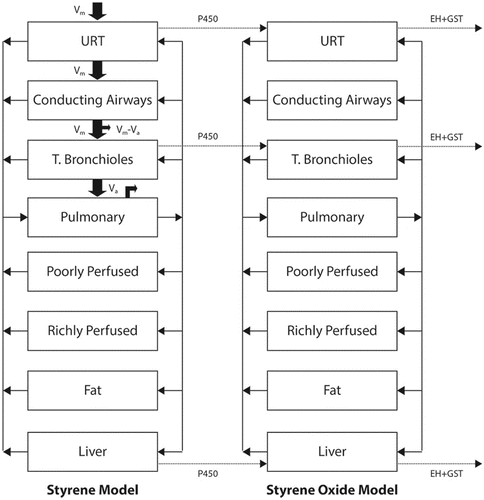
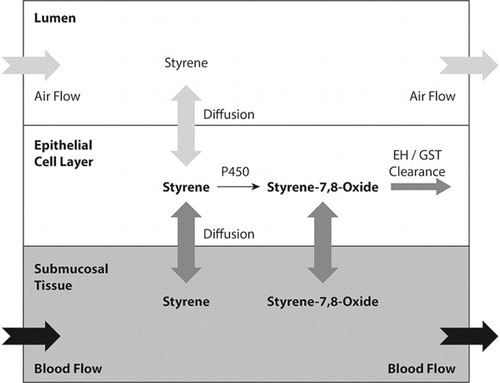
It should be recognized that any modeling approach requires simplifying assumptions and therefore, represents an estimation technique. To date, most, but not all, modeling approaches have assumed respiration can be represented by constant velocity continuous inspiration. Respiration is cyclic, and the absorption/desorption behavior in cyclic respiration may be critical for some vapors.(Citation124,Citation129–Citation131) This is an area for future studies.
It should also be noted that inhalation toxicity studies involve resting, sedentary nose-breathing rats. Particularly for vapors which induce lower airway injury, the sensitive individuals in the workplace may not be resting, but may be exercising, nose-breathing, and hyperventilating. Were this the case, then modeling approaches may need to be extended (after extrapolation of the animal model to humans) to include adjustment from resting to exercising conditions. For one vapor (diacetyl) it has been estimated that delivery to the bronchiolar airways of the exercising human may exceed that in the sedentary rat by as much as 40-fold.(Citation124)
OTHER DOSIMETRY CONSIDERATIONS
As discussed in previous sections, the main uses of dosimetry models and methods in risk assessment include the estimation of the internal, biologically effective dose to the target tissue (in animals or humans), and the adjustment of an animal critical effect level to humans. Several additional and possibly growing uses of dosimetry modeling are discussed in this section.
Route-to-route Dose Extrapolation (to Derive OELs for Inhaled Substances)
Extrapolation of effect levels identified via one route of exposure to another (untested) route of exposure is an approach that allows a risk assessor to more fully utilize the available toxicity database, when test data by the primary route of interest are limited. This application of route-to-route extrapolation is a common practice in OEL derivation as many current ACGIH TLVs; AIHA WEELs, and OELs derived by companies are based on toxicity data derived from oral-dosing studies. Testing by the oral or dermal route generally does not permit contact with the mucous surfaces of the airways, so effects at these locations cannot be adequately evaluated through testing conducted by other routes. For example, an OEL based on robust oral-dosing systemic studies might be very justifiable for protection from systemic effects (e.g., liver toxicity), but such data would not necessarily be informative as to whether sensory irritation of the upper respiratory tract could occur at the derived OEL. Thus, the most common limitation of route-extrapolation for inhalation exposures is the uncertainty surrounding the potential for not capturing portal of entry (i.e., respiratory tract) effects. For this reason, the inhalation effect levels estimated by extrapolation from toxicology studies from other routes of exposure should be compared to any available data for the inhalation route to assess the reasonableness of the derived OEL when considering potential route-specific respiratory tract effects. One approach to address this issue is to weigh the evidence based on mode of action principles and screening bioassays for irritant potential and then adjusting the OEL via the application of uncertainty factors related to database insufficiency. Another approach is to use data directly from irritant screening assays (e.g., RD50 assays) to calculate a provisional OEL and compare this result to the OEL derived for systemic effects. These techniques are described more fully by Maier et al.(Citation132)
A second important consideration for route-to-route extrapolation is the extent to which orally or dermally dosed compounds are systemically available.(Citation133,Citation134) For example, if a compound is poorly absorbed by the oral route, those effects which are observed are attributable to a systemic dose which is lower than the applied dose, and hence the compound is more potent than it appears on the basis of applied dose alone. In addition, if a compound is extensively metabolized at the portal of entry prior to systemic distribution, the lack of systemic effects may be an artifact of the lack of any meaningful delivery to systemic tissues, and a route of exposure which bypasses organs with substantial metabolic capability may produce an effect. Because blood flow from the GI tract passes through the liver prior to the rest of the body, this “first-pass” metabolism effect can yield differences in systematic bioavailability between oral and inhalation exposures to the same administered dose. Some OEL derivation methods (e.g., Naumann et al.(Citation135)) include a specific absorption factor adjustment for this reason.
where OEL is the occupational exposure limit (e.g., in mg/m3), POD is the point of departure (e.g., NOAEL, LOAEL or BMDL) for the test route (e.g., in mg/kg body weight), BW is body weight (e.g., in kg), UFc is the composite uncertainty factor, V is the volume breathed over the time period of concern (e.g., in m3), and BCF is the bioavailability correction factor. Additional examples of using PBPK modeling in route-to-route extrapolation for OEL derivation is provided in section S2 of the online supplemental material.
A recent evaluation of oral-to-inhalation extrapolation concluded that, as a general rule, the route-to-route adjustment based on differences in absorption is not reliable, especially for substances causing local (portal of entry) effects.(Citation136) However, within certain constraints, and particularly when used for screening purposes only (rather than standard setting), route-to-route extrapolation can be useful in the context of evaluating worker exposures. A flow-chart for consideration of route-to-route extrapolation is shown in . For a detailed discussion of the principles and considerations in using route-to-route extrapolation in setting exposure guidance, the reader is referred to the U.S. EPA guidance.(Citation4)
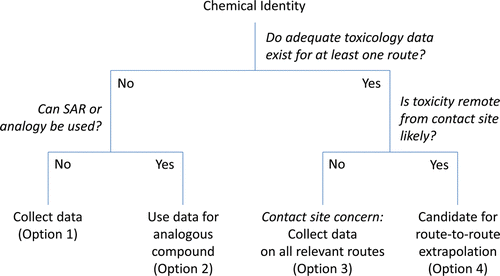
Temporal Considerations in Dose Estimation
Although the development and application of appropriate and validated dosimetry or PBPK models is always preferable for dose estimation, the use of these models (vs. default assumptions) is particularly important for accurate dose estimation when a nonlinear relationship exists between the external exposure and the internal dose to the target tissue. Nonlinearity in the exposure-dose relationship can result from capacity limitation in the uptake, distribution, metabolism, and/or excretion of a toxicant (e.g., saturation of a receptor or enzyme). The dose rate can affect the internal dose, for example, by the overwhelming of clearance mechanisms at a high dose compared to the effective clearance of an equivalent total dose delivered at a lower rate. In these cases, the default dosimetry adjustments may provide poor estimates of the true dose (and response), and thus estimates used to derive human-equivalent POD for the OEL derivation may not be accurate. PBPK and BBDR models that describe the biological mechanisms influencing dose over time can more accurately predict the temporal effects of dose and reduce uncertainty compared to default approaches.(Citation22)
PBPK models have also been proposed for use in adjusting 8-hr OELs for unusual work shift schedules (e.g., >8–10 hr/d or >40-hr workweek),(Citation137) based on the MOA of systemic toxicants. These models require information in humans on the blood:air and tissue:blood partition coefficients, chemical metabolism rate, organ volumes and blood flows, and ventilation rates. The relevant dose metric for most systemic toxicants in these models is either integrated tissue dose or total amount of parent chemical metabolized.
An approach to estimating the cumulative internal dose over time or from multiple routes of exposure) in order to better predict systemic effects is to develop a Biological Exposure Index (BEI).(Citation44,Citation138) BEIs are also used to evaluate effectiveness of workplace exposure controls by providing a measure of internal dose (e.g., through specimens of urine, blood, or exhaled air). Dosimetry modeling (e.g., PBPK) is especially useful in the development and validation of BEIs.(Citation138–Citation140)
Prediction of Internal Dose
Dosimetry modeling can be used to estimate the internal dose at given exposures in a population. PBPK-based models can provide chemical-specific estimates of interspecies and interindividual differences in toxicokinetics (vs. UF of 4 extrapolating from animals to humans or UF of 3.3 within a human population).(Citation30,Citation141,Citation142) For example, the default interspecies UF was reduced to 3 in the U.S. EPA methods due to the use of the DAF.(Citation4) This factor may be reduced further when using more chemical-specific models.(Citation30) However, it is important to note that chemical-specific or MOA class-based estimates may be higher or lower than the default factors.(Citation143,Citation144) Dosimetry models are used to refine the approach many organizations use to apply uncertainty factors for OEL derivation (see Dankovic et al.(Citation28)).
By providing science-based estimates of internal dose, dosimetry models can also be used to evaluate the degree of protection afforded, for example, by the existing (or proposed) OELs or under observed workplace exposure scenarios. This information may be used in margin of exposure (MOE) assessments or in risk characterization. The MOE can be defined as the ratio of a critical effect level (e.g., human-equivalent NOAEL or BMCL) to the estimated exposure(s) in a population. Risk characterization can then describe the health risk to a population at the given exposure(s). For example, Hissink et al. extrapolated from the rat NOAEL for cyclohexane inhalation to a HEC (on the basis of brain concentration of parent compound) using PBPK models.(Citation145) While they note the MOE between the HEC and an OEL, no judgment was made as to the adequacy of the MOE. A similar example has been shown for N-methyl pyrrolidone.(Citation146) Other types of MOE assessments include comparison of the predicted peak concentrations of acetone in richly perfused tissues of microelectronics fabrication workers with the expected concentration of acetone in richly perfused tissues of test animals exposed at the LOAEL for reproductive effects.(Citation147) Delic et al. compared predicted rates of metabolism of chloroform and carbon tetrachloride (generation rate of reactive intermediates) in rodents exposed to the NOAEL to the rates predicted for worker populations exposed at the OELs.(Citation148) Biomarkers were used in an evaluation of an MOE-based assessment compared to a risk assessment using default uncertainty factors.(Citation149)
Most dosimetry models provide predictions for the average conditions, but dosimetry models that describe inter-individual differences in the parameter values can be used to estimate the distribution of doses in a population and better characterize variability in dose estimation. More advanced methods (e.g., Bayesian population analysis using Markov chain Monte Carlo simulation)(Citation150) are useful in providing estimates of the distributions of parameter values in a model, which can be useful in estimating sensitivity to exposures in a population. For example, an evaluation of inter-individual variability in a worker population in solvent doses was performed using PBPK modeling and Monte Carlo simulations.(Citation151) The findings of these analyses are relevant to risk characterization and to the development and evaluation of OELs and BEIs.
Normalization of Doses in Alterative Testing Strategies
In the future, risk assessment may rely more on in vitro (cellular) and in silico (computational) studies, in order to reduce the use of laboratory animals and to increase the information available to evaluate the many substances without OELs (see DeBord et al.(Citation152) for further discussion of these trends). Dosimetry will remain a key element in the interpretation and use of those data, as well as in validation efforts with comparison to in vivo data.
In vitro studies can quickly provide comparative toxicity among a large number of substances.
Challenges to implementation include the comparability of in vitro and in vivo responses and estimation of equivalent doses. Although such studies are still limited, several studies in recent years have shown good agreement between in vitro and in vivo dose-response relationships. For example, studies of poorly-soluble particles have shown good correlation between the in vitro and short-term in vivo inflammation-related responses to poorly-soluble particles when dose is expressed as the total surface dose of particles per surface area of epithelial cells either in the petri dish or in the alveolar region of rat lungs.(Citation153,Citation154) Dosimetry adjustment for nanoparticles has been shown to improve the estimation of the dose reaching the cells and better correlation with acute in vivo endpoints.(Citation155) The In vitro Sedimentation, Diffusion and Dosimetry (ISDD) model accounts for differences in settling velocity based on particle size, density, and specific surface area to improved estimates of particle dose to cells.(Citation156)
Recent studies have also combined in vitro dose-response data with PBPK models to estimate the equivalent in vivo dose and predict response. For example, in vitro toxicity data and in silico kinetic modeling were used to estimate in vivo dose-response curves for developmental toxicity from exposure to glycol ethers.(Citation157) The in vitro effect concentrations (estimated using benchmark modeling) were used as input for the peak blood concentrations into a PBPK model to calculate the doses (mmol/kg) or inhaled exposure concentrations (ppm) of glycol ether that would result in these effect concentrations in the blood. The in vivo and in vitro dose-response curves showed good agreement, and the authors proposed using the in vitro and in silico predicted BMDL values as PODs in risk assessment.
Increased use of in vitro mechanistic and dose-response data in risk assessment for toxicity screening and exposure limit development is an area of active research (e.g., U.S. EPA's NexGen program), with applications to new substances such as nanomaterials.(Citation158) Accurate description of dose in both systems will be key to evaluating the dose-response relationships in vitro and in vivo and to validating these methods for use in risk assessment.(Citation159,Citation160)
DISCUSSION: CHALLENGES TO IMPLEMENTING DOSIMETRY MODELS AND METHODS IN RISK ASESSMENT AND OEL DERIVATION
The application of models and methods that account for dosimetric principles can improve the accuracy of internal dose estimation for quantitative assessment. However, the challenges to the implementing these more complex dosimetry approaches in standard practice include: a precedence for default approaches; the relative ease of use, and the extent of validation required. The hierarchal approach for dosimetry model selection () has been used as standard procedure in the U.S. EPA RfC methods.(Citation4,Citation5) This approach has also been proposed to extend and “harmonize” dosimetry modeling for noncancer and cancer endpoints and for acute versus chronic exposures, with consideration of the MOA.(Citation4,Citation161,Citation162)
Dosimetry models and methods have advanced considerably in recent decades based on the mathematical description of biological observations and principles. Models to predict the deposition and clearance of spherical particles have been well characterized and validated and some are freely available (e.g., MPPD(Citation34)). Modeling software for nonspherical particles and fibers has not yet been developed to the same extent. Gas dosimetry methods include simple interspecies adjustments(Citation4) as well as PBPK models for specific substances, although these models require more specialized expertise. Examples of the available tools and resources for use in dosimetry modeling and risk assessment are provided in .
Table 4 Examples of Available Tools and Resources for Dosimetry Modeling
PBPK models incorporate the biological mechanism data to more accurately estimate the tissue dose. However, to evaluate and validate these models for their applicable uses, standardized criteria and procedures are needed, which include: (1) transparency of model documentation and weight of evidence of the hypothesized mode of action; (2) independent review of the models; and (3) consistent model evaluation approaches.(Citation163,Citation164) To be most useful, PBPK models must also be shown to be robust to dose prediction for a range of conditions given appropriate data.(Citation165) Guidance on the use of PBPK models for developing exposure limits has been published.(Citation10,Citation166)
The options for dosimetry estimation methods in risk assessment will ultimately depend on the type of data available. Limited data necessitates the use of nonspecific default approaches, whereas more detailed and chemical-specific data may enable the use of physiologically-based models. Validation of PBPK and BBDR model predictions, as well as incorporation of population-based distributions of parameters values, are needed for wider acceptance of these models in risk assessment and development of OELs.
ABBREVIATIONS
ACGIH | = | American Conference of Governmental Industrial Hygienists |
BBDR | = | Biologically based dose-response |
BMD | = | Benchmark dose estimate |
BMDL | = | 95% lower confidence limit estimate of the BMD |
BEI | = | Biological Exposure Index |
GST | = | Glutathione S-transferase |
HEC | = | Human-equivalent concentration |
LOAEL | = | Lowest observed adverse effect level |
MOE | = | Margin of exposure |
NOAEL | = | No observed adverse effect level |
OEL | = | Occupational exposure limit |
OSHA | = | Occupational Safety and Health Administration |
PBPK | = | Physiologically based pharmacokinetic |
PEL | = | Permissible exposure limit |
POD | = | Point of departure |
PU | = | Pulmonary |
RDDR | = | Regional deposited dose ratio |
RDGR | = | Regional deposited gas ratio |
RFC | = | Reference concentration |
STEL | = | Short-term exposure limit |
TB | = | Tracheobronchial |
TLV | = | Threshold limit value |
TWA | = | Time-weighted average |
URT | = | Upper respiratory tract |
ACKNOWLEDGMENTS
We would like to thank Dr. Bahman Asgharian, Dr. Harvey Clewell, Dr. David Dankovic, Dr. Kannan Krishnan, and Dr. Andrew Maier for their insightful comments and helpful suggestions on earlier versions of this article.
The findings and conclusions in this article are those of the authors and do not necessarily represent the views or policies of the National Institute for Occupational Safety and Health, the U.S. Environmental Protection Agency, the Department of the Navy, Department of Defense, nor the U.S. Government. This article is not subject to U. S. copyright law.
FUNDING
Dr. Sweeney's contributions to this work were funded by the Naval Medical Research Unit Dayton Work Unit Number 60769.
SUPPLEMENTAL MATERIAL
Supplemental data for this article can be accessed at tandfonline.com/uoeh. AIHA and ACGIH members may also access supplementary material at http://oeh.tandfonline.com/.
JOEH-14-0248-OnLineSupplemental-CLEAN_FINAL.docx
Download MS Word (23.8 KB)REFERENCES
- Wheeler, M., R. Park, A.J. Bailer, and C. Whittaker: Historical context and recent advances in exposure-response estimation for deriving occupational exposure limits. J. Occup. Environ. Hyg. (2015).
- Barnes, D.G., G.P. Daston, J.S. Evans et al. : Benchmark dose workshop: criteria for use of a benchmark dose to estimate a reference dose. Regul. Toxicol. Pharmacol. 21:296–306 (1995).
- U.S. Environmental Protection Agency: “Benchmark Dose Technical Guidance.” 2012. Available at http://www.epa.gov/raf/publications/pdfs/benchmark_dose_guidance.pdf (accessed August 19, 2014).
- U.S. Environmental Protection Agency: “Methods for Derivation of Inhalation Reference Concentrations and Application of Inhalation Dosimetry.” 1994 Available at http://cfpub.epa.gov/ncea/cfm/recordisplay.cfm?deid = 71993 (accessed March 28, 2013).
- U.S. Environmental Protection Agency: “Advances in Inhalation Gas Dosimetry for Derivation of a Reference Concentration (RfC) and Use in Risk Assessment.” 2012. Available at http://cfpub.epa.gov/ncea/cfm/recordisplay.cfm?deid = 244650 (accessed March 28, 2013).
- Jarabek, A.M., B. Asgharian, and F.J. Miller: Dosimetric adjustments for interspecies extrapolation of inhaled poorly soluble particles (PSP). Inhal. Toxicol. 17(7-8):317–334 (2005).
- International Commission on Radiological Protection (ICRP): Human Respiratory Tract Model for Radiological Protection. Tarrytown, NY: Elsevier Science Ltd., 1994.
- Dahl, A.R., R.B. Schlesinger, H.D. Heck, M.A. Medinsky, and G.W. Lucier: Comparative dosimetry of inhaled materials: differences among animal species and extrapolation to man. Fundam. Appl. Toxicol. 16(1):1–13 (1991).
- Schlesinger, R.B.: Comparative deposition of inhaled aerosols in experimental animals and humans: a review. J. Toxicol. Environ. Health 15(2):197–214 (1985).
- U.S. Environmental Protection Agency: “Approaches for the Application of Physiologically Based Pharmacokinetic (PBPK) Models and Supporting Data in Risk Assessment.” 2006. Available at http://cfpub.epa.gov/ncea/cfm/recordisplay.cfm?deid = 157668 (accessed March 28, 2013).
- Hanna, L.M., S.R. Lou, S. Su, and A.M. Jarabek: Mass transport analysis: inhalation rfc methods framework for interspecies dosimetric adjustment. Inhal. Toxicol. 13(5):437–463 (2001).
- Dahl, A.R.: Dose concepts for inhaled vapors and gases. Toxicol. Appl. Pharmacol. 103:185–197 (1990).
- Overton, J.H., and F.J. Miller: Absorption of Inhaled Reactive Gases. In Toxicology of the Lung, D.E. Gardner, J.D. Crapo, and E.J. Massaro (eds.). New York: Raven Press, 1988. pp. 477–507.
- Fiserova-Bergerova, V.: Modeling of Inhalation Exposure to Vapors: Uptake, Distribution, and Elimination. Boca Raton, FL: CRC Press Inc., 1983.
- Asgharian, B., O.T. Price, J.D. Schroeter, J.S. Kimbell, L. Jones, and M. Singal: Derivation of mass transfer coefficients for transient uptake and tissue disposition of soluble and reactive vapors in lung airways. Ann. Biomed. Eng. 39(6):1788–1804 (2011).
- Corley, R.A., S. Kabilan, A.P. Kuprat et al. : Comparative computational modeling of airflows and vapor dosimetry in the respiratory tracts of rat, monkey, and human. Toxicol. Sci. 128(2):500–516 (2012).
- U.S. Environmental Protection Agency: “Guidelines for Carcinogen Risk Assessment.” 2005. Available at http://www.epa.gov/raf/publi-cations/pdfs/CANCER_GUIDELINES_FINAL_3-25-05.PDF (accessed August 19, 2014).
- Boxenbaum, H.: Interspecies scaling, allometry, physiological time, and the ground plan of pharmacokinetics. J. Pharmacokinet. Biopharm. 10(2):201–227 (1982).
- O’Flaherty, E.J.: Interspecies conversion of kinetically equivalent doses. Risk Anal. 9(4): 587–598 (1989).
- U.S. Environmental Protection Agency: “Recommended Use of Body Weight¾ as Default Method in Derivation of the Oral Refernce Dose.” 2011. Available at http://www.epa.gov/raf/publications/interspecies-extrapolation.htm (accessed August 19, 2014).
- U.S. Environmental Protection Agency: EPA request for comments on draft report on cross-species scaling factor for cancer risk assessment. Fed. Regist. 57:24152 (1992).
- Jarabek, A.M.: Interspecies extrapolation based on mechanistic determinants of chemical disposition. Human Ecol. Risk Assess. 1(5):641–662 (1995).
- Jarabek, A.M.: Consideration of temporal toxicity challenges current default approaches. Inhal. Toxicol. 7:927–946 (1995).
- Mercer, R.R., A.F. Hubbs, J.F. Scabilloni et al.: Pulmonary fibrotic response to aspiration of multi-walled carbon nanotubes. Part. Fibre Toxicol. 8:21 (2011).
- Pauluhn, J.: Poorly soluble particulates: searching for a unifying denominator of nanoparticles and fine particles for DNEL estimation. Toxicology 279(1–3):176–188 (2011).
- Sargent, L.M., A.A. Shvedova, A.F. Hubbs et al. : Induction of aneuploidy by single-walled carbon nanotubes. Environ. Mol. Mutagen. 50(8):708–717 (2009).
- Jarabek, A.M.: The application of dosimetry models to identify key processes and parameters for default dose-response assessment approaches. Toxicol. Lett. 79:171–184 (1995).
- Dankovic, D.A., B.D. Naumann, M.L. Dourson, Maier, and L. Levy: The scientific basis of uncertainty factors used in setting occupational exposure limits. J. Occup. Environ. Hyg. (2015).
- WHO: Harmonization Project Document no.2: Chemical-specific Adjustment Factors for Interspecies Differences and Human Variability: Guidance Document for Use of Data in Dose/Concentration-response Assessment. Geneva: World Health Organization, 2005.
- Bogdanffy, M.S., and A.M. Jarabek: Understanding mechanisms of inhaled toxicants: implications for replacing default factors with chemical-specific data. Toxicol. Lett. 82–83:919–932 (1995).
- Kimbell, J.S., J.H. Overton, R.P. Subramaniam et al. : Dosimetry modeling of inhaled formaldehyde: binning nasal flux predictions for quantitative risk assessment. Toxicol. Sci. 64(1):111–121 (2001).
- Kimbell, J.S., R.P. Subramaniam, E.A. Gross, P.M. Schlosser, and K.T. Morgan: Dosimetry modeling of inhaled formaldehyde: comparisons of local flux predictions in the rat, monkey, and human nasal passages. Toxicol. Sci. 64(1):100–110 (2001).
- Overton, J.H., J.S. Kimbell, and F.J. Miller: Dosimetry modeling of inhaled formaldehyde: the human respiratory tract. Toxicol. Sci. 64(1):122–134 (2001).
- ARA: “Multiple-path particle deposition (MPPD 2.1, beta version): A model for human and rat airway particle dosimetry,” by Applied Research Associates, Inc., Raleigh, NC.
- Schroeter, J.D., J.S. Kimbell, E.A. Gross et al.: Application of physiological computational fluid dynamics models to predict interspecies nasal dosimetry of inhaled acrolein. Inhal. Toxicol. 20(3):227–243 (2008).
- Schroeter, J.D., G.J. Garcia, and J.S. Kimbell: A computational fluid dynamics approach to assess interhuman variability in hydrogen sulfide nasal dosimetry. Inhal. Toxicol. 22(4):277–286 (2010).
- Simon, T.W.: Combining physiologically based pharmacokinetic modeling with Monte Carlo simulation to derive an acute inhalation guidance value for trichloroethylene. Regul. Toxicol. Pharmacol. 26(3):257–270 (1997).
- Gargas, M.L., T.R. Tyler, L.M. Sweeney et al.: A toxicokinetic study of inhaled ethylene glycol ethyl ether acetate and validation of a physiologically based pharmacokinetic model for rat and human. Toxicol. Appl. Pharmacol. 165(1):63–73 (2000).
- Gargas, M.L., T.R. Tyler, L.M. Sweeney et al.: A toxicokinetic study of inhaled ethylene glycol monomethyl ether (2-ME) and validation of a physiologically based pharmacokinetic model for the pregnant rat and human. Toxicol. Appl. Pharmacol. 165(1):53–62 (2000).
- Sweeney, L.M., T.R. Tyler, C.R. Kirman et al. : Proposed occupational exposure limits for select ethylene glycol ethers using PBPK models and Monte Carlo simulations. Toxicol. Sci. 62(1):124–139 (2001).
- Kirman, C.R., L.M. Sweeney, M.L. Gargas, and J.H. Kinzell: Evaluation of possible modes of action for acute effects of methyl iodide in laboratory animals. Inhal. Toxicol. 21(6):537–551 (2009).
- Mileson, B.E., L.M. Sweeney, M.L. Gargas, and J. Kinzell: Iodomethane human health risk characterization. Inhal. Toxicol. 21(6):583–605 (2009).
- Sweeney, L.M., C.R. Kirman, S.A. Gannon, K.D. Thrall, M.L. Gargas, and J.H. Kinzell: Development of a physiologically based pharmacokinetic (PBPK) model for methyl iodide in rats, rabbits, and humans. Inhal. Toxicol. 21(6):552–582 (2009).
- ACGIH: Threshold Limit Values for Chemical Substances and Physical Agents and Biological Exposure Indices. Cincinnati, OH: American Conference of Governmental Industrial Hygienists, 2014.
- Bohning, D.E., and M. Lippmann: Particle Deposition and Pulmonary Defense Mechanisms. In Environmental and Occupational Medicine, W.N. Rom (ed.). Boston: Little, Brown and Company, 1992. pp. 171–182.
- Hinds, W.C.: Aerosol Technology: Properties, Behavior, and Measurement of Airborne Particles. New York: John Wiley & Sons, Inc., 1999. pp. 233–259.
- Schulz, H., P. Brand, and J. Heyder: Particle Deposition in the Respiratory Tract. In Particle-lung Interactions, P. Gehr and J. Heyder (eds.). New York: Marcel Dekker Inc., 2000. pp. 229–290.
- Vincent, J.H.: Health-related aerosol measurement: a review of existing sampling criteria and proposals for new ones. J. Environ. Monit. 7(11):1037–1053 (2005).
- Sturm, R., and W. Hofmann: A theoretical approach to the deposition and clearance of fibers with variable size in the human respiratory tract. J. Haz. Mater. 170(1):210–218 (2009).
- Schlesinger, R.B.: Interaction of gaseous and particulate pollutants in the respiratory tract: mechanisms and modulators. Toxicology 105(2–3):315–325 (1995).
- Snipes, M.B.: Long-term retention and clearance of particles inhaled by mammalian species. Crit. Rev. Toxicol. 20(3):175–211 (1989).
- Lioy, P.J., M. Lippmann, and R.F. Phalen: Rationale for particle size-selective air sampling. Ann. Am. Conf. Ind. Hyg. 11:27–34 (1984).
- Stahlhofen, W., G. Rudolf, and A.C. James: Intercomparison of experimental regional aerosol deposition data. J. Aerosol. Med. 2(3):285–308 (1989).
- Freedman, A.P., and S.E. Robinson: Noninvasive Magnetopneumographic Studies of Lung Dust Retention and Clearance in Coal Miners. In Respirable Dust in the Mineral Industries: Health Effects, Characterization and Control, R.L. Frantz and R.V. Ramani (eds.). University Park, PA: The Pennsylvania State University, 1988. pp. 181–186.
- Bailey, M.R., F.A. Fry, and A.C. James: Long-term retention of particles in the human respiratory tract. J. Aerosol. Sci. 16(4):295–305 (1985).
- Miller, F.J.: Dosimetry of particles in laboratory animals and humans in relationship to issues surrounding lung overload and human health risk assessment: a critical review. Inhal. Toxicol. 12(1–2):19–57 (2000).
- Kreyling, W.G.: Interspecies comparison of lung clearance of insoluble particles. J. Aerosol. Med. 3(Suppl 1): S93–S110 (1990).
- Snipes, M.B.: Current Information on Lung Overload in Nonrodent Mammals: Contrast with Rats. In Particle Overload in the Rat Lung and Lung Cancer: Implications for Human Risk Assessment. Proceedings of a Conference Held at the Massachusetts Institute of Technology on March 29 and 30, 1995, J.L. Mauderly and R.J. McCunney (eds.). Washington, DC: Taylor and Francis, 1996. pp. 91–109.
- Yu, C.P.: Extrapolation Modeling of Particle Deposition and Retention from Rats to Humans. In Particle Overload in the Rat Lung and Lung Cancer: Implications for Human Risk Assessment. Proceedings of a Conference Held at the Massachusetts Institute of Technology on March 29 and 30, 1995, J.L. Mauderly and R.J. McCunney (eds.). Washington, DC: Taylor and Francis, 1996. pp. 279–291.
- Nikula, K.J., K.J. Avila, W.C. Griffith, and J.L. Mauderly: Lung tissue responses and sites of particle retention differ between rats and cynomolgus monkeys exposed chronically to diesel exhaust and coal dust. Fundam. Appl. Toxicol. 37(1):37–53 (1997).
- Nikula, K.J., V. Vallyathan, F.H. Green, and F.F. Hahn: Influence of exposure concentration or dose on the distribution of particulate material in rat and human lungs. Environ. Health Perspect. 109(4):311–318 (2001).
- Morrow, P.E.: Possible mechanisms to explain dust overloading of the lungs. Fundam. Appl. Toxicol. 10(3):369–384 (1988).
- Muhle, H., O. Creutzenberg, B. Bellmann, U. Heinrich, and R. Mermelstein: Dust overloading of lungs: investigations of various materials, species-differences, and irreversibility of effects. J. Aerosol. Med. 3(Suppl 1):S111–S128 (1990).
- Kuempel, E.D., and C.L. Tran: Comparison of human lung dosimetry models: implications for risk assessment. Ann. Occup. Hyg. 46(Suppl 1):337–341 (2002).
- Gregoratto, D., M.R. Bailey, and J.W. Marsh: Modelling particle retention in the alveolar-interstitial region of the human lungs. J. Radiol. Prot. 30(3):491–512 (2010).
- Gregoratto, D., M.R. Bailey, and J.W. Marsh: Particle clearance in the alveolar-interstitial region of the human lungs: model validation. Radiat. Prot. Dosimetry 144(1–4):353–356 (2011).
- Bailey, M.R., E. Ansoborlo, R.A. Guilmette, and F. Paquet: Updating the ICRP human respiratory tract model. Radiat. Prot. Dosimetry 127(1-4):31–34 (2007).
- Kuempel, E.D., E.J. O’Flaherty, L.T. Stayner, R.J. Smith, F.H. Green, and V. Vallyathan: A biomathematical model of particle clearance and retention in the lungs of coal miners. I. Model development. Regul. Toxicol. Pharmacol. 34(1):69–87 (2001).
- Kuempel, E.D., C.L. Tran, R.J. Smith, and A.J. Bailer: A biomathematical model of particle clearance and retention in the lungs of coal miners. II. Evaluation of variability and uncertainty. Regul. Toxicol. Pharmacol. 34(1):88–101 (2001).
- Sweeney, L.M., A. Parker, L.T. Haber, C.L. Tran, and E.D. Kuempel: Application of Markov chain Monte Carlo analysis to biomathematical modeling of respirable dust in US and UK coal miners. Regul. Toxicol. Pharmacol. 66(1):47–58 (2013).
- International Agency for Research on Cancer (IARC): “IARC Monographs on the Evaluation of Carcinogenic Risks to Humans. Volume 93: Carbon Black, Titanium Dioxide, and Talc.” 2010. Available at http://monographs.iarc.fr/ENG/Monographs/vol93/mono93.pdf (accessed March 27, 2013).
- NIOSH: “Current Intelligence Bulletin 63: Occupational Exposure to Titanium Dioxide.” 2011. Available at http://www.cdc.gov/niosh/docs/2011-160/pdfs/2011-160.pdf (accessed July 16, 2012).
- CIIT Centers for Health Research, and RIVM: “Multiple-path particle dosimetry (MPPD, version 2.0): A model for human and rat airway particle dosimetry,” by CIIT Centers for Health Research and the National Institute for Public Health and the Environment (RIVM), Research Triangle Park, NC, 2006.
- Anjilvel, S., and B. Asgharian: A multiple-path model of particle deposition in the rat lung. Fundam. Appl. Toxicol. 28(1):41–50 (1995).
- Asgharian, B., W. Hofmann, and R. Bergmann: Particle deposition in a multiple-path model of the human lung. Aerosol. Sci. Tech. 34:332–339 (2001).
- Asgharian, B., O. Price, G. McClellan et al.: Development of a rhesus monkey lung geometry model and application to particle deposition in comparison to humans. Inhal. Toxicol. 24(13):869–899 (2012).
- Kuempel, E.D.: Estimating Nanoparticle Dose in Humans: Issues and Challenges. In Nanotoxicology: Characterization, Dosing and Health Effects, N.A. Monteiro-Riviere and C.L. Tran (eds.). New York: Informa Healthcare, 2007. pp. 141–152.
- Asgharian, B., and O.T. Price: Deposition of ultrafine (nano) particles in the human lung. Inhal. Toxicol. 19(13):1045–1054 (2007).
- Semmler, M., J. Seitz, F. Erbe et al. : Long-term clearance kinetics of inhaled ultrafine insoluble iridium particles from the rat lung, including transient translocation into secondary organs. Inhal. Toxicol. 16(6–7):453–459 (2004).
- Semmler-Behnke, M., S. Takenaka, S. Fertsch et al.: Efficient elimination of inhaled nanoparticles from the alveolar region: evidence for interstitial uptake and subsequent reentrainment onto airways epithelium. Environ. Health Perspect. 115(5):728–733 (2007).
- Takenaka, S., W. Möller, M. Semmler-Behnke et al.: Efficient internalization and intracellular translocation of inhaled gold nanoparticles in rat alveolar macrophages. Nanomedicine (Lond) 7(6):855–865 (2012).
- Geiser, M., and W.G. Kreyling: Deposition and biokinetics of inhaled nanoparticles. Part. Fibre Toxicol. 7:2 (2010).
- Geiser, M., B. Rothen-Rutishauser, N. Kapp et al.: Ultrafine particles cross cellular membranes by nonphagocytic mechanisms in lungs and in cultured cells. Environ. Health Perspect. 113(11):1555–1560 (2005).
- Fertsch-Gapp, S., M. Semmler-Behnke, A. Wenk, and W.G. Kreyling: Binding of polystyrene and carbon black nanoparticles to blood serum proteins. Inhal. Toxicol. 23(8):468–475 (2011).
- Kreyling, W.G., M. Semmler-Behnke, J. Seitz et al.: Size dependence of the translocation of inhaled iridium and carbon nanoparticle aggregates from the lung of rats to the blood and secondary target organs. Inhal. Toxicol. 21(Suppl 1):55–60 (2009).
- Kreyling, W.G., M. Semmler, F. Erbe et al.: Translocation of ultrafine insoluble iridium particles from lung epithelium to extrapulmonary organs is size dependent but very low. J. Toxicol. Environ. Health A 65(20):1513–1530 (2002).
- Oberdörster, G., Z. Sharp, V. Atudorei et al.: Extrapulmonary translocation of ultrafine carbon particles following whole-body inhalation exposure of rats. J. Toxicol. Environ. Health A 65(20):1531–1543 (2002).
- Sweeney L.M., L. MacCalman, L.T. Haber, E.D. Kuempel, and C.L. Tran: Bayesian evaluation of a physiologically-based pharmacokinetic (PBPK) model of long-term kinetics of metal nanoparticles in rats. Regul. Toxicol. Pharmacol. 73(1):151–163 (2015).
- Oberdörster, G., E. Oberdörster, and J. Oberdörster: Nanotoxicology: an emerging discipline evolving from studies of ultrafine particles. Environ. Health Perspect. 113(7):823–839 (2005).
- Oberdörster, G., Z. Sharp, V. Atudorei et al.: Translocation of inhaled ultrafine particles to the brain. Inhal. Toxicol. 16(6–7):437–445 (2004).
- Sunderman, F.W., Jr.: Nasal toxicity, carcinogenicity, and olfactory uptake of metals. Ann. Clin. Lab. Sci. 31(1):3–24 (2001).
- Elder, A., R. Gelein, V. Silva et al.: Translocation of inhaled ultrafine manganese oxide particles to the central nervous system. Environ. Health Perspect. 114(8):1172–1178 (2006).
- Wang, S.M., K. Inthavong, J. Wen, J.Y. Tu, and C.L. Xue: Comparison of micron- and nanoparticle deposition patterns in a realistic human nasal cavity. Respir. Physiol. Neurobiol. 166(3):142–151 (2009).
- Garcia, G.J., and J.S. Kimbell: Deposition of inhaled nanoparticles in the rat nasal passages: dose to the olfactory region. Inhal. Toxicol. 21(14):1165–1175 (2009).
- Baron, P.A.: Application of the thoracic sampling definition to fiber measurement. Am. Ind. Hyg. Assoc. J. 57(9):820–824 (1996).
- Yu, C.P., and B. Asgharian: Mathematical Models of Fiber Deposition in the Lung. In Fiber Toxicology, D.B. Warheit (ed.). San Diego: Academic Press Inc, 1993. pp. 73–98.
- Sturm, R.: A computer model for the simulation of fiber–cell interaction in the alveolar region of the respiratory tract. Comput. Biol. Med. 41:565–573 (2011).
- Asgharian, B., and C.P. Yu: Deposition of inhaled fibrous particles in the huan lung. J. Aerosol. Med. 1(1):37–50 (1988).
- Asgharian, B., and C.P. Yu: Deposition of fibers the rat lung. J. Aerosol. Sci. 20(3):355–366 (1989).
- Asgharian, B., and C.P. Yu: A simplified model of interceptional deposition of fibers at airway bifurcations. Aerosol. Sci. Tech. 11:80–88 (1989).
- Asgharian, B., and S. Anjilvel: A multiple-path model of fiber deposition in the rat lung. Toxicol. Sci. 44(1):80–86 (1998).
- Yu, C.P., Y.J. Ding, L. Zhang et al. : Retention modeling of refractory ceramic fibers (RCF) in humans. Regul. Toxicol. Pharmacol. 25(1):18–25 (1997).
- Yu, C.P., L. Zhang, G. Oberdorster, R.W. Mast, L.R. Glass, and M.J. Utell: Clearance of refractory ceramic fibers (RCF) from the rat lung: development of a model. Environ. Res. 65(2):243–253 (1994).
- Tran, C.L., A.D. Jones, B.G. Miller, and K. Donaldson: Modeling the retention and clearance of manmade vitreous fibers in the rat lung. Inhal. Toxicol. 15(6):553–587 (2003).
- Andersen, M.E., and A.M. Jarabek: Nasal tissue dosimetry issues and approaches for “Category 1” gases: A report on a meeting held in Research Triangle Park, NC, 11–12 February, 1998. Inhal. Toxicol. 13(5):415–436 (2001).
- Nodelman, V., and J.S. Ultman: Longitudinal distribution of chlorine absorption in human airways: a comparison to ozone absorption. J. Appl. Physiol. 87(6):2073–2080 (1999).
- Chang, L.Y., Y. Huang, B.L. Stockstill et al.: Epithelial injury and interstitial fibrosis in the proximal alveolar regions of rats chronically exposed to a simulated pattern of urban ambient ozone. Toxicol. Appl. Pharmacol. 115(2):241–252 (1992).
- Ferng, S.F., C.E. Castro, A.A. Afifi, E. Bermúdez, and M.G. Mustafa: Ozone-induced DNA strand breaks in guinea pig tracheobronchial epithelial cells. J. Toxicol. Environ. Health 51(4):353–367 (1997).
- U.S. Environmental Protection Agency: “2009 Status Report: Advances in Inhalation Dosmimetry of Gases and Vapors with Portal of Entry Effects in the Upper Respiratory Tract.” 2009. Available at http://cfpub.epa.gov/ncea/cfm/recordisplay.cfm?deid = 212131 (accessed August 19, 2014).
- Gargas, M.L., R.J. Burgess, D.E. Voisard, G.H. Cason, and M.E. Andersen: Partition coefficients of low-molecular-weight volatile chemicals in various liquids and tissues. Toxicol. Appl. Pharmacol. 98(1):87–99 (1989).
- Peyret, T., and K. Krishnan: QSARs for PBPK modelling of environmental contaminants. SAR QSAR Environ. Res. 22(1–2):129–169 (2011).
- OSHA: “Occupational Exposure to Methylene Chloride.” 1997. Available at http://www.osha.gov/pls/oshaweb/owadisp.show_document?p_table=FEDERAL_REGISTER&p_id=13600 (accessed August 19, 2014).
- Clewell, H.J., J.M. Gearhart, and M.E. Andersen: Analysis of the Metabolism of Methylene Chloride in the B6C3F1 Mouse and its Implications for Human Carcinogenic Risk. Wright-Patterson Air Force Base, OH: Department of the Navy, Naval Medical Research Institute, submitted to Mr. Tom Hall, Occupational Safety and Health Administration (OSHA), Division of Consumer Affairs, Washington, DC, January 15, 1993. OSHA Docket #H-071, Exhibit #96., 1993.
- David, R.M., H.J. Clewell, P.R. Gentry, T.R. Covington, D.A. Morgott, and D.J. Marino: Revised assessment of cancer risk to dichloromethane II. Application of probabilistic methods to cancer risk determinations. Regul. Toxicol. Pharmacol. 45(1):55–65 (2006).
- Evans, M.V., and J.C. Caldwell: Evaluation of two different metabolic hypotheses for dichloromethane toxicity using physiologically based pharmacokinetic modeling for in vivo inhalation gas uptake data exposure in female B6C3F1 mice. Toxicol. Appl. Pharmacol. 244(3):280–290 (2010).
- Morris, J.B.: Biologically-based modeling insights in inhaled vapor absorption and dosimetry. Pharmacol. Ther. 136(3):401–413 (2012).
- Morris, J.B.: Uptake of styrene in the upper respiratory tract of the CD mouse and Sprague-Dawley rat. Toxicol. Sci. 54(1):222–228 (2000).
- Morris, J.B., and A.R. Buckpitt: Upper respiratory tract uptake of naphthalene. Toxicol. Sci. 111(2):383–391 (2009).
- Struve, M.F., V.A. Wong, M.W. Marshall, J.S. Kimbell, J.D. Schroeter, and D.C. Dorman: Nasal uptake of inhaled acrolein in rats. Inhal. Toxicol. 20(3):217–225 (2008).
- Sarangapani, R., H.J. Clewell, G. Cruzan, and M.E. Andersen: Comparing respiratory-tract and hepatic exposure-dose relationships for metabolized inhaled vapors: a pharmacokinetic analysis. Inhal. Toxicol. 14(8):835–854 (2002).
- Schroeter, J.D., J.S. Kimbell, M.E. Andersen, and D.C. Dorman: Use of a pharmacokinetic-driven computational fluid dynamics model to predict nasal extraction of hydrogen sulfide in rats and humans. Toxicol. Sci. 94(2):359–367 (2006).
- Schroeter, J.D., J.S. Kimbell, A.M. Bonner, K.C. Roberts, M.E. Andersen, and D.C. Dorman: Incorporation of tissue reaction kinetics in a computational fluid dynamics model for nasal extraction of inhaled hydrogen sulfide in rats. Toxicol. Sci. 90(1):198–207 (2006).
- Sarangapani, R., J.G. Teeguarden, G. Cruzan, H.J. Clewell, and M.E. Andersen: Physiologically based pharmacokinetic modeling of styrene and styrene oxide respiratory-tract dosimetry in rodents and humans. Inhal. Toxicol. 14(8):789–834 (2002).
- Gloede, E., J.A. Cichocki, J.B. Baldino, and J.B. Morris: A validated hybrid computational fluid dynamics-physiologically based pharmacokinetic model for respiratory tract vapor absorption in the human and rat and its application to inhalation dosimetry of diacetyl. Toxicol. Sci. 123(1):231–246 (2011).
- Frederick, C.B., M.L. Bush, L.G. Lomax et al.: Application of a hybrid computational fluid dynamics and physiologically based inhalation model for interspecies dosimetry extrapolation of acidic vapors in the upper airways. Toxicol. Appl. Pharmacol. 152(1):211–231 (1998).
- Frederick, C.B., L.G. Lomax, K.A. Black et al.: Use of a hybrid computational fluid dynamics and physiologically based inhalation model for interspecies dosimetry comparisons of ester vapors. Toxicol. Appl. Pharmacol. 183(1):23–40 (2002).
- Morris, J.B., and A.F. Hubbs: Inhalation dosimetry of diacetyl and butyric acid, two components of butter flavoring vapors. Toxicol. Sci. 108(1):173–183 (2009).
- Morris, J.B., B. Asgharian, and J.S. Kimbell: Upper Airway Dosimetry of Gases, Vapors, and Particulate Matter in Rodents. In Toxicology of the Nose and Upper Airways, J.B. Morris and D.J. Shusterman (eds.). New York: Informa Healthcare, 2010. pp. 99–115.
- Gerde, P., and A.R. Dahl: A model for the uptake of inhaled vapors in the nose of the dog during cyclic breathing. Toxicol. Appl. Pharmacol. 109(2):276–288 (1991).
- Johanson, G.: Modelling of respiratory exchange of polar solvents. Ann. Occup. Hyg. 35(3):323–339 (1991).
- Kumagai, S., and I. Matsunaga: A lung model describing uptake of organic solvents and roles of mucosal blood flow and metabolism in the bronchioles. Inhal. Toxicol. 12(6): 491–510 (2000).
- Maier, A., T.J. Lentz, K. MacMahon, L.T. McKernan, C. Whittaker, and P.A. Schulte: State-of-the-science: The evolution of occupational exposure limit derivation and application. J. Occup. Environ. Hyg. (2015).
- Bessems, J.G., and L. Geraets: Proper knowledge on toxicokinetics improves human hazard testing and subsequent health risk characterisation. A case study approach. Regul. Toxicol. Pharmacol. 67:325–334 (2013).
- Peters, S.A.: Identification of intestinal loss of a drug through physiologically based pharmacokinetic simulation of plasma concentration-time profiles. Clin. Pharmacokinet. 47(4):245–259 (2008).
- Naumann, B.D., P.A. Weideman, R. Sarangapani, S.C. Hu, R. Dixit, and E.V. Sargent: Investigations of the use of bioavailability data to adjust occupational exposure limits for active pharmaceutical ingredients. Toxicol. Sci. 112(1):196–210 (2009).
- Rennen, M.A., T. Bouwman, A. Wilschut, J.G. Bessems, and C.D. Heer: Oral-to-inhalation route extrapolation in occupational health risk assessment: a critical assessment. Regul. Toxicol. Pharmacol. 39(1):5–11 (2004).
- Andersen, M.E., M.G. MacNaughton, H.J. Clewell III, and D.J. Paustenbach: Adjusting exposure limits for long and short exposure periods using a physiological pharmacokinetic model. Am. Ind. Hyg. Assoc. J. 48(4):335–343 (1987).
- Fiserova-Bergerova, V.: Application of toxicokinetic models to establish biological exposure indicators. Ann. Occup. Hyg. 34(6):639–651 (1990).
- Droz, P.O.: The use of simulation models for setting BEIs for organic solvents. Annals of the American Conference of Governmental Industrial Hygiesnists 12:339–350 (1985).
- Verner, M.A., R. McDougall, and G. Johanson: Using population physiologically based pharmacokinetic modeling to determine optimal sampling times and to interpret biological exposure markers: The example of occupational exposure to styrene. Toxicol. Lett. 213(2):299–304 (2012).
- International Programme on Chemical Safety (IPCS): “Environmental Health Criteria 170: Assessing Human Health Risks of Chemicals: Derivation of Guidance Values for Health-based Exposure Limits.” 1994. Available at http://www.inchem.org/documents/ehc/ehc/ehc170.htm (accessed March 28, 2013).
- Lipscomb, J.C., M.E. Meek, K. Krishnan, G.L. Kedderis, H. Clewell, and L. Haber: Incorporation of pharmacokinetic and pharmacodynamic data into risk assessments. Toxicol. Mech. Meth. 14(3):145–158 (2004).
- Hattis, D., P. Banati, and R. Goble: Distributions of individual susceptibility among humans for toxic effects. How much protection does the traditional tenfold factor provide for what fraction of which kinds of chemicals and effects? Ann. NY Acad. Sci. 895:286–316 (1999).
- Hattis, D., and K. Silver: Human interindividual variability–a major source of uncertainty in assessing risks for noncancer health effects. Risk Anal. 14(4):421–431 (1994).
- Hissink, A.M., B.M. Kulig, J. Kruse et al.: Physiologically based pharmacokinetic modeling of cyclohexane as a tool for integrating animal and human test data. Int. J. Toxicol. 28(6):498–509 (2009).
- Poet, T.S., C.R. Kirman, M. Bader, C. van Thriel, M.L. Gargas, and P.M. Hinderliter: Quantitative risk analysis for N-methyl pyrrolidone using physiologically based pharmacokinetic and benchmark dose modeling. Toxicol. Sci. 113(2):468–482 (2010).
- Hallock, M.F., K.S. Hammond, E.M. Kenyon, T.J. Smith, and E.R. Smith: Assessment of task and peak exposures to solvents in the microelectronics fabrication industry. Appl. Occup. Environ. Hyg. 8:945–954 (1993).
- Delic, J.I., P.D. Lilly, A.J. MacDonald, and G.D. Loizou: The utility of PBPK in the safety assessment of chloroform and carbon tetrachloride. Regul. Toxicol. Pharmacol. 32(2):144–155 (2000).
- Aylward, L.L., R.A. Becker, C.R. Kirman, and S.M. Hays: Assessment of margin of exposure based on biomarkers in blood: an exploratory analysis. Regul. Toxicol. Pharmacol. 61(1):44–52 (2011).
- Bernillon, P., and F.Y. Bois: Statistical issues in toxicokinetic modeling: a bayesian perspective. Environ. Health Perspect. 108 Suppl 5:883–893 (2000).
- Thomas, R.S., P.L. Bigelow, T.J. Keefe, and R.S. Yang: Variability in biological exposure indices using physiologically based pharmacokinetic modeling and Monte Carlo simulation. Am. Ind. Hyg. Assoc. J. 57(1):23–32 (1996).
- DeBord, D.G., L. Burgon, S. Edwards et al.: Systems biology and biomarkers of early effects for occupational exposure limit setting. J. Occup. Environ. Hyg. (2015).
- Donaldson, K., P.J. Borm, G. Oberdörster, K.E. Pinkerton, V. Stone, and C.L. Tran: Concordance between in vitro and in vivo dosimetry in the proinflammatory effects of low-toxicity, low-solubility particles: the key role of the proximal alveolar region. Inhal. Toxicol. 20(1):53–62 (2008).
- Rushton, E.K., J. Jiang, S.S. Leonard et al.: Concept of assessing nanoparticle hazards considering nanoparticle dosemetric and chemical/biological response metrics. J. Toxicol. Environ. Health A 73(5):445–461 (2010).
- Teeguarden, J.G., P.M. Hinderliter, G. Orr, B.D. Thrall, and J.G. Pounds: Particokinetics in vitro: dosimetry considerations for in vitro nanoparticle toxicity assessments (Erratum in: Toxicol. Sci. 97(2)). Toxicol. Sci. 95(2):300–312 (2007).
- Hinderliter, P.M., K.R. Minard, G. Orr et al.: ISDD: A computational model of particle sedimentation, diffusion and target cell dosimetry for in vitro toxicity studies. Part. Fibre Toxicol. 736 (2010).
- Louisse, J., E. de Jong, J.J. van de Sandt et al.: The use of in vitro toxicity data and physiologically based kinetic modeling to predict dose-response curves for in vivo developmental toxicity of glycol ethers in rat and man. Toxicol. Sci. 118(2):470–484 (2010).
- Han, X., N. Corson, P. Wade-Mercer et al.: Assessing the relevance of in vitro studies in nanotoxicology by examining correlations between in vitro and in vivo data. Toxicology 297(1–3):1–9 (2012).
- Gangwal, S., J.S. Brown, A. Wang et al.: Informing selection of nanomaterial concentrations for ToxCast in vitro testing based on occupational exposure potential. Environ. Health Perspect. 119(11):1539–1546 (2011).
- Oberdörster, G.: Nanotoxicology: in vitro-in vivo dosimetry. Environ. Health Perspect. 120(1):A13; author reply A13 (2012).
- Wiltse, J.A., and V.L. Dellarco: U.S. Environmental Protection Agency's revised guidelines for carcinogen risk assessment: evaluating a postulated mode of carcinogenic action in guiding dose-response extrapolation. Mutat. Res. 464(1):105–115 (2000).
- Bogdanffy, M.S., G. Daston, E.M. Faustman et al.: Harmonization of cancer and noncancer risk assessment: proceedings of a consensus-building workshop. Toxicol. Sci. 61(1):18–31 (2001).
- Loizou, G., M. Spendiff, H.A. Barton et al.: Development of good modelling practice for physiologically based pharmacokinetic models for use in risk assessment: the first steps. Regul. Toxicol. Pharmacol. 50(3):400–411 (2008).
- McLanahan, E.D., H.A. El-Masri, L.M. Sweeney et al.: Physiologically based pharmacokinetic model use in risk assessment–Why being published is not enough. Toxicol. Sci. 126(1):5–15 (2012).
- Clewell, R.A., and H.J. Clewell III: Development and specification of physiologically based pharmacokinetic models for use in risk assessment. Regul. Toxicol. Pharmacol. 50(1):129–143 (2008).
- International Programme on Chemical Safety (IPCS): “Harmonization Project Document No. 9: Characterization and Application of Physiologically Based Pharmacokinetic Models in Risk Assessment.” 2010. Available at http://www.inchem.org/documents/harmproj/harmproj/harmproj9.pdf (accessed August 19, 2014).
- Oberdörster, G.: Dosimetric principles for extrapolating results of rat inhalation studies to humans, using an inhaled Ni compound as an example. Health Phys. 57(Suppl 1):213–220 (1989).
- Kuempel, E.D., C.L. Tran, V. Castranova, and A.J. Bailer: Lung dosimetry and risk assessment of nanoparticles: evaluating and extending current models in rats and humans. Inhal. Toxicol. 18(10):717–724 (2006).
- Reitz, R.H., A.L. Mendrala, and F.P. Guengerich: In vitro metabolism of methylene chloride in human and animal tissues: use in physiologically based pharmacokinetic models. Toxicol. Appl. Pharmacol. 97(2):230–246 (1989).
- Gerrity, T.R., C.J. Henry, and R. Bronaugh: Summary report of the workshops on principles of route-to-route extrapolation for risk assessment. In Principles of Route-to-Route Extrapolation for Risk Assessment, Proceedings of the Workshops: . March and July, Hilton Head, SC and Durham, NC, T.R. Gerrity and C.J. Henry (eds.). New York: Elsevier Science Publishing Co. Inc., 1990. pp. 1–12.
- Asgharian, B., O.T. Price, M. Oldham et al.: Computational modeling of nanoscale and microscale particle deposition, retention and dosimetry in the mouse respiratory tract. Inhal. Toxicol. 26(14):829–842 (2014).
- International Commission on Radiological Protection (ICRP): Basic Anatomical and Physiological Data for Use in Radiological Protection Reference Values. Tarrytown, NY: Elsevier Science Ltd, 2002.
- Brown, R.P., M.D. Delp, S.L. Lindstedt, L.R. Rhomberg, and R.P. Beliles: Physiological parameter values for physiologically based pharmacokinetic models. Toxicol. Ind. Health 13(4):407–484 (1997).
- Davies, B., and T. Morris: Physiological parameters in laboratory animals and humans. Pharm. Res. 10(7):1093–1095 (1993).
- Mercer, R.R., M.L. Russell, V.L. Roggli, and J.D. Crapo: Cell number and distribution in human and rat airways. Am. J. Respir. Cell Mol. Biol. 10(6):613–624 (1994).
- Stone, K.C., R.R. Mercer, P. Gehr, B.L. Stockstill, and J.D. Crapo: Allometric relationships of cell numbers and size in the mammalian lung. Am. J. Respir. Cell Mol. Biol. 6:235–243 (1992).
- ACGIH: Particle Size-Selective Sampling in The Workplace. Report of the ACGIH Technical Committee on Air Sampling Procedures. Cincinnati, OH: American Conference of Governmental Industrial Hygienists, 1984.
- Bachler G., N. von Goetz, and K. Hungerbühler: A physiologically based pharmacokinetic model for ionic silver and silver nanoparticles. Int. J. Nanomedicine 8:3365–3382 (2013).