ABSTRACT
Although circular RNAs (circRNAs) play important roles in regulating gene expression, the understanding of circRNAs in livestock animals is scarce due to the significant challenge to characterize them from a biological sample. In this study, we assessed the outcomes of bovine circRNA identification using six enrichment approaches with the combination of ribosomal RNAs removal (Ribo); linear RNAs degradation (R); linear RNAs and RNAs with structured 3′ ends degradation (RTP); ribosomal RNAs coupled with linear RNAs elimination (Ribo-R); ribosomal RNA, linear RNAs and RNAs with poly (A) tailing elimination (Ribo-RP); and ribosomal RNA, linear RNAs and RNAs with structured 3′ ends elimination (Ribo-RTP), respectively. RNA-sequencing analysis revealed that different approaches led to varied ratio of uniquely mapped reads, false-positive rate of identifying circRNAs, and the number of circRNAs per million clean reads (Padj <0.05). Out of 2,285 and 2,939 highly confident circRNAs identified in liver and rumen tissues, respectively, 308 and 260 were commonly identified from five methods, with Ribo-RTP method identified the highest number of circRNAs. Besides, 507 of 4,051 identified bovine highly confident circRNAs had shared splicing sites with human circRNAs. The findings from this work provide optimized methods to identify bovine circRNAs from cattle tissues for downstream research of their biological roles in cattle.
Introduction
Circular RNAs (circRNAs), together with long noncoding RNAs (lncRNAs) and microRNAs (miRNAs) are classes of regulatory RNA molecules that play various roles in regulating many biological processes via transcriptional, posttranscriptional and translational regulations [Citation1–3]. CircRNAs are generated from pre-mRNAs by back-splicing, which ligate the 5ʹ end of the exons to their 3ʹ end or downstream exons in a reversed orientation to yield closed loop RNA molecules with the ligated site termed as back-splicing junction site (BSJ) [Citation4]. This noncanonical splicing form is the key feature to distinguish circRNAs from other RNA molecules. The circRNAs can be categorized as exonic circRNA (ecircRNA, contain single or multiple exons) and intronic circRNA (ciRNA, contain only intronic sequences) [Citation5]. CircRNAs mainly exist in the cytoplasm and contain many miRNA responsive elements (7–9 nt in length), which can function as miRNAs sponges or decoys, protecting mRNAs from miRNA-targeted degradation [Citation6–8]. As such, circRNAs are considered as competing endogenous RNAs that regulate mRNA transcripts by competing with shared miRNAs. It has also been reported that the sequences of circRNAs are highly conserved between human and mice, which mediates cell proliferation, migration, and invasion [Citation9], highlighting that these circRNAs play critical and conserved roles in biological functions in mammals.
Although numerous circRNAs have been identified in human (421,501 circRNAs) and mice (175,273 circRNAs) [Citation10], less amount of them have been identified in bovine (12,595 circRNAs) [Citation11]. To date, the characterization of circRNAs is still challenging because they only account for 0.002% to 0.03% of total RNA molecules [Citation12], even with RNA-sequencing. Consequently, the resolution and sensitivity of commonly used RNA-sequencing methods like poly(A) enrichment or ribosomal RNA (rRNA) depletion are inefficient to comprehensively detect circRNAs within a cell or a tissue. Therefore, specific enrichment methods are needed to increase the relative amount of circRNAs before sequencing. One approach to enrich circRNAs from biological samples is to degrade the abundant linear RNAs while preserving the loop portion of lariat RNAs and circRNAs using Ribonuclease R (RNase R) [Citation13]. Another approach is to include polyadenylation and poly (A)+ RNA depletion following RNase R treatment to deplete the transcripts with highly structured 3ʹ ends like small nuclear RNA (snRNA), histone mRNAs [Citation14] and lariat RNAs [Citation15]. However, none of these methods have been used to characterize circRNAs from bovine tissues, and it is unclear what is the best approach to identify the bovine circRNAs. In the present study, we aimed to determine the optimal approach to characterize bovine circRNAs by comparing six different circRNA enrichment methods before RNA-sequencing using rumen and liver tissue from cattle. Liver and rumen tissues were selected as they are two key metabolic tissues in cattle.
Results
Enrichment of bovine circRNAs using different approaches
Six approaches were used for the enrichment and identification of circRNAs from bovine liver and rumen tissues prior to library construction and RNA-sequencing (). Specifically, these approaches included 1) ribosomal RNAs removal using Ribo-Zero Kit (Ribo), 2) linear RNAs degradation using RNase R (R), 3) degradation of linear RNAs and RNAs with structured 3′ ends using RNase R followed by poly (A) tailing (polyadenylation) and then poly (A)+ RNA depletion (RTP), 4) the combination of ribosomal RNAs removal and linear RNAs degradation using Ribo-Zero Kit and RNase R (Ribo-R), 5) the combination of ribosomal RNA removal, linear RNAs degradation and RNAs with poly (A) tailing degradation using Ribo-Zero Kit, RNase R and poly (A)+ RNA depletion (Ribo-RP), and 6) the combination of ribosomal RNAs removal, linear RNAs and RNAs with structured 3′ ends degradation using Ribo-Zero Kit, RNase R, poly (A) tailing and poly (A)+ RNA depletion (Ribo-RTP).
Figure 1. The workflow of circRNAs enrichment and sequencing. Total RNA was extracted from three biological replicate sample of cattle liver and rumen tissue. circRNA enriched libraries were constructed using the methods: Ribo: rRNA depletion using Ribo-zero (Green line); R: RNase R (Brown line); RTP: RNase R + Tailing + Poly (A) depletion (Blue line); Ribo-R: Ribo-zero + RNase R (Yellow line); Ribo-RP: Ribo-zero + RNase R + Poly (A) depletion (Red line); Ribo-RTP: Ribo-zero + RNase R + Tailing + Poly (A) depletion (Purple line). The libraries were sequenced using Novaseq 6000 S4 PE100 platform (Illumina). After quality control and alignment, remaining reads were used for circRNAs identification. BSJ: Back-splicing junction.
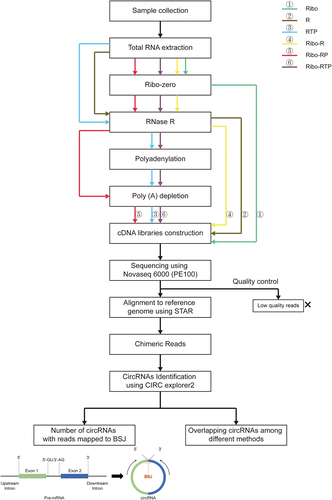
The obtained RNA sequence data and quality control details are shown in . Approximately 31 million clean reads per sample were obtained from Ribo method (28–32 million reads), ~75 million clean reads per sample using R method (61–84 million reads), ~73 million clean reads per sample using RTP method (54–87 million reads), ~79 million clean reads per sample using Ribo-R method (73–85 million reads), ~73 million clean reads per sample using Ribo-RP method (59–83 million reads), and ~ 52 million clean reads per sample using Ribo-RTP method (45–62 million reads). After alignment to the cattle reference genome (Bos taurus ARS-UCD1.2), the ratio of uniquely mapped reads (the proportion of uniquely mapped read counts out of total clean read counts) were compared. There was a statistically significant difference in the ratio of uniquely mapped reads between Ribo and Ribo-R methods (66.94 ± 1.26% vs. 76.84 ± 2.43%, Padj <0.05) () for the liver datasets, but no difference (χ2 = 2.767, asymptotic sig = 0.60) were detected in the ratio of uniquely mapped reads among enrichment methods for the rumen dataset.
Figure 2. The ratio of uniquely mapped reads (a proportion of the reads uniquely mapped to the reference genome out of all clean reads) from different circRNA enrichment methods. The Chi-Square value and the adjusted p value (Asymptotic Sig) of the Kruskal-Wallis H test are shown. Ribo: rRNA depletion using Ribo-zero (Green box); R: RNase R (Brown box); RTP: RNase R + Tailing + Poly (A) depletion (Blue box); Ribo-R: Ribo-zero + RNase R (Yellow box); Ribo-RP: Ribo-zero + RNase R + Poly (A) depletion (Red box); Ribo-RTP: Ribo-zero + RNase R + Tailing + Poly (A) depletion (Purple box).
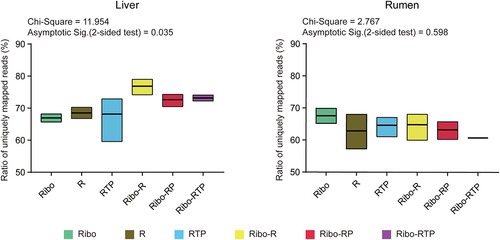
Table 1. Summary of sequencing data and the number of identified circRnas.
Removal of both rRNAs and linear RNAs increased the number of identified circRNAs with low false positive
Totally 50,837 circRNAs were identified in the present study (Supplemental Table 1). Among them, some circRNAs had BSJ site with only one read mapped (BSJ read = 1), and these circRNAs could be false positive. Therefore, we estimated the false-positive rate (FPR) by calculating the proportion of these circRNAs counts to total reads mapped to BSJ sites. Additionally, the circRNAs with more than one read mapped to BSJ site (BSJ reads ≥ 2) were counted and normalized with the number of the clean reads (defined as Number of the CircRNAs Per Million clean reads (NCPM). Both FPR and NCPM values were compared for circRNAs identified from six different methods. For liver datasets, compared to the Ribo method, other methods (R, RTP, Ribo-R, Ribo-RP and Ribo-RTP) decreased FPR and increased NCPM values (). The significant difference was observed between Ribo and Rbio-RTP methods for both FPR value (0.56 ± 0.02 vs. 0.12 ± 0.02, Padj <0.05) and NCPM value (9.65 ± 0.55 vs. 64.56 ± 10.71, Padj <0.05) (). The similar results were observed for rumen tissue datasets that R, RTP, Ribo-R, Ribo-RP and Ribo-RTP methods had lower FPR and higher NCPM values than Ribo method (). Only the FPR value was significantly different between Ribo and Ribo-RP methods for rumen tissue datasets (0.48 ± 0.02 vs. 0.18 ± 0.03, Padj <0.05) ().
Figure 3. The quality and number of identified circRNA. The false-positive rate and the number of circRNA per million clean reads were calculated for liver (A) and rumen (B), respectively. The Chi-Square value and adjusted p value (Asymptotic Sig) of the Kruskal-Wallis H test are shown. The value on the left is for false-positive rate and the value on the right is for the number of circRNA per million clean reads. Different lowercase letters indicate significant differences for pairwise comparison of false-positive rate (Adjusted p < 0.05), and uppercase letters indicate significant differences for pairwise comparison of the number of circRNA per million clean reads (Adjusted p < 0.05). Ribo: rRNA depletion using Ribo-zero (Green); R: RNase R (Brown); RTP: RNase R + Tailing + Poly (A) depletion (Blue); Ribo-R: Ribo-zero + RNase R (Yellow); Ribo-RP: Ribo-zero + RNase R + Poly (A) depletion (Red); Ribo-RTP: Ribo-zero + RNase R + Tailing + Poly (A) depletion (Purple).
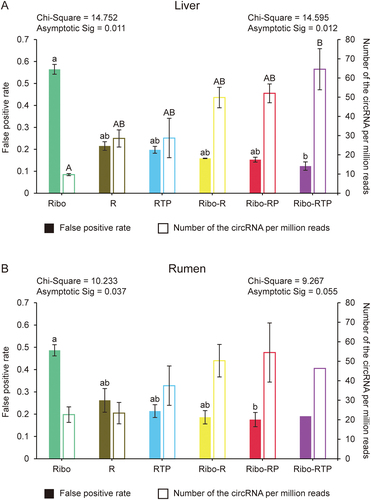
The number of circRNAs identified using different enrichment method
Furthermore, 2,285 (2,278 exonic and 7 intronic circRNAs) and 2,939 (2,923 exonic and 16 intronic circRNAs) highly confident circRNAs (BSJ reads ≥ 2, detected in at least all replicates of one method) were identified from liver and rumen datasets, respectively, and ~ 11% of these circRNAs (308 from liver datasets and 260 from rumen datasets) were commonly identified in more than five methods (). Among six methods, Ribo-RTP method identified the highest number of circRNAs (1,434 exonic and 4 intronic circRNAs from liver tissue datasets and 2,083 exonic and 16 intronic circRNAs from rumen datasets), of which 386 and 1,213 circRNAs could only be identified through Ribo-RTP method from liver and rumen datasets, respectively. Ribo-R method identified the second highest number of circRNAs (1,422 exonic and 4 intronic circRNAs from liver datasets and 1,270 exonic and 3 intronic circRNAs from rumen datasets), of which 326 and 273 circRNAs could only be identified through Ribo-R method from liver and rumen datasets, respectively (). Therefore, the highly confident circRNAs identified from these two methods were compared with other methods (Ribo, R, RTP, and Ribo-RP) with pairwise comparison. More than 80% of highly confident circRNAs identified using Ribo, R, RTP or Ribo-RP method, respectively, could also be identified with both Ribo-R and Ribo-RTP methods from liver tissue datasets (Figure S1a), and more than 75% could be identified from rumen tissue datasets (Figure S1b).
Figure 4. Identified circRNAs intersection between different methods. The upset plots of the liver (a) and rumen (b) were generated based on the circRnas with at least two reads mapped to the back-splicing junction (BSJ) position and identified in all biological replicates within the group. The longitudinal axis represents the intersection size of the circRNA number. The horizontal axis represents the input circRNA number of each group. The dark circles indicate sets that are part of the intersection. The clustered bar on the left bottom presented the total number of exonic circRnas (filled) and intronic circRnas (frame) identified by different method. Ribo: rRNA depletion using Ribo-zero (Green); R: RNase R (Brown); RTP: RNase R + Tailing + Poly (A) depletion (Blue); Ribo-R: Ribo-zero + RNase R (Yellow); Ribo-RP: Ribo-zero + RNase R + Poly (A) depletion (Red); Ribo-RTP: Ribo-zero + RNase R + Tailing + Poly (A) depletion (Purple).
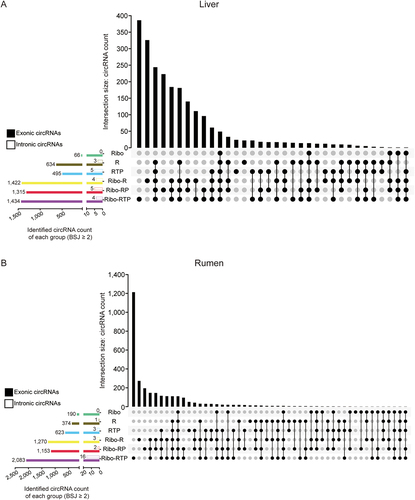
The conservation of circRNAs between cattle and human
We further explored the conservation of highly confident circRNAs identified in present study between bovine and human using the methods reported by Rybak-Wolf et al. [Citation16]. The splicing site coordinates in cattle reference genome of 4,051 cattle circRNAs (BSJ ≥2, detected in all replicates for each tissue) were used to find orthologous coordinates in the human genome (). More than 86% of bovine circRNAs could not be aligned to the human genome or did not share a splicing site with human circRNAs (). In total, 507 circRNAs were defined as conserved circRNAs between two species, most of which were completely homologous to circRNAs (372) identified in humans (). Analysis of the density of conserved circRNAs on cattle chromosomes showed that there is no apparent preference for conserved circRNAs distribution on cattle chromosomes, with highest conserved circRNAs density in chromosome 25 and lowest in chromosome X (). The methods combining rRNAs and linear RNAs removal (Ribo-R, Ribo-RP and Ribo-RTP) identified more conserved circRNAs (from 268 to 396) ().
Figure 5. CircRNA conservation between cattle and human. (a) The workflow used to identify circRNAs conserved between cattle and human. Cattle circRNAs were divided into six types (marked with roman number) and schematic diagrams of conserved circRNAs were represented (Blue: human circRNAs, Red: cattle circRNAs). (b) The proportion of different types of cattle circRNAs when compared with human circRNAs. (c) The density of conserved circRNAs on cattle chromosomes. The number of circRNAs was corrected with chromosome length (Mb).
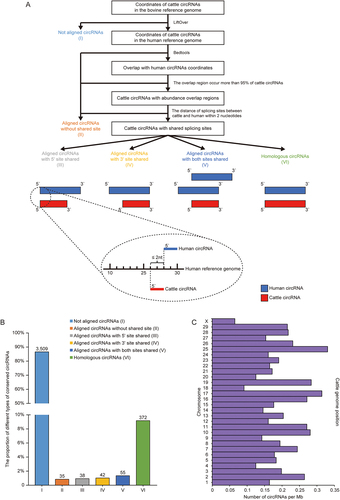
Figure 6. Circos plot of circRNAs conserved between bovine and human genome. The outermost layer is the chromosome name (Bovine: B1 - BX; Human: H1 – HX). The conserved circRNAs identified in different enrichment methods were labelled as black lines in the frame (Ribo-RTP, purple frame; Ribo-RP, red frame; Ribo-R, yellow frame; RTP, blue frame; R, brown frame; Ribo, green frame). The relative length of cattle and human chromosomes were represented by deep purple and deep brown boxes, respectively. The conserved cattle circRNAs and corresponding human circRNAs are linked using lines. Green lines represent links between non-homologous conserved circRNAs, and red lines represent homologous circRNAs.
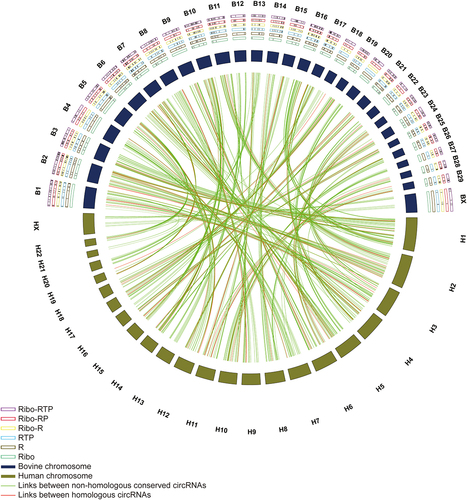
Discussion
Due to the low expression level of circRNAs, the conventional library construction methods without circRNAs enrichment could not detect them comprehensively with current RNA sequencing method. Although some enrichment approaches have been reported in previous studies, they were only used to enrich circRNAs from particular cells. For example, Panda et al. applied the same RTP method to enrich circular RNA in HeLa and C2C12 cells by eliminating RNase R resistant transcripts (like U1 snRNA) [Citation17,Citation18]. Shi et al. analysed the differences in numbers and biotypes of identified human blood circRNAs between rRNAs removal, polyadenylation followed by RNase R treatment, and the ways that combined these two methods in different orders, respectively [Citation19]. However, different from cells, tissues consist of specialized cells which can affect the sensitivity of these methods to enrich circRNAs. For instance, in mice liver, 8,843 circRNAs were identified in the hepatocyte, while only 949 circRNAs derived from the endothelial cell of hepatic sinusoid [Citation20]. Another study revealed that even the pattern of splice isoforms of circRNAs from each parental gene was cell-type specific [Citation21]. These suggest that the different characteristics of circRNAs between cell and tissue, and previous circRNAs enrichment methods from cells may not be able to enrich circRNAs from all cell types within a tissue. To date, the characterization of the comprehensive circRNA profiles from animal tissues is still limited. For instance, one of the most widely used databases CIRCpedia v2 (http://yang-laboratory.com/circpedia/) for circRNA identification consists of 180 datasets from both cell lines and tissues, but only ribosomal RNA removal procedures were performed for datasets generated from tissues with all tissues from non-ruminants [Citation22]. Therefore, our current study provides an iterative procedure to determine whether the combination of the previously reported methods is applicable to enrich circRNAs from cattle liver and rumen tissues.
Specifically, the back splicing junction structure and complex splicing patterns of circRNAs may make them more challenging to map to a reference genome accurately [Citation23]. That could be the reason why the unique mapped reads ratio in the present study was not high. Meanwhile, if rRNA depletion did not work sufficiently during library preparations, many RNA-seq reads generated from rRNA could be mapped to multiple loci and decrease the ratio of uniquely mapped reads because 80% of rRNA contains high sequence similarity [Citation24,Citation25]. In the present study, no difference in the ratio of uniquely mapped reads was observed between Ribo and R methods, indicating that in addition to degrading linear RNAs, RNase R can also degrade part highly structured RNAs with a single-stranded 3’ overhang present, including rRNAs [Citation14,Citation26].
The identification of false-positive circRNAs can result from technical artefacts produced in common RNA-seq library preparation protocols [Citation27]. For instance, two independently transcribed linear RNA molecules can be joined in an apparent non-canonical trans-splicing isoform by reverse transcriptase [Citation28]. In addition, chimeric sequences can be produced when two distinct cDNAs are ligated during the adapter ligation [Citation29]. These artefacts could generate reads that also map to the BSJ site and lead to false circRNAs identification. Moreover, circRNAs only account for up to 0.03% of total RNA molecules in eukaryote [Citation21], while rRNAs account for more than 80% [Citation30], followed by transfer RNA (tRNA) (~15%) and mRNA and other regulatory noncoding RNAs (~5%) [Citation31]. Therefore, if these non-circRNAs molecules are not removed completely, reads generate from these highly expressed transcripts can weaken the signals of circRNAs BSJ site detection even with high sequencing depth. Compared with rRNA removal method (Ribo), linear RNA removal (R, RTP) methods decreased the false-positive rate, indicating that the presence of linear transcripts has a substantial effect on the enrichment and detection of circRNAs. Ribo-R, Ribo-RP and Ribo-RTP had lower false-positive rates than R or RTP, indicating that the combination of Ribo and RNase R methods can remove both rRNAs and linear RNAs simultaneously, which is essential to increase the abundance of circRNAs and decrease the false-positive rate for the circRNA identification. Meanwhile, we further compared the NCPM value with more critical cut-off using the number of circRNAs with at least four reads mapped to BSJ site (BSJ reads ≥ 4) and normalized with the number of unique mapped reads. The NCPM dropped by 80% for Ribo, 66% for R and RTP, 60% for Ribo-R, Ribo-RP and Ribo-RTP (data not shown), suggesting the methods combining Ribo and RNase R methods (Ribo-R, Ribo-RP and Ribo-RTP) have enriched more highly expressed circRNAs than other methods (Ribo, R or RTP), which agrees with previous findings using cells [Citation32,Citation33]. These suggest that Ribo-R, Ribo-RP and Ribo-RTP also be effective methods to enrich circRNAs from tissues.
Compared with ribosome RNA removal (Ribo), linear RNAs removal (R, RTP, Ribo-R, Ribo-RP and Ribo-RTP) increased the number of identified intronic circRNAs, which has not been noted in previous studies. Although the number of intronic circRNAs was much less than exonic circRNAs [Citation34], intronic circRNAs reside in the nucleus and promote gene transcription through binding to U1 small nuclear ribonucleoprotein particle (snRNP) and RNA Polymerase II in promoter region [Citation35]. Due to linear RNAs containing many exons, removed linear RNAs enabled more reads generated from intronic circRNAs with low expression level [Citation17]. Moreover, Ribo-RTP identified a higher number of highly confident circRNAs than Ribo-R method because RNase R could not degrade highly structured 3’ ends RNAs with less than 10 nucleotides in overhang (like small nuclear RNAs) [Citation13,Citation14]. Polyadenylation enabled these remaining structured RNAs to be degraded by poly (A)+ RNA depletion and promoted further enrichment of circular RNAs [Citation18]. It is notable that the costs and labour time varied among different methods with the Ribo-R method costed $207.19 and took 13 h per sample to complete compared to the Ribo-RTP method ($227.6 and 14.25 h per sample) (Figure S2). In addition, both methods could identify more than 75% of highly confident circRNAs compared to other methods (Ribo, R, RTP and Ribo-RP). These indicate that the Ribo-R method could be ideal to identify circRNAs because it identifies sufficient circRNAs in the most timely and cost-effective manner.
Only 12% of cattle circRNAs were conserved with those identified from the human genome, which is lower than previous studies that about 30% of circRNAs were conserved between different species (chimpanzee, mouse, and pig) [Citation36,Citation37]. We speculate that such differences could be due to several reasons. Firstly, previous studies only compared monogastric animals without ruminants (cattle). Thus, the divergence between ruminants and monogastric animals contributes to the low circRNAs conservation rate between cattle and human. Secondly, the circRNAs expression is tissue specific [Citation37,Citation38]. Since rumen circRNAs have not been reported, the low conservation of cattle circRNAs with human ones could be attributed to the fact that these circRNAs were derived from different tissues, especially ruminant-specific tissue (rumen). Thirdly, no standardized circRNA annotation information is available. The human circRNAs we used were from the circAtlas database and were identified using CIRI2 [Citation10,Citation39], while the circRNAs of cattle were identified using CIRCexplorer2 in the present study [Citation4], suggesting that differences in pipeline/software algorithms also impact the identification of conserved circRNAs among different animal species. Nevertheless, more comprehensive annotation of human or mouse genome information enables us to presume the function of bovine circRNAs that are conserved across these mammals. For example, in the present study, circHIPK2 is identified as homologous circRNA between bovine and human, which can regulate astrocyte activation in human astrocytoma cell line [Citation40]. This circRNA is generated from the parental gene homeodomain interacting protein kinase 2 (HIPK2), harbouring a CTCF motif in the promoter region [Citation40]. It has been reported that CTCF underlies the evolution of chromosomal domain architecture, which could be one of the reasons why circHIPK2 is conserved between human and bovine [Citation41]. In addition, HIPK2 could attenuate septic liver injury induced by bacteria [Citation42], suggesting that circHIPK2 may regulate HIPK2 expression related to some pathological condition in cattle liver. More efforts are needed to evaluate the functional potentials of those conserved circRNAs in liver and rumen, which can help generation more knowledge or research directions to study the role of bovine circRNAs in cattle biology.
Conclusion
In summary, linear RNAs depletion can decrease the number of false-positive circRNAs and increase the number of expressed circRNAs. Combining both rRNAs and linear RNAs depletion has the best efficiency for the circRNAs enrichment and covers most of the circRNAs identified by the single method. It is noticeable that the present study focused on circRNAs identification and did not normalize the read count to compare the differences in circRNAs expression profiles between enrichment methods as it was not the primary objective. Although with small sample size, few shared circRNAs were identified between liver and rumen, indicating circRNAs were tissue specific in cattle, which need to be further investigated. Meanwhile, future studies are needed to distinguish low abundant cattle circRNAs from technical artefacts as well as to validate the expression of identified circRNAs using other methods such as qPCR and with a larger size of samples. Although some pipelines used coordinated information of the BSJ site to design divergent primers for specific circRNA amplification and quantification, it could be biased due to the multiple isoforms of circRNAs with the same BSJ site. Regardless, the information generated from this study can be helpful for researchers to choose the best one according to their experimental objective.
Materials and methods
Resource availability
Lead contact
Further information and requests for resources and reagents should be directed to and will be fulfiled by the lead contact, Le Luo Guan (http://[email protected] and [email protected]).
Data resource
Dataset accession numbers, biological sample resource information, critical commercial assays and analysis software information are listed in the Supplemental Table S3.
Any additional information required to reanalyse the data reported in this paper is available from the lead contact upon request.
Sample collection and ethics statement
The liver and rumen samples were collected from three cross-bred (Kinsella Composite) beef steers (15 months old) at Roy-Berg Kinsella research ranch at the University of Alberta, managed based on the guidelines established by the Canadian Council on Animal Care (https://ccac.ca/en/standards/guidelines/). The experimental procedures were approved by the University of Alberta Livestock Animal Care and Use Committee (Protocol No.: AUP00000882). After the animals were slaughtered, the samples were collected and immediately frozen in liquid nitrogen within 10 min and stored under −80°C before further analysis.
Method details
RNA extraction
Frozen tissue was ground to a fine powder in liquid nitrogen using a mortar and pestle. Then, total RNA was extracted using the mirVanaTM miRNA isolation Kit (Ambion, Carlsbad, CA, USA) according to the manufacturer’s instructions. After extraction, the concentration of total RNA was determined based on absorbance on a NanoDrop ND-2000 spectrophotometer (Thermo Fisher Scientific, DE, USA). A Qubit 2.0 Fluorometer (Invitrogen, Carlsbad, CA) and the Agilent 2200 TapeStation (Agilent Technologies, Inc., Santa Clara, USA) was used to assess the quality and integrity of the RNA, respectively. Only RNAs with RIN > 7 were subjected to further experiment.
Methods used to enrich circRNAs through rRNA or linear RNA depletion
The total RNA (1 μg) of each sample was subjected to circRNA enrichment using the following six methods: The ribosome RNA (rRNA) of total RNA was removed through Ribo-zero (defined as Ribo); the linear RNA of total RNA was removed through RNase R (defined as R); both linear RNA and some lariat RNA were removed through RNase R following polyadenylation and poly (A)+ RNA depletion (defined as RTP); both rRNA and linear RNA were removed through Ribo-zero and following with RNase R (defined as Ribo-R); rRNA, linear RNA and RNA with poly (A) structure were removed through Ribo-zero and following with RNase and poly (A)+ RNA depletion (defined as Ribo-RP); rRNA, linear RNA and lariat RNA were removed through Ribo-zero and following RNase R then polyadenylation and poly (A)+ RNA depletion (defined as Ribo-RTP). The procedures were described as follows.
For the methods of Ribo, Ribo-R, Ribo-RP and Ribo-RTP, the rRNA was removed firstly following the guidance of TruSeq® Stranded Total RNA Sample Preparation Guide. Briefly, the rRNA was bound with DNA probes and then removed. Subsequently, referring to the RPAD (RNase R treatment followed by Polyadenylation and poly(A)+ RNA Depletion) method generated by Poonam R et al. [Citation18], the linear RNA of Ribo-R, Ribo-RP and Ribo-RTP methods was depleted with RNase R, which was treated with 20 U (20 U/μL) RNase R (D-Mark bioscience, CA), 1 uL of RiboLock (Thermo Fisher Scientific, USA), and 1×RNase R buffer (according to the total volume of the above supernatant) (provided with RNase R), then incubated at 37°C for 30 min. For removing the lariat RNA that resisted RNase R degradation, the poly (A) tail (Polyadenylation) was added to the RNA product in the Ribo-RTP method using the Poly (A) Tailing Kit (Thermo Fisher Scientific, #AM1350) following RNase R treatment. A 40 uL reaction consisted of 20 uL of RNA sample from previous steps, 2 uL of RNase-free water, 8 uL of 5 × E-PAP buffer (Provided with Poly (A) Tailing Kit), 1 uL of E-PAP (Provided with Poly (A) Tailing Kit), 4 uL of 10 mM ATP Solution (Provided with Poly (A) Tailing Kit), 4 uL of 25 mM Mncl2 (Provided with Poly (A) Tailing Kit) and 1 uL of RiboLock (Thermo Fisher Scientific, USA). Reactions were mixed well and incubated at 37°C for 30 min. The polyadenylated RNA was further depleted in Ribo-RP and Ribo-RTP methods through Poly (A) PuristTM-MAG kit (Thermo Fisher Scientific, #AM1922). Firstly, 10 μL of well-suspended Oligo(dT) MagBeads (Provided by Poly (A) PuristTM-MAG kit) were washed twice by 50 uL of Wash Solution 1 (Provided by Poly (A) PuristTM-MAG kit), then added 40 uL of 2X Binding Solution (Provided by Poly (A) PuristTM-MAG kit) to prepared beads. The 40 μL of RNA product from the previous steps was added to the prepared MagBeads and incubated for 5 min at 72°C, followed by 20 min at 25°C with shaking. The mixture was placed on a magnetic stand for 1 min and the supernatant was collected. Without rRNA depletion, the total RNA sample was directly treated with RNase R in R and RTP methods. Like the Ribo-RTP method, polyadenylation and poly (A)+ RNA depletion were further performed in RTP method. With the exception of the Ribo enrichment method, the final circRNA enriched samples were cleaned up using miRNeasy Mini Kit (Qiagen, CA).
Library preparation and sequencing
The purified circRNAs were used to build the sequencing libraries using the guidance of TruSeq® Stranded Total RNA cDNA library construction kit, starting with the Clean Up RNA before the cDNA synthesis step. Briefly, the fragmented circRNA was used to synthesize first-strand cDNA, then second-strand cDNA, followed by 3’ adenylation and adapter ligation. After enriching DNA fragments, the libraries were validated on Agilent 2200 TapeStation using DNA1000 chip (Agilent Technologies, CA) to check the library size and purity. The cDNA libraries were sequenced at Centre d’expertise et de services Génome Québec (Québec, Canada). The five datasets downloaded from Sun et al. projects (GEO accession number GSE116775) [Citation43] were sequenced using Illumina HiSeq 4000, and other sequencings were performed using Novaseq 6000 S4 PE100 platform (Illumina).
Sequence data processing and circRNA identification
Trimmomatic (0.39) was used to remove the adapter sequence and low-quality reads (Q < 20). The clean reads were aligned to the bovine reference genome (Bos taurus ARS-UCD1.2) using STAR (2.7.9a) with the detection of chimeric (fusion) alignments (–chimSegmentMin 10). Subsequently, the aligned reads were used for circRNAs identification through CIRCexplorer2 (https://circexplorer2.readthedocs.io/en/latest/) [Citation4] with annotating pipeline. The number of reads mapped to the back splicing junction (BSJ) site of circRNA was counted, and only the circRNAs with more than one read mapped to BSJ (BSJ reads ≥ 2) were considered as expressed circRNAs.
The number of circRNAs identified in different enrichment methods
Only highly confident circRNAs (BSJ reads ≥ 2, detected in at least all replicates of one method) were maintained to explore the intersection among six methods using TBtools (https://github.com/CJ-Chen/TBtools) [Citation44] with the function of Upset Plot. Pairwise intersections between Ribo-R and other methods (Ribo, R, RTP, and Ribo-RP) were performed to see how many highly confident circRNAs identified in these methods could also be identified by Ribo-R (Coverage) or not (Non-coverage), the same comparison was also conducted for Ribo-RTP. Besides, these methods’ union of highly confident circRNAs (identified in at least one of Ribo, R, RTP, and Ribo-RP methods) were compared with Ribo-R and Ribo-RTP, respectively.
Identification of conserved circRNAs between human and cattle
The highly confident circRNAs (BSJ reads ≥ 2, detected in at least all replicates of one method) of liver and rumen were integrated and investigated the conservation by Rybak-Wolf et al.‘s method [Citation16]. Briefly, the cattle circRNAs genome position (ARS-UCD1.2) was converted into the human genome position (GRCh38.p13) using UCSC LiftOver with the chain file (bosTau9ToHg38.over.chain.gz) [Citation45]. Subsequently, the genome position of human circRNAs was downloaded from circAtlas database [Citation10] and used to search for overlap regions with cattle circRNAs on human genome position using bedtools (2.30.0) [Citation46]. Only the cattle circRNAs with overlap regions exceeding 95% will be further investigated to see if there is a shared splicing site with human circRNAs (The splicing site of cattle circRNAs detected in ±2 nucleotide interval around human circRNA sites was defined as a shared splicing site). Cattle circRNAs could be divided into six categories: I) Not aligned circRNAs (cattle circRNAs could not be mapped into human genome); II) Aligned circRNAs without shared sites (cattle circRNAs could be mapped into human genome without a shared splicing site); III) Aligned circRNAs with 5“ site shared (cattle circRNAs could be mapped into human genome with only 5” site shared with human circRNAs); IV) Aligned circRNAs with 3“ site shared (cattle circRNAs could be mapped into human genome with only 3” site shared with human circRNAs); V) Aligned circRNAs with both sites shared (cattle circRNAs could be mapped into human genome with both 5“ and 3” site shared by different human circRNAs); VI) Homologous circRNAs (cattle circRNAs could be mapped to human genome with 5’ and 3’ sites shared with same human circRNAs). The circRNAs belonging to III-VI categories were defined as conserved circRNAs between human and cattle. The number of conserved circRNAs was corrected with cattle chromosome length (Mb) to calculate the density of conserved circRNAs. The circos plot of circRNAs conserved between bovine and human genome were generated by the Advanced Circos function of TBtools [Citation44].
Statistical analyses
The ratio of uniquely mapped reads was defined as a proportion of the reads uniquely mapped to the reference bovine genome (Bos taurus ARS-UCD1.2) out of all clean reads. The false-positive rate (FPR) of circRNAs was detected using the number of circRNAs with only one read mapped to BSJ (BSJ read = 1) divided by the number of total reads mapped to BSJ. The number of expressed circRNAs (BSJ reads ≥ 2) was further normalized with sequencing depth using the number of circRNAs divided by clean reads (per million) (NCPM).
The difference in the ratio of uniquely mapped reads, false-positive rate, and the normalized number of identified circRNA with sequencing depth among methods was tested using IBM SPSS Statistics. Due to the data did not meet the assumption of homogeneity of variance (p < 0.05), statistical analyses were conducted using the non-parametric Kruskal–Wallis H Test. If the overall test showed significant differences across different methods (Asymptotic Sig < 0.05), pairwise comparisons were performed. A different letter was used to indicate significant differences between methods (Adjusted p < 0.05). The figures were generated using Excel, Graphpad Prism and TBtools, and modified using Adobe Illustrator CS6.
Author contributions
J.W. and L.L.G. conceived and designed experiments. R.J.G. and T.A.M. supplied the samples. J.W. performed experiments. Y.W., J.W., and L.L.G. implemented the computational pipelines for analysis RNA-seq data. Y.W. and J.W. analysed the RNA-seq data. Y.W and L.L.G wrote the manuscript with input from all authors.
Supplemental_Table_3_revised.pdf
Download PDF (83.8 KB)Figure_S2.tif
Download TIFF Image (750.8 KB)Supplemental_Table_2.pdf
Download PDF (3.1 MB)Figure_S1.tif
Download TIFF Image (2 MB)Supplemental_Table_1.pdf
Download PDF (14.4 MB)Disclosure statement
No potential conflict of interest was reported by the author(s).
Data availability statement
The five sequencing datasets of Ribo method were downloaded from Sun et al. projects (GEO accession number GSE116775) [Citation43]. All the sequencing datasets generated from current study have been deposited at the National Center for Biotechnology Information (NCBI) Gene Expression Omnibus (GEO) database (https://www.ncbi.nlm.nih.gov/geo/) under accession number GSE226717.
Supplementary material
Supplemental data for this article can be accessed online at https://doi.org/10.1080/15476286.2024.2356334
Additional information
Funding
References
- Misir S, Wu N, Yang BB. Specific expression and functions of circular RNAs. Cell Death Differ. 2022;29(3):481–491. doi: 10.1038/s41418-022-00948-7
- Bridges MC, Daulagala AC, Kourtidis A. Lnccation: lncRNA localization and function. J Cell Bio. 2021;220(2):220. doi: 10.1083/jcb.202009045
- Malmuthuge N, Guan LL. Noncoding RNAs: Regulatory molecules of host–microbiome crosstalk. Trends Microbiol. 2021;29(8):713–724. doi: 10.1016/j.tim.2020.12.003
- Zhang X-O, Dong R, Zhang Y, et al. Diverse alternative back-splicing and alternative splicing landscape of circular RNAs. Genome Res. 2016;26(9):1277–1287. doi: 10.1101/gr.202895.115
- Rong D, Sun H, Li Z, et al. An emerging function of circRNA-miRnas-mRNA axis in human diseases. Oncotarget. 2017;8(42):73271. doi: 10.18632/oncotarget.19154
- Kleaveland B, Shi CY, Stefano J, et al. A network of noncoding regulatory RNAs acts in the mammalian brain. Cell. 2018;174(2):350–62. e17. doi: 10.1016/j.cell.2018.05.022
- Kartha RV, Subramanian S. Competing endogenous RNAs (ceRnas): new entrants to the intricacies of gene regulation. Front Genet. 2014;5:8. doi: 10.3389/fgene.2014.00008
- Mitra A, Pfeifer K, Park K-S. Circular RNAs and competing endogenous RNA (ceRNA) networks. Transl Cancer Res. 2018;7(S5):S624. doi: 10.21037/tcr.2018.05.12
- Zheng J, Liu X, Xue Y, et al. TTBK2 circular RNA promotes glioma malignancy by regulating miR-217/HNF1β/Derlin-1 pathway. J Hematol Oncol. 2017;10(1):1–19. doi: 10.1186/s13045-017-0422-2
- Wu W, Ji P, Zhao F. CircAtlas: an integrated resource of one million highly accurate circular RNAs from 1070 vertebrate transcriptomes. Genome Biol. 2020;21(1):1–14. doi: 10.1186/s13059-020-02018-y
- Robic A, Cerutti C, Kühn C, et al. Comparative analysis of the circular transcriptome in muscle, liver, and testis in three livestock species. Front Genet. 2021;12:660. doi: 10.3389/fgene.2021.665153
- Hon C-C, Ramilowski JA, Harshbarger J, et al. An atlas of human long non-coding RNAs with accurate 5′ ends. Nature. 2017;543(7644):199–204. doi: 10.1038/nature21374
- Suzuki H, Zuo Y, Wang J, et al. Characterization of RNase R-digested cellular RNA source that consists of lariat and circular RNAs from pre-mRNA splicing. Nucleic Acids Res. 2006;34(8):e63–e. doi: 10.1093/nar/gkl151
- Xiao M-S, Wilusz JE. An improved method for circular RNA purification using RNase R that efficiently removes linear RNAs containing G-quadruplexes or structured 3′ ends. Nucleic Acids Res. 2019;47(16):8755–8769. doi: 10.1093/nar/gkz576
- Suzuki H, Tsukahara T. A view of pre-mRNA splicing from RNase R resistant RNAs. Int J Mol Sci. 2014;15(6):9331–9342. doi: 10.3390/ijms15069331
- Rybak-Wolf A, Stottmeister C, Glažar P, et al. Circular RNAs in the mammalian brain are highly abundant, conserved, and dynamically expressed. Mol Cell. 2015;58(5):870–885. doi: 10.1016/j.molcel.2015.03.027
- Panda AC, De S, Grammatikakis I, et al. High-purity circular RNA isolation method (RPAD) reveals vast collection of intronic circRnas. Nucleic Acids Res. 2017;45(12):e116–e. doi: 10.1093/nar/gkx297
- Pandey PR, Rout PK, Das A, et al. RPAD (RNase R treatment, polyadenylation, and poly (A)+ RNA depletion) method to isolate highly pure circular RNA. Methods. 2019;155:41–48. doi: 10.1016/j.ymeth.2018.10.022
- Shi H, Zhou Y, Jia E, et al. Comparative analysis of circular RNA enrichment methods. RNA Biol. 2022;19(1):55–67. doi: 10.1080/15476286.2021.2012632
- Wu W, Zhang J, Cao X, et al. Exploring the cellular landscape of circular RNAs using full-length single-cell RNA sequencing. Nat Commun. 2022;13(1):1–14. doi: 10.1038/s41467-022-30963-8
- Salzman J, Chen RE, Olsen MN, et al. Cell-type specific features of circular RNA expression. PLOS Genet. 2013;9(9):e1003777. doi: 10.1371/journal.pgen.1003777
- Dong R, Ma X-K, Li G-W, et al. CIRCpedia v2: an updated database for comprehensive circular RNA annotation and expression comparison. Int J Geno Prot. 2018;16(4):226–233. doi: 10.1016/j.gpb.2018.08.001
- Zheng Y, Ji P, Chen S, et al. Reconstruction of full-length circular RNAs enables isoform-level quantification. Genome Med. 2019;11(1):1–20. doi: 10.1186/s13073-019-0614-1
- Deschamps-Francoeur G, Simoneau J, Scott MS. Handling multi-mapped reads in RNA-seq. Computat Struct Biotechnol J. 2020;18:1569–1576. doi: 10.1016/j.csbj.2020.06.014
- Dobin A, Gingeras TR. Mapping RNA‐seq reads with STAR. Curr Protoc Bioinform. 2015;51(1):.11.4. 1–.4. 9. doi: 10.1002/0471250953.bi1114s51
- Hossain ST, Malhotra A, Deutscher MP. How RNase R degrades structured RNA: role of the helicase activity and the S1 domain. J Biol Chem. 2016;291(15):7877–7887. doi: 10.1074/jbc.M116.717991
- Szabo L, Salzman J. Detecting circular RNAs: bioinformatic and experimental challenges. Nat Rev Genet. 2016;17(11):679–692. doi: 10.1038/nrg.2016.114
- Houseley J, Tollervey D, Preiss T. Apparent non-canonical trans-splicing is generated by reverse transcriptase in vitro. PLOS ONE. 2010;5(8):e12271. doi: 10.1371/journal.pone.0012271
- Quail MA, Kozarewa I, Smith F, et al. A large genome center’s improvements to the Illumina sequencing system. Nat Methods. 2008;5(12):1005–1010. doi: 10.1038/nmeth.1270
- O’Neil D, Glowatz H, Schlumpberger M. Ribosomal RNA depletion for efficient use of RNA‐seq capacity. Curr Protoc Mol Biol. 2013;103(1):4.19. 1–4. 8. doi: 10.1002/0471142727.mb0419s103
- Westermann AJ, Gorski SA, Vogel J. Dual RNA-seq of pathogen and host. Nature Rev Microbiol. 2012;10(9):618–630. doi: 10.1038/nrmicro2852
- Ma N, Tie C, Yu B, et al. Circular RNAs regulate its parental genes transcription in the AD mouse model using two methods of library construction. Faseb J. 2020;34(8):10342–10356. doi: 10.1096/fj.201903157R
- Xin R, Gao Y, Gao Y, et al. isoCirc catalogs full-length circular RNA isoforms in human transcriptomes. Nat Commun. 2021;12(1):1–11. doi: 10.1038/s41467-020-20459-8
- Das D, Das A, Sahu M, et al. Identification and characterization of circular intronic RNAs derived from insulin gene. Int J Mol Sci. 2020;21(12):4302. doi: 10.3390/ijms21124302
- Qu S, Zhong Y, Shang R, et al. The emerging landscape of circular RNA in life processes. RNA Biol. 2017;14(8):992–999. doi: 10.1080/15476286.2016.1220473
- Sun L-F, Zhang B, Chen X-J, et al. Circular RNAs in human and vertebrate neural retinas. RNA Biol. 2019;16(6):821–829. doi: 10.1080/15476286.2019.1591034
- Santos-Rodriguez G, Voineagu I, Weatheritt RJ. Evolutionary dynamics of circular RNAs in primates. Elife. 2021;10:e69148. doi: 10.7554/eLife.69148
- Xia S, Feng J, Lei L, et al. Comprehensive characterization of tissue-specific circular RNAs in the human and mouse genomes. Brief Bioinform. 2017;18:984–992. doi: 10.1093/bib/bbw081
- Gao Y, Wang J, Zhao F. CIRI: an efficient and unbiased algorithm for de novo circular RNA identification. Genome Biol. 2015;16(1):1–16. doi: 10.1186/s13059-014-0571-3
- Huang R, Zhang Y, Han B, et al. Circular RNA HIPK2 regulates astrocyte activation via cooperation of autophagy and ER stress by targeting MIR124–2HG. Autophagy. 2017;13(10):1722–1741. doi: 10.1080/15548627.2017.1356975
- Vietrirudan M, Barrington C, Henderson S, et al. Comparative hi-C reveals that CTCF underlies evolution of chromosomal domain architecture. Cell Rep. 2015;10(8):1297–1309. doi: 10.1016/j.celrep.2015.02.004
- Jiang Z, Bo L, Meng Y, et al. Overexpression of homeodomain-interacting protein kinase 2 (HIPK2) attenuates sepsis-mediated liver injury by restoring autophagy. Cell Death Dis. 2018;10(1):1–16. doi: 10.1038/s41419-018-1236-z
- Sun H-Z, Zhu Z, Zhou M, et al. Gene co-expression and alternative splicing analysis of key metabolic tissues to unravel the regulatory signatures of fatty acid composition in cattle. RNA Biol. 2021;18(6):854–862. doi: 10.1080/15476286.2020.1824060
- Chen C, Chen H, Zhang Y, et al. TBtools: an integrative toolkit developed for interactive analyses of big biological data. Mol Plant. 2020;13(8):1194–1202. doi: 10.1016/j.molp.2020.06.009
- Lee BT, Barber GP, Benet-Pagès A, et al. The UCSC genome browser database: 2022 update. Nucleic Acids Res. 2022;50(D1):D1115–D22. doi: 10.1093/nar/gkab959
- Quinlan AR, Hall IM. BEDTools: a flexible suite of utilities for comparing genomic features. Bioinformatics. 2010;26(6):841–842. doi: 10.1093/bioinformatics/btq033