Abstract
Lake Okeechobee is a large shallow lake with over 44% (as of 2006) being underlain with phosphorus-enriched mud sediments. Wind-induced sediment re-suspension may play a significant role in the nutrient cycling of this lake. It is critical to develop optimal models with low uncertainty to map the distribution and changes of total phosphorus (TP) over time. Geographically Weighted Regression (GWR) is a powerful spatial regression method that examines the details of relationship between the target variable and independent variables and their changes over space. The calibrated GWR models were applied to data sets of organic mud sediment from Lake Okeechobee collected in 1988, 1998 and 2006. Three sets of ancillary data were also used in the models: total iron (Fe), which was strongly and positively correlated to TP (0.69–0.72); mud thickness, which was moderately and positively correlated to TP (0.57–0.72); and site elevation, which was weakly but negatively correlated to TP (−0.46- −0.55). The GWR models were compared with Ordinary Kriging (OK), Ordinary Least-squares Regression (OLS) and Regression-Kriging (RK) to determine their accuracy. GWR models use both spatial auto-correlation and correlation between TP and independent variables to improve the model performance. The GWR models were superior to OK and RK models previously applied to these data sets. The GWR (total Fe) and GWR (mud thickness and site elevation) models were the most accurate based on lower root mean square errors (RMSE). GWR (Fe) and GWR (Thickness and Elevation) models were used to map TP concentrations and TP mass. Using TP concentrations and mud volumes, TP mass in the lake sediments was estimated for each sample set. The TP mass increased about 38–44% from 1988 to 1998 and decreased about 30–34% from 1998 to 2006. TP mass was similar in 1988 and 2006.
Introduction
Lake Okeechobee is a large shallow lake with over 44% of the lakebed containing phosphorus-enriched mud sediments (Yan and James Citation2007). Because of the strong link between water quality and resuspended sediments (James and Zhang Citation2008), the distribution of these sediments, their compositions and changes over time, sediment re-suspension and interaction with water quality are of direct concern for lake management and restoration efforts to improve the lake’s environmental quality. In 1988, a comprehensive survey reported that the upper 10 cm of mud sediments within the lake contained an estimated 28,600 metric tons of P (Reddy, Sheng, and Jones Citation1995). A second survey in 1998 produced similar estimates (Fisher, Reddy, and James Citation2001). Both studies indicated a large variation (coefficient of variation greater than 28%) in these estimates.
In order to improve the estimates of P content and distribution of P in the sediments, generic and robust spatial modelling techniques can be used. Kriging and its variants have been widely recognized as primary spatial interpolation techniques from the 1950s. In the 1990s, with the emergence of geographical information science (GIS) and remote-sensing technologies, exhaustively mapped ancillary variables (e.g. digital elevation terrain attributes), images and other derivatives (such as land-use data) are used to estimate soil parameters directly with linear regression models (Gessler et al. Citation1995; Moore et al. Citation1993). This approach is termed environmental correlation (McKenzie and Ryan Citation1999) since quantitative environmental variables were used in the regression. In the last decade, a number of ‘hybrid’ interpolation techniques, which combine kriging and ancillary variables, have been developed and tested. Kriging combined with regression (McBratney et al. Citation2000) is considered as one of the most popular methods with few estimated model parameters (Knotters, Brusm, and Oude Voshaar Citation1995; Hengl Citation2009). Most recently, an optimized regional regression (ORR) model was proposed to estimate house value and it produced the most accurate predictions compared to four other regression methods (Lu et al. Citation2013).
Pebesma (Citation2004) selected models for spatial prediction based on three questions: (1) is a physical model defined; (2) do sampled variables correlate with environmental variables and (3) do residuals show spatial autocorrelation? Four potential models can be chosen depending on how the questions are answered for the area of interest (). If a deterministic model is not defined, and correlations exist between sampled variables and environmental factors, then a statistically significant regression model is defined. The regression residuals are then examined for spatial autocorrelation. If no spatial autocorrelation is identified with the residuals, the regression coefficients can be estimated using ordinary least squares (OLS). Otherwise, mixed models like regression-kriging (RK) and Geographically Weighted Regression (GWR) can be applied. If the data are not correlated to environmental factors but do exhibit spatial autocorrelation, the variogram of the target variable (describing the degree of spatial dependence of the variable) can be analysed and OK and its variants can be used for estimation. Otherwise, a global mean is the best estimate for the area of interest.
Figure 1. Model selection processes for spatial prediction (OLS: ordinary least square, OK: ordinary kriging; RK: regression-kriging and GWR: geographically weighted regression).
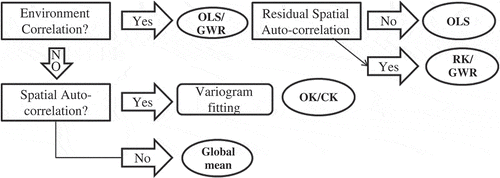
The objective of this study is to develop optimal spatial models for accurate estimates of total phosphorus (TP) concentrations and mass in Lake Okeechobee sediments over time (1988–2006). Spatial changes also are examined to identify the potential effects of extreme environmental forcing during 2004 and 2005 hurricane seasons. Several unique GWR models were calibrated and validated to describe the sediment characteristics, and then the best models were used to calculate TP and the changes in TP from 1988 to 2006.
Materials and methodology
Study area and data
Lake Okeechobee is a large shallow lake with an area of approximately 1730 km2 and an average depth of 2.7 m (). It is the largest freshwater lake in the southern United States. It is located in the southern peninsula of Florida with a subtropical climate. Average rainfall is 1.12 m (SFWMD Citation2014), evaporation is in excess of 1.3 m (Abtew Citation2001) and water temperatures range from 10°C on rare occasions in winter to 32°C in summer. The lake experiences major storm events once or twice every decade (James and Pollman Citation2011).
Lake Okeechobee is turbid and has experienced decades of excessive phosphorus loading, primarily from agricultural runoff. This excessive P load has accumulated primarily in mud sediments that comprise 44% of the lakebed (James and Pollman Citation2011). While three major regions of the lake are easily defined – the pelagic, nearshore and littoral () – there are distinct regions comprising different types of sediments. In addition to the mud sediments, three other sediment types have been found: (1) peat, primarily on the south rim of the lake; (2) sand, in the nearshore region on the west side of the lake and (3) marl/rock, along an outcrop just north of the peat region (Fisher, Reddy, and James Citation2001).
Sediment cores were obtained from Lake Okeechobee in 1988, 1998 (Fisher, Reddy, and James Citation2001) and 2006 (Balance Environmental Management (BEM) Systems and University of Florida Citation2007; Yan and James Citation2012) at 170 sampling points (). The thickness of mud sediments at the top of each core was measured. Fisher, Reddy, and James (Citation2001) describe methods used to extract and analyse total nitrogen (TN), TP, iron (Fe), calcium (Ca) and carbon (TC) from the top 10 cm of each core (). These values along with their coordinate locations were used to create a number of geospatial models as described below.
Table 1. Summary table of nutrients (mg/g), mud thickness (cm) and elevation (ft).
In addition, the lake bottom elevation was surveyed during July–September 2008. The 681,796 survey data points were integrated with a LiDAR (Light Detection and Ranging) survey data collected during 2007–2009 for the lakeshore areas. These data sets were combined to develop an accurate digital elevation model (500 feet by 500 feet) for the entire area of Lake Okeechobee (). Elevation values for the 170 sample locations were extracted from the integrated elevation raster to be used in geospatial models.
GWR models
There are a number of assumptions associated with spatial statistical prediction models. Variables of interest must be stationary, which means that the statistical properties of the variables (e.g. mean, standard deviation and covariance) are the same over space and time for the area of interest. However, spatial data exhibit two properties: spatial dependence and spatial heterogeneity, and this makes it difficult to meet the assumptions and requirements of traditional (non-spatial) statistical methods. For example, geographical properties vary from place to place. A local spatial model can estimate temperature values at un-sampled locations, better than a global model, based on the values at nearby locations and known values of elevation (or other ancillary variables).
There are different strategies to deal with spatial autocorrelation in regression model residuals, such as re-sampling until the input variables no longer exhibit statistically significant spatial autocorrelation, or isolating the spatial and non-spatial components of each input variable using a spatial filtering regression method. For the traditional non-spatial OLS models, regional variation (non-stationarity) can be eliminated by defining/reducing the size of the study area so that the processes within it are all stationary. A better approach is to use methods that incorporate regional variation into the regression model such as GWR (Brunsdon, Fotheringham, and Charlton Citation2010; Fotheringham, Brunsdon, and Charlton Citation2002).
GWR is a spatial regression method developed by Brunsdon, Fotheringham, and Charlton (Citation2010), Fotheringham, Brunsdon, and Charlton (Citation2002), building on the works of Hastie and Tibshirani (Citation1990) and Loader (Citation1999). It can calibrate a multiple regression model that allows different relationships between the dependent and independent variables to exist at different locations in space (non-stationarity). GWR is a local spatial regression approach based on the ‘First Law of Geography’: everything is related to everything else, but closer things are more related to each other (Tobler Citation1970). As a local analysis technique, GWR tends to serve as a ‘microscope’ that amplifies details of the data that are otherwise hidden. GWR provides a local model of the predicted variable or process by fitting a regression equation to every feature in the data set. When used properly, these methods are powerful and reliable statistics for examining linear relationships. With these characteristics, GWR is recommended as an additional spatial interpolation technique that may increase interpolation accuracy. GWR assumes that relationships between the target variable and ancillary variables are not constant over space, and the error terms are independent and identically distributed (i.i.d.) across space. Therefore, the coefficients (βks) vary from location to location; the interpolation can then be expressed as
where yi is the predicted value at the , β0 is the model intercept, βk is the coefficient of the kth explanatory variable, n is the the number of available ancillary variables, xk = kth is the explanatory variable, k = 1, …, n, εi is the error associated with the ith observation, (ui vi) are the coordinates of the ith observation in space and
is a realization of the function
at point i.
It is assumed that each prediction is generated through a Gaussian or Gaussian-like spatial process in which the strength of the correlation among observations declines as distance increases. With such an assumption, it is possible to mimic the spatial process at any particular location in space, and generate a set of local predictions that are based on weighting of available observations according to the distance and the prediction location. The raster surfaces of model coefficient values created by GWR can be examined to evaluate regional variation in the model explanatory variables. The spatially consistent (stationary) relationships between the dependent variable and each explanatory variable and how they change across the study area can be evaluated as well as the coefficient distribution that shows where and how much variation is present.
GWR also can be used for prediction when it is applied to sampled data. First, a data set is specified with all of the explanatory variables for locations where the dependent variable is unknown. GWR then calibrates the regression equation using known dependent variable values from the input feature class, and creates a new output feature class with dependent variable estimates.
Weighting functions and bandwidth selection
The parameters estimated for a GWR model are dependent on the spatial weighting function and the bandwidth selected. The weighting function determines the importance of a local observation based on its proximity to the sample point (). The bandwidth determines which nearby observations are considered when calibrating coefficients for a sample point. Bandwidths may be constant (fixed kernel) or variable (adaptive kernel) that vary by the location of a data point.
Figure 3. GWR weighting schemes and bandwidths. (a) Fixed weighting scheme. (b) Adaptive weighting schemes.
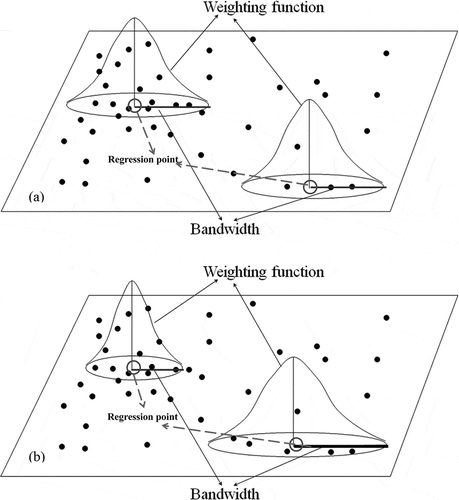
The weighted function for parameter estimation was developed by Fotheringham, Brunsdon, and Charlton (Citation2002):
where the bold symbols are matrices, is an estimate of β and
is an n by n matrix whose off-diagonal elements are zero and whose diagonal elements denote the geographic weighting of each of the n observed data for regression point i.
The weights are chosen such that those observations near the point in space where the parameter estimates are derived have more influence on the result than observations further away. Two schemes are used for the weight calculation: bi-square and Gaussian. In the case of the Gaussian scheme, the weight for the ith observation wij is
In the bi-square function
With the fixed kernel-weighting scheme, the same shape and magnitude of the selected weighting functions are applied to every sample point over the space (). Fixed schemes might produce estimates with large variances where data are sparse, while masking subtle local variations where data are dense. Adaptive schemes adjust according to the density of data: shorter bandwidths are selected where data are dense and longer bandwidths selected where data are sparse. Adaptive kernels are often used when data are not evenly distributed ().
An optimal bandwidth (or nearest neighbours) can be selected by satisfying either least cross-validation (CV) score (the difference between the observed value and the GWR calibrated value), or least Akaike Information Criterion (AIC). Four GWR sub-models – Adaptive AICc, Adaptive CV, Fixed AICc and Fixed CV – are the different combinations of weighting functions and optimization criteria. In GWR, a corrected AIC (AICc) was used (Hurvich, Simonoff, and Tsai Citation1998), and it was first suggested for normal linear regression by Sugiura (Citation1978). Hurvich, Simonoff, and Tsai (Citation1998) demonstrated the small-sample superiority of AICc over AIC, and justified the use of AICc in the frameworks of non-linear regression models and autoregressive models. The AICc can be calculated using the following equation:
where n is the number of observations in the data set, is the estimate of the standard deviation of the residuals, S is the hat matrix and tr(S) is the trace of the hat matrix.
Cross-validation minimization can be used to search for an optimal bandwidth:
Model development
OLS models of observed data were first developed to find significant relationships between TP and other ancillary data. After one or more candidate regression models are identified using the ArcGIS 9.3.1 OLS statistical regression tool, the GWR model is applied for the selected regression models. The ArcGIS GWR statistical tool creates coefficient raster surfaces for the model intercept and each explanatory variable.
Corrected AICc was the measure chosen to determine goodness-of-fit. The AICc compares models of the same target variable and it contains a penalty for the complexity (degrees of freedom) of the model. The AICc provides a measure of the information distance between the fitted model and the unknown ‘true’ model. This distance is not an absolute measure but a relative measure known as the Kullback–Leibler information distance. AICc can compare the global OLS model with a local GWR model. Other model performance indicators were also calculated: the sum of the squared residuals (SSR), sigma (the estimated standard deviation for the residuals) and R2.
Training and validation data sets were created with the Geostatistical Analyst extension of ArcGIS 9.3.1 by randomly selecting the 170 samples into a 75–25% split. ArcGIS OLS tool and Stepwise regression tools of R software were used to select the ‘best’ subset of predictors. Redundant (collinear) variables were removed using the variance inflation factor (VIF < 7.5). Two measures of model accuracy and precision were determined for validation points:
Mean prediction error (ME):
Root mean square prediction error (RMSE):
where l is the number of validation points, and
are estimated and measured values at validation points
, E is the expected value of the variable and
is the variance.
In order to compare accuracy of prediction between variables of different types, the RMSE was normalized by the total variation. This is called the normalized root mean squared deviation or error (NRMSD or NRMSE):
where Xmax and Xmin are the maximum and minimum predicted values in the validation data points, respectively. Equation (9) determines the amount of global variation explained by the model. A value of NRMSE that is close to 40% means a fairly satisfactory accuracy of prediction (R-square = 85%). If NRMSE > 71%, then the model accounts for less than 50% of variability at the validation points (Hengl Citation2009). The validation results were calculated through a series of GIS processes using the ModelBuilder tool.
We also developed a histogram of errors at validation points and compared the errors estimated by the model and the true mapping error at validation points. This can help us detect outlier points where the errors are extreme compared to other locations. Scatter plots of predicted versus the measured values at validation points are used to derive a correlation coefficient to test the model’s ability. For comparison, OK, OLS and RK models were also examined and their errors were calculated.
In this study, the best GWR models were selected based on AICc, SSR (or sigma) and the adjusted R2. If at least two of the SSR, AIC and adjusted R2 values for Model A were better than those for Model B, while the remaining one (if any) was similar to that of Model B, then Model A was considered better. The model output feature class table includes fields for observed and predicted y-values, condition number (cond), Local R2, residuals, explanatory variable coefficients and standard errors and can be examined for local errors and local collinearity.
Results
Mud thickness varied temporally and spatially. The maximum mud thickness for 1988, 1998 and 2006 were 66, 74 and 51 cm, respectively. The mean thickness declined slightly from 12.47 cm in 1988 to 11.17 cm in 1998, then dropped to 8.27 cm in 2006 (). The values of TP in sediments formed a bimodal distribution for each year sampled (). TP varies in different zones, with the highest values in mud zones (>850 mg/kg), lower values in peat zones (250–450 mg/kg) and the lowest in sand and rocks zones (0–300 mg/kg) (Yan Citation2011). The 2006 TP values ranged from 17 to 999 mg/kg, with mean and median values of 566 and 397 mg/kg, respectively. The 1998 TP values ranged from near zero to over 1793 mg/kg, with mean and median values of 650 and 475 mg/kg, respectively. The 1988 TP ranged from 39 to 1708 mg/kg, with mean and median values of 670 and 664 mg/kg, respectively (). The TP concentrations of the three data sets show a second-order trend change along the north–south direction and relatively weak trend (first-order trend) in the east–west direction.
Correlations (r) of the measured values show that TP is strongly and positively correlated with Fe (0.79–0.94), moderately and positively correlated with mud thickness (0.57–0.72), and weakly and negatively correlated with site elevation (−0.47 to −0.55) in all three data sets. Other parameters were not significantly correlated to TP ().
Table 2. Correlation coefficients and their significances of sediment nutrients (mg/kg), mud thickness (cm) and elevation (ft).
Model selection and calibration
Two statistically significant OLS models with different independent variables were selected for TP concentration: total Fe alone, and mud thickness (Th) & elevation (Elev) together, respectively (). Each parameter was significantly related to TP independent of the others in the OLS models. The Global Moran’s I Index was used to calculate the spatial autocorrelation of the residuals.
Table 3. Statistically significant calibrated OLS models for Iron (Fe) and mud thickness and lakebed elevation (Th & Elev).
For all three data sets total Fe as a predictor alone explained over 70% of the model variation while mud thickness explained over 45% of the variation (). The model residuals were either clustered (1988 and 2006 data sets) or randomly distributed (1998) as shown by the Jarque–Bera (JB) statistic. Koenker’s Breusch–Pagan (BP) value K is not significant, indicating the correlation between TP and total Fe is stationary in the study area. Correlation analysis shows that both mud thickness and elevation are statistically independent. The K (BP) statistic is not significant, indicating that the relationship between TP and thickness and elevation are also stationary in the study area.
GWR (TP vs. mud thickness and elevation) model
Four GWR sub-models (Adaptive AICc, Adaptive CV, Fixed AICc and Fixed CV) were developed using mud thickness and elevation as independent predictors. For all data sets the Fixed CV model had the best overall results (). For the 2006 data set, the Fixed CV model has the lowest errors (SSR, Sigma), better model performance (lower AICc value) and the model explained much of the variability (higher R2). This sub-model was further evaluated in the validation process. Its residuals were dispersed based on the Moran Index value (Yan Citation2011). For the 1998 TP data, all the GWR sub-models performed similarly with small differences and weakly dispersed residuals.
Table 4. Analyses of the GWR calibration model (TP vs. Th & Elev).
The model coefficient maps show very strong spatial gradients of the relationships to TP concentrations (). In the 2006 data, mud thickness was positively correlated with TP, with a decreasing correlation from the eastern open water region of the lake to the western littoral region of the lake (); site elevation was negatively correlated to TP in the central lake zone and positively correlated in most other regions of the lake. The 1988 and 1998 models had similar relationships ().
GWR (TP vs. total Fe) model
For 1988 and 2006 data, all GWR sub-models performed better than the corresponding OLS model based on AICc and R2. Among the GWR sub-models, CV models (Adaptive CV and Fixed CV) were better than the AICc models. The two CV models were very close in both AICc and R2 values, but the Fixed CV has much lower SSR. The Fixed CV model was considered a better model for TP estimation (). For 1998 data, the Fixed AICc model performed the best. Total Fe was positively correlated with TP in all three data sets and the coefficients increased from east to west ().
Table 5. Analyses of the GWR calibration model (TP vs. Fe).
GWR model validation and error analysis
Using elevation and mud thickness as independent variables, TP values were estimated on a 500 ft by 500 ft grid. The predicted values at the validation sites were compared to observed data. The residual errors for the 2006 validation data were normally distributed within a narrow region in the quantile-comparison plots (Yan Citation2011). Both residual errors of 1998 and 1988 were skewed with some large positive errors (underestimates) in the 1988 data. All scatter plots demonstrated moderate fits for the measured and estimated values with R2 ranges between 0.67 and 0.69 ().
Figure 7. Scatter plots of the GWR models (TP vs. Th & Elev) validation set for 1988, 1998 and 2006.
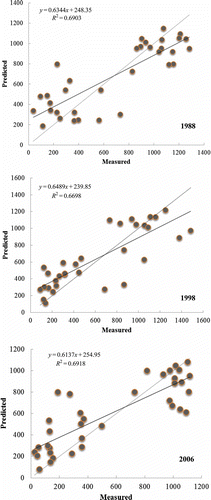
The coefficient values and intercepts change over the study area for Fe (), and both values were used to calculate TP. All the residual errors were skewed distributions with higher positive errors (underestimates) for 2006 and higher negative errors (overestimates) for both 1988 and 1998 data sets (Yan Citation2011). Modelled versus predicted comparisons of the 2006 validation data were very good, with R2 of 0.88 (). With several extreme values removed from the 1988 and 1998 validation data, the comparisons of the modelled versus predicted were also good as well with R2 of 0.87 and 0.86, respectively.
Model comparison and TP mass calculations using optimal models
In addition to GWR models, errors of OK, OLS and RK models were determined (). For all models evaluated, the GWR models (TP vs. Fe and TP vs. Th & Elev) had lower RMSE and the lowest ME for TP 2006 and TP 1988 data sets (). For 1998 TP data, OK has the lowest mean errors but higher RMSE than the OLS and GWR models.
Table 6. Comparison of TP model errors.
Based on model validation errors, the GWR (Fe) and GWR (Th and Elev) models were used for TP concentration prediction and mass calculation. For the GWR (TP vs. Fe) model, total Fe was estimated using OK for the whole lake bottom first. TP concentrations (a, c and e of ) and mass (b, d and f of ) were calculated using ArcGIS tools. For the GWR (Th and Elev) model, the mud thickness raster and the lake bottom elevation data raster were used to calculate the TP concentrations (a, c and e of ) and mass (b, d and f of ).
Figure 9. TP concentration (a, c and e) (mg/kg) and weights (b, d and f) (kg) estimated using the GWR model (TP vs. Fe) for 1988, 1998 and 2006.
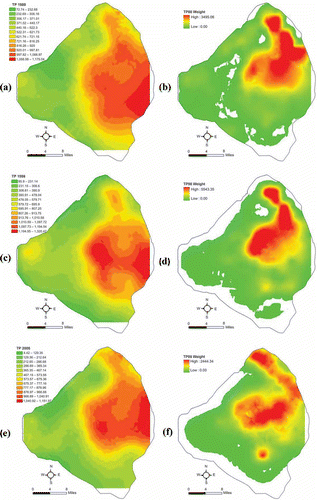
Figure 10. TP concentration (a, c and e) (mg/kg) and weights (b, d and f) (kg) using the GWR model (TP vs. Th & Elev) for 1988, 1998 and 2006.
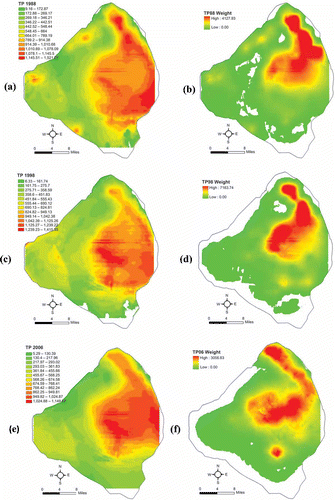
The results showed similar changes for TP mass: increase from 1988 to 1998 by 38–44%; decrease from 1998 to 2006 by 30–34%. From 1988 to 2006 (28-year period), there were small declines in TP mass: 1.0E + 06 kg or 2% reduction for OK and GWR (Fe) models, and 4.2E + 06 kg or 10% reduction for the GWR (Th and Elev) model ().
Table 7. TP mass (kg) and changes over time.
Conclusions
GWR models provided the best predictions of TP in sediments for Lake Okeechobee because of the strong positive relationships between TP and total Fe, and moderate positive relationships between TP and mud thickness and site elevation. The small search windows used on local samples for estimation were an advantage of GWR models over global OLS models. OK models used only the weak spatial autocorrelation among TP data and led to the highest RMSE; their smaller mean errors are mainly due to smaller search windows used in variogram fitting. Regression-Kriging models use both weak spatial autocorrelation among TP values, weak correlation between TP and mud thickness and site elevation, and they performed better than OK models. These calibrated models may add new approaches to analyse other lakes or water bodies that are faced with similar challenges such as Lake George, Uganda and Lake Tai-Hou, China (Havens et al. Citation2007).
Mud sediments in Lake Okeechobee contain high amounts of P (Reddy, Sheng, and Jones Citation1995). Much of this mud has accumulated since the 1940s (Brezonik and Engstrom Citation1998). Brezonik and Engstrom (Citation1998) found that these sediments consist of approximately equal amounts of organic, nonapatite inorganic-P and apatite inorganic-P. Thus, correlations of P to total organic carbon and calcium (found in apatite inorganic-P) in these mud sediments show the value of these ancillary measurements for developing robust models. Using the GWR technique, both organic carbon and calcium could be used to develop P predictions in Lake Okeechobee sediments. However, thickness of mud sediments, which contains an easily measured surrogate for organic content, and bottom elevation, which indicates where sediments will accumulate more readily (Jin, Ji, and Hamrick Citation2002), produce more accurate models than Ca and TOC.
Discussion
Sediments accumulate in the central deep regions of Lake Okeechobee (Brezonik and Engstrom Citation1998). This is due primarily to the wind-driven gyres that are set up on the lake and tend to deposit sediments in the deeper central areas of the Lake (Jin, Ji, and Hamrick Citation2002). Over time this material has collected in these regions, and has been mostly undisturbed as demonstrated from core dating using Pb210 (Brezonik and Engstrom Citation1998) and the observance of sub-millimetre laminations in the mud sediments that disappeared only within the top few centimetres (Kirby, Hobbs, and Mehta 1994). The dramatic changes in the thickness and elevation coefficients for the GWR model () between the 1998 and 2006 period are indicative of the three major hurricanes – Frances, Jeanne and Wilma – that passed close to the Lake on 5 September 2004, 26 September 2004 and 24 October 2005, respectively. The hurricanes re-suspended a large amount of sediment and redistributed it throughout the lake (Jin et al. Citation2011).
The strong positive relationship between TP and Fe is related to the binding of inorganic phosphorus to oxidized iron in the upper few centimetres of sediment (Moore et al. Citation1993). Moore et al. (Citation1993) suggested that the abundance of iron in the Lake Okeechobee system produces conditions that control P exchange by precipitation and absorption in the upper oxic layer of sediment rather than diffusion from the anoxic sediments below. These authors demonstrated the process by incubating sediment cores under alternating oxic/anoxic water column conditions. They found higher fluxes of P under anoxic conditions, suggesting that oxidized Fe was controlling the rate of this flux. This indicates that more soluble P could be sequestered under sediment layers with higher Fe. Because Fe is observed in regions with and without mud, it is a much better predictor than mud thickness. Fe also is better than elevation in predicting P because it is mobile and it affects the geochemistry of P.
Moore and Reddy (Citation1994) used a similar framework to investigate the role of pH on P exchange from Lake Okeechobee sediments. They found that very acidic conditions (pH 5.5) resulted in SRP release. However, Lake Okeechobee pH is somewhat basic (approximately 8.2 – James, Smith, and Jones Citation1995) and the sediment pH is rather stable (7.0–7.5 – Moore and Reddy Citation1994). Thus, pH was not a useful parameter in these GWR models.
Between 1988 and 1998 the lake received an estimated net load of phosphorus of 2584 metric tons (Havens and James Citation2005); from 1999 to 2006 the estimated net load was 1949 metric tons (James and Zhang Citation2008). The changes predicted by the models are much greater and variable than these numbers derived from nutrient budgets. The tremendous variability among the sediment-sampled cores is also confounded by the small number of samples per area (less than 1 per square km). The large amount of error could account for the differences between the net loads to the lake and net change observed in the models. By sampling more intensively in areas where large changes in TP and ancillary data occur, model accuracy could be improved and thus improve estimates of change.
Sediment–water interactions are important to the health of Lake Okeechobee. Re-suspension of the mud sediments, especially during high lake water levels, can lead to reduced submerged aquatic vegetation, which negatively affects fish recruitment (Havens et al. Citation2005). Diffusive phosphorus fluxes, primarily from the mud sediments, produce an internal load that is equivalent to external loads (Fisher, Reddy, and James Citation2005). This can lead to delays of in-lake phosphorus in response to eternal loads (James and Pollman Citation2011). Given a program that measures sediment content of TP and ancillary data, accurate GIS models can be developed to evaluate spatial variability on these lakes. Such information could be used to manage these lakes through targeted chemical or dredging techniques (Søndergaard, Jensen, and Jeppesen Citation2003).
Acknowledgements
This research was supported by South Florida Water Management District. The authors wish to thank Susan Gray, Jun Han, David Unsell, Ming Chen, Kim O’Dell and Jenifer Barnes for their comments and suggestions to the previous version of this manuscript. The authors also wish to thank the three anonymous reviewers for their valuable comments and recommendations, which enhanced the manuscript significantly.
References
- Abtew, W. 2001. “Evaporation Estimation for Lake Okeechobee in South Florida.” Journal of Irrigation and Drainage Engineering 127: 140–147. doi:10.1061/(ASCE)0733-9437(2001)127:3(140).
- BEM System, University of Florida. 2007. Lake Okeechobee Sediment Quality Final Report. West Palm Beach: South Florida Water Management District.
- Brezonik, P. L., and D. R. Engstrom. 1998. “Modern and Historic Accumulation Rates of Phosphorus in Lake Okeechobee, Florida.” Journal of Paleolimnology 20: 31–46. doi:10.1023/A:1007939714301.
- Brunsdon, C., A. S. Fotheringham, and M. E. Charlton. 2010. “Geographically Weighted Regression: A Method for Exploring Spatial Nonstationarity.” Geographical Analysis 28 (4): 281–298. doi:10.1111/j.1538-4632.1996.tb00936.x.
- Fisher, M. M., K. R. Reddy, and R. T. James. 2001. “Long-Term Changes in the Sediment Chemistry of a Large Shallow Subtropical Lake.” Lake and Reservoir Management 17: 217–232. doi:10.1080/07438140109354132.
- Fisher, M. M., K. R. Reddy, and R. T. James. 2005. “Internal Nutrient Loads from Sediments in a Shallow, Subtropical Lake.” Lake and Reservoir Management 21: 338–349. doi:10.1080/07438140509354439.
- Fotheringham, A. S., C. Brunsdon, and M. E. Charlton. 2002. Geographically Weighted Regression: The Analysis of Spatially Varying Relationships. Chichester: Wiley.
- Gessler, P. E., I. D. Moore, N. J. McKenzie, and P. J. Ryan. 1995. “Soil-Landscape Modelling and Spatial Prediction of Soil Attributes.” International Journal of Geographical Information Systems 9 (4): 421–432. doi:10.1080/02693799508902047.
- Hastie, T. J., and R. J. Tibshirani. 1990. Generalized Additive Models. London: Chapman and Hall.
- Havens, K. E., D. Fox, S. Gornak, and C. Hanlon. 2005. “Aquatic Vegetation and Largemouth Bass Population Responses to Water-Level Variations in Lake Okeechobee, Florida (USA).” Hydrobiologia 539: 225–237. doi:10.1007/s10750-004-4876-1.
- Havens, K. E., and R. T. James. 2005. “The Phosphorus Mass Balance of Lake Okeechobee, Florida: Implications for Eutrophication Management.” Lake and Reservoir Management 21: 139–148. doi:10.1080/07438140509354423.
- Havens, K. E., K.-R. Jin, N. Iricanin, and R. T. James. 2007. “Phosphorus Dynamics at Multiple Time Scales in the Pelagic Zone of a Large Shallow Lake in Florida, USA.” Hydrobiologia 581: 25–42. doi:10.1007/s10750-006-0502-8.
- Hengl, T. 2009. A Practical Guide to Geostatistical Mapping. 2nd ed. Amsterdam: University of Amsterdam.
- Hurvich, C. M., J. S. Simonoff, and C. L. Tsai. 1998. “Smoothing Parameter Selection in Nonparametric Regression Using an Improved Akaike Information Criterion.” Journal of the Royal Statistical Society: Series B (Statistical Methodology) 60: 271–293. doi:10.1111/1467-9868.00125.
- James, R. T., and C. D. Pollman. 2011. “Sediment and Nutrient Management Solutions to Improve the Water Quality of Lake Okeechobee.” Lake and Reservoir Management 27 (1): 28–40. doi:10.1080/07438141.2010.536618.
- James, R. T., V. H. Smith, and B. L. Jones. 1995. “Historical Trends in the Lake Okeechobee Ecosystem III. Water Quality.” Archives Hydrobiol Supplement 107: 49–69.
- James, R. T., and J. Zhang. 2008. “Lake Okeechobee Protection Program – State of the Lake and Watershed.” In South Florida Environmental Report, Chapter 10, edited by G. W. Redfield and S. Efron, 10-11–10-102. West Palm Beach: South Florida Water Management District.
- Jin, K. R., N. B. Chang, Z. G. Ji, and R. T. James. 2011. “Hurricanes Affect the Sediment and Environment in Lake Okeechobee.” Critical Reviews in Environmental Science and Technology 41 (sup1): 382–394. doi:10.1080/10643389.2010.531222.
- Jin, K. R., Z. G. Ji, and J. H. Hamrick. 2002. “Modeling Winter Circulation in Lake Okeechobee, Florida.” Journal of Waterway, Port, Coastal, and Ocean Engineering 128: 114–125. doi:10.1061/(ASCE)0733-950X(2002)128:3(114).
- Kirby, R., C. H. Hobbs, and A. J. Mehta. 1994. “Shallow Stratigraphy of Lake Okeechobee, Florida: A Preliminary Reconnaissance.” Journal of Coastal Research 10 (2): 339–350.
- Knotters, M., D. Brusm, and J. Oude Voshaar. 1995. “A Comparison of Kriging, Co-Kriging and Kriging Combined with Regression for Spatial Interpolation of Horizon Depth with Censored Observations.” Geoderma 67 (3–4): 227–246. doi:10.1016/0016-7061(95)00011-C.
- Loader, C. 1999. Local Regression and Likelihood. New York: Springer.
- Lu, Z. Y., J. Im, L. J. Quackenbush, and S. Yoo. 2013. “Remote Sensing-Based House Value Estimation Using an Optimized Regional Regression Model.” Photogrammetric Engineering & Remote Sensing 79: 809–882.
- McBratney, A. B., I. O. A. Odeh, T. F. A. Bishop, M. S. Dunbar, and T. M. Shatar. 2000. “An Overview of Pedometric Techniques for Use in Soil Survey.” Geoderma 97: 293–327. doi:10.1016/S0016-7061(00)00043-4.
- McKenzie, N. J., and P. J. Ryan. 1999. “Spatial Prediction of Soil Properties Using Environmental Correlation.” Geoderma 89 (1–2): 67–94. doi:10.1016/S0016-7061(98)00137-2.
- Moore, I., P. Gessler, G. Nielsen, and G. Peterson. 1993. “Soil Attribute Prediction Using Terrain Analysis.” Soil Science Society of America Journal 57 (2): 443. doi:10.2136/sssaj1993.03615995005700020026x.
- Moore, P. A. J., and K. R. Reddy. 1994. “Role of Eh and pH on Phosphorus Geochemistry in Sediments of Lake Okeechobee, Florida.” Journal of Environment Quality 23: 955. doi:10.2134/jeq1994.00472425002300050016x.
- Pebesma, E. J. 2004. “Multivariable Geostatistics in S: The Gstat Package.” Computers & Geosciences 30 (7): 683–691. doi:10.1016/j.cageo.2004.03.012.
- Reddy, K. R., Y. P. Sheng, and B. L. Jones 1995. “Lake Okeechobee Phosphorus Dynamics Study.” In Summary, 84. Final report submitted to the South Florida Water Management District, West Palm Beach, Fl, Contract Number C91-2393.
- SFWMD. 2014. “SFWMD Average Rainfall (30 Year: 1981-2010).” Accessed April 4, 2014. http://my.sfwmd.gov/portal/page/portal/xweb%20weather/rainfall%20historical%20%28normal%20florida%20annual%20rainfall%20map%29
- Søndergaard, M., J. P. Jensen, and E. Jeppesen. 2003. “Role of Sediment and Internal Loading of Phosphorus in Shallow Lakes.” Hydrobiologia 506–509: 135–145. doi:10.1023/B:HYDR.0000008611.12704.dd.
- Sugiura, N. 1978. “Further Analysts of the Data by Akaike’s Information Criterion and the Finite Corrections.” Communications in Statistics – Theory and Methods 7: 13–26. doi:10.1080/03610927808827599.
- Tobler, W. 1970. “A Computer Movie Simulating Urban Growth in the Detroit Region.” Economic Geography 46: 234. doi:10.2307/143141.
- Yan, Y. Y. 2011. “Development of Methods for Spatial Modeling of Sediments Quality in Lake Okeechobee, Florida.” Diss., Florida International University, Miami, FL, 200.
- Yan, Y. Y., and R. T. James 2007. “Spatial-Temporal Modeling of Sediments from 1988 to 2006, Lake Okeechobee, Florida.” ESRI User Conference, San Diego, CA, June 17–21.
- Yan, Y. Y., and R. T. James. 2012. “Spatial Modeling of Mud Thickness and Mud Weight Calculation (1988-2006), Lake Okeechobee.” Florida Geography 43: 16–36.