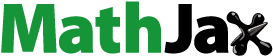
Abstract
The recently proposed Disturbance Index (DI) has been repeatedly shown to accurately detect stand-replacing disturbances. We assess the utility of the DI for monitoring more subtle disturbances using a weighting scheme that increases its sensitivity to specific disturbances. The weights were derived separately for each Landsat image in a dense time-series using linear discriminate analysis based on training data collected during a year of recovery after controlled prairie burns in the Tallgrass Prairie National Preserve in Kansas. The weights drew closer to zero as recovery progressed and the spectral differences between unburned and recovering sites diminished. The initial classification accuracy was very high, nearly 94%, but the accuracy decreased as recovery advanced. By about 100 days after the initial fire, the classification accuracy was not statistically better than what might be achieved by chance. The results clearly highlight the value of statistically fitting the DI to specific disturbances and local conditions using derived weights.
1. Introduction
While the effects of burning and subsequent recovery on grassland condition have been well studied and documented (Johnson and Matchett Citation2001; Knapp Citation1985; Neary et al. Citation1999; Ojima et al. Citation1994), the spectral response of recovering grasslands over time has received less attention. Lentile et al. (Citation2006), in their excellent survey of remote sensing of fires, note that most researchers focus on measuring effects and conditions very shortly after fire has occurred. There are many studies that have used satellite imagery to study fires in forests (Chu and Guo Citation2014; Díaz-Delgado, Lloret, and Pons Citation2003; Mitchell and Yuan Citation2010; Van Leeuwen Citation2008; Viedma et al. Citation1997; White et al. Citation1996), and chaparral (Keeley, Brennan, and Pfaff Citation2008) and scrub communities (Li and Potter Citation2012), but there are fewer studies that focus on grassland fires. One exception, Sankey, Wallace, and Ravi (Citation2013), used Fourier harmonic analysis performed on time-series’ satellite imagery to estimate the amount of time needed by vegetation to recover after fires in the rangelands of the Great Basin, USA. They found that vegetation that burned earlier in the year required more time to return to its pre-fire condition. Weber, Seefeldt, and Moffet (Citation2009) found that rangeland fire severity was more accurately mapped using short-term imagery rather than anniversary date imagery. Burn severity has been generically defined as the time required for an ecosystem to return to its pre-fire condition (Lentile et al. Citation2006).
The biggest difficulty in long-term monitoring of fire recovery is that the effects of the fire become increasingly difficult to detect using satellite imagery as recovery progresses. The methods used to classify an image collected shortly after a fire may not be useful for detecting the same fire scar after a period of recovery, especially in grasslands that recover relatively quickly. To be most effective, the classification method should be optimized for each image throughout the recovery period. Healey et al. (Citation2005) proposed a simple Disturbance Index (DI) that they argued can be weighted to increase its sensitivity to specific disturbances (Thayn Citation2013). By statistically increasing the sensitivity of the DI to the subtle differences between undisturbed and nearly recovered disturbances in a time-series of satellite imagery, researchers may be able to more accurately and more precisely monitor recovery. The current analysis serves the following purposes: First, the DI is a relatively new index whose sensitivity, especially in grasslands, has not yet been thoroughly assessed. Second, many researchers have focused on forest fires, examining the relationship between fire damage and changes in spectral reflectance patterns (Díaz-Delgado, Lloret, and Pons Citation2003; Roy, Boschetti, and Trigg Citation2006; White et al. Citation1996), and this work helps expand their findings to grasslands. Third, grasslands regenerate quickly, so studying the recovery of prairie fires allows researchers to assess recovery time within a single year, and finally, we demonstrate that the effects of prairie fires are detectable even after recovery has advanced, primarily because the weighting process exaggerates any spectral differences that remain.
2. Methodology
The DI is based on the tasseled cap transformation (TCT; Crist and Cicone Citation1984; Crist and Kauth Citation1986; Kauth and Thomas Citation1976), which rotates the original spectral values recorded in the satellite imagery to separate brightness (average reflectance), greenness (an estimate of photosynthetic activity), and wetness (moisture content) components. Healey et al. (Citation2005) reasoned that disturbed areas would demonstrate higher brightness and lower greenness and wetness values than undisturbed locations. Therefore:
where Br, Gr, and Wr are the rescaled brightness, greenness, and wetness TCT components, respectively.
Before the DI is calculated, each TCT component is rescaled as z-scores using the mean and standard deviation of healthy, undisturbed vegetation. This converts the DI to the distance, in tasselled cap feature space, between each pixel’s value and those of undisturbed vegetation, making the DI very simple to interpret. DI values greater than zero indicate disturbance; DI values close to zero indicate little to no change; and DI values less than zero suggest an increase in vegetation, possibly due to recovery. The z-score calculation is similar to the cross-correlation change detection method proposed by Koeln and Bissonnette (Citation2000).
Z-score calculation is a normalizing procedure, somewhat similar to dark object subtraction (Chavez Citation1988, 104–108), which can reduce the effects of slight atmospheric noise in images with relatively homogenous conditions (Huang et al. Citation2010; Masek et al. Citation2008). The derived weights also helped compensate for whatever slight atmospheric noise was present. The images used in this analysis were clear of clouds.
Z-scores have essentially the same range so the TCT components have equivalent weight in the calculation of the DI, implying that the contribution of each TCT component to identifying the disturbed areas is equal to that of the other components. It is likely, however, that the relative importance of each component varies by location, disturbance type, climate condition, recovery time, and other factors. Healey et al. (Citation2005) suggested that the DI might be profitably fit to local conditions by supplying higher weights to the TCT components that contribute the most to distinguishing between disturbed and undisturbed areas. This would increase the sensitivity of the DI to specific disturbances of interest and minimize the effects of other differences that may be present. Since the TCT components are converted to z-scores and have equivalent ranges, these weights would be relative to one another, i.e., a component with a weight of two would be twice as important as a component weighted with a one. The equation for a weighted DI is
where β1, β2, and β3 are the weights assigned to the brightness, greenness, and wetness components, respectively. Applying weights effectively rotates the plane of transformation in three-dimensional tasseled-cap space to achieve maximum separation of disturbed and undisturbed vegetation. Researchers who have used the DI noted that it is especially sensitive to severe disturbances (Hais et al. Citation2009; Masek et al. Citation2008; Thayn Citation2013) and relatively less sensitive to slight disturbances (Healey et al. Citation2005). Weighting the DI should increase its sensitivity to slight disturbances or to recovering disturbances, making it ideal for monitoring prairie fire recovery.
The sensitivity of a DI used to detect clear-cut and bark beetle disturbances was increased by weighting with ones the brightness and wetness components and with a zero for the greenness component (Hais et al. Citation2009). This weighting scheme was used since the expected decrease in canopy greenness was offset by a rapid increase in understory growth that occurred shortly after the disturbances. Thayn (Citation2013) found that a classification of a weighted DI was more accurate than that of an unweighted DI for mapping invasive gypsy moth defoliation in the Apostle Island National Lakeshore. A weighted DI was used to monitor 15 years of recovery of the 1988 fires in Yellowstone National Park – the weights for wetness and brightness increased and the weight for greenness decreased as recovery progressed (Klotzbach and Thayn Citation2014).
2.1. Study site
The Tallgrass Prairie National Preserve in Kansas encompasses 44.1 km2, and is owned by The Nature Conservancy (TNC) and governed by the US National Park Service (NPS). Native prairie grasses there have not been replaced with croplands because the shallow bedrock that underlies the Flint Hills region prohibits plowing. Each spring, the NPS burns specific areas of the preserve to promote prairie diversity and health. These burned areas are the focus of this research. The initial burning occurred between 27 March and 4 April 2000, and additional burns, in the south of the study area, occurred between 4 April and 12 April. The Tallgrass Prairie National Preserve averages 683 mm of precipitation during the growing season (April to October). The average growing season temperature is around 19.9°C.
Maps of previous years’ burn scars, graciously provided by resource managers at NPS, were used to guide the collection of training data used to calculate z-scores and to derive the coefficients used to weight the DI. These maps were also used as ground reference data for the accuracy assessment. The training and reference data were collected randomly and independent of one another.
2.2. Satellite data
Nine dates of Landsat imagery from 2000 – four from Landsat 5 Thematic Mapper (TM) and five from Landsat 7 Enhanced Thematic Mapper (ETM+) – were downloaded from the Global Visualization Viewer (GLOVIS) website maintained by the US Geological Survey (). The mean temporal spacing of the images is 21 days, while the minimum spacing is eight days. The largest spacing, 56 days, occurs between 12 April and 7 June because dense and frequent clouds obscured the satellite imagery. The prescribed burns of 2000 were studied because that is the most recent year before the scan line error of Landsat 7 with a sufficient cloud-free time-series of imagery.
Table 1. Dates and sensors of the nine images used in the analysis. The first image was collected before the grasslands were burned.
2.3. Analysis
All satellite images were converted to TCT components using the appropriate coefficients and ERDAS Imagine software (Intergraph Citation2013). The z-scores of the TCT components were based on the mean and standard deviation of unburned grasslands. These were calculated from 111 randomly selected points from within areas known to have not been burned during 2000 (). The majority of these points were from outside of the Tallgrass Prairie National Preserve since most of the preserve was burned. These points are independent of those used to optimize the DI and of those used to calculate classification accuracy.
Table 2. Descriptive statistics of (1) the locations used to calculate the mean and standard deviation used in generating the z-scores, (2) training points submitted to the linear discriminant analysis to find the weights for the DI, (3) the Disturbance Index, and (4) the Weighted Disturbance Index.
To our knowledge, the only research to mathematically derive weights for the DI, rather than to assign them based on a priori knowledge of the disturbance (see Hais et al. Citation2009), is the recent effort to track recovery of the 1988 fires in Yellowstone National Park (Klotzbach and Thayn Citation2014) and an earlier effort to detect gypsy moth defoliation in northern Wisconsin (Thayn Citation2013). Both used the widely popular iterative downhill simplex Nelder and Mead (Citation1965) optimization to maximize a Kruskal–Wallis test statistic that emphasized the difference between disturbed and undisturbed forest. The Nelder–Mead optimization performs well when the solution is unimodal (Nelder and Mead Citation1965); unfortunately, there seem to be multiple linear combinations of TCT components that maximize the difference between disturbed and undisturbed vegetation (Klotzbach and Thayn Citation2014). As we experimented with the Nelder–Mead optimization, we noticed that the collection of possible weights’ solutions does not resemble a unimodal peak as much as a bow-tie-shaped plateau with many possible combinations of weights that yield similar results. Under these conditions, the results of the Nelder–Mead optimization are somewhat suspect.
Since the optimization solution is not unimodal, we used a linear discriminant analysis (LDA) to determine the most appropriate weights. LDA finds a linear combination of continuous variables using an eigenfunction rotation that maximizes the difference between two or more categorical variables (McLachlan Citation2004). LDA is similar to principal component analysis or factor analysis since they also look for linear combinations of variables that best explain the data. In this example, the three normalized TCT components were rotated in three-dimensional feature space until the primary axis encompassed the most difference between unburned and burned prairie training data. The coefficients used in the LDA to linearly combine the TCT components were used in the calculation of the weighted DI (Equation (2)), which is also a linear process.
Classification of the weighted DI was done using a maximum likelihood Bayesian a posteriori estimate (Venables and Ripley Citation2002). The LDA and subsequent classification were done using the “lda” function of the R statistical environment (R Core Team Citation2012). LDA is quicker than an iterative series of Kruskal–Wallis tests and returns a more repeatable solution.
The accuracy assessment was based on 171 points randomly distributed across the study site, which were independent of those used to calculate the z-scores or to find the weighting coefficients. The confusion matrix was analyzed using the equations of Olofsson et al. (Citation2013), Stehman (Citation2013), and Stehman and Foody (Citation2009), which use occurrence weights to estimate the area of the disturbance and to calculate confidence intervals for the producers, users, and overall accuracies.
Accuracy assessments of classification results serve two primary purposes: first, to measure the accuracy of the produced thematic map image and second, to determine whether the classification procedure was more accurate than what may be expected by chance (Huberty Citation1994). The chance accuracy of classifications involving just two classes can be high, especially when one class dominates the landscape (Fielding and Bell Citation1997). In this study, around 80% of the area was burned. A classification scheme that could not discern between burned and unburned grasslands and classified the entire image as burned would have an overall chance accuracy of 80%. This is called the maximum chance accuracy. If an a priori classification were used and 80% of the pixels were randomly classified as burned, the overall chance accuracy would be nearly 70%, also known as the proportional chance accuracy. Even a very poor classification scheme could realistically be expected to produce a thematic map with 70–80% accuracy. To determine whether our classification results were statistically significantly better than these chance or artificial accuracies, and thereby demonstrate that the weighted DI and the subsequent classification were valid and robust, we calculated standard normal z-statistics that compared the chance accuracies to the actual accuracy results (Fielding and Bell Citation1997; Huberty Citation1994, 104–108).
3. Results
contains the descriptive statistics of samples of pixels from the 4 April image, which was collected just after the initial fires. Unburned grassland areas had higher values in the brightness component and lower values in the greenness and wetness components. In all components, the pixel values for the unburned areas were more concentrated around their mean. The z-scores of the unburned areas all had a mean of zero and a standard deviation of one since the mean and standard deviation of healthy, unburned grasslands were used to calculate the z-scores. Because the mean and standard deviation of unburned areas were used to calculate the z-scores, the mean values of the burned areas are their distances to unburned condition in TCT feature space. The mean of the brightness component was about five standard deviations below that of unburned grasses, suggesting that burned areas were significantly darker than the unburned areas, surely due to the accumulation of ash. This should contribute to distinguishing between the two grassland conditions.
The means of both the greenness and wetness components were between two and three standard deviations greater than those of unburned areas. For both of these bands, the traditional naming seems inappropriate. The spectral profile of ash begins at about 5% at 0.4 μm and climbs gradually to about 40% at 1.3 μm (Smith et al. Citation2005). The greenness component heavily weights the difference between red and near-infrared (NIR) reflectance as a surrogate for photosynthetic activity; however, the spectral profile of ash also shows lower reflectance in red and a higher reflectance in NIR, so, at least in this example, the “greenness” component is likely a surrogate measure of ash concentration rather than photosynthesis. The same is true for the wetness component. The data presented in suggest that burned areas are more moist that unburned areas, except that ash (and water) absorbs shortwave infrared (SWIR) wavelengths (Eva and Lambin Citation1998), so the wetness component is likely a surrogate measure of ash concentration rather than of moisture.
The mean DI value for the unburned areas is zero, with a standard deviation of 2.710, while those of the burned areas are −9.860 and 4.753, respectively. Once the DIs have been weighted, their standard deviations decrease, making the differences between them more statistically significant.
shows the difference between burned and unburned TCT component values throughout the recovery period, calculated by subtracting the mean of a random sample of burned areas from that of a random sample of unburned areas. Differences greater than zero indicate that the values of unburned areas were greater than those of burned areas, while negative differences indicate that burned areas had larger values. The biggest difference is in the brightness component early in the recovery process. At this point, the burned grasslands were covered in ash and thus were darker than the unburned areas. The peak difference is about a week after the last fire, after which the differences decrease until late recovery where they are near zero.
Figure 1. The difference in the TCT component values between burned and unburned areas, calculated by subtracting the mean of burned pixels from that of unburned pixels. Any value greater than zero indicates that the values of the unburned areas were larger than those of the burned areas.
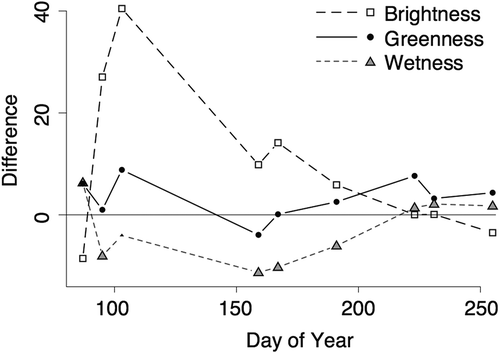
The solid line of shows that the greenness component was higher in the burned areas after the fire because of high ash concentration, which declines until about halfway through recovery, maybe because new grass shoots had appeared, which were lighter than the ash, or because some of the ash had been removed by wind or rain. Shortly after day 150, the greenness component of the unburned areas began to increase faster than that of the burned areas, but after about day 225, the difference between the two grassland conditions was essentially zero. The behavior of the wetness component, in this case tied much more closely to SWIR absorption by ash than by moisture, suggests that after the fire, the burned areas absorbed more SWIR than the unburned areas. Shortly after day 150, the difference in SWIR absorption between the two grassland conditions began to decrease until about day 225 when the difference was near zero.
shows the weighted DI images collected after the initial burn. In all cases, the lighter areas are those that were burned. The northern half and the southwest corner of the Tallgrass Prairie Nature Preserve had been burned by day 95. By day 103 most of the preserve, except for a rectangle of land near the southwest corner, had been burned. The unburned rectangle is present in all the images, although it becomes less distinct as recovery progresses. Each image is contrast stretched to two of its own standard deviations, which makes relative comparison between the images difficult; however, stretching each image internally made it easier to note the difference between the burned and unburned grasslands toward the end of the recovery period.
Figure 2. Weighted DI images of the study site for each of the eight images collected after the initial burn date. The Tallgrass Prairie National Preserve is delineated by the dashed line. The light areas are those that were burned, while the dark areas were not. The relative shades of color indicate the difference between the two classes. Notice that after the initial fires, days 95 and 103, the difference is stark, but that it declines throughout the recovery period. North is at the top of the images.
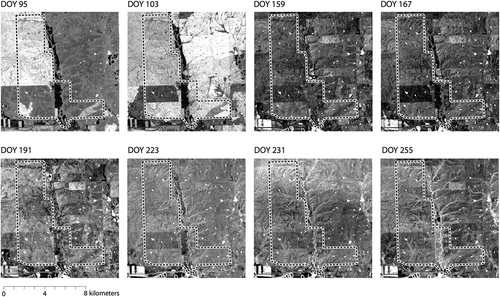
The weights of the brightness component moved toward zero (), and the slope of their fitted line was statistically significant (t = −3.332, p = 0.016), suggesting that the importance of this component decreased dramatically during recovery. However, by day 255, the weights were still close to −1, so brightness was still an important difference between the burned and unburned areas even into the later stages of recovery. The weights assigned to the wetness component also approached zero, suggesting that the variation encompassed by that component became less important over time. The slope of the line fitted to the weights of the wetness component is moderately statistically significant (t = −1.645, p = 0.151). Greenness was the only component whose relative weight remained constant throughout recovery; in other words, the slope of its fitted line was statistically equal to zero (t = −1.179, p = 0.283). In this example, the greenness component was the most consistent component for distinguishing between disturbed and undisturbed prairie grasses throughout recovery.
Figure 3. The coefficients used to weight the tasseled cap components in the disturbance index (DI). The relative importance of the brightness and greenness components decreases slightly, while that of the greenness component stays relatively constant.
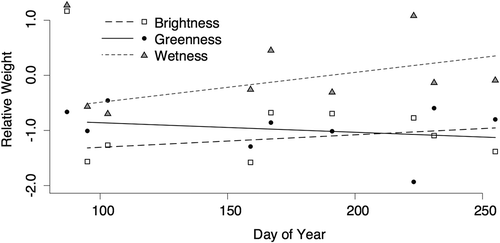
On day 87, before the fires had occurred, the weighted DI values for burned and unburned areas were close to one and were statistically indistinguishable even though the values had been weighted (). On day 95 (4 April), after the initial fires, the mean weighted DI of the burned areas was close to two, while that of the unburned areas it was near negative three. As recovery progressed, the values of both burned and unburned areas moved asymptotically toward zero. Remember that DI values near zero indicate undisturbed vegetation (Healey et al. Citation2005). By approximately day 175, some 100 days after the fire, there was considerable overlap between the ranges of the burned and unburned weighted DI values. This suggests that recovery had progressed sufficiently by that time that the burned grass had returned to its pre-burned condition (at least spectrally, and at this scale). The applied weights were derived from and unique to each image, so while the undisturbed nature of the unburned grasses did not change, the value of their weighted DI did. The relative difference in the DI values between burned and unburned grasses, not their absolute values, is the key to monitoring recovery using the weighted DI.
Figure 4. Weighted Disturbance Index values for burned and unburned grasslands through 160 days of recovery. The DI for both classes converges toward zero until they are spectrally difficult to distinguish from one another.
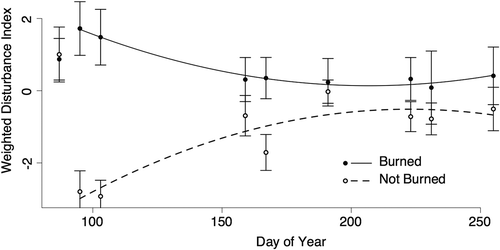
The overall accuracy of the classification of the 4 April 2000 image was 93.7 ± 3.3% (). As expected, the user and producer accuracies of the unburned areas were less than those of the burned areas since the unburned area occupied such a small portion of the study site. The user accuracy for the unburned class was the lowest at 76.3 ± 13.8%. The user and producer accuracies for the burned areas were better than 90%. By this date, nearly 37 km2 of the grassland had been burned, while about 7 km2 had not been burned. The values reported in include 95% confidence intervals.
Table 3. Accuracy assessment of the classified weighted DI image of day 95 (4 April). Part (a) shows the area-based weights; area estimates; and users, producers, and overall accuracies with 95% confidence intervals. Part (b) shows the observed and expected frequencies of correctly classified pixels, the corresponding z-statistics, and the IOC index values. The Overall p (proportional) is the expected number of sample points classified correctly if 80% of the image were randomly assigned to the burned class, since about 80% of the study area was known a priori to be burned. The Overall m (maximum) is the expected number of sample pixels correctly classified if every pixel were classed as burned (the class with the largest area). In every case, the actual classification is statistically significantly more accurate than the chance accuracies at an alpha of 0.001.
Even very poor analysis methods can return high classification accuracies when the study area is dominated by a single land-cover class (Fielding and Bell Citation1997). To demonstrate that our classification results were superior to those that might result by chance, we compared them statistically. The overall accuracy of about 94% was statistically superior to the chance accuracies of 70% (the proportional chance accuracy) and 80% (the maximum chance accuracy) at an alpha of 0.001. contains the observed frequency of correctly classified pixels, the expected frequency of correctly classified pixels that could be achieved by chance, the corresponding normal statistics, and the Improvement Over Chance (IOC) index values, all calculated according to Huberty (Citation1994, 104–108). For example, of the 32 unburned pixels from the assessment sample, 29 were classified correctly. The proportional chance frequency of correctly classified unburned pixels would be just over six pixels. The observed accuracy is statistically significantly better than the chance accuracy (z = 10.363, p < 0.000) and we can expect 88.4% fewer errors when using the weighted DI method over what might be achieved by chance.
also reports statistical comparisons of the overall accuracies under the proportional (Overall p) and the maximum (Overall m) assumptions. Of the 171 pixels from the assessment sample, 159 were correctly classified. Under the proportional chance assumption, when roughly 80% of all pixels were randomly classified as burned based on a priori knowledge, the number of correctly classified pixels would have been nearly 119. The weighted DI method produced a statistically superior result (z = 6.678, p < 0.000) and produced 77.0% fewer errors. The DI method also outperformed the maximum chance accuracy, or the highest possible chance accuracy (z = 3.969, p < 0.000), producing 62.9% fewer misclassified pixels.
The overall accuracy of the classified images decreased as recovery progressed (). The first two dates, those closest to the burn date, had accuracies greater than 90%. By early June, however, the classification accuracy was lower than 80%, which was not statistically significantly better than the maximum chance accuracy created by simply classifying all pixels as disturbed. In , the solid dots are those that were statistically better than this chance accuracy at an alpha of 0.05, and the open, white dots are those that were not statistically better than the artificial accuracy. The day 167 and day 223 images were statistically better than the chance accuracy; nevertheless, the overall trend is a decrease in accuracy as recovery progresses. Clearly, by about day 175 (about 100 days after the initial fire) the difference between burned and unburned prairie was no longer reliably detectable using a weighted DI.
Figure 5. Overall accuracy estimates of classified weighted Disturbance Index images, which decline as the difference between burned and unburned sites decreases through recovery. The filled dots are statistically significantly more accurate than the maximum chance accuracy at an alpha of 0.05. The open dots are not statistically better than chance.
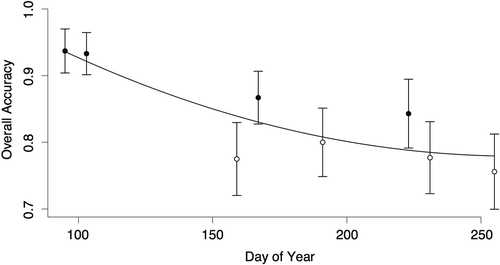
4. Discussion
The DI, after being weighted using an LDA, accurately distinguished between burned and unburned prairie grasslands. Classification accuracy, which was very high initially, dropped steadily as the burned areas recovered and the ash was replaced by healthy, verdant grass. The method was able to detect the burned areas, with accuracies statistically better than chance, through about 100 days of recovery. The classification accuracies after 100 days of recovery were still close to 80%, but they were not high enough to be statistically better than chance, under the maximum chance assumption (Huberty Citation1994,104–109).
Despite the classification success, the DI did not behave as might be expected. Burned grassland areas had lower values in the brightness TCT component than unburned areas, which contradicts the theory underlying the DI (Healey et al. Citation2005). Healey and his colleagues applied the DI to detecting disturbed forest in three different years at each of three different locations. A “stand-replacing fire” occurred in one of these scenes, while in the other eight scenes the disturbances were caused by clear-cut logging (Healey et al. Citation2005, 303). While Healey and his colleagues state that their disturbances involved complete removal of vegetation, they provide only the dates of the satellite images, not the dates of the harvesting. It is possible that some time separated the two events and that, like in Hais et al. (Citation2009), the understory might still be present or even augmented since the canopy was removed. Healey et al (Citation2005, 307) explain that the DI was less successful in their site where “re-vegetation after disturbance is fastest” and “clearcuts or fires occurring in the beginning of a monitoring period may be covered with grass, shrubs or even small trees after only 4 years.” In grasslands, all vegetation is consumed and the ground is covered in a layer of ash that absorbs incident sunlight, thereby lowering the brightness TCT values until some recovery occurs.
The greenness component also behaved differently. The DI is based on the assumption that the greenness component will be lower in disturbed areas since healthy vegetation is more photosynthetically active. The greenness component is based primarily on NIR) reflectance, which is very high over healthy vegetation. Smith et al. (Citation2005, especially ); showed that black ash absorbs NIR wavelengths while white ash reflects them. shows that the greenness component values of the burned areas were higher than those of the unburned areas, likely because the NIR light waves were being reflected by the lighter ash.
The wetness behaved as expected, but for different reasons. The wetness component is based heavily on the SWIR band of Landsat imagery, which is absorbed by moisture and so is typically low over healthy, turgid vegetation. Smith et al. (Citation2005) used field-collected spectral reflectance data to demonstrate that the albedo of SWIR is lower in burned areas. Eva and Lambin (Citation1998) found that SWIR reflectance over open savannah grasslands fell by nearly 50% after burning and that, 15 days later, it was still about 30% lower than its pre-fire value. Ash absorbs SWIR radiation, just as moisture does, which would lower the wetness component as though the burned areas were moister than the unburned areas. For detecting burn scars in grasslands, the wetness TCT component ought to be called the ash component. Smith et al. (Citation2005) found that infrared bands were correlated with fire duration and with mean fire temperature; thus, the usefulness of the wetness, or ash, component for studying grassland fires may merit additional study.
The differences in spectral patterns between burned and unburned prairie were different from those that underlie the DI; however, weighting the DI using the coefficients of the LDA seems to have compensated for the differences. Those TCT components that seem to be reversed over prairie fires were “corrected” by reversing the sign of the corresponding weight. This is an example of when fitting the DI to local conditions using a weighting scheme undoubtedly worked better than using the original, unweighted DI, just as suggested by Healey et al. (Citation2005).
The weights derived using the LDA ranged from about −1.5 to about 0.25. Weights farther from zero indicate that the corresponding TCT component encompasses a more important distinction between disturbed and undisturbed grasslands. The weights derived during the present analysis suggest that the brightness component was the most important, followed by the greenness component and finally the wetness component. Similar to the work carried out by Klotzbach and Thayn (Citation2014), the weights changed as recovery progressed, but were generally consistent relative to one another. Over time, the weights moved closer to zero, suggesting that the spectral differences between burned and unburned areas diminished with recovery until the burned areas were spectrally similar to the unburned areas. The weights assigned to the brightness and wetness TCT components approached zero more quickly than did those assigned to the greenness component. While greenness did not initially contain the most important difference between burned and unburned prairie grasses, that difference was perhaps the most reliable distinction throughout the recovery period. The larger absolute weights assigned to brightness and greenness seem to suggest that they are more important contributors to disturbance detection than is wetness, at least in tallgrass prairie ecosystems.
Healey et al. (Citation2005) suggest that positive DI values indicate disturbance, values near zero indicate no disturbance, and negative values indicate recovery. This makes interpreting the DI very intuitive; however, applying weights shifts the thresholds, making interpretation less straightforward. Immediately after the fires, the burned areas had large positive weighted DI values (which is in line with the original suggestion), but unburned areas had small negative values (which originally indicated recovery). The initial flush of vegetation in the spring had the same effect on the weighted DI as recovery after the fires, so the negative DI values suggest undisturbed areas rather than recovering areas. As recovery progressed, the relative size of the weighted DI decreased for both burned and unburned areas until they were both near zero, suggesting undisturbed vegetation. At no time during recovery did the weighted DI for burned areas drop below zero, which, according to the original suggestion, would indicate recovery. When weighting the DI, researchers should not rely on the intuitive threshold suggested by Healey et al. (Citation2005), but should base their interpretation on field observations.
The weighted DI could distinguish between burned and unburned grasslands with a very high initial classification accuracy. It monitored the return of photosynthetically active grasses through recovery with reliable but decreasing levels of classification accuracy until the spectral differences between recovered and unburned grass were too subtle to detect. This occurred around day 175, about 100 days after the initial fires. Lentile et al. (Citation2006) suggest that burn severity could be reported as the time required for a burned area to return to its pre-burn condition. Díaz-Delgado, Lloret, and Pons (Citation2003) and White et al. (Citation1996) suggest that burn severity has a strong influence on how quickly associated reflectance patterns return to a burned area. Future work might fit a curve to the p-values of the normal z-statistic comparing classification accuracy with artificial accuracies to predict the date on which the burned and unburned areas are statistically indistinguishable. The difference between this date and the date of the fire might be used as a satellite-based measure of burn severity.
Disclosure statement
No potential conflict of interest was reported by the authors.
Acknowledgment
We also wish to thank the reviewers, whose comments and suggestions vastly improved our analysis and our writing.
Additional information
Funding
References
- Chavez, P. S. 1988. “An Improved Dark-Object Subtraction Technique for Atmospheric Scattering Correction of Multispectral Data.” Remote Sensing of Environment 24: 459–479. doi:10.1016/0034-4257(88)90019-3.
- Chu, T., and X. Guo. 2014. “Remote Sensing Techniques in Monitoring Post-Fire Effects and Patterns of Forest Recovery in Boreal Forest Regions: A Review.” Remote Sensing 6: 470–520. doi:10.3390/rs6010470.
- Core Team, R. 2012. R: A Language and Environment for Statistical Computing. Vienna: R Foundation for Statistical Computing.
- Crist, E. P., and R. C. Cicone. 1984. “A Physically-Based Transformation of Thematic Mapper Data—The TM Tasseled Cap.” IEEE Transactions on Geoscience and Remote Sensing GE-22: 256–263. doi:10.1109/TGRS.1984.350619.
- Crist, E. P., and R. J. Kauth. 1986. “The Tasseled Cap De-Mystified.” Photogrammetric Engineering and Remote Sensing 52: 81–86.
- Díaz-Delgado, R., F. Lloret, and X. Pons. 2003. “Influence of Fire Severity on Plant Regeneration by Means of Remote Sensing Imagery.” International Journal of Remote Sensing 24: 1751–1763. doi:10.1080/01431160210144732.
- Eva, H., and E. F. Lambin. 1998. “Burnt Area Mapping in Central Africa Using ATSR Data.” International Journal of Remote Sensing 19: 3473–3497. doi:10.1080/014311698213768.
- Fielding, A. H., and J. F. Bell. 1997. A Review of Methods for the Assessment of Prediction Errors in Conservation Presence/Absence Models. Cambridge: ROYAUME-UNI: Cambridge University Press.
- Hais, M., M. Jonášová, J. Langhammer, and T. Kučera. 2009. “Comparison of Two Types of Forest Disturbance Using Multitemporal Landsat TM/ETM+ Imagery and Field Vegetation Data.” Remote Sensing of Environment 113: 835–845. doi:10.1016/j.rse.2008.12.012.
- Healey, S. P., W. B. Cohen, Y. Zhiqiang, and O. N. Krankina. 2005. “Comparison of Tasseled Cap-Based Landsat Data Structures for Use in Forest Disturbance Detection.” Remote Sensing of Environment 97: 301–310. doi:10.1016/j.rse.2005.05.009.
- Huang, C., S. N. Goward, J. G. Masek, N. Thomas, Z. Zhu, and J. E. Vogelmann. 2010. “An Automated Approach for Reconstructing Recent Forest Disturbance History Using Dense Landsat Time Series Stacks.” Remote Sensing of Environment 114: 183–198. doi:10.1016/j.rse.2009.08.017.
- Huberty, C. J. 1994. Applied Discriminant Analysis. New York: Wiley.
- Intergraph. 2013. Imagine [Computer software]. Atlanta, GA: Hexagon Geospatial.
- Johnson, L. C., and J. R. Matchett. 2001. “Fire and Grazing Regulate Belowground Processes in Tallgrass Prairie.” Ecology 82: 3377–3389. doi:10.1890/0012-9658(2001)082[3377:FAGRBP]2.0.CO;2.
- Kauth, R. J., and G. S. Thomas. 1976. “The Tasseled Cap – a Graphical Description of the Spectral-Temporal Development of Agricultural Crops as Seen by Landsat.” In Proceedings of the Symposium on Machine Processing of Remotely Sensed Data, 4B41–44B51. West Lafayette: Purdue University.
- Keeley, J. E., T. Brennan, and A. H. Pfaff. 2008. “Fire Severity and Ecosytem Responses following Crown Fires in California Shrublands.” Ecological Applications 18: 1530–1546. doi:10.1890/07-0836.1.
- Klotzbach, M., and J. B. Thayn. 2014. “Improved Monitoring of Forest Disturbance and Succession Using an Optimized Satellite Image Index.” Geographical Bulletin 55: 97–108.
- Knapp, A. K. 1985. “Effect of Fire and Drought on the Ecophysiology of Andropogon Gerardii and Panicum Virgatum in a Tallgrass Prairie.” Ecology 66: 1309–1320.
- Koeln, G., and J. Bissonnette. 2000. “Cross-Correlation Analysis: Mapping Landcover Change with a Historic Landcover Database and a Recent, Single-Date Multispectral Image.” Proceedings of 2000 ASPRS Annual Convention, Washington, DC.
- Lentile, L. B., Z. A. Holden, A. M. Smith, M. J. Falkowski, A. T. Hudak, P. Morgan, S. A. Lewis, P. E. Gessler, and N. C. Benson. 2006. “Remote Sensing Techniques to Assess Active Fire Characteristics and Post-Fire Effects.” International Journal of Wildland Fire 15: 319–345.
- Li, S., and C. Potter. 2012. “Patterns of Aboveground Biomass Regeneration in Post-Fire Coastal Scrub Communities.” GIScience & Remote Sensing 49: 182–201. doi:10.2747/1548-1603.49.2.182.
- Masek, J. G., C. Huang, R. Wolfe, W. Cohen, F. Hall, J. Kutler, and P. Nelson. 2008. “North American Forest Disturbance Mapped from a Decadal Landsat Record.” Remote Sensing of Environment 112: 2914–2926. doi:10.1016/j.rse.2008.02.010.
- McLachlan, G. 2004. Discriminant Analysis and Statistical Pattern Recognition. New York: John Wiley & Sons.
- Mitchell, M., and F. Yuan. 2010. “Assessing Forest Fire and Vegetation Recovery in the Black Hills, South Dakota.” GIScience & Remote Sensing 47: 276–299. doi:10.2747/1548-1603.47.2.276.
- Neary, D. G., C. C. Klopatek, L. F. DeBano, and P. F. Ffolliott. 1999. “Fire Effects on Belowground Sustainability: A Review and Synthesis.” Forest Ecology and Management 122: 51–71. doi:10.1016/S0378-1127(99)00032-8.
- Nelder, J. A., and R. Mead. 1965. “A Simplex Method for Function Minimization.” The Computer Journal 7: 308–313. doi:10.1093/comjnl/7.4.308.
- Ojima, D. S., D. Schimel, W. Parton, and C. Owensby. 1994. “Long- and Short-Term Effects of Fire on Nitrogen Cycling in Tallgrass Prairie.” Biogeochemistry 24: 67–84. doi:10.1007/BF02390180.
- Olofsson, P., G. M. Foody, S. V. Stehman, and C. E. Woodcock. 2013. “Making Better Use of Accuracy Data in Land Change Studies: Estimating Accuracy and Area and Quantifying Uncertainty Using Stratified Estimation.” Remote Sensing of Environment 129: 122–131. doi:10.1016/j.rse.2012.10.031.
- Roy, D. P., L. Boschetti, and S. N. Trigg. 2006. “Remote Sensing of Fire Severity: Assessing the Performance of the Normalized Burn Ratio.” IEEE Geoscience and Remote Sensing Letters 3: 112–116. doi:10.1109/LGRS.2005.858485.
- Sankey, J. B., C. S. Wallace, and S. Ravi. 2013. “Phenology-Based, Remote Sensing of Post-Burn Disturbance Windows in Rangelands.” Ecological Indicators 30: 35–44. doi:10.1016/j.ecolind.2013.02.004.
- Smith, A., M. J. Wooster, N. A. Drake, F. M. Dipotso, M. J. Falkowski, and A. T. Hudak. 2005. “Testing the Potential of Multi-Spectral Remote Sensing for Retrospectively Estimating Fire Severity in African Savannahs.” Remote Sensing of Environment 97: 92–115. doi:10.1016/j.rse.2005.04.014.
- Stehman, S. V. 2013. “Estimating Area from an Accuracy Assessment Error Matrix.” Remote Sensing of Environment 132: 202–211. doi:10.1016/j.rse.2013.01.016.
- Stehman, S. V., and G. M. Foody. 2009. “Accuracy Assessment.” In The SAGE Handbook of Remote Sensing, edited by T. A. Warner, M. D. Nellis, and G. M. Foody, 288–309. Thousand Oaks, CA: Sage.
- Thayn, J. B. 2013. “Using a Remotely Sensed Optimized Disturbance Index to Detect Insect Defoliation in the Apostle Islands, Wisconsin, USA.” Remote Sensing of Environment 136: 210–217. doi:10.1016/j.rse.2013.05.008.
- Van Leeuwen, W. J. 2008. “Monitoring the Effects of Forest Restoration Treatments on Post-Fire Vegetation Recovery with MODIS Multitemporal Data.” Sensors 8: 2017–2042. doi:10.3390/s8032017.
- Venables, W. N., and B. D. Ripley. 2002. Modern Applied Statistics with S. 4th ed. New York: Springer.
- Viedma, O., J. Melia, D. Segarra, and J. Garcia-Haro. 1997. “Modeling Rates of Ecosystem Recovery after Fires by Using Landsat TM Data.” Remote Sensing of Environment 61: 383–398. doi:10.1016/S0034-4257(97)00048-5.
- Weber, K. T., S. Seefeldt, and C. Moffet. 2009. “Fire Severity Model Accuracy Using Short-Term, Rapid Assessment versus Long-Term, Anniversary Date Assessment.” GIScience & Remote Sensing 46: 24–38. doi:10.2747/1548-1603.46.1.24.
- White, J. D., K. C. Ryan, C. C. Key, and S. W. Running. 1996. “Remote Sensing of Forest Fire Severity and Vegetation Recovery.” International Journal of Wildland Fire 6: 125–136.