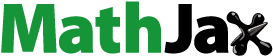
ABSTRACT
Particulate matter (PM) is a widely used indicator of air quality. Satellite-derived aerosol products such as aerosol optical depth (AOD) have been a useful source of data for ground-level PM monitoring. However, satellite-based approaches for PM monitoring have limitations such as impacts of cloud cover. Recently, many studies have documented advances in modeling for monitoring PM over the globe. This review examines recent papers on ground-level PM monitoring for the past 10 years focusing on modeling techniques, sensor types, and areas. Satellite-based retrievals of AOD and commonly used approaches for estimating PM concentrations are also briefly reviewed. Research trends and challenges are discussed based on the review of 130 papers. The limitations and challenges include spatiotemporal scale issues, missing values in satellite-based variables, sparse distribution of ground stations for calibration and validation, unbalanced distribution of PM concentrations, and difficulty in the operational use of satellite-based PM estimation models. The literature review suggests there is room for further investigating: 1) the spatial extension of PM monitoring to global scale; 2) the synergistic use of satellite-derived products and numerical model output to improve PM estimation accuracy, gap-filling, and operational monitoring; 3) the use of more advanced modeling techniques including data assimilations; 4) the improvement of emission data quality; and 5) short-term (hours to days) PM forecasts through combining satellite data and numerical forecast model results.
1. Introduction
Particulate matter (PM) is a general proxy indicator for air pollution. PM is a complex mixture of solid and liquid particles – particularly sulfate, nitrate, ammonia, black carbon, mineral dust, and water – floating in the air (World Health Organization Citation2018). The human health effects of PM2.5 (PM with a diameter smaller than 2.5 ) range from allergies to serious chronic diseases and premature death (Zhang et al. Citation2015). Therefore, it is important to continuously monitor ground PM concentrations, which can directly relate to human health, rather than the total amount of atmospheric PM at all vertical levels.
Ground PM information can be measured in the field; however, the density of existing ground stations is limited and most stations are focused on urban areas. In addition, no matter how uniformly or densely distributed, ground stations are point-measurements hence inherently not continuous on the spatial domain. In contrast, satellite sensor systems have the advantage of regularly collecting data over vast areas, hence largely overcoming the spatial limitations of ground measurements. In particular, geostationary satellite sensor systems provide data at high temporal resolution (~ minutes), which is good for air quality monitoring. Estimation of ground PM concentrations using satellite data has been widely studied, especially using aerosol products such as aerosol optical depth (AOD). AOD, a physical quantity showing the amount of aerosol in the atmosphere, characterizes the degree to which solar energy is scattered and absorbed by aerosols. Recent studies have proposed various approaches to estimate PM concentrations using the relationship between AOD and ground PM data, mainly divided into three groups: empirical-statistical approaches (Bai et al. Citation2016; de Hoogh et al. Citation2016; Zou et al. Citation2016; Guo et al. Citation2017; He and Huang Citation2018b) including artificial intelligence (Brokamp et al. Citation2018; Di et al. Citation2016; Hu et al. Citation2017; Park et al. Citation2019a), chemical transport models (CTMs) (Geng et al. Citation2015; van Donkelaar et al. Citation2019), and vertical correction models (Li et al. Citation2018b; Zhang and Li Citation2015). The performance of each method varies depending on the study area and period, and the spatial and temporal resolutions of data. Thus, it should be noted that a single method does not always perform the best across different applications.
Despite the advantages mentioned above, there are limitations in using satellite data. Although the use of AOD is crucial for estimating ground PM concentrations, AOD is not available for areas obscured by cloud cover and data quality over bright surface areas is often unreliable (Chen et al. Citation2018a; Goldberg et al. Citation2019; Guo et al. Citation2017; He and Huang Citation2018b; Huang et al. Citation2018; Liu, Weng, and Li. Citation2019; Tian and Chen Citation2010). Furthermore, in the case of empirical models, there is high uncertainty where ground stations do not exist, which intensifies limitations for obtaining spatially continuous ground PM concentrations (Bai et al. Citation2016; Hu et al. Citation2013; Zhang et al. Citation2016). In addition, since most studies have focused on how accurately ground concentrations can be estimated using historical data, there are still questions as to whether operational models have practical application and if the near-term prediction of ground PM concentrations is possible. While ground and satellite observations have been used to monitor PM concentrations (Li et al. Citation2018a; Park et al. Citation2019a; Zhang et al. Citation2018a), numerical models such as GEOS-Chem have been used for both monitoring and predicting PM (de Hoogh et al. Citation2016; Geng et al. Citation2015; van Donkelaar et al. Citation2016). Recently, satellite products (i.e. AOD) have been combined with numerical models to predict future PM concentrations (Dumitrache et al. Citation2016; Kumar et al. Citation2019; Pang et al. Citation2018).
While there are several review papers on predicting ground PM concentrations, they are limited to specific study areas, methods, and sensor types and have summarized commonly used methods or focused on comparing existing approaches (Baihuiqian, Heal, and Reis Citation2018; Sowden, Mueller, and Blake Citation2018; Zhang et al. Citation2018c). Sowden, Mueller, and Blake (Citation2018) reviewed ground dust monitoring with geostationary satellite remote sensing, reporting that high temporal resolution of geostationary satellite sensor systems fulfills the temporal requirement for air quality monitoring and assessment. Conversely, to our knowledge, there are few papers that contain comprehensive information describing different methods, and their associated performance, in estimating ground PM concentrations using satellite-derived aerosol products (Chu et al. Citation2016). Chu et al. (Citation2016) reviewed seven widely used PM prediction models in terms of their basic background, advantages, and disadvantages. The focus of that paper was on the process and methods of calculating PM concentrations from satellite-derived AOD, such as the performance of each method and the contribution of input variables. According to Chu et al. (Citation2016), the mixed-effects model (MEM) and CTM-based approaches performed well on a global scale, while the geographically weighted regression model (GWR) worked well on a regional scale. However, an in-depth analysis of satellite-derived products including AOD, the limitations of PM estimation processes, and technical trends and future directions deserve further exploration.
Therefore, this paper focuses on approaches used for quantification of ground PM concentrations using satellite-derived products such as AOD, and comprehensively reviews relevant papers that have been published during the last 10 years. We describe which satellite sensor systems and AOD retrieval algorithms have been used, and what methods have been used to properly identify the relationship between ground PM concentrations and satellite-derived products. In addition, we discuss the limitations associated with various approaches for estimating ground PM concentrations using satellite products and auxiliary data, and future research needs to mitigate them from varied perspectives. A particular interest is the operational use of ground PM estimation models. This review is organized into five sections, starting with this overview in Section 1. Section 2 briefly explains retrieval methods for satellite-derived AOD. Commonly used methods for estimating ground PM concentrations are reviewed in Section 3. The challenges and limitations found in the reviewed papers are discussed in Section 4. Finally, conclusion and future research needs are presented in Section 5.
2. Satellite-based data for estimating PM concentrations
2.1. Satellite-based data sources for PM monitoring
Air quality monitoring studies have utilized various satellite-based aerosol products including AOD, Ångström exponent (AE), fine-mode fraction (FMF), and aerosol profiles. lists currently available satellite measurements relevant to aerosols. Although there are more satellite instruments that provide aerosol products, only those commonly used are listed. While most of the instruments listed in provide aerosol products without vertical distribution, the Cloud-Aerosol Lidar with Orthogonal Polarization (CALIOP) provides an aerosol vertical profile. These instruments have slightly different AOD retrieval algorithms depending on the wavelength range or sensor type. In the following subsection, the AOD retrieval methods are briefly described when using visible light (VIS), infrared (IR), and ultraviolet (UV) wavelengths from the optical sensors and Light Detection and Ranging (LiDAR) data from the active sensors.
Table 1. Currently available satellite sensors for retrieving aerosol information.
Among a multitude of satellite sensor data, Moderate Resolution Imaging Spectroradiometer (MODIS)-based AOD data have dominated use in the literature (> 50% of the reviewed papers). Including studies using MODIS-based AOD data produced by other AOD retrieval algorithms such as Multi-Angle Implementation of Atmospheric Correction algorithm and Simplified Aerosol Retrieval Algorithm, the studies using MODIS data account for almost three-quarters of the reviewed papers. This is because MODIS has a long operational period (MODIS Terra: 1999-present, MODIS Aqua: 2002-present) and its spatial and temporal resolutions are appropriate for regional, continental, and even global investigations. However, the designed lifespan of MODIS has passed and other satellite data such as Visible Infrared Imaging Radiometer Suite (VIIRS) may be needed as a substitute in the future.
The Landsat series have been also widely used to quantify ground PM concentrations at a local scale. Researchers have used Landsat-derived AOD and meteorological factors to estimate PM concentrations using multiple linear regression (MLR). For example, the dense dark vegetation (DDV) method using MLR (Chen et al. Citation2014; Luo et al. Citation2015) is often applied to the retrieval of Landsat-based AOD. However, the DDV method is based on the assumption that the ratio of visible and shortwave IR bands is constant for dense dark vegetation thus it cannot be applied to areas with high reflectance or mixed dark and bright surface. The high resolution of Landsat data may be an advantage for urban applications if a new approach overcoming the limitation of the DDV method is applied in the future (Zhao et al. Citation2018). In addition, there is a continued effort in quantifying AOD using high spatial resolution satellite sensor data such as the Sentinel series. The resultant high spatial resolution AOD will benefit urban PM research (Mei et al. Citation2018; Li and Zhang Citation2019).
2.2. AOD retrievals by satellite observations
Aerosol retrieval methods based on optical sensors on satellites can be divided by the wavelength range used, i.e. VIS, IR, or UV. Many optical sensors cover both VIS and IR wavelengths. A representative retrieval method using optical sensors is the MODIS AOD retrieval algorithm (Levy et al. Citation2013). Since land and ocean surfaces have different reflectance characteristics, separate algorithms were developed for each. In the MODIS dark-target algorithm, land surface reflectance is calculated using three bands after masking cloud and bright surface (e.g. snow and deserts) pixels while ocean surface reflectance is calculated using six wavelengths after cloud and turbid water masking. The calculated surface reflectance is compared to the lookup table (LUT) reflectance produced by a radiative transfer model. The LUTs for land and ocean consist of different combination pairs of fine and coarse modes. The retrieved products over the land and ocean include total AOD at 0.55 μm and FMF.
Other AOD retrieval algorithms based on optical sensor data have a similar process flow as the MODIS AOD algorithm, although the spectral bands used to determine surface reflectance are different for each instrument. The Ozone Monitoring Instrument (OMI) AOD retrieval algorithm uses VIS and UV spectral bands: 17 wavelengths from 330–500 nm for the ocean and two wavelengths (342.5 and 388 nm) in the near UV for land (Stammes and Noordhoek Citation2002). The Multi-angle Imaging Spectroradiometer (MISR) retrieval algorithm uses 4 VIS bands (446, 558, 566, and 672 nm) at 9 different view zenith angles (nadir, ±26.1°, ±45.6°, ±60.0°, and ±70.5°) (Martonchik et al. Citation2002).
AOD products under various wavelength conditions have advantages and disadvantages depending on the characteristics of each wavelength. The surface is easy to observe using the VIS spectrum because transmittance is very high. However, areas that always have high surface reflectivity have low sensitivity in detecting aerosols (Korea Meteorological Administration Citation2015). The IR spectrum has the advantage of being able to observe aerosols over high surface reflectance and even at night. The drawback of using longer wavelengths is that scattering intensity is weak when the wavelength is longer than the diameter of aerosol particles. The UV spectrum has relatively low surface reflectance, which reduces sensitivity errors related to land surface reflectance properties. It is sensitive to aerosol absorption and thus less affected by clouds and snow (Torres et al. Citation2002). However, aerosol products using the UV range have a relatively low spatial resolution. Therefore, VIS bands, suitable for small particle size aerosols, are commonly used in marine and terrestrial vegetation areas with relatively low reflectance (Fukushima and Toratani Citation1997; King et al. Citation1999; Korea Meteorological Administration Citation2015). IR bands are suitable for large particle size aerosols using the difference of brightness temperature between split windows (Ackerman and Strabala Citation1994). The UV range is useful for detecting absorbing aerosols including smoke and dust, which cannot be effectively distinguished in the VIS range (Li, Li, and Mao Citation2012).
LiDAR emits energy directly from the sensor and acquires data based on the returned signals reflected or scattered by the Earth surface and atmosphere. LiDAR data can provide vertical distribution of aerosols while passive satellite data can be used to provide the overall characteristics of aerosols such as total column concentrations. CALIOP is a satellite-based LiDAR instrument. The CALIOP aerosol retrieval algorithm provides profiles of the ratio between extinction and backscattering coefficients through the elastic backscatter LiDAR equation (Young et al. Citation2012). Since LiDAR data tend to have narrow spatial coverage, they cannot be used solely to estimate spatially continuous ground-level PM concentrations. However, they have been used as an important satellite data source to calibrate AOD parameters that reflect the vertical profile of aerosols (Gong et al. Citation2017).
3. Commonly used approaches for estimating PM concentrations
A total of 130 studies from the past 10 years that predicted ground PM1, PM2.5, or PM10 concentrations using satellite-derived AOD as the main input predictor were reviewed. The characteristics of the studies reviewed are briefly presented in Table S1. Commonly used approaches for estimation of PM concentrations can be divided into three categories: empirical-statistical approaches including artificial intelligence, CTM-based approaches, and other approaches such as vertical correction modeling (). Hereafter, AOD refers to aerosol products. briefly summarizes the advantages and disadvantages of the three types of modeling approaches.
Figure 1. Approaches for estimating ground-level PM concentrations using satellite-derived aerosol products.
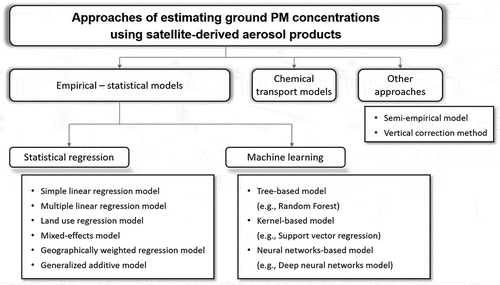
Table 2. Summary of advantages and disadvantages of commonly used approaches for estimating PM concentrations.
Early studies estimating PM concentrations were conducted using MLR, which is a relatively simple and traditional regression model (Gupta and Christopher Citation2009b). Land use regression (LUR) is usually developed using MLR based on land use-related variables that reflect the surrounding environmental characteristics such as the area of industrial land, road length, traffic counts, and population density. MLR and LUR are simple, fast and suitable for modeling the linear relationships between dependent and independent variables. However, they may not work well for estimating ground-level PM concentrations, where input variables may have a nonlinear relationship with the dependent variable. Many recent studies have used MLR and LUR for comparison with other advanced approaches for estimating PM concentrations (Gupta and Christopher Citation2009a; Saunders, Kahl, and Ghorai Citation2014; Goldberg et al. Citation2019). The accuracy of MLR in several studies was quite bad with R2 values less than 0.25, while the best model in each study had R2 greater than 0.49 (Xu et al. Citation2018; Zang et al. Citation2018, Citation2019).
GWR models have been widely used in ground PM estimation, as they account for spatial variability and local effects (Jiang et al. Citation2017; Xiao, Lang, and Christakos Citation2018). In addition to the GWR concept allowing different weights by geographical distance, Geographically and Temporally Weighted Regression (GTWR) assigns different temporal weights on past data from a target time by a specific kernel based on the temporal range. GTWR models are usually compared to GWR and Temporally Weighted Regression (TWR). GTWR models with spatiotemporal weighting have performed better than models with spatial (i.e. GWR) or temporal (i.e. TWR) weighting only (Bai et al. Citation2016; Guo et al. Citation2017). While GWR (and GTWR) has been increasingly used in PM estimation studies in recent years to consider nonstationarity in a study area, the uneven distribution of stations may increase modeling error (Vellingiri et al. Citation2016). Thus, it is important to determine appropriate parameters such as spatial range depending on study area conditions (e.g. the density of ground stations).
MEM is one of the widely used methods in recent years for the estimation of PM concentrations (). Frequently, variables used in MEM simultaneously consider fixed and random effects, to reflect the background AOD-PM relationship with fixed effects, and time and region-dependent variability with random effects (Chudnovsky et al. Citation2014; Kloog et al. Citation2015; Lee, Chatfield, and Strawa Citation2016; Zhang et al. Citation2018c). MEM can incorporate the temporal and spatial variability of the variables in a specific region using a random effect term (Xie et al. Citation2015). In other words, the random effect can be used to reduce overfitting of a model to calibration sample data (Kloog et al. Citation2015).
Figure 2. Frequency distribution of the top 7 most commonly used methods in reviewed studies that predicted ground PM concentrations using satellite AOD as the main input predictor during the past 10 years.
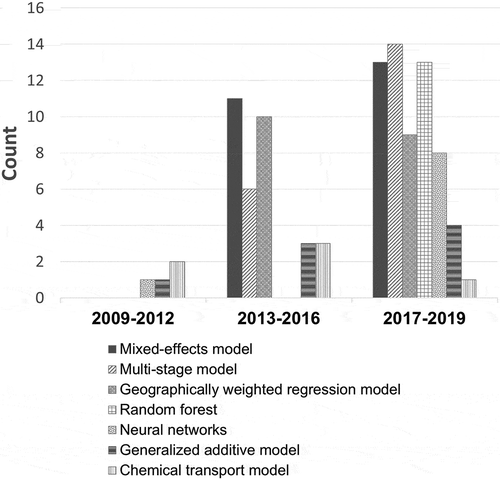
The generalized additive model (GAM) is utilized to estimate PM concentrations using input variables that have a nonlinear relationship with the target variables (Paciorek et al. Citation2008; Sorek-Hamer et al. Citation2013; Zou et al. Citation2017; Chen et al. Citation2018a). GAM is an extension of MLR that allows nonlinear functions of each variable with additive characteristics of the model using a linear combination of values (James et al. Citation2013). While there is a disadvantage that the interaction between input variables cannot be implemented in the model, this could be incorporated by adding explanatory variables of the form . GAM and Generalized Additive Mixed Models (GAMM) are increasingly being fused with other approaches for PM estimation. In such a hybrid model, the GAM and GAMM are usually used to estimate the residuals from predicted PM concentrations in previous stages such as MEM and interpolation (Kloog et al. Citation2014; Ma et al. Citation2016; de Hoogh et al. Citation2018; Hua et al. Citation2019; Stafoggia et al. Citation2019).
Machine learning is a good way to solve nonlinearity. Three types of machine learning techniques (i.e. tree-based, kernel-based, and neural networks-based) have been used to estimate surface PM concentrations. Among tree-based techniques, the classification and regression tree (CART), random forest (RF), gradient boosting machine (GBM) and extreme gradient boosting (XGB) are well known and frequently used. Although CART is very intuitive in terms of explaining the model, it suffers from overfitting especially when a tree splits to the end without pruning. While CART is not preferred for estimating PM concentrations, it has been used for comparing to other models (Jiang and Christakos Citation2018; Xu et al. Citation2018). RF developed by Breiman (Citation2001) is the most popular and widely used tree-based model using the bagging technique. It has the advantage of reducing variance and controlling the overfitting problems of CART. There has been a dramatic increase in PM estimation studies using RF recently (Hu et al. Citation2017; Brokamp et al. Citation2018; Bi et al. Citation2019; Park et al. Citation2019a). GBM and XGB are representative boosting methods that have the advantage of reducing both bias and variance of models (Friedman Citation2001; Chen and Guestrin Citation2016). Both approaches have overfitting problems, which requires tuning of model parameters (James et al. Citation2013).
Support vector regression (SVR), which is a representative algorithm using the kernel method, has been compared to other approaches in PM estimation studies (Yeganeh et al. Citation2017; Li et al. 2018a; Xu et al. Citation2018). Li et al. (2018a) compared four methods (i.e. orthogonal regression, decision tree, RF, and SVR) with 5-fold cross-validation (CV). The R2 values of the SVR models with MODIS Terra and Aqua AOD were 0.72 and 0.78, respectively, which was the second-highest performance among the four models following RF. There are several examples of studies that used SVR with other techniques in a multi-stage model (Stafoggia et al. Citation2017; de Hoogh et al. Citation2018; Yang, Xu, and Jin Citation2019).
Many neural network algorithms have been used for PM estimation, including back-propagation neural networks (BPNN), generalized regression neural network (GRNN), deep belief network (DBN), and their modifications (e.g. Principal Component Analysis (PCA)-GRNN, and Geoi-DBN). The performance of BPNN in estimating ground PM concentrations has been reported as being better than MLR but worse than that of other machine learning models such as SVR (Gupta and Christopher Citation2009a; Yeganeh et al. Citation2017). Various neural network models have been developed by applying structural deformations to improve the accuracy of the PM estimation model of typical neural networks (Li et al. Citation2017; Zang et al. Citation2019).
Empirical statistical models rely on ground measurements, thus CTM-based PM estimation can be a great approach when ground observation data are not available (Geng et al. Citation2015). Widely used CTMs for modeling PM concentrations are the Community Multiscale Air Quality (CMAQ) and the Weather Research and Forecasting with Chemistry (WRF-Chem) models (Zhang et al. Citation2018c). Although CTM can estimate temporally and spatially continuous information of air pollutants including their vertical profiles, the ground-level concentrations are highly uncertain and vary with different study regions due to inaccurate initialization, uncertainties and lack of information in emission inventories and complicated physicochemical atmospheric states (Zhang et al. Citation2018c). In addition, the spatial resolution associated with CTMs is generally too coarse to interpret complex spatial patterns on the ground especially for urban areas (Di et al. Citation2016). Therefore, early-stage CTM-based approaches use a conversion factor for a simple linear relationship between AOD and PM concentrations. CTM is sometimes combined with the corrected initial conditions using satellite AOD to improve the performance in estimating ground PM concentrations from the CTM model alone (Geng et al. Citation2015; van Donkelaar et al. Citation2015; Pang et al. Citation2018).
In addition to the empirical-statistical and CTM-based approaches, several studies have applied physical correction approaches (e.g. semi-empirical model and Specific Particle Swarm Extinction Mass Conversion Algorithm) to estimate ground PM concentrations (Ghotbi, Sotoudeheian, and Arhami Citation2016; Li et al. Citation2018b; Tian and Chen Citation2010; Wang et al. Citation2010). These vertical correction approaches can increase relevance by adjusting atmospheric vertical column AOD to lower height level AOD closer to the surface when AOD is used for estimating ground-level PM concentrations (Tian and Chen Citation2010). This vertical correction method has the advantage of being able to consider the physical dynamics of atmospheric aerosols. However, it still causes moderate uncertainty because it can only correct AOD using a few limited aerosol extinction factors such as relative humidity (RH), atmospheric boundary layer height and planetary boundary layer height (PBLH).
The advantages and disadvantages of PM estimation models vary for each approach. Therefore, researchers have developed many fusion approaches to empirical and statistical techniques to make a more robust model by mitigating weaknesses in any single model (Di et al. Citation2019). As shows, studies using multi-stage models are increasing. Using various multi-stage models, the estimation of PM concentration has improved quantitatively and qualitatively by considering the residuals between estimated results and in-situ data, and by filling empty pixels in estimated results due to missing AOD data (de Hoogh et al. Citation2018; Geng et al. Citation2018a; Hua et al. Citation2019; Stafoggia et al. Citation2019; Yao et al. Citation2019). The vertical-RH correction method has usually been performed alone. However, some studies used vertical-RH corrected PM concentrations as a target variable, yielding slightly better performance than using the original AOD, PBLH, and RH as input variables (Nabavi, Haimberger, and Abbasi Citation2019).
4. Limitations and challenges
Various approaches have been developed to estimate ground PM concentrations over the past decade. Although the performance of satellite-based PM estimation models has improved, some limitations still exist, including spatiotemporal scale issues, missing values in the satellite-based variables, sparse distribution of ground stations for calibration/validation, unbalanced distribution of PM concentrations, and difficulty in the operational use of satellite-based PM estimation models. This section discusses these five major limitations and challenges.
4.1. Spatiotemporal scale issues
A common problem identified in PM estimation studies using satellite data is scale issues related to the inclusion of different data sources on a spatiotemporal domain. Geostationary satellite sensors provide images for a specific area by maintaining the same speed as the earth rotation speed and have the advantage of high temporal resolution at intervals of minutes or hours compared to sensors in sun-synchronous polar orbits (~ days). However, geostationary sensors also have a fixed field of view causing limited spatial coverage (i.e. looking at only one side of the globe), and generally have a low spatial resolution (e.g. ~ 1–2 km). In contrast, polar-orbiting satellite sensors can provide information with a higher spatial resolution (e.g. 0.5 m to 100 m), which can be very helpful for examining detailed spatial variations over a region, especially for observing urban areas (Zhang et al. Citation2018b). There are many examples of polar-orbiting satellite data being used for long-term spatiotemporal analysis of ground PM concentrations or aerosols in the atmosphere over a region (Ma et al. Citation2016; Lin et al. Citation2018; Bai et al. Citation2019). While the temporal resolution of polar-orbiting sensors is relatively low, this can be mitigated by reducing the revisit time through synergistic use of multiple sensor data (Zheng et al. Citation2016).
Geostationary satellite sensor data have been used for continuous PM monitoring in areas with a large variation over time especially for mega-urban areas due to their advantage of high temporal resolution (Sun et al. Citation2019; Wang et al. Citation2019). However, this does not mean that geostationary satellite data are confined to monitoring. They can be also used for long-term spatiotemporal analysis if long-term data are acquired. In addition, a fusion of satellite data with multiple spatial resolutions has been emerged to estimate ground concentration at a higher spatial resolution (He and Huang Citation2018a, Citation2018b). Furthermore, more recent geostationary satellite data (e.g. Geostationary Ocean Color Imager, Himawari-8) have begun to provide advanced specifications in terms of spatial, temporal, and spectral resolutions. Research using these advanced geostationary satellite data is becoming more active (Liu, Weng, and Li. Citation2019; Zhang et al. Citation2019). High temporal resolution (< 10 minutes) of geostationary satellite sensor systems is very effective for operational monitoring air quality in specific regions such as East Asia.
Another consideration is that no matter how the spatial resolution of a satellite sensor system is improved, satellite data are provided in the form of a grid. Conversely, ground observations at stations are provided as points. There are often large discrepancies in the spatial variations of PM concentrations that exist in a grid of satellite observations as compared to point-based ground observations. Such scale issues have been reported in many studies where in situ PM concentration data is used as a reference for training or validation of the algorithms under investigation (Han et al. Citation2018; Shi et al. Citation2018), and some researchers have tried different methods to overcome this scale problem. When multiple stations are located in a grid (i.e. satellite observations), they can be regarded as individual samples (Hu et al. Citation2013), they can be simply averaged (Fang et al. Citation2016), or a weighted averaging approach using the distance from the center of the grid can be adopted to generate a representative value of the grid (Park et al. Citation2019a).
Studies within different domains (e.g. soil moisture) have tried to mitigate scale issues related to collocation between grid-based satellite data and point-based observations and calculate the uncertainty of matching two different types of data. For example, Chen et al. (Citation2017) adopted the concept of triple collocation (TC; (Stoffelen Citation1998)) to calculate a random error variance from three satellite-derived soil moisture datasets in reference to ground data. Several studies have also proposed extended TC methods to assess relative errors of soil moisture data caused by different spatial scales (Gruber et al. Citation2016). These approaches for uncertainty analysis may also be very useful in applications related to ground PM concentrations.
In addition to the spatial scale, satellite observations and ground station data do not perfectly match in temporal scale (Hu et al. Citation2017). For this reason, most studies used station data closest to satellite observation time (Jiang et al. Citation2017; Shen et al. Citation2018). Alternatively, a certain time interval (e.g. 1 hour) covering a specific satellite observation time is used to average ground PM data (Jung, Hwang, and Chen Citation2018). These approaches may also result in uncertainty in the estimation of ground PM observations.
4.2. Missing values in satellite-based products
One of the major limitations of satellite-based products is missing values (i.e. NoData). Many studies commonly use satellite-based aerosol products such as AOD, AE, FMF, and single scattering albedo as key parameters. However, these products generally do not contain information on areas covered by clouds and bright surfaces (e.g. desert, snow, and ice). Gap-filling approaches are frequently adopted to reduce missing values (Song et al. Citation2014; Xu et al. Citation2015; Geng et al. Citation2015; Lv et al. Citation2016, Citation2017; Xiao et al. Citation2017; Geng et al. Citation2018a; Zhang et al. Citation2018a; Chen et al. Citation2019; Stafoggia et al. Citation2019). AOD gap-filling approaches are summarized mainly into four groups: multiple AOD product blending, single parameter estimation (i.e. interpolation), multiple parameter estimation, and the use of model-based AOD.
One gap-filling approach regularly applied combines multiple AODs spatially or temporally. The spatially combined AOD is generated using more than two satellite-derived products with different observation times (e.g. MODIS Terra and Aqua AODs) or different observation sensors (e.g. MODIS and MISR) (Geng et al. Citation2015, Citation2018b; Han et al. Citation2018). The temporally combined AOD is usually generated by compositing AODs from one satellite data for several days (Xu et al. Citation2015; Goldberg et al. Citation2019). Although some of the missing values are filled using spatially or temporally combining approaches, generally not all missing values of AOD can be recovered with high quality. The second gap-filling approach is a single parameter estimation (i.e. spatiotemporal interpolation) of data to fill the missing values in the satellite-derived AOD data (Song et al. Citation2014; Zhang et al. Citation2018a; Chen et al. Citation2019). This approach has the advantage of providing a spatially continuous product when information is present under clear sky conditions. The disadvantage of this approach is that the uncertainty greatly increases depending on the pixels available for interpolation. The third approach is the multiple parameter estimation using other predictors. In order to estimate satellite-based AOD, multiple imputation methods and RF were used with various input variables such as model-based AOD, meteorological variable, and cloud fraction (Xiao et al. Citation2017; Huang et al. Citation2018; Bi et al. Citation2019). Lastly, some studies employed CTM or air quality model-based AOD instead of missing AOD values (Di et al. Citation2016; Hu et al. Citation2017; Stafoggia et al. Citation2019). While the use of these gap-filling approaches allows the representation of a seamless AOD distribution, they are not yet fully matured and deserve further exploration.
4.3. Sparse distribution of ground stations
The distribution of ground PM stations is often unbalanced depending on the region under investigation. Ground stations are sparsely distributed in rural and mountainous areas while typically concentrated over mega-urban areas. When PM estimation models, especially empirical models, are applied, PM concentrations estimated around sparsely distributed (or no) stations can have higher biases when compared to urban areas with a dense distribution of stations. While this increases the uncertainty of PM concentration estimation models, the density of ground stations can be used to provide estimates of this uncertainty.
4.4. Unbalanced distribution of PM concentrations
The unbalanced distributions of target variables (i.e. ground PM concentrations) are one of the challenges to train the empirical and statistical model. Previous studies reported poor performance of models at high PM concentration ranges due to smaller sample size when compared to the lower concentration areas (Ma et al. Citation2014; Xu et al. Citation2018; Zang et al. Citation2018). One method to mitigate such a problem is to increase high concentration samples using oversampling techniques (Park et al. Citation2019a). Park et al. (Citation2019a) applied the oversampling and subsampling techniques to balance the distribution of PM concentrations in terms of training samples, which is positively skewed (i.e. much more samples in low concentrations).
4.5. Difficulty in the operational use
The last main limitation lies in the difficulty of the operational use of satellite-based PM estimation models. Epidemical studies have shown the negative health effects of exposure to PM, increasing public concern about air pollution. The ultimate objective of the estimation of ground-level PM concentrations should focus not only on specific research topics but also the operational use of research findings (i.e. real-time models in operation), and thus timely, accurate PM concentration status and forecasts must be provided to the public. Although CTMs such as CMAQ are widely used for monitoring and forecasting air quality for an operational purpose, they have relatively coarse spatial resolution and high uncertainty. In order to improve the accuracy of PM concentrations estimated from CTMs, correction approaches using satellite-based AOD were developed (van Donkelaar et al. Citation2016, Citation2019). van Donkelaar et al. (Citation2016) estimated global PM2.5 concentrations using GWR and CTM-based approaches. Their models worked well in North America but didn’t provide good estimates for Asia or Africa. The mean and variance of PM concentrations in North America were low while those in East Asia and West Africa were relatively high. Such results might be because the CTMs are based on past data with initial conditions, and couldn’t reflect the rapidly changing real-time information.
Most studies based on empirical models validated the model performance using data for validation separated from training data within the same study period (You et al. Citation2016). Alternatively, there are some studies where data for predictive validation came from completely independent dates than the training data (Huang et al. Citation2018). While validation results were relatively good when validation data came from the same time period as the training data, prediction results using data collected on different days or years were worse than the validation results. Huang et al. (Citation2018) constructed their model using training data for the 2013–2015 period and performed two validations: 10-fold CV using training data, and validation using 2016 data. The overall 10-fold CV results using daily data showed R2 of 0.88 and RMSE of 14.89 μg/m3. The results of predictive validation for 2016 showed R2 of 0.74 and RMSE of 17.8 μg/m3 using monthly averaged data. Park et al. (Citation2019a) divided data into 80% for model development (i.e. model calibration and validation) and 20% for hindcast validation by date during 2015–2016 considering PM concentration levels over South Korea. The model validation results showed good performance with R2 of 0.78 and 0.73, and RMSE of 17.08 and 8.25 μg/m3 for PM10 and PM2.5, respectively. However, the hindcast validation produced relatively poor performance with R2 of 0.28 and 0.48, and RMSE of 28.07 and 17.3 μg/m3 for PM10 and PM2.5, respectively.
5. Conclusion and future research needs
This paper reviewed studies from the last 10 years that used satellite-derived aerosol products and other types of data to estimate ground-level PM concentrations. Research trends for ground-level PM monitoring were examined in terms of modeling techniques with their pros and cons. The limitations and challenges that arise from estimating PM concentrations using satellite AOD and other data were also discussed.
Based on the thorough reviews of the recent papers, we suggest that the following five aspects should be the core elements of future research. The first is a move to global scale PM monitoring. As air pollution, particularly PM, and its negative impact on human health becomes a critical societal problem in Asia (especially China and India), interest in monitoring and quantification of ground-level PM concentrations have dramatically increased (Xie et al. Citation2015; Qin et al. Citation2018; Xiao, Lang, and Christakos Citation2018; Zhai et al. Citation2018; Wang et al. Citation2019). This corresponds to an increasing number of PM studies for China and India (i.e. 63.85% and 2.31% of the reviewed papers, respectively) (Figure S1). PM concentrations are not isolated by a specific region, country or continent resulting in intercontinental transport of air pollutants, which means inter-country and inter-continental collaborations (e.g. through data sharing) are becoming very important (Anenberg et al. Citation2014). In addition, there is a strong need for generalization since many locally developed models do not work well for different areas (Di et al. Citation2016; Brokamp et al. Citation2018; Chen et al. Citation2018b). Therefore, research on global scale PM monitoring is expected to become more active (Saunders, Kahl, and Ghorai Citation2014; Silva et al. Citation2017; Ngo, Bao, and Zhong Citation2018). Three geostationary satellite sensors for air quality monitoring are scheduled to be launched within the next few years. These are the South Korean Geostationary Environment Monitoring Spectrometer (GEMS), the American Tropospheric Emission: Monitoring POllution (TEMPO), and the European Union Sentinel-4 missions for monitoring air quality in Asia, America, and Europe, respectively. In addition, more polar-orbiting satellite sensors that can be used for monitoring air quality will be launched in the future. In keeping with these plans, more research on the operational monitoring of ground-level PM concentrations globally is expected.
The second is the synergistic use of satellite-derived products and numerical model output to further improve PM estimation accuracy, gap-filling, and operational monitoring performance. Since satellite-derived products (i.e. from optical sensors) have inherent limitations due to clouds, such a synergy is crucial from multiple perspectives. While many of the empirical models used in the studies reviewed resulted in very high accuracy in validation these often showed relatively low capacity for PM prediction (Huang et al. Citation2018). Real-time training can be an alternative method to ensure prediction reliability.
The third future research focus is the use of more advanced modeling techniques. Machine learning has become widely used for ground-level PM monitoring and this trend will likely continue with the use of more advanced algorithms such as deep learning. In addition to machine learning, spatially continuous ground-level PM concentrations with uncertainty information can be integrated into a physical model through data assimilations. Park et al. (Citation2019b) proposed an RF-based cascade approach to estimate spatially continuous PM10 and PM2.5 concentrations for all-sky conditions by generating seamless AOD distribution. Satellite- and model-based products were used to produce seamless AOD and PM concentrations over East Asia including eastern China, Korea, and Japan.
The fourth focus area is the improvement of emission data quality. Among variables used as predictors in estimating PM concentration, AOD (and other aerosol variables) is the only one directly related to ground PM concentrations. Although air pollutant emission data can be used as good explanatory variables, there are huge uncertainties in the data. Many studies used emission inventory data, which are provided in terms of the total amount of emission each year (Kloog et al. Citation2011; Chudnovsky et al. Citation2014; Geng et al. Citation2015; Stafoggia et al. Citation2017). It is difficult to reflect the spatiotemporal variations of air pollutant concentrations from emission data. Emission model output has usually been used as input data for CTM (Yang et al. Citation2017). Some studies have used emission model data as predictors in PM estimation models (Park et al. Citation2019a). However, the quality of emission models is not good enough to represent detailed spatiotemporal information about emissions. Emission information is highly relevant for estimating air pollutant concentrations and if the accuracy is improved, emission models can be used as important variables in PM estimation models.
The last anticipated future research focus is the improvement of short-term (hours to days) forecast skills by combining satellite data and numerical forecast model output. Such forecasting can be achieved using past trajectory data or bias correction of numerical model prediction using satellite data. For example, Mao, Shen, and Feng (Citation2017) used AOD for the prior day, backward trajectory AOD and other auxiliary meteorological and temporal variables in a neural network to forecast hourly PM2.5 concentrations for the subsequent three days in China. As an alternative, satellite-derived AOD can help enhance the forecasting skills of CTM. Kumar et al. (Citation2019) proposed a PM forecasting model using CMAQ by assimilating MODIS AOD using the 3DVAR scheme of the community Gridpoint Statistical Interpolation system for the CMAQ model. Their assimilated forecast model produced better performance than the basic CMAQ model in most contiguous United States and they concluded that assimilation with satellite-based AOD is ready to play an operational role in air quality forecasting.
In the past, satellite remote sensing of air quality was largely limited to monitoring at low temporal resolution (e.g. monthly) and examining spatio-temporal patterns of air quality parameters. Advances in satellite sensors (e.g. GEMS) and modeling techniques (e.g. deep learning) enable shifts in research direction toward operational monitoring and forecasts of air quality (e.g. PMs), and incorporation of multiple data sources to improve monitoring and forecast skills. These efforts will, consequently, improve our responses to public health problems and broader intercountry issues on air pollution.
Supplemental Material
Download MS Word (267.7 KB)Disclosure statement
No potential conflict of interest was reported by the authors.
Supplementary Material
Supplemental data for this article can be accessed here.
Additional information
Funding
References
- Ackerman, S. A., and K. I. Strabala. 1994. “Satellite Remote Sensing of H2SO4 Aerosol Using the 8- to 12-μm Window Region: Application to Mount Pinatubo.” Journal of Geophysical Research: Atmospheres 99 (D9): 18639–18649. doi:10.1029/94JD01331.
- Anenberg, S. C., J. J. West, H. Yu, M. Chin, M. Schulz, D. Bergmann, I. Bey, et al. 2014. “Impacts of Intercontinental Transport of Anthropogenic Fine Particulate Matter on Human Mortality.” Air Quality, Atmosphere & Health 7 (3): 369–379. doi:10.1007/s11869-014-0248-9.
- Bai, K., M. Ma, N.-B. Chang, and W. Gao. 2019. “Spatiotemporal Trend Analysis for Fine Particulate Matter Concentrations in China Using High-resolution Satellite-derived and Ground-measured PM2.5 Data.” Journal of Environmental Management 233: 530–542. doi:10.1016/j.jenvman.2018.12.071.
- Bai, Y., L. Wu, K. Qin, Y. Zhang, Y. Shen, and Y. Zhou. 2016. “A Geographically and Temporally Weighted Regression Model for Ground-level PM2.5 Estimation from Satellite-derived 500 M Resolution AOD.” Remote Sensing 8 (3): 262. doi:10.3390/rs8030262.
- Baihuiqian, H., R. M. Heal, and S. Reis. 2018. “Land-Use Regression Modelling of Intra-Urban Air Pollution Variation in China: Current Status and Future Needs.” Atmosphere 9: 4. doi:10.3390/atmos9040134.
- Bi, J., J. H. Belle, Y. Wang, A. I. Lyapustin, A. Wildani, and Y. Liu. 2019. “Impacts of Snow and Cloud Covers on Satellite-derived PM2.5 Levels.” Remote Sensing of Environment 221: 665–674. doi:10.1016/j.rse.2018.12.002.
- Breiman, L. 2001. “Random Forests.” Machine Learning 45 (1): 5–32. doi:10.1023/A:1010933404324.
- Brokamp, C., R. Jandarov, M. Hossain, and P. Ryan. 2018. “Predicting Daily Urban Fine Particulate Matter Concentrations Using a Random Forest Model.” Environmental Science & Technology 52 (7): 4173–4179. doi:10.1021/acs.est.7b05381.
- Chen, F., W. T. Crow, A. Colliander, M. H. Cosh, T. J. Jackson, R. Bindlish, R. H. Reichle, et al. 2017. “Application of Triple Collocation in Ground-Based Validation of Soil Moisture Active/Passive (SMAP) Level 2 Data Products.” IEEE Journal of Selected Topics in Applied Earth Observations and Remote Sensing 10 (2): 489–502. doi:10.1109/JSTARS.2016.2569998.
- Chen, G., L. D. Knibbs, W. Zhang, S. Li, W. Cao, J. Guo, H. Ren, et al. 2018a. “Estimating Spatiotemporal Distribution of PM1 Concentrations in China with Satellite Remote Sensing, Meteorology, and Land Use Information.” Environmental Pollution 233 :1086–1094. doi:10.1016/j.envpol.2017.10.011.
- Chen, G., S. Li, L. D. Knibbs, N. A. S. Hamm, W. Cao, T. Li, J. Guo, H. Ren, M. J. Abramson, and Y. Guo. 2018b. “A Machine Learning Method to Estimate PM2.5 Concentrations across China with Remote Sensing, Meteorological and Land Use Information.” Science of the Total Environment 636: 52–60. doi:10.1016/j.scitotenv.2018.04.251.
- Chen, T., and C. Guestrin. 2016. “XGBoost: A Scalable Tree Boosting System.” In Proceedings of the 22nd ACM SIGKDD International Conference on Knowledge Discovery and Data Mining, 785–794. San Francisco, California, USA: ACM.
- Chen, Y., W. Han, S. Chen, and L. Tong. 2014. Estimating Ground-level PM2.5 Concentration Using Landsat 8 in Chengdu, China. Vol. 9259. SPIE Asia-Pacific Remote Sensing: SPIE. Beijing, China.
- Chen, Z.-Y., T.-H. Zhang, R. Zhang, Z.-M. Zhu, J. Yang, P.-Y. Chen, C.-Q. Ou, and Y. Guo. 2019. “Extreme Gradient Boosting Model to Estimate PM2.5 Concentrations with Missing-filled Satellite Data in China.” Atmospheric Environment 202: 180–189. doi:10.1016/j.atmosenv.2019.01.027.
- Chu, Y., Y. Liu, X. Li, Z. Liu, H. Lu, Y. Lu, Z. Mao, X. Chen, N. Li, and M. Ren. 2016. “A Review on Predicting Ground PM2.5 Concentration Using Satellite Aerosol Optical Depth.” Atmosphere 7 (10): 129. doi:10.3390/atmos7100129.
- Chudnovsky, A. A., P. Koutrakis, I. Kloog, S. Melly, F. Nordio, A. Lyapustin, Y. Wang, and J. Schwartz. 2014. “Fine Particulate Matter Predictions Using High Resolution Aerosol Optical Depth (AOD) Retrievals.” Atmospheric Environment 89: 189–198. doi:10.1016/j.atmosenv.2014.02.019.
- de Hoogh, K., H. Héritier, M. Stafoggia, N. Künzli, and I. Kloog. 2018. “Modelling Daily PM2.5 Concentrations at High Spatio-temporal Resolution across Switzerland.” Environmental Pollution 233: 1147–1154. doi:10.1016/j.envpol.2017.10.025.
- de Hoogh, K., J. Gulliver, A. van Donkelaar, R. V. Martin, J. D. Marshall, M. J. Bechle, G. Cesaroni, M. C. Pradas, A. Dedele, and M. Eeftens. 2016. “Development of West-European PM2.5 And NO2 Land Use Regression Models Incorporating Satellite-derived and Chemical Transport Modelling Data.” Environmental Research 151: 1–10. doi:10.1016/j.envres.2016.07.005.
- Di, Q., H. Amini, L. Shi, I. Kloog, R. Silvern, J. Kelly, M. B. Sabath, et al. 2019. “An Ensemble-based Model of PM2.5 Concentration across the Contiguous United States with High Spatiotemporal Resolution.” Environment International 130 :104909. doi:10.1016/j.envint.2019.104909.
- Di, Q., I. Kloog, P. Koutrakis, A. Lyapustin, Y. Wang, and J. Schwartz. 2016. “Assessing PM2.5 Exposures with High Spatiotemporal Resolution across the Continental United States.” Environmental Science & Technology 50 (9): 4712–4721. doi:10.1021/acs.est.5b06121.
- Dumitrache, R. C., A. Iriza, B. A. Maco, C. D. Barbu, M. Hirtl, S. Mantovani, O. Nicola, A. Irimescu, V. Craciunescu, and A. Ristea. 2016. “Study on the Influence of Ground and Satellite Observations on the Numerical Air-quality for PM10 over Romanian Territory.” Atmospheric Environment 143: 278–289. doi:10.1016/j.atmosenv.2016.08.063.
- Fang, X., B. Zou, X. Liu, T. Sternberg, and L. Zhai. 2016. “Satellite-based Ground PM2.5 Estimation Using Timely Structure Adaptive Modeling.” Remote Sensing of Environment 186: 152–163. doi:10.1016/j.rse.2016.08.027.
- Friedman, J. H. 2001. “Greedy Function Approximation: A Gradient Boosting Machine.” The Annals of Statistics 29 (5): 1189–1232. doi:10.1214/aos/1013203451.
- Fukushima, H., and M. Toratani. 1997. “Asian Dust Aerosol: Optical Effect on Satellite Ocean Color Signal and a Scheme of Its Correction.” Journal of Geophysical Research: Atmospheres 102 (D14): 17119–17130. doi:10.1029/96JD03747.
- Geng, G., N. L. Murray, D. Tong, S. Joshua, X. H. Fu, P. Lee, X. Meng, H. H. Chang, and Y. Liu. 2018b. “Satellite-Based Daily PM2.5 Estimates during Fire Seasons in Colorado.” Journal of Geophysical Research: Atmospheres 123 (15): 8159–8171. doi:10.1029/2018JD028573.
- Geng, G., N. L. Murray, H. H. Chang, and Y. Liu. 2018a. “The Sensitivity of Satellite-based PM2.5 Estimates to Its Inputs: Implications to Model Development in Data-poor Regions.” Environment International 121: 550–560. doi:10.1016/j.envint.2018.09.051.
- Geng, G., Q. Zhang, R. V. Martin, A. van Donkelaar, H. Huo, H. Che, J. Lin, and K. He. 2015. “Estimating Long-term PM2.5 Concentrations in China Using Satellite-based Aerosol Optical Depth and a Chemical Transport Model.” Remote Sensing of Environment 166: 262–270. doi:10.1016/j.rse.2015.05.016.
- Ghotbi, S., S. Sotoudeheian, and M. Arhami. 2016. “Estimating Urban Ground-level PM10 Using MODIS 3km AOD Product and Meteorological Parameters from WRF Model.” Atmospheric Environment 141: 333–346. doi:10.1016/j.atmosenv.2016.06.057.
- Goldberg, D. L., P. Gupta, K. Wang, C. Jena, Y. Zhang, Z. Lu, and D. G. Streets. 2019. “Using Gap-filled MAIAC AOD and WRF-Chem to Estimate Daily PM2.5 Concentrations at 1 Km Resolution in the Eastern United States.” Atmospheric Environment 199: 443–452. doi:10.1016/j.atmosenv.2018.11.049.
- Gong, W., Y. Huang, T. Zhang, Z. Zhu, Y. Ji, and H. Xiang. 2017. “Impact and Suggestion of Column-to-Surface Vertical Correction Scheme on the Relationship between Satellite AOD and Ground-Level PM2.5 in China.” Remote Sensing 9: 10. doi:10.3390/rs9101038.
- Gruber, A., C. H. Su, S. Zwieback, W. Crow, W. Dorigo, and W. Wagner. 2016. “Recent advances in (soil moisture) triple collocation analysis.” International Journal of Applied Earth Observation and Geoinformation 45: 200-11. doi:10.1016/j.jag.2015.09.002.
- Guo, Y., Q. Tang, D.-Y. Gong, and Z. Zhang. 2017. “Estimating Ground-level PM2.5 Concentrations in Beijing Using a Satellite-based Geographically and Temporally Weighted Regression Model.” Remote Sensing of Environment 198: 140–149. doi:10.1016/j.rse.2017.06.001.
- Gupta, P., and S. A. Christopher. 2009a. “Particulate Matter Air Quality Assessment Using Integrated Surface, Satellite, and Meteorological Products: 2. A Neural Network Approach.” Journal of Geophysical Research: Atmospheres 114 (D20): D20205, doi:10.1029/2008JD011497.
- Gupta, P., and S. A. Christopher. 2009b. “Particulate Matter Air Quality Assessment Using Integrated Surface, Satellite, and Meteorological Products: Multiple Regression Approach.” Journal of Geophysical Research: Atmospheres 114 (D14): D14205, doi:10.1029/2008JD011496.
- Han, W., L. Tong, Y. Chen, R. Li, B. Yan, and X. Liu. 2018. “Estimation of High-Resolution Daily Ground-Level PM2.5 Concentration in Beijing 2013–2017 Using 1 Km MAIAC AOT Data.” Applied Sciences 8 (12). doi:10.3390/app8122624.
- He, Q., and B. Huang. 2018a. “Satellite-based High-resolution PM2.5 Estimation over the Beijing-Tianjin-Hebei Region of China Using an Improved Geographically and Temporally Weighted Regression Model.” Environmental Pollution 236: 1027–1037. doi:10.1016/j.envpol.2018.01.053.
- He, Q., and B. Huang. 2018b. “Satellite-based Mapping of Daily High-resolution Ground PM 2.5 In China via Space-time Regression Modeling.” Remote Sensing of Environment 206: 72–83. doi:10.1016/j.rse.2017.12.018.
- Hu, X., J. H. Belle, X. Meng, A. Wildani, L. A. Waller, M. J. Strickland, and Y. Liu. 2017. “Estimating PM2.5 Concentrations in the Conterminous United States Using the Random Forest Approach.” Environmental Science & Technology 51 (12): 6936–6944. doi:10.1021/acs.est.7b01210.
- Hu, X., L. A. Waller, M. Z. Al-Hamdan, W. L. Crosson, M. G. Estes, S. M. Estes, D. A. Quattrochi, J. A. Sarnat, and Y. Liu. 2013. “Estimating Ground-level PM2.5 Concentrations in the Southeastern U.S. Using Geographically Weighted Regression.” Environmental Research 121: 1–10. doi:10.1016/j.envres.2012.11.003.
- Hua, Z., W. Sun, G. Yang, and Q. Du. 2019. “A Full-Coverage Daily Average PM2.5 Retrieval Method with Two-Stage IVW Fused MODIS C6 AOD and Two-Stage GAM Model.” Remote Sensing 11 (13). doi:10.3390/rs11131558.
- Huang, K., Q. Xiao, X. Meng, G. Geng, Y. Wang, A. Lyapustin, D. Gu, and Y. Liu. 2018. “Predicting Monthly High-resolution PM2.5 Concentrations with Random Forest Model in the North China Plain.” Environmental Pollution 242: 675–683. doi:10.1016/j.envpol.2018.07.016.
- James, G., D. Witten, T. Hastie, and R. Tibshirani. 2013. An Introduction to Statistical Learning. Vol. 112. New York: Springer.
- Jiang, M., W. Sun, G. Yang, and D. Zhang. 2017. “Modelling Seasonal GWR of Daily PM2.5 With Proper Auxiliary Variables for the Yangtze River Delta.” Remote Sensing 9 (4): 346. doi:10.3390/rs9040346.
- Jiang, Q., and G. Christakos. 2018. “Space-time Mapping of Ground-level PM2.5 And NO2 Concentrations in Heavily Polluted Northern China during Winter Using the Bayesian Maximum Entropy Technique with Satellite Data.” Air Quality, Atmosphere & Health 11 (1): 23–33. doi:10.1007/s11869-017-0514-8.
- Jung, C.-R., B.-F. Hwang, and W.-T. Chen. 2018. “Incorporating Long-term Satellite-based Aerosol Optical Depth, Localized Land Use Data, and Meteorological Variables to Estimate Ground-level PM2.5 Concentrations in Taiwan from 2005 to 2015.” Environmental Pollution 237: 1000–1010. doi:10.1016/j.envpol.2017.11.016.
- King, M. D., Y. J. Kaufman, D. Tanré, and T. Nakajima. 1999. “Remote Sensing of Tropospheric Aerosols from Space: Past, Present, and Future.” Bulletin of the American Meteorological Society 80 (11): 2229–2260. doi:10.1175/1520-0477(1999)080<2229:RSOTAF>2.0.CO;2.
- Kloog, I., A. A. Chudnovsky, A. C. Just, F. Nordio, B. A. Petros Koutrakis, A. L. Coull, Y. Wang, and J. Schwartz. 2014. “A New Hybrid Spatio-temporal Model for Estimating Daily Multi-year PM2.5 Concentrations across Northeastern USA Using High Resolution Aerosol Optical Depth Data.” Atmospheric Environment 95: 581–590. doi:10.1016/j.atmosenv.2014.07.014.
- Kloog, I., B. A. Petros Koutrakis, H. Coull, J. Lee, and J. Schwartz. 2011. “Assessing Temporally and Spatially Resolved PM2.5 Exposures for Epidemiological Studies Using Satellite Aerosol Optical Depth Measurements.” Atmospheric Environment 45 (35): 6267–6275. doi:10.1016/j.atmosenv.2011.08.066.
- Kloog, I., M. Sorek-Hamer, A. Lyapustin, B. Coull, A. C. Yujie Wang, J. S. Just, and D. M. Broday. 2015. “Estimating Daily PM2.5 And PM10 across the Complex Geo-climate Region of Israel Using MAIAC Satellite-based AOD Data.” Atmospheric Environment 122: 409–416. doi:10.1016/j.atmosenv.2015.10.004.
- Korea Meteorological Administration. 2015. “Forecaster Training Manual (Satellite Meteorology).” http://web.kma.go.kr/down/e-learning/middle/middle_06_1.pdf
- Kumar, R., L. D. Monache, J. Bresch, P. E. Saide, Y. Tang, Z. Liu, A. M. da Silva, et al. 2019. “Toward Improving Short-Term Predictions of Fine Particulate Matter over the United States via Assimilation of Satellite Aerosol Optical Depth Retrievals.” Journal of Geophysical Research: Atmospheres 124 (5): 2753–2773. doi:10.1029/2018JD029009.
- Lee, H. J., R. B. Chatfield, and A. W. Strawa. 2016. “Enhancing the Applicability of Satellite Remote Sensing for PM2.5 Estimation Using MODIS Deep Blue AOD and Land Use Regression in California, United States.” Environmental Science & Technology 50 (12): 6546–6555. doi:10.1021/acs.est.6b01438.
- Levy, R. C., S. Mattoo, L. A. Munchak, L. A. Remer, A. M. Sayer, F. Patadia, and N. C. Hsu. 2013. “The Collection 6 MODIS Aerosol Products over Land and Ocean.” Atmospheric Measurement Techniques 6 (11): 2989–3034. doi:10.5194/amt-6-2989-2013.
- Li, L., B. Chen, Y. Zhang, Y. Zhao, Y. Xian, X. Guang, H. Zhang, and L. Guo. 2018a. “Retrieval of Daily PM2.5 Concentrations Using Nonlinear Methods: A Case Study of the Beijing–Tianjin–Hebei Region, China.” Remote Sensing 10 (12). doi:10.3390/rs10122006.
- Li, Q., C. Li, and J. Mao. 2012. “Evaluation of Atmospheric Aerosol Optical Depth Products at Ultraviolet Bands Derived from MODIS Products.” Aerosol Science and Technology 46 (9): 1025–1034. doi:10.1080/02786826.2012.687475.
- Li, T., H. Shen, C. Zeng, Q. Yuan, and L. Zhang. 2017. “Point-surface Fusion of Station Measurements and Satellite Observations for Mapping PM2.5 Distribution in China: Methods and Assessment.” Atmospheric Environment 152: 477–489. doi:10.1016/j.atmosenv.2017.01.004.
- Li, X., and X. Zhang. 2019. “Predicting Ground-level PM2.5 Concentrations in the Beijing-Tianjin-Hebei Region: A Hybrid Remote Sensing and Machine Learning Approach.” Environmental Pollution 249: 735–749. doi:10.1016/j.envpol.2019.03.068.
- Li, Y., Y. Xue, J. Guang, L. She, C. Fan, and G. Chen. 2018b. “Ground-Level PM2.5 Concentration Estimation from Satellite Data in the Beijing Area Using a Specific Particle Swarm Extinction Mass Conversion Algorithm.” Remote Sensing 10 (12). doi:10.3390/rs10121906.
- Lin, C. Q., G. Liu, A. K. H. Lau, Y. Li, C. C. Li, J. C. H. Fung, and X. Q. Lao. 2018. “High-resolution Satellite Remote Sensing of Provincial PM2.5 Trends in China from 2001 to 2015.” Atmospheric Environment 180: 110–116. doi:10.1016/j.atmosenv.2018.02.045.
- Liu, J., F. Weng, and Z. Li. 2019. “Satellite-based PM2.5 Estimation Directly from Reflectance at the Top of the Atmosphere Using a Machine Learning Algorithm.” Atmospheric Environment 208: 113–122. doi:10.1016/j.atmosenv.2019.04.002.
- Luo, N., M. S. Wong, W. Zhao, X. Yan, and F. Xiao. 2015. “Improved Aerosol Retrieval Algorithm Using Landsat Images and Its Application for PM10 Monitoring over Urban Areas.” Atmospheric Research 153: 264–275. doi:10.1016/j.atmosres.2014.08.012.
- Lv, B., Y. Hu, H. H. Chang, A. G. Russell, J. Cai, B. Xu, and Y. Bai. 2017. “Daily Estimation of Ground-level PM2.5 Concentrations at 4 Km Resolution over Beijing-Tianjin-Hebei by Fusing MODIS AOD and Ground Observations.” Science of the Total Environment 580: 235–244. doi:10.1016/j.scitotenv.2016.12.049.
- Lv, B., Y. Hu, H. H. Chang, A. G. Russell, and Y. Bai. 2016. “Improving the Accuracy of Daily PM2.5 Distributions Derived from the Fusion of Ground-Level Measurements with Aerosol Optical Depth Observations, a Case Study in North China.” Environmental Science & Technology 50 (9): 4752–4759. doi:10.1021/acs.est.5b05940.
- Ma, Z., X. Hu, L. Huang, J. Bi, and Y. Liu. 2014. “Estimating Ground-Level PM2.5 In China Using Satellite Remote Sensing.” Environmental Science & Technology 48 (13): 7436–7444. doi:10.1021/es5009399.
- Ma, Z., X. Hu, M. A. Sayer, R. Levy, Q. Zhang, Y. Xue, S. Tong, J. Bi, L. Huang, and Y. Liu. 2016. “Satellite-Based Spatiotemporal Trends in PM2.5 Concentrations: China, 2004–2013.” Environmental Health Perspectives 124 (2): 184–192. doi:10.1289/ehp.1409481.
- Mao, X., T. Shen, and X. Feng. 2017. “Prediction of Hourly Ground-level PM2.5 Concentrations 3 Days in Advance Using Neural Networks with Satellite Data in Eastern China.” Atmospheric Pollution Research 8 (6): 1005–1015. doi:10.1016/j.apr.2017.04.002.
- Martonchik, J. V., D. J. Diner, K. A. Crean, and M. A. Bull. 2002. “Regional Aerosol Retrieval Results from MISR.” IEEE Transactions on Geoscience and Remote Sensing 40 (7): 1520–1531. doi:10.1109/TGRS.2002.801142.
- Mei, L., V. Rozanov, M. Vountas, J. P. Burrows, and A. Richter. 2018. “XBAER-derived Aerosol Optical Thickness from OLCI/Sentinel-3 Observation.” Atmospheric Chemistry and Physic 18 (4): 2511–2523. doi:10.5194/acp-18-2511-2018.
- Nabavi, S. O., L. Haimberger, and E. Abbasi. 2019. “Assessing PM2.5 Concentrations in Tehran, Iran, from Space Using MAIAC, Deep Blue, and Dark Target AOD and Machine Learning Algorithms.” Atmospheric Pollution Research 10 (3): 889–903. doi:10.1016/j.apr.2018.12.017.
- Ngo, N. S., X. Bao, and N. Zhong. 2018. “Local Pollutants Go Global: The Impacts of Intercontinental Air Pollution from China on Air Quality and Morbidity in California.” Environmental Research 165: 473–483. doi:10.1016/j.envres.2018.04.027.
- Paciorek, C. J., Y. Liu, H. Moreno-Macias, and S. Kondragunta. 2008. “Spatiotemporal Associations between GOES Aerosol Optical Depth Retrievals and Ground-Level PM2.5.” Environmental Science & Technology 42 (15): 5800–5806. doi:10.1021/es703181j.
- Pang, J., Z. Liu, X. Wang, J. Bresch, J. Ban, D. Chen, and J. Kim. 2018. “Assimilating AOD Retrievals from GOCI and VIIRS to Forecast Surface PM2.5 Episodes over Eastern China.” Atmospheric Environment 179: 288–304. doi:10.1016/j.atmosenv.2018.02.011.
- Park, S., J. Lee, J. Im, C.-K. Song, J. Kim, S. Lee, R. Park, S.-M. Kim, J. Yoon, and S.-K. Kim. 2019b. “Estimation of Spatially Continuous Particulate Matter Concentrations under All Sky through the Synergistic Use of Satellite-based AOD and Numerical Models”. Science of the Total Environment. (In review).
- Park, S., M. Shin, J. Im, C. K. Song, M. Choi, J. Kim, S. Lee, et al. 2019a. “Estimation of Ground-level Particulate Matter Concentrations through the Synergistic Use of Satellite Observations and Process-based Models over South Korea.” Atmospheric Chemistry and Physic 19 (2): 1097–1113. doi:10.5194/acp-19-1097-2019.
- Qin, K., J. Zou, J. Guo, M. Lu, M. Bilal, K. Zhang, F. Ma, and Y. Zhang. 2018. “Estimating PM1 Concentrations from MODIS over Yangtze River Delta of China during 2014–2017.” Atmospheric Environment 195: 149–158. doi:10.1016/j.atmosenv.2018.09.054.
- Saunders, R. O., J. D. W. Kahl, and J. K. Ghorai. 2014. “Improved Estimation of PM2.5 Using Lagrangian Satellite-measured Aerosol Optical Depth.” Atmospheric Environment 91: 146–153. doi:10.1016/j.atmosenv.2014.03.060.
- Shen, H., T. Li, Q. Yuan, and L. Zhang. 2018. “Estimating Regional Ground-Level PM2.5 Directly From Satellite Top-Of-Atmosphere Reflectance Using Deep Belief Networks.” Journal of Geophysical Research: Atmospheres 123 (24): 13,875–13,86. doi:10.1029/2018JD028759.
- Shi, Y., H. C. Ho, Y. Xu, and E. Ng. 2018. “Improving Satellite Aerosol Optical Depth-PM2.5 Correlations Using Land Use Regression with Microscale Geographic Predictors in a High-density Urban Context.” Atmospheric Environment 190: 23–34. doi:10.1016/j.atmosenv.2018.07.021.
- Silva, R. A., J. J. West, J.-F. Lamarque, D. T. Shindell, W. J. Collins, G. Faluvegi, G. A. Folberth, et al. 2017. “Future Global Mortality from Changes in Air Pollution Attributable to Climate Change.” Nature Climate Change 7 (9): 647–651. doi:10.1038/nclimate3354.
- Song, W., H. Jia, J. Huang, and Y. Zhang. 2014. “A Satellite-based Geographically Weighted Regression Model for Regional PM2.5 Estimation over the Pearl River Delta Region in China.” Remote Sensing of Environment 154: 1–7. doi:10.1016/j.rse.2014.08.008.
- Sorek-Hamer, M., A. W. Strawa, R. B. Chatfield, R. Esswein, A. Cohen, and D. M. Broday. 2013. “Improved Retrieval of PM2.5 From Satellite Data Products Using Non-linear Methods.” Environmental Pollution 182: 417–423. doi:10.1016/j.envpol.2013.08.002.
- Sowden, M., U. Mueller, and D. Blake. 2018. “Review of Surface Particulate Monitoring of Dust Events Using Geostationary Satellite Remote Sensing.” Atmospheric Environment 183: 154–164. doi:10.1016/j.atmosenv.2018.04.020.
- Stafoggia, M., J. Schwartz, C. Badaloni, T. Bellander, E. Alessandrini, G. Cattani, F. De’Donato, A. Gaeta, G. Leone, and A. Lyapustin. 2017. “Estimation of Daily PM10 Concentrations in Italy (2006–2012) Using Finely Resolved Satellite Data, Land Use Variables and Meteorology.” Environment International 99: 234–244. doi:10.1016/j.envint.2016.11.024.
- Stafoggia, M., T. Bellander, S. Bucci, M. Davoli, K. de Hoogh, F. De’ Donato, C. Gariazzo, et al. 2019. “Estimation of Daily PM10 and PM2.5 Concentrations in Italy, 2013–2015, Using a Spatiotemporal Land-use Random-forest Model.” Environment International 124 :170–179. doi:10.1016/j.envint.2019.01.016.
- Stammes, P., and R. Noordhoek. 2002. “OMI Algorithm Theoretical Basis Document Volume III Version 2.0.” https://eospso.gsfc.nasa.gov/sites/default/files/atbd/ATBD-OMI-03.pdf.
- Stoffelen, A. 1998. “Toward the True Near-surface Wind Speed: Error Modeling and Calibration Using Triple Collocation.” Journal of Geophysical Research: Oceans 103 (C4): 7755–7766. doi:10.1029/97JC03180.
- Sun, Y., Q. Zeng, B. Geng, X. Lin, B. Sude, and L. Chen. 2019. “Deep Learning Architecture for Estimating Hourly Ground-Level PM2.5 Using Satellite Remote Sensing.” IEEE Geoscience and Remote Sensing Letters 16 (9): 1343–1347. doi:10.1109/LGRS.2019.2900270.
- Tian, J., and D. Chen. 2010. “A Semi-empirical Model for Predicting Hourly Ground-level Fine Particulate Matter (PM2.5) Concentration in Southern Ontario from Satellite Remote Sensing and Ground-based Meteorological Measurements.” Remote Sensing of Environment 114 (2): 221–229. doi:10.1016/j.rse.2009.09.011.
- Torres, O., P. K. Bhartia, J. R. Herman, A. Sinyuk, P. Ginoux, and B. Holben. 2002. “A Long-Term Record of Aerosol Optical Depth from TOMS Observations and Comparison to AERONET Measurements.” Journal of the Atmospheric Sciences 59 (3): 398–413. doi:10.1175/1520-0469(2002)059<0398:ALTROA>2.0.CO;2.
- van Donkelaar, A., R. V. Martin, C. Li, and R. T. Burnett. 2019. “Regional Estimates of Chemical Composition of Fine Particulate Matter Using a Combined Geoscience-Statistical Method with Information from Satellites, Models, and Monitors.” Environmental Science & Technology 53 (5): 2595–2611. doi:10.1021/acs.est.8b06392.
- van Donkelaar, A., R. V. Martin, M. Brauer, N. Christina Hsu, R. A. Kahn, R. C. Levy, A. Lyapustin, A. M. Sayer, and D. M. Winker. 2016. “Global Estimates of Fine Particulate Matter Using a Combined Geophysical-statistical Method with Information from Satellites, Models, and Monitors.” Environmental Science & Technology 50 (7): 3762–3772. doi:10.1021/acs.est.5b05833.
- van Donkelaar, A., R. V. Martin, R. J. D. Spurr, and R. T. Burnett. 2015. “High-Resolution Satellite-Derived PM2.5 From Optimal Estimation and Geographically Weighted Regression over North America.” Environmental Science & Technology 49 (17): 10482–10491. doi:10.1021/acs.est.5b02076.
- Vellingiri, K., K.-H. Kim, J.-M. Lim, J.-H. Lee, C.-J. Ma, B.-H. Jeon, J.-R. Sohn, P. Kumar, and C.-H. Kang. 2016. “Identification of Nitrogen Dioxide and Ozone Source Regions for an Urban Area in Korea Using Back Trajectory Analysis.” Atmospheric Research 176–177: 212–221. doi:10.1016/j.atmosres.2016.02.022.
- Wang, W., F. Mao, B. Zou, J. Guo, L. Wu, Z. Pan, and L. Zang. 2019. “Two-stage Model for Estimating the Spatiotemporal Distribution of Hourly PM1.0 Concentrations over Central and East China.” Science of the Total Environment 675: 658–666. doi:10.1016/j.scitotenv.2019.04.134.
- Wang, Z., L. Chen, J. Tao, Y. Zhang, and L. Su. 2010. “Satellite-based Estimation of Regional Particulate Matter (PM) in Beijing Using vertical-and-RH Correcting Method.” Remote Sensing of Environment 114 (1): 50–63. doi:10.1016/j.rse.2009.08.009.
- World Health Organization. “Ambient (Outdoor) Air Quality and Health.” Accessed 02 August 2019. https://www.who.int/news-room/fact-sheets/detail/ambient-outdoor-air-quality-and-health
- Xiao, L., Y. Lang, and G. Christakos. 2018. “High-resolution Spatiotemporal Mapping of PM2.5 Concentrations at Mainland China Using a Combined BME-GWR Technique.” Atmospheric Environment 173: 295–305. doi:10.1016/j.atmosenv.2017.10.062.
- Xiao, Q., Y. Wang, H. H. Chang, X. Meng, G. Geng, A. Lyapustin, and Y. Liu. 2017. “Full-coverage High-resolution Daily PM2.5 Estimation Using MAIAC AOD in the Yangtze River Delta of China.” Remote Sensing of Environment 199: 437–446. doi:10.1016/j.rse.2017.07.023.
- Xie, Y., Y. Wang, K. Zhang, W. Dong, B. Lv, and Y. Bai. 2015. “Daily Estimation of Ground-Level PM2.5 Concentrations over Beijing Using 3 Km Resolution MODIS AOD.” Environmental Science & Technology 49 (20): 12280–12288. doi:10.1021/acs.est.5b01413.
- Xu, J.-W., R. V. Martin, A. Van Donkelaar, J. Kim, M. Choi, Q. Zhang, G. Geng, Y. Liu, Z. Ma, and L. Huang. 2015. “Estimating Ground-level PM2.5 In Eastern China Using Aerosol Optical Depth Determined from the GOCI Satellite Instrument.” Atmospheric Chemistry and Physics 15 (22): 13133–13144. doi:10.5194/acp-15-13133-2015.
- Xu, Y., H. C. Ho, M. S. Wong, C. Deng, Y. Shi, T.-C. Chan, and A. Knudby. 2018. “Evaluation of Machine Learning Techniques with Multiple Remote Sensing Datasets in Estimating Monthly Concentrations of Ground-level PM2. 5.” Environmental Pollution 242: 1417–1426. doi:10.1016/j.envpol.2018.08.029.
- Yang, L., H. Xu, and Z. Jin. 2019. “Estimating Ground-level PM2.5 Over a Coastal Region of China Using Satellite AOD and a Combined Model.” Journal of Cleaner Production 227: 472–482. doi:10.1016/j.jclepro.2019.04.231.
- Yang, X., Y. Zheng, G. Geng, H. Liu, H. Man, Z. Lv, K. He, and K. de Hoogh. 2017. “Development of PM2.5 And NO2 Models in a LUR Framework Incorporating Satellite Remote Sensing and Air Quality Model Data in Pearl River Delta Region, China.” Environmental Pollution 226: 143–153. doi:10.1016/j.envpol.2017.03.079.
- Yao, F., J. Wu, W. Li, and J. Peng. 2019. “A Spatially Structured Adaptive Two-stage Model for Retrieving Ground-level PM2.5 Concentrations from VIIRS AOD in China.” ISPRS Journal of Photogrammetry and Remote Sensing 151: 263–276. doi:10.1016/j.isprsjprs.2019.03.011.
- Yeganeh, B., M. G. Hewson, S. Clifford, L. D. Knibbs, and L. Morawska. 2017. “A Satellite-based Model for Estimating PM2.5 Concentration in A Sparsely Populated Environment Using Soft Computing Techniques.” Environmental Modelling & Software 88: 84–92. doi:10.1016/j.envsoft.2016.11.017.
- You, W., Z. Zang, L. Zhang, M. Zhang, X. Pan, and Y. Li. 2016. “A Nonlinear Model for Estimating Ground-level PM10 Concentration in Xi’an Using MODIS Aerosol Optical Depth Retrieval.” Atmospheric Research 168: 169–179. doi:10.1016/j.atmosres.2015.09.008.
- Young, S. A., M. A. Vaughan, R. E. Kuehn, and D. M. Winker. 2012. “The Retrieval of Profiles of Particulate Extinction from Cloud–Aerosol Lidar and Infrared Pathfinder Satellite Observations (CALIPSO) Data: Uncertainty and Error Sensitivity Analyses.” Journal of Atmospheric and Oceanic Technology 30 (3): 395–428. doi:10.1175/JTECH-D-12-00046.1.
- Zang, L., F. Mao, J. Guo, W. Gong, W. Wang, and Z. Pan. 2018. “Estimating Hourly PM1 Concentrations from Himawari-8 Aerosol Optical Depth in China.” Environmental Pollution 241: 654–663. doi:10.1016/j.envpol.2018.05.100.
- Zang, L., F. Mao, J. Guo, W. Wang, Z. Pan, H. Shen, B. Zhu, and Z. Wang. 2019. “Estimation of Spatiotemporal PM1.0 Distributions in China by Combining PM2.5 Observations with Satellite Aerosol Optical Depth.” Science of the Total Environment 658: 1256–1264. doi:10.1016/j.scitotenv.2018.12.297.
- Zhai, L., S. Li, B. Zou, H. Sang, X. Fang, and S. Xu. 2018. “An Improved Geographically Weighted Regression Model for PM2.5 Concentration Estimation in Large Areas.” Atmospheric Environment 181: 145–154. doi:10.1016/j.atmosenv.2018.03.017.
- Zhang, R., B. Di, Y. Luo, X. Deng, M. L. Grieneisen, Z. Wang, G. Yao, and Y. Zhan. 2018a. “A Nonparametric Approach to Filling Gaps in Satellite-retrieved Aerosol Optical Depth for Estimating Ambient PM2.5 Levels.” Environmental Pollution 243: 998–1007. doi:10.1016/j.envpol.2018.09.052.
- Zhang, R., G. Wang, S. Guo, M. L. Zamora, Q. Ying, Y. Lin, W. Wang, M. Hu, and Y. Wang. 2015. “Formation of Urban Fine Particulate Matter.” Chemical Reviews 115 (10): 3803–3855. doi:10.1021/acs.chemrev.5b00067.
- Zhang, T., L. Zang, Y. Wan, W. Wang, and Y. Zhang. 2019. “Ground-level PM2.5 Estimation over Urban Agglomerations in China with High Spatiotemporal Resolution Based on Himawari-8.” Science of the Total Environment 676: 535–544. doi:10.1016/j.scitotenv.2019.04.299.
- Zhang, T., W. Gong, W. Wang, Y. Ji, Z. Zhu, and Y. Huang. 2016. “Ground Level PM2.5 Estimates over China Using Satellite-Based Geographically Weighted Regression (GWR) Models are Improved by Including NO2 and Enhanced Vegetation Index (EVI).” International Journal of Environmental Research and Public Health 13 (12). doi:10.3390/ijerph13121215.
- Zhang, T., Z. Zhu, W. Gong, Z. Zhu, K. Sun, L. Wang, Y. Huang, et al. 2018b. “Estimation of Ultrahigh Resolution PM2.5 Concentrations in Urban Areas Using 160 M Gaofen-1 AOD Retrievals.” Remote Sensing of Environment 216 :91–104. doi:10.1016/j.rse.2018.06.030.
- Zhang, X., Y. Chu, Y. Wang, and K. Zhang. 2018c. “Predicting Daily PM2.5 Concentrations in Texas Using High-resolution Satellite Aerosol Optical Depth.” Science of the Total Environment 631–632: 904–911. doi:10.1016/j.scitotenv.2018.02.255.
- Zhang, Y., and Z. Li. 2015. “Remote Sensing of Atmospheric Fine Particulate Matter (PM2.5) Mass Concentration near the Ground from Satellite Observation.” Remote Sensing of Environment 160: 252–262. doi:10.1016/j.rse.2015.02.005.
- Zhao, Y., B. Huang, A. Marinoni, and P. Gamba. 2018. “High Spatiotemporal Resolution PM2.5 Concentration Estimation with Satellite and Ground Observations: A Case Study in New York City.” Paper presented at the 2018 IEEE International Conference on Environmental Engineering (EE), Milan, Italy, March 12–14.
- Zheng, Y., Q. Zhang, Y. Liu, G. Geng, and K. He. 2016. “Estimating Ground-level PM2.5 Concentrations over Three Megalopolises in China Using Satellite-derived Aerosol Optical Depth Measurements.” Atmospheric Environment 124: 232–242. doi:10.1016/j.atmosenv.2015.06.046.
- Zou, B., J. Chen, L. Zhai, X. Fang, and Z. Zheng. 2017. “Satellite Based Mapping of Ground PM2.5 Concentration Using Generalized Additive Modeling.” Remote Sensing 9 (1). doi:10.3390/rs9010001.
- Zou, B., Q. Pu, M. Bilal, Q. Weng, L. Zhai, and J. E. Nichol. 2016. “High-resolution Satellite Mapping of Fine Particulates Based on Geographically Weighted Regression.” IEEE Geoscience and Remote Sensing Letters 13 (4): 495–499. doi:10.1109/LGRS.2016.2520480.