ABSTRACT
The trend of automation, artificial intelligence, and algorithms has taken hold of strategic communication research and practice. While research on specific automated communication phenomena, such as AI influencers and chatbots, is steadily increasing in fields such as marketing and journalism, its implications for strategic communication have not yet been addressed. To offer a comprehensive overview, a systematic review of 76 articles was conducted to study the conceptualisations, methods, and normative reflections of automated communication across different social science disciplines. Findings show that automated communication has been studied in various contexts, though rarely in strategic communication. Clear and consistent terminology is often lacking and various conceptualisations for sub-phenomena, if present at all, are put forward; automated communication as an overall phenomenon has not yet been defined. Moreover, a plethora of theoretical and methodological approaches have been used, pointing to a lack of coherent knowledge. Thus, current research on automated communication is fragmented and misses a unified understanding of the phenomenon. As a remedy, this study (1) provides a typology of automated communication, (2) proposes a definition of the concept, and (3) discusses its implications for strategic communication research and practice including a research agenda.
Introduction
The field of strategic communication, here understood as “the purposeful use of communication by an organization or other entity to engage in conversations of strategic significance to its goals” (Zerfass et al., Citation2018, p. 493), will inevitably make increasing use of automated communication (Wiesenberg et al., Citation2017). Already now, news organisations, such as the Associated Press and Forbes, automatically create journalistic reports on the weather, sports, or corporate earnings (Graefe, Citation2016). The same technology could be and is used in organisations to create social media posts (Löffler et al., Citation2021), press releases, and website content. Companies such as Spotify and Starbucks use chatbots for customer service purposes (Tsai et al., Citation2021). Artificial Intelligence (AI) influencer Miquela, a computer-generated character with almost 2.6 million followers as of May 2024 (@lilmiquela on Instagram), has been engaged in campaigns by Balenciaga and Chanel (Da Silva Oliveira & Chimenti, Citation2021).
While research on these individual applications is growing, there is no consensus yet on the overall phenomenon of automated communication (Hepp et al., Citation2023). Indeed, the concept is still quite ambiguous: A plurality of terms exists with partly vague definitions and inconsistent uses. Löffler et al. (Citation2021) describe automated communication rather broadly as being “based on the methods and technologies of Big Data” (p. 117). Specifically focusing on communicative AI, Guzman and Lewis (Citation2020) encompass with this term all “technologies designed to carry out specific tasks within the communication process that were formerly associated with humans” (p. 72) while Hepp et al. (Citation2023) question “whether all automation in the communication process – including editing videos or automated translations – should be called communicative AI” (p. 47). Drawing mainly from automated journalism research, in this article automated communication, for now, is understood as “the process of using algorithms to automatically generate [content] from data” (Haim & Graefe, Citation2017, p. 1044) “for the central purpose of communication” (Hepp et al., Citation2023, p. 48).
The impact of automation on a societal, economic, and political level is much debated. Yet, despite its potential influence on strategic decision-making (Galloway & Swiatek, Citation2018), how automation impacts (strategic) communication is largely unanswered (Illia et al., Citation2023). Since automated communication is developed by computer scientists and applied in an array of organisational functions – e.g., marketing/advertising (e.g., Kietzmann et al., Citation2021), human resources (e.g., Budhwar et al., Citation2022) – and contexts – e.g., business ethics (e.g., Illia et al., Citation2023), journalism (e.g., Carlson, Citation2015), public relations (e.g., Galloway & Swiatek, Citation2018) – research is spread across disciplines pursuing diverging focal points as well as theoretical and methodological approaches.
Hence, a holistic overview of the conceptualisations, methods of analysis, and reflections of automated communication is overdue. This study aims to (1) create a typology of automated communication, (2) formulate a definition of the phenomenon, and (3) detail the implications of the automation trend for strategic communication research and practice. By consolidating the findings of previous research by means of a systematic review, this article contributes to a mutual understanding of automated communication facilitating future research and making the phenomenon and field more accessible to other social sciences and practitioners.
In the following, a theoretical background is provided on research on automated communication in the social sciences. Next, the systematic review method is reported in detail after which the results are presented along the three main elements: conceptualisation, analysis, and reflection. Here, a typology of automated communication is offered. A discussion including the implications for strategic communication research and practice follows, also providing a research agenda. The article concludes by broaching some limitations of this article and giving an outlook on automated communication (in strategic communication).
Research on automated communication in the social sciences
Referencing Actor-Network-Theory (Latour, Citation2005), it is argued that AI technologies, though being non-human, have become active participants, for example, in journalism (Primo & Zago, Citation2015) and advertising (Wu et al., Citation2023), indicating their versatility. The vast application possibilities of automated communication have been accompanied by an increased academic interest, first in journalism and marketing research. Roughly three major research topics can be identified: automated journalism, synthetic media, and bots. Moreover, endeavours have been made to find concepts encompassing different (combinations of) related phenomena: Conversational AI, generative AI, and communicative AI.
Research on the automation trend in journalism started in the 2010s (van der Kaa & Krahmer, Citation2014); a phenomenon known under various labels such as algorithmic (Dörr, Citation2016), automated (Carlson, Citation2015), computational (Clerwall, Citation2014), and robot journalism (van der Kaa & Krahmer, Citation2014). The plurality of terms may be explained by the numerous criticisms surrounding these expressions; robot journalism, for example, has been critiqued for the incorrect use of the term robot in this context (Danzon-Chambaud, Citation2021). Automated journalism is regarded as the least controversial label (Danzon-Chambaud, Citation2021) and has been conceptualised as “algorithmic processes that convert data into narrative news texts with limited to no human intervention beyond the initial programming” (Carlson, Citation2015, p. 417).
Nevertheless, AI is not only used to automatically generate news stories. More recently, the notion of synthetic media has emerged which incorporates audio-visual content generated by algorithms such as deepfakes and AI influencers (Kalpokas, Citation2021; Pawelec, Citation2022). Especially deepfakes – realistic-looking images and videos that have been manipulated and/or generated by AI technologies (Kietzmann et al., Citation2021) – and their capabilities for deception and potentially harmful consequences on an individual, organisational, and governmental level have been discussed (Chesney & Citron, Citation2019). AI influencers – virtual personalities often equipped with anthropomorphised features interacting with followers via social media – increasingly gain entry into marketing practice and research as an alternative to human influencers (Thomas & Fowler, Citation2021).
Current technological advancements have also accelerated the development and application of different kinds of bots (Ferrara et al., Citation2016): For instance, (1) chatbots are software programmes interacting with users (Shawar & Atwell, Citation2007) to “sustain interaction purely through text-based inputs and outputs” (Ling et al., Citation2021, p. 1031); (2) voice bots (Klaus & Zaichkowsky, Citation2020), such as Apple’s Siri and Amazon’s Alexa, are activated and respond via speech (Hoy, Citation2018); (3) social bots operate on social media “trying to emulate and possibly alter [other users’] behavior” (Ferrara et al., Citation2016, p. 96). Research on bots originates from various disciplines, such as education (Hwang & Chang, Citation2023), library studies (Hoy, Citation2018), marketing (Blut et al., Citation2021), and psychology (Ling et al., Citation2021). This may explain why different terminology is used and different perspectives have been applied (Lebeuf et al., Citation2019).
Concerning bots, the term conversational AI has emerged which typically refers to rule-based technologies, meaning that they are only able to provide pre-programmed responses (Lim et al., Citation2023). However, more advanced versions, such as ChatGPT, a large language model, can also be seen as an example of generative AI which is defined as “a technology that (i) leverages deep learning models to (ii) generate human-like content (e.g., images, words) in response to (iii) complex and varied prompts (e.g., languages, instructions, questions)” (Lim et al., Citation2023, p. 2). ChatGPT is also referred to as communicative AI though (Hepp et al., Citation2023), another term that has recently surfaced (Dehnert & Mongeau, Citation2022; Guzman & Lewis, Citation2020; Hepp et al., Citation2023; Natale, Citation2021; Stenbom et al., Citation2023); however, it should be noted that it is discussed on a meta-level whether it is appropriate to speak of communication when interacting with algorithms (Esposito, Citation2022). „To more precisely define the field of research on the automation of communication“(Hepp et al., Citation2023, p. 41), communicative AI “(1) is based on various forms of automation designed for the central purpose of communication, (2) is embedded within digital infrastructures, and (3) is entangled with human practices” (Hepp et al., Citation2023, p. 47). However, the fact that ChatGPT is both seen as generative AI and communicative AI calls into question whether a broader, more encompassing perspective may be fruitful to reflect the entire field of automated communication.
A need for synthesis of existing reviews of automated communication
This, to the best of our knowledge, is the first systematic review in English looking at the phenomenon of automated communication comprehensively. García-Orosa et al. (Citation2023) published a systematic review of algorithms and communication in Spanish. Apart from this more general line of investigation, sub-topics related to automated communication have been reviewed before. Existing literature reviews and meta-analyses deal with (1) definitional issues surrounding the individual phenomena of automated journalism (Danzon-Chambaud, Citation2021), deepfakes (Vasist & Krishnan, Citation2022), AI influencers (Byun & Ahn, Citation2023), and chatbots (Syvänen & Valentini, Citation2020), (2) the applied theoretical and methodological approaches (Danzon-Chambaud, Citation2021; Lim et al., Citation2022; Syvänen & Valentini, Citation2020; Vasist & Krishnan, Citation2022), (3) the impact on stakeholders, including audience reactions towards automated journalism (Graefe & Bohlken, Citation2020), AI influencers’ impact on consumer attitudes and behaviours (Laszkiewicz & Kalinska-Kula, Citation2023), chatbots’ influence on customer loyalty (Jenneboer et al., Citation2022), factors involved in users’ adoption and use of conversational agents (Ling et al., Citation2021; van Pinxteren et al., Citation2020), and (4) the opportunities and challenges of deepfakes (Westerlund, Citation2019) and chatbots (Hwang & Chang, Citation2023; Syvänen & Valentini, Citation2020).
The semantical and conceptual discrepancies within the individual phenomena as well as the different – seemingly independent from each other – endeavours to define broader concepts indicate the need for a comprehensive consolidation of extant literature’s findings. Thus, this study explores:
How is automated communication examined (conceptualised, analysed, reflected upon) in social science research and what are the implications for strategic communication?
Method
A systematic review was conducted to identify, evaluate, and synthesise relevant research “in a […] transparent, and reproducible way” (Snyder, Citation2019, p. 334). This method is increasingly used in the social sciences (Davis et al., Citation2014) and is especially useful for interdisciplinary topics. Its objective approach minimises bias to provide a comprehensive overview of relevant studies across disciplines that fit pre-defined in- and exclusion criteria to answer an explicit research question. It allows to outline the current state of research as a starting point for future studies (Booth et al., Citation2022).
Sample
Due to the interdisciplinary nature of the topic, articles addressing automation, AI, and algorithms in communication are not exclusively published in communication science journals but also in adjacent social science publications. Thus, peer-reviewed articles were retrieved from four social science databases: Business Source Premier, Communication & Mass Media Complete, PsycINFO, and Web of Science Core Collection. To collect the sample, an extensive search string was developed incorporating the main interests of this study (1) automated communication, (2) public communication, and (3) organisations (Appendix A; the supplementary materials and dataset are available at the authors’ online repository: https://doi.org/10.17605/OSF.IO/3KZVP). Search terms for automated communication included references to automation, AI, and algorithms. To be as inclusive as possible, and inspired by Zerfass et al. (Citation2018), the more general term of public communication was chosen to include potential synonyms for strategic communication (e.g., corporate communication, organisational communication), cover different fields in which strategic communication is used (e.g., political communication, science communication), and to include the addressee’s perspective of strategic communication (e.g., public perception, public sphere). Also in line with Zerfass et al.’s (Citation2018) understanding of strategic communication, various kinds of organisations that make use of it were referenced (e.g., institutions, NGOs). The full search string underwent multiple rounds of testing and refinement before the final sample was drawn. Title, abstract, keywords, and research area were searched. The third part of the search string, (3) organisations, was not used for Communication & Mass Media Complete and PsycINFO since it restricted the search results too much. No time constraints were applied.
Inclusion and exclusion criteria
The sample was collected via three searches conducted between 28 November 2022 and 2 June 2023. In total, the searches yielded 2,286 peer-reviewed results. Moreover, 22 articles were identified through reference list checking using a snowball sampling approach. After de-duplication (13.7%), 1,992 articles were eligible for screening which two independent coders performed according to pre-defined in- and exclusion criteria. The software Rayyan was used to screen the titles and abstracts. As typical for a systematic review, the initial search resulted in a large number of articles that was subsequently reduced as many articles were still outside the research scope (Lock, Citation2019; Siitonen et al., Citation2024), covering unrelated topics such as autonomous, self-driving cars and automated analysis procedures (for more exclusion decisions, see and the research diary in the supplementary materials). Since all systematic searches need to carefully balance the exhaustiveness of searching and specificity, a significant reduction of articles from the database search is the norm (Lock & Giani, Citation2021). After the first round of screening, 135 articles remained. The second round of screening involved checking the full texts of the articles. For both screenings, any disagreements on decisions were solved between the two coders in consensus sessions. 76 articles were ultimately included in the final sample (see and the list of references of included articles).
Figure 1. PRISMA flow diagram of the systematic review (adapted from Page et al., Citation2021).
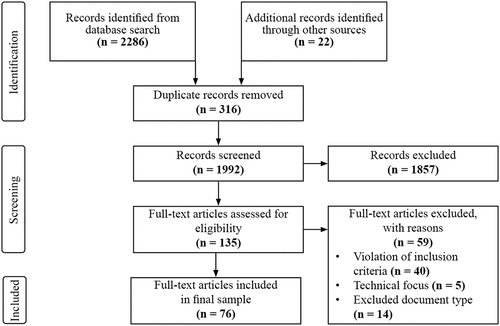
Conceptual and empirical articles were considered for inclusion (Booth et al., Citation2022). Articles were included if they (1) mainly focused on thoroughly conceptualising and/or analysing automated communication according to the working definition and/or reflecting upon its consequences, (2) examined people’s interaction with, handling of and/or reactions towards automated communication, and (3) were published and peer-reviewed. Since the focus was on peer-reviewed research and to facilitate the comparison of studies, only journal articles were included (Syvänen & Valentini, Citation2020). Hence, articles were excluded if they (1) mainly focused on the technical aspects of automated communication, were (2) literature reviews, (3) news articles, (4) book chapters, (5) book reviews, (6) editorials, essays, or forum articles, (7) commentaries, (8) encyclopedia entries, (9) dissertations, (10) conference proceedings or working papers, and (11) not written in English.
Coding scheme and data analysis
Both, quantitative and qualitative approaches were chosen for the analysis (Syvänen & Valentini, Citation2020). To conduct a quantitative content analysis, a coding scheme was prepared (Riffe et al., Citation2014) using pre-defined and open codes. To assess inter-coder reliability and to ensure replicability, a random sample of around 11% (n = 8) of the included articles was coded independently by two coders. A Krippendorff’s alpha > .667 was deemed acceptable (Krippendorff, Citation2004; Riffe et al., Citation2014). The resulting values ranged from .702 to 1; thus, inter-coder reliability was given. The coding scheme was divided into formal and content variables (Appendix B).
Formal variables covered basic information about the articles: article type (conceptual vs. empirical), author(s), author affiliation(s), title, publication year, journal name, and journal field. To determine the journal field, four citation indices for academic publications were consulted (Appendix B). The classifications were then refined by applying a communication-centered perspective.
The content variables corresponded to the three main elements of the research question: conceptualisation, analysis, and reflection. Conceptualisations are important to uncover the agreed-upon meanings of concepts (Chaffee, Citation1991); conceptual variables included the context in which automated communication was investigated (e.g., journalism, marketing), the type of automated communication that was explored (e.g., bots, deepfakes), the terminology used to address these phenomena, and the applied theoretical lens (e.g., expectancy violations theory, uses and gratifications theory). The investigated context was ascertained by the original authors’ perspective and objective (e.g., if they aimed to examine the effects of automated communication for the journalism field, the coded context was journalism). Initially, advertising and marketing had been coded separately; ultimately, the former was grouped with the latter (Keller, Citation2001). Lock et al.’s (Citation2020) compilation of communication theories was used as a guideline for the categorisation of the applied theoretical lens. Conceptualisation(s) of the overall and/or specific automated communication phenomenon (including the respective reference(s)) were extracted for a qualitative analysis. To shed light on how automated communication has been analysed, the variables research design and research method were coded for empirical articles. Lastly, reflections were also extracted for a qualitative analysis to further investigate normative assumptions about opportunities and challenges (Geise et al., Citation2022) on automated communication. Reflections were taken from article paragraphs titled theoretical and/or practical implications/reflections/opportunities/challenges/contributions/promises/perils.
To obtain more in-depth knowledge on how automated communication is (1) conceptualised and (2) reflected upon, a constructionist reflexive thematic analysis was conducted (Braun & Clarke, Citation2006; Braun et al., Citation2019). A theoretical “analyst-driven” approach was taken (Braun & Clarke, Citation2006, p. 84) since the focus was on particular aspects of the data corpus: (1) How can specific automated communication phenomena be grouped into higher-order clusters to create a typology? (2) Which aspects were reflected upon? The aim was to systematically reproduce what has been discussed in existing literature. Hence, to prevent interpretation as best as possible and to truly identify what has been explicitly written, the open coding and subsequent iterative theme development were done on a semantic level. Therefore, the final generated themes may resemble more “topic summaries” than “fully realised themes” (Braun & Clarke, Citation2021, p. 345).
Results
Overview of the studies
The sample (N = 76) included conceptual (n = 7) and empirical (n = 69) articles. Research on automated communication has considerably increased over the years (). Most articles were published in communication journals (n = 42), followed by journals in marketing (n = 13), psychology (n = 8), business (n = 5), interdisciplinary journals (n = 4), journals in information and library science (n = 2), as well as education and political science (n = 1, respectively). Five journals stand out in which at least four articles were published: Digital Journalism (n = 9), Computers in Human Behavior (n = 7), Journalism Practice (n = 7), Journal of Advertising (n = 4), and Psychology & Marketing (n = 4). In total, the articles were published in 40 different journals.
In the sample, ten different contexts were found. Most research was conducted in journalism (n = 30) and marketing (n = 24). This is followed by the contexts of political communication (n = 6), general communication (n = 5), education and general human-machine communication (n = 3, respectively), culture (n = 2) and lastly, health communication, science communication and strategic communication/PR (n = 1, respectively).
How is automated communication conceptualised in existing research?
Types
Before a closer look at terminology and conceptualisations is possible, first, the studied types, subtypes, and specific automated communication phenomena need to be reviewed ().
Table 1. Proposed typology of automated communication.
Two types of automated communication were uncovered in the sampled articles: AI agents (n = 54) – the entities (i.e., algorithms) that automatically communicate and/or generate content – and AI-generated content (n = 32) – the content that is automatically created by the before-mentioned algorithmic entities (). Both types are strongly connected since the former is always necessary to achieve the latter. Nevertheless, even though more attention has been given to the entities so far, the content has been the exclusive and sole focus in many articles which is why AI-generated content has been proposed as a type of automated communication in its own right.
Differentiating further, the type AI agent is split into two subtypes: Communicative agents (n = 34) – whose main purpose is to interact with addressees – and generative agents (n = 21) – whose main purpose is to generate content. The AI-generated content type is also divided into two subtypes: AI-generated text (n = 22) – generated content in a textual format – and AI-generated audio/visual content (n = 12) – generated content in audio and/or visual formats.
Within the AI agent type, more specific automated communication phenomena were identified which are used to communicate (n = 6) compared to those that automatically generate content (n = 2). Chatbots (n = 17) have been investigated the most within the classification of communicative agents followed by AI influencers (n = 10) – both predominantly in a marketing context (n = 10 and n = 9, respectively). The label unspecified agent (n = 2) was assigned when it was not obvious which specific kind of AI agent was analysed; in both cases, it was most probably a chatbot or voice assistant. In extant literature, voice assistants are sometimes also referred to as “speech-based chatbots” (Rese et al., Citation2020, p. 11). To differentiate between chatbots and voice assistants, the former are here understood as text-based, the latter as speech-based. Considering generative agents (and in general), AI journalists were investigated most often (n = 20). Looking closer at the subtype AI-generated text, AI-generated news stories (n = 19) make up the largest part of this classification and the more general AI-generated content type. Both these findings are in line with the analysis results that automated communication has been explored most within the journalism field. As for AI-generated audio/visual content, particularly AI-generated videos (n = 9) have been the object of investigation.
The various phenomena of automated communication () were studied to different extents within the aforementioned contexts ().
Table 2. Investigated automated communication phenomena according to context.
Even though both types – AI agent and AI-generated content – have been investigated within journalism, the most researched context in the sample, the focus was almost exclusively on the generative aspect of automated communication. In contrast, marketing, the second most researched context, showed a greater emphasis on the communicative aspect of automated communication. Within the context of strategic communication/PR, only research on social bots (n = 1) has been published.
Terminology
Within the analysed articles, consistent terminology of the discussed types was predominantly lacking. For instance, 25 different terms were used to describe an AI journalist. Danzon-Chambaud’s (Citation2021) and Syvänen and Valentini’s (Citation2020) reviews on automated journalism and chatbots, respectively, likewise uncovered a plurality of labels for their respective research interests. Moreover, Syvänen and Valentini (Citation2020) found that chatbots were also referred to by more general terms such as agents and (conversational) bots. Similarly, this analysis revealed that different specific automated communication phenomena were labelled with the same general term; AI influencers (e.g., Arsenyan & Mirowska, Citation2021), chatbots (e.g., Tsai et al., Citation2021), and voice assistants (e.g., Huang et al., Citation2022) have all been referred to as virtual agents, for example. This finding provides a rationale for being able to group certain specific automated communication phenomena – as has been done in the proposed typology (). However, to avoid conceptual ambiguity, it also suggests that specific and consistent labels for the individual studied phenomena are necessary to determine which exact research objects are investigated. In the sample, Garvey et al. (Citation2023) and Söderlund et al. (Citation2022) only talk about virtual agents opening up speculations about which specific kind is meant leading to the unspecified agent label in the analysis.
Conceptualisations
Conceptualisations are essential to specify the study object and to find agreed-upon meanings of the concepts under investigation (Chaffee, Citation1991). Nevertheless, almost half of the articles did not explicitly conceptualise or define the research object. Specific automated communication phenomena were conceptualised occasionally; explicit definitions were provided for AI journalist (n = 2), AI influencer (n = 5), chatbot (n = 2), social bot (n = 2), voice assistant (n = 2), and AI-generated news story (n = 3). Regarding the introduced subtypes, AI-generated audio/visual content was sometimes defined explicitly (n = 5). However, while three articles mentioned the term automated communication (Hepp, Citation2020; Rese et al., Citation2020; Wiesenberg & Tench, Citation2020) and one article used the term AI communication (Jungherr & Schroeder, Citation2023), the other 72 articles did not label the emerging trend of automation, AI, and algorithms in communication explicitly. Hence, they also neither conceptualised nor defined it. Among the articles concentrating on journalistic practices (and one from marketing), the trend of automated journalism (including equivalent terms such as computational and robot journalism) was explicitly defined instead (n = 19).
Theoretical approaches
Almost the same number of articles applied no theory (n = 31) as those that applied one (n = 32). Rarely, two (n = 11) or three theories (n = 2) were applied. Furthermore, a plurality of theoretical frameworks and models, namely 36 different ones, were used within the studies. Twenty-seven communication theories and models were identified (Lock et al., Citation2020). In addition, four theories from psychology, two from sociology and three Others were applied ().
Table 3. Theories applied most often in automated communication research.
How is automated communication analysed in existing research?
Out of the empirical studies (n = 69), most utilised a quantitative research design (n = 53). Considerably less employed a qualitative (n = 12) or mixed-methods (n = 4) research approach. Within the quantitative research design, experiments were used the most (n = 38), followed by survey methodology (n = 15) – together making up roughly three-quarters of the sample. Within the qualitative design, qualitative interviews were found the most (n = 9), though expert interviews were mostly missing, followed by qualitative content analysis (n = 3) and case study methodology (n = 3). Other methods employed were quantitative content analysis (n = 2) and scenario development methodology (n = 1).
How is automated communication reflected upon in existing research?
Five major themes were discovered (Appendix C) in the analysis of how automated communication was reflected upon in the sampled articles (n = 35).
An organisation’s relationship management is changing due to the integration of automated communication (e.g., Garvey et al., Citation2023) which creates tension within and outside an organisation in and beyond its industry. Inside organisations, the relationship between AI agents and communication professionals is still unclear (e.g., Dörr & Hollnbuchner, Citation2017) and the function AI agents take on (e.g., replacements, equal collaborators, subordinate assistants) is questioned and negotiated (e.g., Wang et al., Citation2022). Externally, the relationship with existing stakeholders is changing (e.g., Alboqami, Citation2023). With new stakeholders, such as programmers or Big Tech companies, a relationship needs to be established (e.g., Danzon-Chambaud & Cornia, Citation2023).
In the articles it is further discussed that the integration of these new players (e.g., programmers, Big Tech companies) changes the field’s boundaries, dependencies, and responsibilities leading to (perceived) issues with autonomy. To be able to make use of automated communication, communication professionals need access to (quality) data. Thus, they are increasingly dependent on these stakeholders. This new dependency limits their autonomy and impacts their self-government. Hence, as more outside players impinge on their work, it is becoming more interdisciplinary, extending the field’s boundaries (e.g., Danzon-Chambaud & Cornia, Citation2023). Moreover, as more and more agency is ascribed to AI (e.g., Hepp, Citation2020), responsibilities are potentially shared with AI agents (e.g., Cloudy et al., Citation2021) regarding accountability (e.g., Jamil, Citation2021) as well as decision-making (e.g., Flavián et al., Citation2023), and new skills need to be acquired (e.g., Danzon-Chambaud & Cornia, Citation2023).
The alignment of the integration of automated communication with an organisation’s social, political, and cultural environment is essential; more precisely, it needs to align with an organisation’s industry (e.g., Chandra et al., Citation2022), products and services (e.g., Franke et al., Citation2023), collaborators (e.g., Ham et al., Citation2023), intended messages (e.g., Arango et al., Citation2023), and target groups (e.g., Huang et al., Citation2022). For instance, automated communication phenomena are often described as opaque black box technologies (Pasquale, Citation2015) which may not be suitable for the finance industry in which transparency is seen as vital (Chandra et al., Citation2022). Furthermore, when advertising cosmetic products, human influencers are preferred over AI-generated versions suggesting that some – e.g., technical – product categories are more appropriate for the use of automated communication phenomena than others (Franke et al., Citation2023). The degree of anthropomorphisation of AI agents needs to be taken into account as well. While a more human-like nature, as is the case with AI influencer Miquela (@lilmiquela on Instagram), may facilitate identification with these phenomena, the opposite effect of uncanniness and creepiness can also quickly occur (e.g., Chandra et al., Citation2022).
Ethics is another often discussed factor. The integration of automated communication raises various ethical concerns considering issues such as bias (e.g., Dörr & Hollnbuchner, Citation2017), corporate (social) responsibility (e.g., Hepp, Citation2020), data privacy (e.g., Cheng & Jiang, Citation2020), liability (e.g., Jamil, Citation2021), mis-/disinformation (e.g., Cloudy et al., Citation2021), and transparency (e.g., Liu & Wei, Citation2019).
A topic that is less prevalent but relevant nonetheless is the question of whether the integration of automated communication phenomena challenges the idea of creativity and originality (e.g., Campbell et al., Citation2022) and whether AI can even imitate human creativity (e.g., Wang et al., Citation2022).
Discussion, implications, and a future research agenda
The need for a unified understanding of automated communication
The systematic review showed that a comprehensive definition of automated communication is needed. Across journal fields and studied contexts, inconsistent terminology is used, where various conceptualisations, if present at all, for sub-phenomena such as AI influencers and chatbots, are put forward. Automated communication, however, is neither conceptualised nor defined. Instead, definitions exist for sub-fields, such as automated journalism (e.g., Carlson, Citation2015; Dörr, Citation2016), or single phenomena of automated communication. On the one hand, some definitions emphasise content generation (e.g., Haim & Graefe, Citation2017). On the other hand, definitions focus solely on the communicative function of AI (e.g., Guzman & Lewis, Citation2020; Hepp et al., Citation2023). The analysis revealed, however, that within the field of journalism, AI journalists generate content while within the field of marketing, AI influencers are used to communicate with followers. Thus, as shown in the proposed typology (), here it is argued that automated communication is both – content generation and communication. Hence, communicative AI (Hepp et al., Citation2023) is but one part of automated communication, next to content generation. Incorporating this perspective, we follow Zerfass et al. (Citation2018) and propose a definition of automated communication to facilitate a “shared language that […] resonates with scholars and practitioners” (p. 502):
Automated communication is the process in which an artificial intelligence agent interacts with human or non-human actors by generating textual, audio, or visual content, by distributing it, or both.
The proposed definition contains four components that demand further explanation: the (1) process, (2) artificial intelligence agent, (3) type of interaction partner, and (4) AI-generated content.
Communication here is understood as a dynamic process of an ongoing exchange between at least two involved parties. It is not a mere information transmission but a continuous interaction dependent on contextual factors (e.g., source/receiver of communication, purpose of communication) affecting each other (Berlo, Citation1960). This communication is an essential part of both automated communication types – AI agent and AI-generated content (); communication with an AI agent has to take place to interact with an addressee and to create AI-generated content.
An AI agent can take on the role of a sender, receiver, and/or intermediary in the communication process. As a sender, an AI agent initiates the communication process and/or transmits information on demand. An AI influencer publishes a post on social media (Arsenyan & Mirowska, Citation2021) or an AI text agent produces a text upon request (Illia et al., Citation2023). Being the receiver of communication, an AI agent is addressed and interprets the given message; for instance, Amazon’s Alexa, a voice assistant, is activated by using specific key phrases (Flavián et al., Citation2023). Regarding the intermediary role, an AI agent can act as a go-between. Social bots often serve as intermediaries distributing information on social media, filtering and selecting the messages that are presented to the receiver (Keller & Klinger, Citation2019), but not necessarily generating content. Though not explicitly part of this study, recommender systems may also act as intermediaries (Roy & Dutta, Citation2022).
An AI agent does not only differ in its main purpose (communicative and generative) but also in its technical capacities. A rule-based chatbot (Chandra et al., Citation2022) simply follows pre-defined rules to arrive at a reply to a request. This fits the original idea of automation in which a system’s “actions are predefined from the beginning and it cannot change them into the future” (Vagia et al., Citation2016, p. 191). However, depending on its application purpose and its machine learning capabilities, an AI agent can also be more autonomous. AI-powered chatbots (Huang et al., Citation2022), such as ChatGPT (Jungherr & Schroeder, Citation2023), for instance, can adapt the content they provide, the style it is presented in, and the length of their reply to the user request; they can learn from user input and improve their accuracy and communication abilities. Thus, taking different levels of autonomy in automation into account (Vagia et al., Citation2016), the proposed definition can be used for AI agents exhibiting different degrees of autonomy.
While it is indisputable that a communication process includes an interaction partner, the proposed definition acknowledges and highlights that the interaction partners of automated communication can be human – voice assistants are accessed by humans (Flavián et al., Citation2023) – as well as non-human (Latour, Citation2005) – social bots can interact with each other without human intervention (Keller & Klinger, Citation2019).
AI-generated content is the output that is automatically created, distributed, or both by an AI agent in the automated communication process. It comes in different formats: textual (Wu et al., Citation2020), audio (Diakopoulos & Johnson, Citation2021), and/or visual (Campbell et al., Citation2022).
The unification effort done with this article by proposing a definition of automated communication and a typology of its applications () represents a first step in creating a strong foundation for the field of automated communication. Reflecting the entire field of automated communication to clearly outline its scope and demarcations, provides common ground and contextual understanding among researchers (Chaffee, Citation1991). This, in turn, facilitates the systematic study of automated communication to consistently advance future research in strategic communication and beyond. The use of uniform terminology adds clarity, simplifies comparability, and heightens the discussion quality of results leading to meaningful theoretical, methodological, and empirical contributions.
Since the automation trend is increasingly taking hold of the practice of strategic communication (Wiesenberg et al., Citation2017), this article also adds a significant aspect to Zerfass et al.’s (Citation2018) definition of the field. While they mention various examples of organisations and entities that communicate strategically, non-human actors – such as AI agents – had not yet been acknowledged. AI agents, however, are involved in a magnitude of strategic communication aspects, such as decision-making, relationship management, and message development. Thus, they affect various strategic communication contexts, such as management, marketing, and public relations.
The need for a unified theoretical framework for automated communication
Current research on automated communication is fragmented with regard to theoretical frameworks. If applied at all, the theoretical approaches are quite pluralistic suggesting a varied interest in the emerging topic; however, it also shows that no overarching theoretical framework exists yet. Thus, a guiding question is how far automated communication needs a completely new theoretical paradigm to provide a common ground for shared understanding and further future research in strategic communication and beyond.
Initially, scholars built upon conceptual frameworks such as Computer-Mediated Communication (e.g., Edwards et al., Citation2014). However, as it does not account for technology taking on the role of an active participant rather than being a passive tool (Gunkel, Citation2012), a paradigm shift towards Human-Machine Communication has been recommended (Mou & Xu, Citation2017); an approach that is progressively adopted (e.g., Guzman & Lewis, Citation2020; Lewis et al., Citation2019). Simultaneously, the Artificial Intelligence-Mediated Communication (Hancock et al., Citation2020) and Human-AI Interaction (Sundar, Citation2020) frameworks have been introduced. Nevertheless, only the Human-Machine Communication paradigm is seldom referenced in the sampled articles (e.g., Mou & Xu, Citation2017; Schapals & Porlezza, Citation2020). Hence, first, in extant research, different variations of theoretical frameworks are being established; however, seemingly independent from one another. Second, these overarching paradigms have not been applied in empirical research yet.
Instead, a cluster of studies has applied the Computers Are Social Actors framework that purports that humans interact with technology the same way they interact with humans, even though cognisant of the differences (Gambino et al., Citation2020). With the trend of anthropomorphisation of AI applications (Coeckelbergh, Citation2022), such a theoretical perspective helps to study micro-level effects. A look at the diversity of applied approaches offers inspiration for alternative perspectives:
At a meso-level, stakeholder and network theory, particularly with a focus on non-human actors (Latour, Citation2005), is fruitful. The move towards Human-Machine Communication highlights that AI agents are ascribed a more equal standing in relationships – also in organisational settings. This perspective has been somewhat discussed on a theoretical level with regard to public relations (Somerville, Citation2021; Verhoeven, Citation2018) but rarely used empirically (Schölzel & Nothhaft, Citation2016). Therefore, to give insights into how much agency AI agents have and how this may impact strategic decisions, actions, and outcomes, a better understanding of the balance of power and the dependencies between human and non-human actors in an organisational context is still needed.
At a macro-level, complex adaptive systems theory (Lock, Citation2023) and updated conceptions of the public sphere (Jungherr & Schroeder, Citation2023) can inform the study of automated strategic communication. Algorithms in themselves form complex systems, often referred to as black boxes (Pasquale, Citation2015). Studying their involvement in communication requires a holistic perspective to account “for the dynamic interaction between individual, organisational, and system levels in a digital communication environment” which further heightens the intricacies of the strategic communication process (Lock, Citation2023, p. 1). The opacity of algorithms, moreover, compromises essential components of the public sphere, namely transparency and availability of information. AI agents are susceptible to algorithmic bias (Kordzadeh & Ghasemaghaei, Citation2022) and contribute to the filter bubble phenomenon (Cho et al., Citation2023); both may hinder an informed public discourse and skew the input and output of strategic communication.
The need for a re-orientation of the professional profile of strategic communication
With the advent of automated communication, a question particularly relevant to strategic communication is what relationship management has to look like in such digital spheres (Lock, Citation2019). The relationship with existing stakeholders is adapting; chatbots, voice assistants, and AI influencers may be the first point of contact that (new) customers interact with (Huang et al., Citation2022). Strategic communication can build on existing marketing research regarding the effects that AI agents have on consumers’ perceptions of the product and company, and expand this to organisations and social judgments (Jacobs & Liebrecht, Citation2023). Furthermore, findings from research on AI influencers have the potential to guide future studies on corporate influencers (Borchers, Citation2019).
Due to this delegation of tasks, the responsibilities of strategic communication professionals are changing. Heide et al. (Citation2018) mention a lack of studies explicitly on communication professionals and their understanding of their role and work in strategic communication. In this vein, studying the role conceptions of strategic communication professionals in the age of AI is a sensible next step. Moreover, as the relationship towards automated communication phenomena is still negotiated in organisations (Dörr & Hollnbuchner, Citation2017), understanding the role conceptions of AI agents in relation to strategic communication professionals (e.g., concerning the degree of collaboration between humans and AI) is just as relevant.
In addition, strategic communication professionals are dependent on programmers for the provision and maintenance of the necessary software and hardware. Thus, basic knowledge of programming (Danzon-Chambaud & Cornia, Citation2023) and big data (Wiesenberg et al., Citation2017) as well as some training in the use of automated communication (López Jiménez & Ouariachi, Citation2021) would offer them more control and autonomy. However, the different competency levels may lead to a divide between tech-savvy and more traditionally trained communication professionals increasing tension within the field.
The need for understanding the ethical implications of AI on (strategic) communication
Automated communication comes with a myriad of ethical questions (Illia et al., Citation2023) that also touch upon normative directions of strategic communication research and practice. Scepticism towards AI technologies is fuelled by (falsely) emphasising their futuristic capabilities (Johnson & Verdicchio, Citation2017) in academic research, media reports, and organisational publications; especially concerning how much agency, autonomy, and ultimately, control they truly have. Building upon Gunkel’s (Citation2012) ideas, a general line of inquiry to calming public freight and strengthening public acceptance focuses on the key question of when an AI agent turns from a tool to an autonomous agent and what its limits are. Related to this is organisations’ conduct regarding the use of and communication about AI agents; more in-depth studies on “machinewashing” (Seele & Schultz, Citation2022, p. 1063) seem appropriate. Moreover, similar to considerations in journalism, the ethical question arises of whether the use of and authorship by AI agents need to be declared and how (Montal & Reich, Citation2017). Another one concerns the unanswered question of corporate responsibility and how far automated strategic communication by organisations contributes to societal values in the sense of “shared strategic communication” (Lock et al., Citation2016, p. 87).
Limitations
To be able to provide a comprehensive and detailed overview of how automated communication is conceptualised, analysed, and reflected upon, the decision was made to exclusively focus on peer-reviewed academic research. Nevertheless, grey literature, conference proceedings, book chapters, and the like certainly present other valuable insights worth studying.
It was ensured that the articles were of high quality; the quality assessment is not part of this article, though, as the criteria for conceptual and empirical articles were not of equal importance and, therefore, not easily grouped into broader categories.
Another limitation is the disregard of recommender systems which were not part of the search string and are only considered in the discussion. However, this, to the best of our knowledge, is the first article to look at several phenomena of automated communication and combine findings from these different subjects. Thus, making it useful to gain entry into the topic and field.
Conclusion
The consequences of organisations’ use of automated communication on the perception of professionalism and control in the management of strategic communication is a conundrum that deserves attention by both researchers and practitioners. Boundary setting here is essential to uncover how AI agents and humans can best collaborate (e.g., delegation, supervision, replacement) and to clearly show the limits that AI has. Of interest, especially with regard to strategic communication, are process theories that map and explain the automated communication process in and by organisations holistically, i.e., its causes, contents, and consequences. Analysing the effects that automated communication has on stakeholders is indispensable given the expected prevalence of this technology. A question that arises is whether the definition of digital communicative organisation-stakeholder relationships “where all parties are aware of the communicative act” (Lock, Citation2019, p. 9) holds if one or both parties are AI agents and the message is automated.
Acknowledgements
The authors would like to thank the second coder of this study, Maria Tsuker, for her diligent and meticulous coding. Furthermore, they acknowledge support by the German Research Foundation project number 512648189 and the Open Access Publication Fund of the Thueringer Universitaets- und Landesbibliothek Jena.
Disclosure statement
No potential conflict of interest was reported by the author(s).
References
- Berlo, D. K. (1960). The process of communication. An introduction to theory and practice. Holt, Rinehart and Winston.
- Blut, M., Wang, C., Wünderlich, N. V., & Brock, C. (2021). Understanding anthropomorphism in service provision: A meta-analysis of physical robots, chatbots, and other AI. Journal of the Academy of Marketing Science, 49(4), 632–658. https://doi.org/10.1007/s11747-020-00762-y
- Booth, A., Sutton, A., Clowes, M., & Martyn-St James, M. (2022). Systematic approaches to a successful literature review. (3rd ed.). SAGE.
- Borchers, N. S. (2019). Social media influencers in strategic communication. International Journal of Strategic Communication, 13(4), 255–260. https://doi.org/10.1080/1553118X.2019.1634075
- Braun, V., & Clarke, V. (2006). Using thematic analysis in psychology. Qualitative Research in Psychology, 3(2), 77–101. https://doi.org/10.1191/1478088706qp063oa
- Braun, V., & Clarke, V. (2021). One size fits all? What counts as quality practice in (reflexive) thematic analysis? Qualitative Research in Psychology, 18(3), 328–352. https://doi.org/10.1080/14780887.2020.1769238
- Braun, V., Clarke, V., Hayfield, N., & Terry, G. (2019). Thematic analysis. In P. Liamputtong (Ed.), Handbook of research methods in health social sciences (pp. 843–860). Springer. https://doi.org/10.1007/978-981-10-5251-4_103
- Budhwar, P., Malik, A., Silva de, M., & Thevisuthan, P. (2022). Artificial intelligence - challenges and opportunities for international HRM: A review and research agenda. The International Journal of Human Resource Management, 33(6), 1065–1097. https://doi.org/10.1080/09585192.2022.2035161
- Burgoon, J. K. (1978). A communication model of personal space violations: Explication and an initial test. Human Communication Research, 4(2), 129–142. https://doi.org/10.1111/j.1468-2958.1978.tb00603.x
- Burgoon, J. K., & Jones, S. B. (1976). Toward a theory of personal space expectations and their violations. Human Communication Research, 2(2), 131–146. https://doi.org/10.1111/j.1468-2958.1976.tb00706.x
- Byun, K. J., & Ahn, S. J. (2023). A systematic review of virtual influencers: Similarities and differences between human and virtual influencers in interactive advertising. Journal of Interactive Advertising, 23(4), 293–306. https://doi.org/10.1080/15252019.2023.2236102
- Chaffee, S. H. (1991). Explication. SAGE Publications.
- Chesney, B., & Citron, D. (2019). Deep fakes: A looming challenge for privacy, democracy, and national security. California Law Review, 107(6), 1753–1820. https://doi.org/10.2139/ssrn.3213954
- Cho, H., Lee, D., & Lee, J. G. (2023). User acceptance on content optimization algorithms: Predicting filter bubbles in conversational AI services. Universal Access in the Information Society, 22(4), 1325–1338. https://doi.org/10.1007/s10209-022-00913-8
- Coeckelbergh, M. (2022). Three responses to anthropomorphism in social robotics: Towards a critical, relational, and hermeneutic approach. International Journal of Social Robotics, 14(10), 2049–2061. https://doi.org/10.1007/s12369-021-00770-0
- Danzon-Chambaud, S. (2021). A systematic review of automated journalism scholarship: Guidelines and suggestions for future research [version 1; peer review: 2 approved]. Open Research Europe, 1(4), Article 4, 4–19. https://doi.org/10.12688/openreseurope.13096.1
- Da Silva Oliveira, A. B., & Chimenti, P. (2021). “Humanized robots”: A proposition of categories to understand virtual influencers. Australasian Journal of Information Systems, 25, 1–27. https://doi.org/10.3127/ajis.v25i0.3223
- Davis, F. D. (1989). Perceived usefulness, perceived ease of use, and user acceptance of information technology. MIS Quarterly, 13(3), 319–340. https://doi.org/10.2307/249008
- Davis, J., Mengersen, K., Bennett, S., & Mazerolle, L. (2014). Viewing systematic reviews and meta-analysis in social research through different lenses. Springer Plus, 3(1), 1–9. https://doi.org/10.1186/2193-1801-3-511
- Dehnert, M., & Mongeau, P. A. (2022). Persuasion in the age of artificial intelligence (AI): Theories and complications of AI-based persuasion. Human Communication Research, 48(3), 386–403. https://doi.org/10.1093/hcr/hqac006
- Dörr, K. N. (2016). Mapping the field of algorithmic journalism. Digital Journalism, 4(6), 700–722. https://doi.org/10.1080/21670811.2015.1096748
- Esposito, E. (2022). Artificial communication: How algorithms produce social intelligence. The MIT Press.
- Ferrara, E., Varol, O., Davis, C., Menczer, F., & Flammini, A. (2016). The rise of social bots. Communications of the ACM, 59(7), 96–104. https://doi.org/10.1145/2818717
- Galloway, C., & Swiatek, L. (2018). Public relations and artificial intelligence: It’s not (just) about robots. Public Relations Review, 44(5), 734–740. https://doi.org/10.1016/j.pubrev.2018.10.008
- Gambino, A., Fox, J., & Ratan, R. A. (2020). Building a stronger CASA: Extending the computers are social actors paradigm. Human-Machine Communication, 1(1), 71–86. https://doi.org/10.30658/hmc.1.5
- García-Orosa, B., Canavilhas, J., & Vázquez-Herrero, J. (2023). Algoritmos y comunicación: Revisión sistematizada de la literatura: Algorithms and communication: A systematized literature review. Comunicar, 31(74), 9–21. https://doi.org/10.3916/C74-2023-01
- Geise, S., Klinger, U., Magin, M., Müller, K. F., Nitsch, C., Riesmeyer, C., Rothenberger, L., Schumann, C., Sehl, A., Wallner, C., & Zillich, A. F. (2022). The normativity of communication research: A content analysis of normative claims in peer-reviewed journal articles (1970–2014). Mass Communication & Society, 25(4), 528–553. https://doi.org/10.1080/15205436.2021.1987474
- Graefe, A. (2016). Guide to automated journalism. Tow Center for Digital Journalism, Columbia University. https://doi.org/10.7916/D80G3XDJ
- Graefe, A., & Bohlken, N. (2020). Automated journalism: A meta-analysis of readers’ perceptions of human-written in comparison to automated news. Media and Communication, 8(3), 50–59. https://doi.org/10.17645/mac.v8i3.3019
- Gunkel, D. J. (2012). Communication and artificial intelligence: Opportunities and challenges for the 21st century. Communication+1, 1(1), 1–26. https://doi.org/10.7275/R5QJ7F7R
- Guzman, A. L., & Lewis, S. C. (2020). Artificial intelligence and communication: A human–machine communication research agenda. New Media & Society, 22(1), 70–86. https://doi.org/10.1177/1461444819858691
- Hancock, J. T., Naaman, M., & Levy, K. (2020). AI-mediated communication: Definition, research agenda, and ethical considerations. Journal of Computer-Mediated Communication, 25(1), 89–100. https://doi.org/10.1093/jcmc/zmz022
- Heide, M., Platen von, S., Simonsson, C., & Falkheimer, J. (2018). Expanding the scope of strategic communication: Towards a holistic understanding of organizational complexity. International Journal of Strategic Communication, 12(4), 452–468. https://doi.org/10.1080/1553118X.2018.1456434
- Hepp, A., Loosen, W., Dreyer, S., Jarke, J., Kannengießer, S., Katzenbach, C., Malaka, R., Pfadenhauer, M., Puschmann, C., & Schulz, W. (2023). ChatGPT, LaMDA, and the hype around communicative AI: The automation of communication as a field of research in media and communication studies. Human-Machine Communication, 6, 41–63. https://doi.org/10.30658/hmc.6.4
- Hoy, M. B. (2018). Alexa, Siri, Cortana, and more: An introduction to voice assistants. Medical Reference Services Quarterly, 37(1), 81–88. https://doi.org/10.1080/02763869.2018.1404391
- Hwang, G. J., & Chang, C. Y. (2023). A review of opportunities and challenges of chatbots in education. Interactive Learning Environments, 31(7), 4099–4112. https://doi.org/10.1080/10494820.2021.1952615
- Jacobs, S., & Liebrecht, C. (2023). Responding to online complaints in webcare by public organizations: The impact on continuance intention and reputation. Journal of Communication Management, 27(1), 1–20. https://doi.org/10.1108/JCOM-11-2021-0132
- Jenneboer, L., Herrando, C., & Constantinides, E. (2022). The impact of chatbots on customer loyalty: A systematic literature review. Journal of Theoretical & Applied Electronic Commerce Research, 17(1), 212–229. https://doi.org/10.3390/jtaer17010011
- Johnson, D. G., & Verdicchio, M. (2017). AI anxiety. Journal of the Association for Information Science and Technology, 68(9), 2267–2270. https://doi.org/10.1002/asi.23867
- Kalpokas, I. (2021). Problematising reality: The promises and perils of synthetic media. SN Social Sciences, 1(1), 1–11. https://doi.org/10.1007/s43545-020-00010-8
- Katz, E., Blumler, J. G., & Gurevitch, M. (1973). Uses and gratifications research. Public Opinion Quarterly, 37(4), 509–523. https://doi.org/10.1086/268109
- Keller, K. L. (2001). Mastering the marketing communications mix: Micro and macro perspectives on integrated marketing communication programs. Journal of Marketing Management, 17(7–8), 819–847. https://doi.org/10.1362/026725701323366836
- Kietzmann, J., Mills, A. J., & Plangger, K. (2021). Deepfakes: Perspectives on the future “reality” of advertising and branding. International Journal of Advertising, 40(3), 473–485. https://doi.org/10.1080/02650487.2020.1834211
- Klaus, P., & Zaichkowsky, J. (2020). AI voice bots: A services marketing research agenda. The Journal of Services Marketing, 34(3), 389–398. https://doi.org/10.1108/JSM-01-2019-0043
- Kordzadeh, N., & Ghasemaghaei, M. (2022). Algorithmic bias: Review, synthesis, and future research directions. European Journal of Information Systems, 31(3), 388–409. https://doi.org/10.1080/0960085X.2021.1927212
- Krippendorff, K. (2004). Content analysis: An introduction to its methodology (2nd ed.). SAGE Publications.
- Laszkiewicz, A., & Kalinska-Kula, M. (2023). Virtual influencers as an emerging marketing theory: A systematic literature review. International Journal of Consumer Studies, 47(6), 2479–2494. https://doi.org/10.1111/ijcs.12956
- Latour, B. (2005). Reassembling the social: An introduction to actor-network theory. Oxford University Press.
- Lebeuf, C., Zagalsky, A., Foucault, M., & Storey, M. A. (2019). Defining and classifying software bots: A faceted taxonomy. Proceedings of the 2019 IEEE/ACM 1st International Workshop on Bots in Software Engineering (pp. 1–6). IEEE. https://doi.org/10.1109/BotSE.2019.00008
- Lewis, S. C., Guzman, A. L., & Schmidt, T. R. (2019). Automation, journalism, and human-machine communication: Rethinking roles and relationships of humans and machines in news. Digital Journalism, 7(4), 409–427. https://doi.org/10.1080/21670811.2019.1577147
- Lim, W. M., Gunasekara, A., Pallant, J. L., Pallant, J. I., & Pechenkina, E. (2023). Generative AI and the future of education: Ragnarök or reformation? A paradoxical perspective from management educators. International Journal of Management Education, 21(2), Article 100790, 1–13. https://doi.org/10.1016/j.ijme.2023.100790
- Lim, W. M., Kumar, S., Verma, S., & Chaturvedi, R. (2022). Alexa, what do we know about conversational commerce? Insights from a systematic literature review. Psychology & Marketing, 39(6), 1129–1155. https://doi.org/10.1002/mar.21654
- Ling, E. C., Tussyadiah, I., Tuomi, A., & Stienmetz, J. (2021). Factors influencing users’ adoption and use of conversational agents: A systematic review. Psychology & Marketing, 38(7), 1021–1051. https://doi.org/10.1002/mar.21491
- Lock, I. (2019). Explicating communicative organization-stakeholder relationships in the digital age: A systematic review and research agenda. Public Relations Review, 45(4), 1–13. https://doi.org/10.1016/j.pubrev.2019.101829
- Lock, I. (2023). Conserving complexity: A complex systems paradigm and framework to study public relations’ contribution to grand challenges. Public Relations Review, 49(2), 1–11. https://doi.org/10.1016/j.pubrev.2023.102310 2
- Lock, I., & Giani, S. (2021). Finding more needles in more haystacks: Rigorous literature searching for systematic reviews and meta-analyses in management and organization studies. https://doi.org/10.31235/osf.io/8ek6h
- Lock, I., Seele, P., & Heath, R. L. (2016). Where grass has no roots: The concept of ‘shared strategic communication’ as an answer to unethical astroturf lobbying. International Journal of Strategic Communication, 10(2), 87–100. https://doi.org/10.1080/1553118X.2015.1116002
- Lock, I., Wonneberger, A., Verhoeven, P., & Hellsten, I. (2020). Back to the roots? The applications of communication science theories in strategic communication research. International Journal of Strategic Communication, 14(1), 1–24. https://doi.org/10.1080/1553118X.2019.1666398
- Löffler, N., Röttger, U., & Wiencierz, C. (2021). Data-based strategic communication as a mediator of trust: Recipients’ perception of an NPO’s automated posts. In B. Blöbaum (Ed.), Trust and communication (pp. 115–134). Springer. https://doi.org/10.1007/978-3-030-72945-5_6
- López Jiménez, E. A., & Ouariachi, T. (2021). An exploration of the impact of artificial intelligence (AI) and automation for communication professionals. Journal of Information, Communication and Ethics in Society, 19(2), 249–267. https://doi.org/10.1108/JICES-03-2020-0034
- Mori, M. (1970). Bukimi no tani [The uncanny valley]. Energy, 7, 33–35. https://cir.nii.ac.jp/crid/1370013168736887425
- Nass, C., & Moon, Y. (2000). Machines and mindlessness: Social responses to computers. The Journal of Social Issues, 56(1), 81–103. https://doi.org/10.1111/0022-4537.00153
- Natale, S. (2021). Communicating through or communicating with: Approaching artificial intelligence from a communication and media studies perspective. Communication Theory, 31(4), 905–910. https://doi.org/10.1093/ct/qtaa022
- Page, M. J., McKenzie, J. E., Bossuyt, P. M., Boutron, I., Hoffmann, T. C., Mulrow, C. D., Shamseer, L., Tetzlaff, J. M., Akl, E. A., Brennan, S. E., Chou, R., Glanville, J., Grimshaw, J. M., Hróbjartsson, A., Lalu, M. M., Li, T., Loder, E. W., Mayo-Wilson, E., McDonald, S. , and Mohler, D. (2021). The PRISMA 2020 statement: An updated guideline for reporting systematic reviews. Systematic Reviews, 10(1). https://doi.org/10.1186/s13643-021-01626-4
- Pasquale, F. (2015). The black box society: The secret algorithms that control money and information. Harvard University Press.
- Pawelec, M. (2022). Deepfakes and democracy (theory): How synthetic audio-visual media for disinformation and hate speech threaten core democratic functions. Digital Society, 1(2), 1–37. https://doi.org/10.1007/s44206-022-00010-6
- Primo, A., & Zago, G. (2015). Who and what do journalism? An actor-network perspective. Digital Journalism, 3(1), 38–52. https://doi.org/10.1080/21670811.2014.927987
- Reeves, B., & Nass, C. (1996). The media equation: How people treat computers, television, and new media like real people and places. Cambridge University Press.
- Riffe, D., Lacy, S., & Fico, F. (2014). Analyzing media messages: Using quantitative content analysis in research (3rd ed.). Routledge.
- Roy, D., & Dutta, M. (2022). A systematic review and research perspective on recommender systems. Journal of Big Data, 9(1), 1–36. https://doi.org/10.1186/s40537-022-00592-5
- Schölzel, H., & Nothhaft, H. (2016). The establishment of facts in public discourse: Actor-network-theory as a methodological approach in PR-research. Public Relations Inquiry, 5(1), 53–69. https://doi.org/10.1177/2046147X15625711
- Seele, P., & Schultz, M. D. (2022). From greenwashing to machinewashing: A model and future directions derived from reasoning by analogy. Journal of Business Ethics, 178(4), 1063–1089. https://doi.org/10.1007/s10551-022-05054-9
- Shawar, B. A., & Atwell, E. (2007). Chatbots: Are they really useful? Journal for Language Technology and Computational Linguistics, 22(1), 29–49. https://doi.org/10.21248/jlcl.22.2007.88
- Siitonen, M., Laajalahti, A., & Venäläinen, P. (2024). Mapping automation in journalism studies 2010–2019: A literature review. Journalism Studies, 25(3), 299–318. https://doi.org/10.1080/1461670X.2023.2296034
- Snyder, H. (2019). Literature review as a research methodology: An overview and guidelines. Journal of Business Research, 104(1), 333–339. https://doi.org/10.1016/j.jbusres.2019.07.039
- Somerville, I. (2021). Public relations and actor-network-theory. In C. Valentini (Ed.), Public relations (pp. 525–540). De Gruyter Mouton. https://doi.org/10.1515/9783110554250-027
- Stenbom, A., Wiggberg, M., & Norlund, T. (2023). Exploring communicative AI: Reflections from a Swedish newsroom. Digital Journalism, 11(9), 1622–1640. https://doi.org/10.1080/21670811.2021.2007781
- Sundar, S. S. (2008). The MAIN model: A heuristic approach to understanding technology effects on credibility. In M. J. Metzger & A. J. Flanagin (Eds.), Digital media, youth, and credibility (pp. 73–100). The MIT Press. https://doi.org/10.1162/dmal.9780262562324.073
- Sundar, S. S. (2020). Rise of machine agency: A framework for studying the psychology of human–AI interaction (HAII). Journal of Computer-Mediated Communication, 25(1), 74–88. https://doi.org/10.1093/jcmc/zmz026
- Syvänen, S., & Valentini, C. (2020). Conversational agents in online organization–stakeholder interactions: A state-of-the-art analysis and implications for further research. Journal of Communication Management, 24(4), 339–362. https://doi.org/10.1108/JCOM-11-2019-0145
- Vagia, M., Transeth, A. A., & Fjerdingen, S. A. (2016). A literature review on the levels of automation during the years. What are the different taxonomies that have been proposed? Applied Ergonomics, 53, 190–202. https://doi.org/10.1016/j.apergo.2015.09.013
- van der Kaa, H., & Krahmer, E. J. (2014). Journalist versus news consumer: The perceived credibility of machine written news. In Computation + Journalism Symposium 2014, New York, USA.
- van Pinxteren, M., Pluymaekers, M., & Lemmink, J. (2020). Human-like communication in conversational agents: A literature review and research agenda. Journal of Service Management, 31(2), 203–225. https://doi.org/10.1108/JOSM-06-2019-0175
- Vasist, P. N., & Krishnan, S. (2022). Deepfakes: An integrative review of the literature and an agenda for future research. Communications of the Association for Information Systems, 51(1), Article 14, 556–602. https://doi.org/10.17705/1CAIS.05126
- Verhoeven, P. (2018). On Latour: Actor-networks, modes of existence and public relations. In Ø. Ihlen & M. Fredriksson (Eds.), Public relations and social theory (2nd ed., pp. 99–116). Routledge.
- Westerlund, M. (2019). The emergence of deepfake technology: A review. Technology Innovation Management Review, 9(11), 39–52. https://doi.org/10.22215/timreview/1282
- Wiesenberg, M., Zerfass, A., & Moreno, A. (2017). Big data and automation in strategic communication. International Journal of Strategic Communication, 11(2), 95–114. https://doi.org/10.1080/1553118X.2017.1285770
- Wu, S., Wong, P. W., Tandoc, E. C., & Salmon, C. T. (2023). Reconfiguring human-machine relations in the automation age: An actor-network analysis on automation’s takeover of the advertising media planning industry. Journal of Business Research, 168, 1–8. https://doi.org/10.1016/j.jbusres.2023.114234
- Zerfass, A., Verçiç, D., Nothaft, H., & Werder, K. P. (2018). Strategic communication: Defining the field and its contribution to research and practice. International Journal of Strategic Communication, 12(4), 487–505. https://doi.org/10.1080/1553118X.2018.1493485
Articles included in the systematic review
- Ahmad, N., Haque, S., & Ibahrine, M. (2023). The news ecosystem in the age of AI: Evidence from the UAE. Journal of Broadcasting & Electronic Media, 67(3), 323–352. https://doi.org/10.1080/08838151.2023.2173197
- Ahmed, S. (2023). Navigating the maze: Deepfakes, cognitive ability, and social media news skepticism. New Media & Society, 25(5), 1108–1129. https://doi.org/10.1177/14614448211019198
- Alboqami, H. (2023). Trust me, I’m an influencer! Causal recipes for customer trust in artificial intelligence influencers in the retail industry. Journal of Retailing and Consumer Service, 72, Article 103242, 1–12. https://doi.org/10.1016/j.jretconser.2022.103242
- Arango, L., Singaraju, S. P., & Niininen, O. (2023). Consumer responses to AI-generated charitable giving ads. Journal of Advertising, 52(4), 486–503. https://doi.org/10.1080/00913367.2023.2183285
- Arsenyan, J., & Mirowska, A. (2021). Almost human? A comparative case study on the social media presence of virtual influencers. International Journal of Human-Computer Studies, 155, Article 102694, 1–16. https://doi.org/10.1016/j.ijhcs.2021.102694
- (Articles are referenced according to how they appeared at the time of the analysis. Since the analysis, some articles have been published offline as well; hence, the publication year may differ).
- Campbell, C., Plangger, K., Sands, S., Kietzmann, J., & Bates, K. (2022). How deepfakes and artificial intelligence could reshape the advertising industry. Journal of Advertising Research, 62(4), 241–251. https://doi.org/10.2501/JAR-2022-017
- Carlson, M. (2015). The robotic reporter: Automated journalism and the redefinition of labor, compositional forms, and journalistic authority. Digital Journalism, 3(3), 416–431. https://doi.org/10.1080/21670811.2014.976412
- Chandra, S., Shirish, A., & Srivastava, S. C. (2022). To be or not to be … human? Theorizing the role of human-like competencies in conversational artificial intelligence agents. Journal of Management Information Systems, 39(4), 969–1005. https://doi.org/10.1080/07421222.2022.2127441
- Cheng, Y., & Jiang, H. (2020). How do AI-driven chatbots impact user experience? Examining gratifications, perceived privacy risk, satisfaction, loyalty, and continued use. Journal of Broadcasting & Electronic Media, 64(4), 592–614. https://doi.org/10.1080/08838151.2020.1834296
- Clerwall, C. (2014). Enter the robot journalist: Users’ perceptions of automated content. Journalism Practice, 8(5), 519–531. https://doi.org/10.1080/17512786.2014.883116
- Cloudy, J., Banks, J., & Bowman, N. D. (2021). The str(AI)ght scoop: Artificial intelligence cues reduce perceptions of hostile media bias. Digital Journalism, 1–20. https://doi.org/10.1080/21670811.2021.1969974
- Cover, R. (2022). Deepfake culture: The emergence of audio-video deception as an object of social anxiety and regulation. Continuum: Journal of Media & Cultural Studies, 36(4), 609–621. https://doi.org/10.1080/10304312.2022.2084039
- Danzon-Chambaud, S., & Cornia, A. (2023). Changing or reinforcing the “rules of the game”: A field theory perspective on the impacts of automated journalism on media practitioners. Journalism Practice, 17(2), 174–188. https://doi.org/10.1080/17512786.2021.1919179
- Diakopoulos, N., & Johnson, D. (2021). Anticipating and addressing the ethical implications of deepfakes in the context of elections. New Media & Society, 23(7), 2072–2098. https://doi.org/10.1177/1461444820925811
- Dobber, T., Metoui, N., Trilling, D., Helberger, N., & de Vreese, C. (2021). Do (microtargeted) deepfakes have real effects on political attitudes? The International Journal of Press/Politics, 26(1), 69–91. https://doi.org/10.1177/1940161220944364
- Dörr, K. N., & Hollnbuchner, K. (2017). Ethical challenges of algorithmic journalism. Digital Journalism, 5(4), 404–419. https://doi.org/10.1080/21670811.2016.1167612
- Edwards, C., Edwards, A., Spence, P. R., & Shelton, A. K. (2014). Is that a bot running the social media feed? Testing the differences in perceptions of communication quality for a human agent and a bot agent on Twitter. Computers in Human Behavior, 33, 372–376. https://doi.org/10.1016/j.chb.2013.08.013
- Flavián, C., Akdim, K., & Casaló, L. V. (2023). Effects of voice assistant recommendations on consumer behavior. Psychology & Marketing, 40(2), 328–346. https://doi.org/10.1002/mar.21765
- Franke, C., Groeppel-Klein, A., & Müller, K. (2023). Consumers’ responses to virtual influencers as advertising endorsers: Novel and effective or uncanny and deceiving? Journal of Advertising, 52(4), 523–539. https://doi.org/10.1080/00913367.2022.2154721
- Garvey, A. M., Kim, T. W., & Duhachek, A. (2023). Bad news? Send an AI. Good news? Send a human. Journal of Marketing, 87(1), 10–25. https://doi.org/10.1177/00222429211066972
- Graefe, A., Haim, M., Haarmann, B., & Brosius, H. B. (2018). Readers’ perception of computer-generated news: Credibility, expertise, and readability. Journalism, 19(5), 595–610. https://doi.org/10.1177/1464884916641269
- Haim, M., & Graefe, A. (2017). Automated news. Better than expected? Digital Journalism, 5(8), 1044–1059. https://doi.org/10.1080/21670811.2017.1345643
- Ham, J., Li, S., Shah, P., & Eastin, M. S. (2023). The “mixed” reality of virtual brand endorsers: Understanding the effect of brand engagement and social cues on technological perceptions and advertising effectiveness. Journal of Interactive Advertising, 23(2), 98–113. https://doi.org/10.1080/15252019.2023.2185557
- Hameleers, M., van der Meer, T., & Dobber, T. (2022). You won’t believe what they just said! The effects of political deepfakes embedded as vox populi on social media. Social Media + Society, 8(3), 1–12. https://doi.org/10.1177/20563051221116346
- Hepp, A. (2020). Artificial companions, social bots and work bots: Communicative robots as research objects of media and communication studies. Media Culture & Society, 42(7–8), 1410–1426. https://doi.org/10.1177/0163443720916412
- Ho, A., Hancock, J., & Miner, A. S. (2018). Psychological, relational, and emotional effects of self-disclosure after conversations with a chatbot. Journal of Communication, 68(4), 712–733. https://doi.org/10.1093/joc/jqy026
- Huang, R., Kim, M., & Lennon, S. (2022). Trust as a second-order construct: Investigating the relationship between consumers and virtual agents. Telematics and Informatics, 70, Article 101811, 1–12. https://doi.org/10.1016/j.tele.2022.101811
- Illia, L., Colleoni, E., & Zyglidopoulos, S. (2023). Ethical implications of text generation in the age of artificial intelligence. Business Ethics, the Environment & Responsibility, 32(1), 201–210. https://doi.org/10.1111/beer.12479
- Jamil, S. (2021). Automated journalism and the freedom of media: Understanding legal and ethical implications in competitive authoritarian regime. Journalism Practice, 1–24. https://doi.org/10.1080/17512786.2021.1981148
- Jang, W., Chun, J. W., Kim, S., & Kang, Y. W. (2023). The effects of anthropomorphism on how people evaluate algorithm-written news. Digital Journalism, 11(1), 103–124. https://doi.org/10.1080/21670811.2021.1976064
- Jia, C. (2020). Chinese automated journalism: A comparison between expectations and perceived quality. International Journal of Communication, 14, 2611–2632. https://ijoc.org/index.php/ijoc/article/view/13334/3083
- Jia, C., & Johnson, T. J. (2021). Source credibility matters: Does automated journalism inspire selective exposure? International Journal of Communication, 15, 3760–3781. https://ijoc.org/index.php/ijoc/article/view/16546
- Jung, J., Song, H., Kim, Y., Im, H., & Oh, S. (2017). Intrusion of software robots into journalism: The public’s and journalists’ perceptions of news written by algorithms and human journalists. Computers in Human Behavior, 71, 291–298. https://doi.org/10.1016/j.chb.2017.02.022
- Jungherr, A., & Schroeder, R. (2023). Artificial intelligence and the public arena. Communication Theory, 33(2–3), 164–173. https://doi.org/10.1093/ct/qtad006
- Keller, T. R., & Klinger, U. (2019). Social bots in election campaigns: Theoretical, empirical, and methodological implications. Political Communication, 36(1), 171–189. https://doi.org/10.1080/10584609.2018.1526238
- Kreps, S., McCain, R. M., & Brundage, M. (2022). All the news that’s fit to fabricate: AI-generated text as a tool of media misinformation. Journal of Experimental Political Science, 9(1), 104–117. https://doi.org/10.1017/XPS.2020.37
- Lee, Y. A., Huang, K. T., Blom, R., Schriner, R., & Ciccarelli, C. A. (2021). To believe or not to believe: Framing analysis of content and audience response on top 10 deepfake videos on YouTube. Cyberpsychology, Behavior and Social Networking, 24(3), 1–6. https://doi.org/10.1089/cyber.2020.0176
- Lermann Henestrosa, A., Greving, H., & Kimmerle, J. (2023). Automated journalism: The effects of AI authorship and evaluative information on the perception of a science journalism article. Computers in Human Behavior, 138, Article 107445, 1–11. https://doi.org/10.1016/j.chb.2022.107445
- Li, J., Kizilcec, R., Bailenson, J., & Ju, W. (2016). Social robots and virtual agents as lecturers for video instruction. Computers in Human Behavior, 55, 1222–1230. https://doi.org/10.1016/j.chb.2015.04.005
- Liu, B., & Wei, L. (2019). Machine authorship in situ. Digital Journalism, 7(5), 635–657. https://doi.org/10.1080/21670811.2018.1510740
- Lou, C., Kiew, S., Chen, T., Lee, T., Ong, J., & Phua, Z. (2022). Authentically fake? How consumers respond to the influence of virtual influencers. Journal of Advertising, 1–18. https://doi.org/10.1080/00913367.2022.2149641
- Magno, F., & Dossena, G. (2022). The effects of chatbots’ attributes on customer relationships with brands: PLS-SEM and importance–performance map analysis. The TQM Journal, 1–14. https://doi.org/10.1108/TQM-02-2022-0080
- Mirowska, A., & Arsenyan, J. (2023). Sweet escape: The role of empathy in social media engagement with human versus virtual influencers. International Journal of Human-Computer Studies, 174, Article 103008, 1–18. https://doi.org/10.1016/j.ijhcs.2023.103008
- Mohd Rahim, N. I., Iahad, N. A., Yusof, A. F., & Al-Sharafi, M. A. (2022). AI-based chatbots adoption model for higher-education institutions: A hybrid PLS-SEM neural network modelling approach. Sustainability, 14(19), Article 12726, 1–22. https://doi.org/10.3390/su141912726
- Montal, T., & Reich, Z. (2017). I, robot. You, journalist. Who is the author? Authorship, bylines and full disclosure in automated journalism. Digital Journalism, 5(7), 829–849. https://doi.org/10.1080/21670811.2016.1209083
- Mou, Y., & Xu, K. (2017). The media inequality: Comparing the initial human-human and human-AI social interactions. Computers in Human Behavior, 72, 432–440. https://doi.org/10.1016/j.chb.2017.02.067
- Neo, M. (2022). The Merlin project: Malaysian students’ acceptance of an AI chatbot in their learning process. Turkish Online Journal of Distance Education, 23(3), 31–48. https://doi.org/10.17718/tojde.1137122
- Noain-Sánchez, A. (2022). Addressing the impact of artificial intelligence on journalism: The perception of experts, journalists and academics. Communication & Society, 35(3), 105–121. https://doi.org/10.15581/003.35.3.105-121
- Rajaobelina, L., Prom Tep, S., Arcand, M., & Ricard, L. (2021). Creepiness: Its antecedents and impact on loyalty when interacting with a chatbot. Psychology & Marketing, 38(12), 2339–2356. https://doi.org/10.1002/mar.21548
- Rese, A., Ganster, L., & Baier, D. (2020). Chatbots in retailers’ customer communication: How to measure their acceptance? Journal of Retailing and Consumer Service, 56, Article 102176, 1–14. https://doi.org/10.1016/j.jretconser.2020.102176
- Sánchez Gonzales, H. M., & Sánchez González, M. (2020). Conversational bots used in political news from the point of view of the user’s experience: Politibot. Communication & Society, 33(4), 155–168. https://doi.org/10.15581/003.33.4.155-168
- Sands, S., Campbell, C. L., Plangger, K., & Ferraro, C. (2022). Unreal influence: Leveraging AI in influencer marketing. European Journal of Marketing, 56(6), 1721–1747. https://doi.org/10.1108/EJM-12-2019-0949
- Sands, S., Campbell, C., Plangger, K., & Pitt, L. (2022). Buffer bots: The role of virtual service agents in mitigating negative effects when service fails. Psychology & Marketing, 39(11), 2039–2054. https://doi.org/10.1002/mar.21723
- Sands, S., Ferraro, C., Demsar, V., & Chandler, G. (2022). False idols: Unpacking the opportunities and challenges of falsity in the context of virtual influencers. Business Horizons, 65(6), 777–788. https://doi.org/10.1016/j.bushor.2022.08.002
- Schapals, A. K., & Porlezza, C. (2020). Assistance or resistance? Evaluating the intersection of automated journalism and journalistic role conceptions. Media and Communication, 8(3), 16–26. https://doi.org/10.17645/mac.v8i3.3054
- Schultz, B., & Sheffer, M. L. (2017). Newspaper trust and credibility in the age of robot reporters. Journal of Applied Journalism & Media Studies, 6(2), 339–355. https://doi.org/10.1386/ajms.6.2.339_1
- Söderlund, M., Oikarinen, E. L., & Tan, T. M. (2022). The hard-working virtual agent in the service encounter boosts customer satisfaction. The International Review of Retail, Distribution & Consumer Research, 32(4), 388–404. https://doi.org/10.1080/09593969.2022.2042715
- Tandoc, E. C., Jr., Yao, L. J., & Wu, S. (2020). Man vs. machine? The impact of algorithm authorship on news credibility. Digital Journalism, 8(4), 548–562. https://doi.org/10.1080/21670811.2020.1762102
- Thäsler-Kordonouri, S., & Barling, K. (2023). Automated journalism in UK local newsrooms: Attitudes, integration, impact. Journalism Practice, 1–18. https://doi.org/10.1080/17512786.2023.2184413
- Thomas, V. L., & Fowler, K. (2021). Close encounters of the AI kind: Use of AI influencers as brand endorsers. Journal of Advertising, 50(1), 11–25. https://doi.org/10.1080/00913367.2020.1810595
- Tsai, W. H., Liu, Y., & Chuan, C. H. (2021). How chatbots’ social presence communication enhances consumer engagement: The mediating role of parasocial interaction and dialogue. Journal of Research in Interactive Marketing, 15(3), 460–482. https://doi.org/10.1108/JRIM-12-2019-0200
- Túñez-López, J. M., Fieiras Ceide, C., & Vaz-Álvarez, M. (2021). Impact of artificial intelligence on journalism: Transformations in the company, products, contents and professional profile. Communication & Society, 34(1), 177–193. https://doi.org/10.15581/003.34.1.177-193
- van Dalen, A. (2012). The algorithms behind the headlines. How machine-written news redefines the core skills of human journalists. Journalism Practice, 6(5–6), 648–658. https://doi.org/10.1080/17512786.2012.667268
- van Pinxteren, M., Pluymaekers, M., Lemmink, J., & Krispin, A. (2023). Effects of communication style on relational outcomes in interactions between customers and embodied conversational agents. Psychology & Marketing, 40(5), 938–953. https://doi.org/10.1002/mar.21792
- Waddell, T. F. (2018). A robot wrote this? How perceived machine authorship affects news credibility. Digital Journalism, 6(2), 236–255. https://doi.org/10.1080/21670811.2017.1384319
- Waddell, T. F. (2019a). Attribution practices for the man-machine marriage: How perceived human intervention, automation metaphors, and byline location affect the perceived bias and credibility of purportedly automated content. Journalism Practice, 13(10), 1255–1272. https://doi.org/10.1080/17512786.2019.1585197
- Waddell, T. F. (2019b). Can an algorithm reduce the perceived bias of news? Testing the effect of machine attribution on news readers’ evaluations of bias, anthropomorphism, and credibility. Journalism & Mass Communication Quarterly, 96(1), 82–100. https://doi.org/10.1177/1077699018815891
- Wang, J., Li, S., Xue, K., & Chen, L. (2022). What is the competence boundary of algorithms? An institutional perspective on AI-based video generation? Displays, 73, Article 102240, 1–10. https://doi.org/10.1016/j.displa.2022.102240
- Wang, J., & Tanes-Ehle, Z. (2023). Examining the effects of conversational chatbots on changing conspiracy beliefs about science: The paradox of interactivity. Journal of Broadcasting & Electronic Media, 67(1), 68–89. https://doi.org/10.1080/08838151.2022.2153842
- Whittaker, L., Kietzmann, J., Letheren, K., Mulcahy, R., & Russell-Bennett, R. (2023). Brace yourself! Why managers should adopt a synthetic media incident response playbook in an age of falsity and synthetic media. Business Horizons, 66(2), 277–290. https://doi.org/10.1016/j.bushor.2022.07.004
- Wiesenberg, M., & Tench, R. (2020). Deep strategic mediatization: Organizational leaders’ knowledge and usage of social bots in an era of disinformation. International Journal of Information Management, 51. https://doi.org/10.1016/j.ijinfomgt.2019.102042
- Wölker, A., & Powell, T. E. (2021). Algorithms in the newsroom? News readers’ perceived credibility and selection of automated journalism. Journalism, 22(1), 86–103. https://doi.org/10.1177/1464884918757072
- Wu, Y. (2020). Is automated journalistic writing less biased? An experimental test of auto-written and human-written news stories. Journalism Practice, 14(8), 1008–1028. https://doi.org/10.1080/17512786.2019.1682940
- Wu, Y., Mou, Y., Li, Z., & Xu, K. (2020). Investigating American and Chinese subjects’ explicit and implicit perceptions of AI-generated artistic work. Computers in Human Behavior, 104, Article 106186, 1–11. https://doi.org/10.1016/j.chb.2019.106186
- Yang, J., Chuenterawong, P., Lee, H., & Chock, T. M. (2022). Anthropomorphism in CSR endorsement: A comparative study on human- vs. cartoon-like virtual influencers’ climate change messaging. Journal of Promotion Management, 1–30. https://doi.org/10.2139/ssrn.4145550
- Zheng, Y., Zhong, B., & Yang, F. (2018). When algorithms meet journalism: The user perception to automated news in a cross-cultural context. Computers in Human Behavior, 86, 266–275. https://doi.org/10.1016/j.chb.2018.04.046