Abstract
Aberrant activation or disruption of autophagy promotes tumorigenesis in various preclinical models of cancer, but whether the autophagy pathway is a target for recurrent molecular alteration in human cancer patient samples is unknown. To address this outstanding question, we surveyed 211 human autophagy-associated genes for tumor-related alterations to DNA sequence and RNA expression levels and examined their association with patient survival outcomes in multiple cancer types with sequence data from The Cancer Genome Atlas consortium. We found 3 (RB1CC1/FIP200, ULK4, WDR45/WIPI4) and one (ATG7) core autophagy genes to be under positive selection for somatic mutations in endometrial carcinoma and clear cell renal carcinoma, respectively, while 29 autophagy regulators and pathway interactors, including previously identified KEAP1, NFE2L2, and MTOR, were significantly mutated in 6 of the 11 cancer types examined. Gene expression analyses revealed that GABARAPL1 and MAP1LC3C/LC3C transcripts were less abundant in breast cancer and non-small cell lung cancers than in matched normal tissue controls; ATG4D transcripts were increased in lung squamous cell carcinoma, as were ATG16L2 transcripts in kidney cancer. Unsupervised clustering of autophagy-associated mRNA levels in tumors stratified patient overall survival in 3 of 9 cancer types (acute myeloid leukemia, clear cell renal carcinoma, and head and neck cancer). These analyses provide the first comprehensive resource of recurrently altered autophagy-associated genes in human tumors, and highlight cancer types and subtypes where perturbed autophagy may be relevant to patient overall survival.
Abbreviations
AA | = | autophagy-associated |
ATG | = | autophagy-related |
BRCA | = | breast invasive carcinoma |
CNA | = | copy-number alteration |
COAD | = | colon adenocarcinoma |
F | = | frequency |
FAB | = | French-American-British |
FC | = | median fold-change |
GBM | = | glioblastoma multiforme |
HNSC | = | head and neck squamous cell carcinoma |
KIRC | = | kidney renal clear cell carcinoma |
LAML | = | acute myeloid leukemia |
LUAD | = | lung adenocarcinoma |
LUSC | = | lung squamous cell carcinoma |
NMF | = | non-negative matrix factorization |
OS | = | overall survival |
OV | = | ovarian serous cystadenocarcinoma |
PPIs | = | protein-protein interactions |
READ | = | rectum adenocarcinoma |
RPKM | = | reads-per-kilobase-per-million-mapped reads |
SNV | = | single nucleotide variants |
UCEC | = | uterine corpus endometrial carcinoma. |
Introduction
To harvest energy and nutrients from cellular components, (macro)autophagy shuttles long-lived proteins and damaged organelles to lysosomes for degradation and subsequent nutrient release into the cytoplasm, thus recycling building blocks for new macromolecules. Autophagy remains active at basal rates under normal conditions, but is induced in response to stress as a cell survival mechanism to increase nonselective bulk degradation or to selectively target cytoplasmic constituents via cargo-specific autophagy receptors. Cancer cells experience many stresses known to induce autophagy, including nutrient deprivation, hypoxia, and damage from anticancer treatments, and some cancers may exploit increased autophagy as a drug-resistance mechanism or to promote tumorigenesis.Citation1 However, defective autophagy can also promote tumorigenesis through the accumulation of genotoxic cellular waste, which facilitates the acquisition of driver mutations and chromosomal lesions that can transform precancerous cells or benefit established tumor cells. This dual role for autophagy in cancer pathogenesis is well documented in preclinical cancer models;Citation2 however, whether these findings translate to patients remains unknown.Citation3 A fundamental and unexplored question is whether, and in what disease contexts, autophagy-associated (AA) genes are targets for recurrent molecular alteration in human cancer. As detailed below, we use the term AA to refer to not only the core ATG genes, but also to genes encoding known interactors of ATG proteins and regulators of the autophagy process (see also Methods and Table S1).
Recent efforts to catalog molecular alterations in cancer patients at the genomic level have produced large accessible repositories of human tumor data.Citation4-6 Our study presents a cross-cancer survey of tumor-associated molecular alteration of human autophagy genes, key autophagy regulators, and important pathway interactors from analyzed (level 3) patient sequence data (DNA and RNA) provided by The Cancer Genome Atlas (TCGA) consortium. We analyzed patterns of Darwinian selective pressure for somatic mutations identified in AA genes, examined AA gene expression changes between tumor and adjacent normal tissue samples, and identified differentially abundant autophagy pathway genes between patient groups (obtained from unsupervised clustering on AA transcript abundance) that display significant differential overall survival (OS). Our catalog of DNA and RNA changes to AA genes present in cancer patient samples will both inform current investigations and stimulate further research into the consequences of altered autophagy-associated genes in human cancers.
Results
The core autophagy machinery is not targeted by high-frequency somatic single nucleotide mutation across cancers
Human orthologs and paralogs of 31 autophagy-related (ATG) genes first identified in yeast form the core of our query gene set of 211 human AA genes. To sample cancer-associated alteration from the wider autophagy interaction network,Citation7 our gene set further included AA genes culled from the literature that participate in key upstream signaling networks (e.g., the MTOR subnetwork, AMP activated protein kinase complex, BECN1/Beclin 1 interactome), function as selective autophagy receptors (e.g., SQSTM1/p62, various MAP1LC3/LC3-interacting proteins), participate in known autophagy-associated cross-talk with other pathways (e.g., endocytic trafficking, cell death, immunity), or have been implicated in pathogenic autophagy modulation in disease contexts, such as cancer and neurodegeneration (see Materials and Methods; Table S1). diagrams the query gene set in the context of its known human protein-protein interactions (PPIs), obtained from the manually curated Reactome DatabaseCitation8 of pathways and reactions. We clustered AA PPIs into modules of high connectivity using the Reactome's spectral partition based network clustering algorithm,Citation9 and further labeled modules with enriched GO biological process terms (FDR < 0.01) that highlight some of the shared alternate functions of autophagy pathway interactors (e.g., roles in apoptosis, metabolism, vesicle-mediated transport, and signal transduction), which provide opportunities for autophagy crosstalk with other cellular pathways.
Figure 1. Network representation of core autophagy proteins, autophagy regulators and pathway interactors, clustered into functional modules of human protein-protein interactions by the Reactome Functional Interaction Network. Schematic showing the functional interconnectedness of 162 of 211 autophagy-associated proteins curated from literature. Edges represent human protein-protein interactions (PPI) reported in the Reactome database. Cellular functional compartments that are important in autophagy modulation are indicated by color-coded modules annotated by module-enriched GO Biological Process terms (FDR < 0.01). Node size ranks proteins by PPI count.
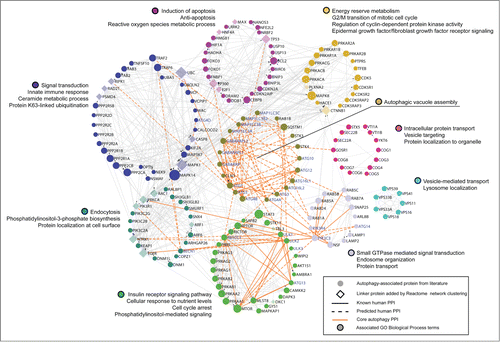
To assess whether AA genes were significant targets of somatic mutation in cancer, we first mined TCGA level 3 mutation data for coding, somatic, single nucleotide variants (SNV) identified in our gene set, across 11 cancer types: breast invasive carcinoma (BRCA), colon adenocarcinoma (COAD), glioblastoma multiforme (GBM), head and neck squamous cell carcinoma (HNSC), kidney renal clear cell carcinoma (KIRC), acute myeloid leukemia (LAML), lung adenocarcinoma (LUAD), lung squamous cell carcinoma (LUSC), ovarian serous cystadenocarcinoma (OV), rectum adenocarcinoma (READ), and uterine corpus endometrial carcinoma (UCEC). To distinguish between potential driver mutations and passenger mutations, we applied a method that searches for patterns of selective pressure adapted from the method described by Greenman et al.Citation10 and identified significantly mutated AA genes in 6 out of 11 cancer types (Benjamini-Hochberg (BH) adjusted P < 0.05; ; mutation frequency (F) and annotation in Table S2): GBM, HNSC, KIRC, LUAD, LUSC, and UCEC. Core autophagy genes (i.e., genes that build autophagosomes) were not found to be significantly mutated across most cancer types; however, UCEC and KIRC had significantly mutated core genes at patient frequencies of 0.06 or less: RB1CC1/FIP200 (0.060), ULK4 (0.048), WDR45/WIPI4 (0.044) and ATG4C (0.040) in UCEC, and ATG7 (0.032) in KIRC. In addition, a single truncating hotspot mutation was identified in RB1CC1 in UCEC (R1321* [F = 0.012], where the asterisk denotes a premature stop codon and infers a truncated protein; Table S2). Across cancers, as previously reported by TCGA, we confirmed 4 AA genes to be significantly mutated at frequencies of between 0.048 and 0.176 in 2 or more cancer types (): CDKN2A,Citation11,12 KEAP1,Citation11 NFE2L2/NRF2,Citation11-13 and MTOR.Citation13,14 Within cancer types, UCEC had the highest number of significantly mutated AA genes, including: PPP2R1A and BIRC6 (F > 0.1); LRRK2, TAB3, CDK5RAP2, RABGAP1, and RB1CC1 (F = 0.05–0.1); TBC1D15, ULK4, MTMR7, WDR45, DNM1L, MAPK8, PRKACG, TRAPPC8, ATG4C, RAB24, CDKN2AIP, HACE1, KBTBD7, PRKAA2, VTI1B, ARF6, CDK5R1, DIRAS3, and PRKAR2B (F< 0.05). The remaining cancer types showed fewer significantly mutated AA genes: PIK3C2G in GBM (F = 0.031); CDKN2A (F = 0.176) and NFE2L2 (F = 0.056) in HNSC; MTOR (F = 0.082), ATG7 (F = 0.014) and HIF1A (F = 0.01) in KIRC; KEAP1 (F = 0.157), STK11/LKB1 (F = 0.074), and CDKN2A (F = 0.048) in LUAD; and CDKN2A (F = 0.124), NFE2L2 (F = 0.146), and KEAP1 (F = 0.112) in LUSC. Previously reported hotspot mutations with frequencies of at least 0.01 were confirmed in 5 autophagy regulators and/or pathway interactors (Table S2). The highest frequency hotspots included R80* in CDKN2ACitation15 (F = 0.046, HNSC), P179R in PPP2R1ACitation15 (F = 0.032, UCEC), D29H/G/N/Y and R34Q/G/P in NFE2L2Citation11,16,17 (F = 0.028, LUSC), S2215Y in MTORCitation18,19 (F = 0.012, UCEC), and R470C in KEAP1Citation20 (F = 0.011, LUSC). Known hotspot substitutions in or near the DLG and ETGE KEAP1-binding motifs in NFE2L2Citation21 were observed in multiple cancers (HNSC, LUSC, UCEC); similarly, convergent hotspot mutations were present in CDKN2ACitation15 (W110* and E120*) in both HNSC and LUSC, which are squamous cell carcinomas of distinct tissue type that share mutational landscapes.Citation11
Figure 2. Circos plot overview of mutation frequency for significantly mutated autophagy-associated genes found in patients in 6 different cancers. Ribbons connect cancer type to mutated gene, ribbon color denotes levels of mutation frequency (range 0.012–0.176). Mutated genes were found to be under positive selection for somatic single nucleotide mutation, as assessed by an analysis of patterns of Darwinian selective pressure (adjusted P < 0.05). UCEC, uterine corpus endometrial carcinoma; LUSC, lung squamous cell carcinoma; LUAD, lung adenocarcinoma; HNSC, head and neck squamous cell carcinoma; KIRC, kidney renal clear cell carcinoma; GBM, glioblastoma multiforme.
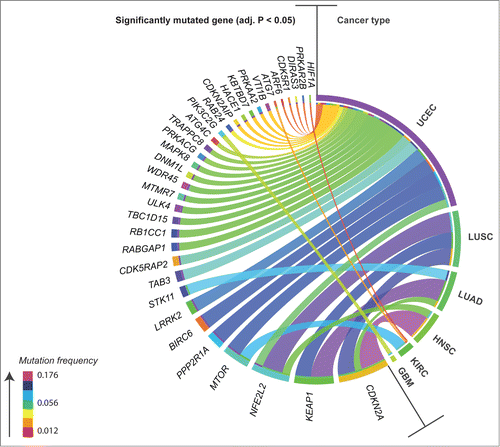
The low frequency of mutation of core autophagy genes suggests that the autophagy machinery is functional in patients of the 11 cancer types investigated, and therefore remains exploitable by these tumor types.
Autophagy regulators and pathway interactors show tumor-associated transcriptional modulation
To identify AA genes that showed a significant change in transcript abundance in tumor tissue, we performed a differential abundance analysis between tumor and adjacent normal tissue for BRCA (), KIRC (), LUAD (), and LUSC (). To determine whether observed differential expression could result from underlying copy-number alterations, we further stratified abundance of differentially expressed AA genes by copy-number status and examined whether a significant increase or decrease of mRNA abundance (Wilcoxon rank sum test P < 0.05) existed between patients with no somatic CNV versus patients with amplifications (in the case of increased differential expression) or homozygous deletions (in the case of decreased differential expression) (Table S3).
Figure 3 (See previous page). Differential gene expression analysis of pooled tumor versus pooled matched normal tissue gene abundance for 97 invasive breast carcinomas (BRCA) and 65 clear cell renal carcinomas (KIRC). (Right) Boxplots of normalized RNA-seq derived gene abundance (RPKM) displayed for autophagy-associated genes differentially expressed in tumor tissue compared to adjacent matched normal tissue in BRCA and KIRC patients. Differentially expressed genes showed significant (adjusted P < 0.05) differences in mRNA levels with a median fold-change > 2. (Left) Unsupervised clustering on only differentially expressed autophagy-associated genes (log2 transformed RPKM) grouped matched tumor and normal samples together.
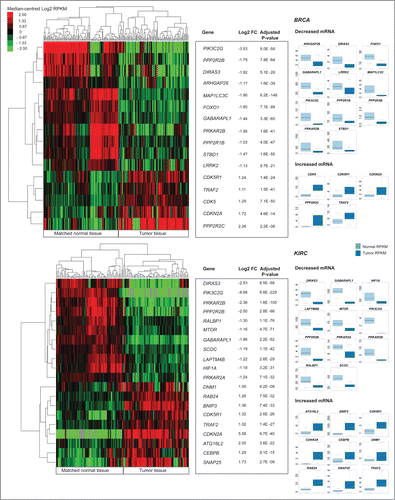
Figure 4. Differential expression analysis of pooled tumor versus pooled matched normal gene abundance for 17 lung squamous cell carcinomas (LUAD) and 25 lung adenocarcinomas (LUSC). (Right) Boxplots of normalized RNA-seq derived gene abundance (RPKM) displayed for autophagy-associated genes differentially expressed in tumor tissue compared to adjacent matched normal tissue in LUAD and LUSC patients. Differentially expressed genes showed significant (adjusted P < 0.05) differences in mRNA levels with a median fold-change > 2. (Left) Unsupervised clustering on only differentially expressed autophagy-associated (log2 transformed RPKM) genes grouped matched tumor and normal samples together.
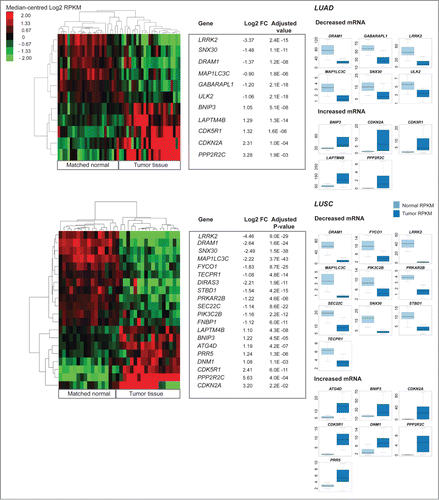
Differentially expressed (Wilcoxon rank sum test P < 0.05 and FC > 2.0 [RPKM]) core autophagy genes in tumor tissue included GABARAPL1 (BRCA, KIRC, LUAD), MAP1LC3C (BRCA, LUAD, LUSC), ATG4D (LUSC), and ATG16L2 (KIRC) (). In addition to GABARAPL1 and MAP1LC3C, 14 AA genes were differentially expressed in 2 or more cancer types (). With one exception (LAPTM4B), all differentially expressed genes identified in multiple cancers changed expression in the same direction, regardless of cancer type. Genes showing increased abundance in multiple cancers included: CDK5R1, CDKN2A, BNIP3, LAPTM4B, PPP2R2C, DNM1, and TRAF2; genes with decreased abundance in multiple cancers included: DIRAS3, GABARAPL1, LRRK2, MAP1LC3C, PRKAR2B, DRAM1, PIK3C2G, PPP2R2B, SNX30, and STBD1.
Table 1. Summary of autophagy-associated genes found to be differentially expressed between tumor and matched normal tissue, in 2 or more cancer types
Within cancer types, KIRC and LUSC cancers showed the largest number of differentially expressed AA genes. Genes uniquely differentially expressed in KIRC included ATG16L2, CEBPB, RAB24, and SNAP25 (increased mRNA), and HIF1A, MTOR, PRKAR2A, RALBP1, and SCOC (decreased mRNA). In LUSC, ATG4D and PRR5 transcripts were increased, while FNBP1, FYCO1, PIK3C2B, SEC22C, and TECPR1 transcripts were decreased. There were 4 differentially expressed genes unique in BRCA: CDK5 (increased mRNA) and ARHGAP26, FOXO1, and PPP2R1B (decreased mRNA). ULK2 was differentially expressed in LUAD only, showing decreased mRNA in tumors.
Only 3 differentially expressed AA genes showed a significant difference of mRNA levels (Wilcoxon rank sum test P < 0.05) in patients with focal amplifications or homozygous deletions compared to patients normal for copy-number alterations: CDK5R1 (FC = 2.45) and CDKN2A (FC = 7.48) showed recurrent (patient count > 5) amplification in BRCA, while RAB24 (FC = 1.31) showed recurrent amplification in KIRC (Table S3).
To further investigate changes to AA gene expression in patients bearing alterations of various clinically relevant tumor suppressors, oncogenes, chromosomal aberrations, or clinical phenotype (e.g., PAM50 subtype in BRCA, HPV infection status in HNSC, TP53 mutation in LAML), we stratified BRCA, HNSC, KIRC, LAML, LUAD, LUSC, and UCEC tumor transcript abundance for all 211 AA genes by the alteration status of patients (; Table S5). summarizes AA genes that showed significant increases or decreases of mRNA in tumors that harbored alterations in known disease genes or had reported clinical phenotypes, versus patients with no such alterations or reported phenotypes (list of all tested alterations and phenotypes in Methods; Wilcoxon rank sum test P < 0.05 and FC > 1.5 (RPKM)). With the exception of increased ATG3 expression in LAML patients with the French–American–British (FAB) M5 subtype and various changes in expression for members of the GABARAP family, core autophagy genes did not appear to be transcriptionally modulated in response to additional changes present in oncogenes and tumor suppressors, or to the phenotypes tested here. However, LAPTM4B, SQSTM1, and TNFSF10/TRAIL showed dynamic increases and decreases in mRNA level across multiple cancer types, depending on additional alterations or clinical conditions present in patients ().
Table 2. Summary of autophagy-associated genes found to have significantly increased or decreased mRNA in patients with disease-specific molecular alterations or clinical phenotypes (previously reported to be key in literature or identified in this study), compared to patients not harboring those alterations or phenotypes, in 7 cancer types
Differential expression analysis of tumor versus matched normal adjacent patient tissue revealed significantly increased and decreased AA transcript abundance that did not appear to be driven by underlying copy-number alterations, including 16 AA genes that were modified in the same direction in multiple cancer types. Although it remains to be assessed whether these recurrent changes to AA transcript abundance across tumors results in predictable changes to the autophagy status of tumors, transcriptional regulation of these known autophagy modifiers may represent a more general cancer-associated mechanism of tumor-associated autophagy modulation.
Unsupervised consensus clustering of autophagy-associated transcript abundance in tumors stratifies patient overall survival and links differential expression of autophagy regulators and interactors to enriched clinical features within patient groups
To discover whether distinct AA transcript abundance across the autophagy pathway could be associated with particular patient outcomes, we applied the non-negative matrix factorization (NMF) algorithmCitation22 to perform unsupervised consensus clustering of patient tumor samples on the mRNA levels of all 211 AA genes, and then applied differential survival analysis on patient groups identified by the NMF clustering. Of 9 cancers tested, 3 cancer types (LAML, KIRC and HNSC) showed significant differences in overall survival (OS) between patients grouped by differential AA gene expression: LAML (Log-rank test P = 0.0021), KIRC (P = 0.00010), and HNSC (P = 0.0140) (). Not all cancer types had equivalent lengths of follow-up time; therefore, it is possible that with further follow-up, other cancers would show significant differences in OS between cluster groups. To explore whether the differences in AA transcript abundance that grouped patients together reflected shared disease states, we assessed whether disease-specific clinical characteristics (such as tumor stage or diagnostic molecular feature) were significantly enriched across patient groups (Fisher exact test, adjusted P < 0.05) (). We then determined which AA genes were most influential in grouping patients with enriched clinical characteristics together by identifying AA genes that were differentially expressed within a single NMF-clustered patient group versus all others (Wilcoxon rank sum test P < 0.05 and median ratio of RPKM > 1.5) (; Table S4). Similar to results of the tumor versus normal analysis of differentially expressed AA genes, we found core transcript abundance to be largely unchanged between differentially surviving patient groups across cancer types. However, ULK1, ATG3, ATG7, and GABARAP had increased mRNA compared to all other groups in LAML patients with intermediate OS (LAML-G1 and -G2), ATG16L2 and GABARAPL1 showed increased abundance in KIRC patient groups with poor OS (KIRC-G2 and –G3), and ATG4D showed increased abundance in the best OS group in HNSC (HNSC-G4). The remainder of differentially expressed genes between patient groups included autophagy regulators and pathway interactors that did not show a common pattern of differential expression with respect to patient overall survival across cancer types, indicating heterogeneous expression of autophagy-associated genes between the tumor types investigated. Further, AA genes showed intratumoral heterogeneity of expression between patient groups enriched for distinct, disease-related molecular alterations and clinical phenotypes.
Figure 5 (See previous page). Unsupervised consensus clustering of autophagy-associated tumor gene abundance stratified patient overall survival for acute myeloid leukemia (LAML), kidney clear cell renal carcinoma (KIRC), and head and neck squamous cell carcinoma (HNSC), linking differential expression of autophagy regulators and interactors to enriched clinical features within patient groups. Unsupervised consensus clustering by non-negative matrix factorization (NMF) on mRNA levels of 211 autophagy-associated genes stratified patients into groups of significantly different overall survival (Log rank P < 0.05). Kaplan-Meier curves of overall survival are shown for HNSC, KIRC, and LAML. Disease-specific clinical covariates (diagnostic, prognostic and molecular phenotypes) found to be enriched within overall survival (OS) groups following clustering (Fisher exact test P < 0.05) are drawn below respective cancer types. Patient status for each covariate was obtained from clinical metadata collected by TCGA. Patient counts (n) for each covariate are noted. Enrichment is depicted as color-coded distributions of the relative frequency of each feature across OS groups per cancer type. Differentially expressed autophagy-associated genes, identified within individual OS groups compared to all other OS groups combined (Wilcoxon rank sum test P < 0.05; median fold-change > 1.5), are reported per cancer type. Boxplots of normalized RNA-seq derived gene abundance (RPKM) of differentially expressed genes are drawn for each OS group.
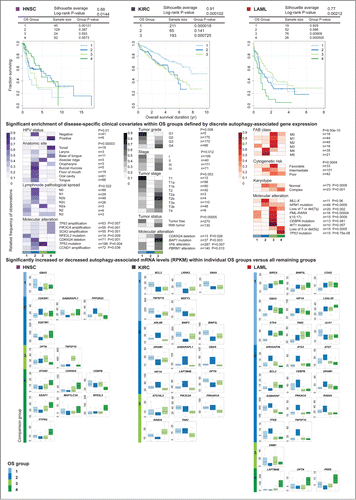
The best surviving (LAML-G3) and poorest surviving (LAML-G4) NMF-clustered LAML patient groups displayed OS curve separation compared to all others (P = 0.00091 and P = 0.00051, respectively; 0.0040 and 0.0024 after a Bonferroni correction). LAML-G3 showed the highest enrichment of patients with FAB classifications M0, M1, M2, and M3, the designation of ‘favorable cytogenetic risk,’ and the following molecular features: WT1 mutation, CEBPA mutation, PML-RARA fusion, chromosome translocation t(15;17), CBFB-MYH11 fusion, and loss of chromosome 7 or deletion of 7q. Although no AA genes were differentially expressed in LAML-G3 compared to all other groups, DNM1 transcripts (a mitophagy-related gene previously identified as part of the M3 specific dysregulomeCitation23) were increased in both patient groups enriched for the M3 subtype (LAML-G3 and –G1). Poor surviving LAML-G4 patients showed the highest enrichment for TP53 mutation, loss of chromosome 5 or deletion of 5q, and the designation of ‘complex cytogenetics,’ as well as increased transcript abundance for the AA modulators LAPTM4B, OPTN, and PRR5. LAML-G1 and -G2 showed intermediate survival compared to other groups; LAML-G2 had the highest enrichment of FAB classifications M4 and M5, designations of ‘intermediate cytogenetic risk’ and ‘normal karyotype,’ as well as enrichment for MLL-X fusion, NPM1 mutation, and CBFB-MYH11 fusions. In addition to the striking increases to core gene abundance mentioned above, LAML-G2 patients showed increased TNFSF10/TRAIL, TFEB, RAB24, and DRAM1 transcripts, among others ().
A similar analysis in KIRC defined 3 patient groups, with KIRC-G1 (most favorable OS) and KIRC-G3 (poor OS) displaying significantly different survival curves (P = 0.000018 and P = 0.00073, respectively; 0.00005 and 0.0022 after Bonferroni correction) (). Clinical covariates were divided between 2 of the 3 patient groups, KIRC-G1 and -G3. KIRC-G1 showed the highest enrichment of patients with lower tumor grade (G1, G2; P = 0.008), lower tumor stage (T1a, T1b; P = 0.053), lower stage (stage I; P = 0.012) and ‘tumor free’ designation (P = 0.00005), and increases in various autophagy pathway interactors (). Conversely, KIRC-G3, which showed increased ATG16L2 transcripts, was enriched with patients with higher tumor grade (G4), higher tumor stage (T2a, T2b), and higher stage (stage III, stage IV), and included almost all instances of BAP1 mutation (previously associated with poor OS by TCGACitation14). KIRC-G2 (with similar poor OS as KIRC-G3) contained the remainder of patients with advanced tumor stage (T4) not clustered in KIRC-G3, as well as increased ARL8B, GABARAPL1, GBAS, HIF1A, and LAPTM4B transcripts and decreased BNIP3, BNIP3L, DRAM1, and OPTN transcripts.
Clustering of HNSC patients resulted in a 4-group solution (). HNSC-G4 (with the most favorable OS) displayed a significantly different curve from all others (P = 0.0012; 0.0048 after the Bonferroni correction). HNSC-G4 showed enrichment for human papillomavirus (HPV) infection positive (+) patients, various known disease-related amplifications (TP63, PIK3CA, and SOX2), and increased abundance of a number of AA genes, including ATG4D, KEAP1, MAP1LC3A, and NFE2L2. HPV-negative (−) patients clustered in HNSC-G2 (intermediate OS), which was enriched for CDKN2A deletions and a large proportion of TP53 mutations, and showed decreased GABARAPL1 and SQSTM1 transcripts. The poorest OS group (HNSC-G1) contained the majority of NFE2L2/NRF2 mutations and showed striking increases of TNFSF10/TRAIL and OPTN abundance.
In summary, unsupervised clustering of abundance profiles for AA genes stratified patient OS, revealing associations between differentially expressed autophagy regulators and interactors and patient groups enriched for molecular alterations and disease phenotypes relevant for diagnosis, prognosis, and treatment of patients in LAML, KIRC, and HNSC.
Discussion
Autophagy can support either tumor suppression or tumor promotion depending on the additive influences of tissue type, disease subtype and stage, tumor genetic background, and therapeutic regimen.Citation24 Given this, we did not expect to draw general conclusions about the activity of the autophagy pathway across heterogeneous collections of tumor samples, particularly given the fact of tumor heterogeneity reported to exist between cancer types and subtypes, between patients, and within individual tumors.Citation4 In line with these ideas, our analyses revealed a diverse array of autophagy regulators and pathway interactors that were targeted for point mutation or showed differential expression in tumors, in specific disease contexts (). At the same time, our survey of single-nucleotide mutations targeting the autophagy pathway in 11 cancer types, coupled with our differential gene expression analysis of the pathway in matched tumor and normal samples in 4 of those cancer types, showed that the core autophagy machinery remains largely intact at the DNA level and is expressed at similar transcript levels as matched normal tissue, at least for the cancer types for which data was available from TCGA at the time of this study. Few ATG genes were found to be recurrently point-mutated (5 of 37 mammalian ATGs and paralogs), while those that were tended to be mutated at low frequency. Similarly, only 6 ATGs were differentially expressed (median FC > 2) in tumors versus matched normal tissues. The presence of a functional autophagy machinery in tumors suggests that the pathway is available for pathological modulation in tumors, and our data indicates this would most likely occur via the altered expression or function of autophagy regulators and/or pathway interactors. Transcriptional regulation of mammalian ATG genes has only recently begun to be investigated in depth;Citation25 however, the largely invariable gene expression of ATG genes found in this study suggests a pattern of transcriptional robustness similar to that of genes in biological contexts where little tolerance for deviation exists, such as during development.Citation26 The core autophagy machinery may be protected from alteration in some human cancers, an idea supported by recent studies in murine models of lung cancer that find autophagy to be essential for tumor progression.Citation27,28
Figure 6 (See previous page). Summary by gene of molecular alterations found to target autophagy-associated genes across multiple cancer types. This overview includes all significant somatic point mutation and differential gene expression identified in autophagy-associated genes in this study. Significant results were obtained from a differential expression analysis of tumor versus matched adjacent normal tissue for 4 cancer types examined (BRCA, KIRC, LUAD, and LUSC; detailed in ), as well as from a differential expression analysis between patient groups showing significantly different overall survival for 3 of 9 cancer types (HNSC, KIRC and LAML; detailed in ). Rows represent autophagy-associated genes found to have at least one significant alteration (either mutation or differential expression) in at least one context investigated. Groups of columns describe a single, major analysis in this study. The first column reports genes found to be significantly mutated in any of 6 of 11 cancer types investigated (detailed in ), with annotation of cancer type and mutation frequency found to the left. Cancer type, relative overall survival (OS) of patient groups, and value for differential expression of mRNA are color-coded, as described in the legend.
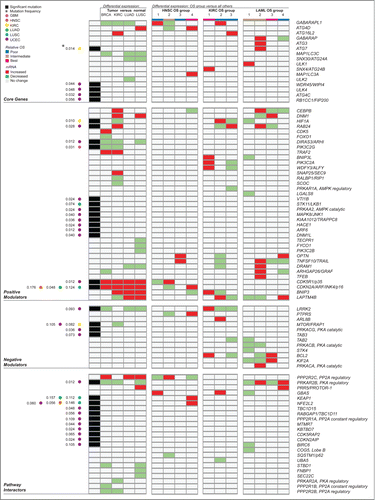
Importantly, we found that clustering abundance profiles of AA genes stratified patient OS in 3 of 9 cancer types and grouped patients with similar pathophysiology together, linking the differential expression of autophagy regulators and interactors to clinical features known to influence patient outcome. This suggests that clinically relevant differential pathway activities exist between subsets of patients in LAML, KIRC, and HNSC. The associations we have identified between changes in AA gene expression, clinical phenotype and patient outcome remain, at this time, predictions of a shared biology that may underpin disease in these novel groupings of patients. Identification of causal molecules and cell processes will require functional studies at the post-transcriptional level that examine the magnitude of influence these changes have on autophagy modulation, disease phenotype and in vitro and in vivo survival, including whether altered autophagy is upstream or downstream of the specific disease processes enriched in patient groups. Nevertheless, our analyses have revealed that distinct combinations of dynamically expressed autophagy modulators exist within the larger transcriptional communities that cumulatively drive distinct pathologies. Further investigation of the autophagy status and tumorigenic capacity of cells that recapitulate the disease type, genetic background and autophagy-associated gene expression profiles identified in our study may reveal novel autophagy-associated mechanisms of cancer progression.
Tumor heterogeneity and the context dependence of autophagy status is consistent with our having identified few commonalities of AA gene alteration across cancers. However, we noted that several genes were differentially abundant in similar directions in tumors compared to matched normal tissues in at least 2 cancer types (). GABARAPL1 had decreased mRNA in BRCA, KIRC and LUAD. GABARAP family members are involved in autophagosome maturation, but the expression pattern and regulation of GABARAPL1 differs. Unlike other GABARAP or MAP1LC3/LC3 family members, GABARAPL1 possesses an estrogen-responsive element and can be activated by estradiol-17β, via ESR1/estrogen receptor α.Citation29 Reduced GABARAPL1 expression has been reported in various cancer cell lines compared to normal tissues,Citation30 and is correlated with higher histological grade in breast adenocarcinoma patients.Citation31 A mechanism has yet to be proposed for the association of reduced GABARAPL1 with increased pathogenesis in breast cancer; however, reduced autophagy function seems unlikely given the redundancy of GABARAPL1 in autophagosome biogenesis. Further study of a tumor suppressor role for GABARAPL1 may reveal novel autophagy-independent functions.
Lysosomes and mitochondria are organelles where pathology and autophagy dysregulation often overlap.Citation32-34 Two autophagy inducers, LAPTM4B, a lysosomal transmembrane glycoprotein required for lysosome function and autophagosome maturation, and BNIP3, a BH3 domain-containing protein that can induce both apoptosis and hypoxia-mediated mitophagy, showed increased mRNA in multiple tumor types. Upregulated LAPTM4B mRNA and protein levels have been documented in multiple cancers and cell lines, where they correlate with pathological grade and prognosis in vivo and promote proliferation, migration, invasion, and drug resistance in vitro.Citation35 In this study, LAPTM4B overexpression was identified in LUAD and LUSC tumor samples compared to normal tissues (), as well as in patients with poor OS in KIRC and LAML (), and in patients with basal-like BRCA (Table S5). Increased autophagy has been shown to contribute to malignant phenotypes in breast cancer cells with overexpressed LAPTM4B;Citation36 therefore, it seems plausible that increased LAPTM4B-mediated autophagy could promote tumor progression in other disease types with LAPTM4B overexpression. Deregulation of BNIP3, a hypoxia responsive gene which can induce cell death and mitophagy, is associated with aggressive disease in cancer.Citation37 We found BNIP3 overexpression in KIRC and in both histological subtypes of lung cancer, compared to normal tissues. Previous studies show that BNIP3 overexpression enhances tumor growth in lung cancer xenografts and correlates with poor survival in lung cancer patients.Citation38,39 BNIP3's contribution to tumorigenesis has yet to be linked to its role in mitophagy; however, the fact that increased levels of BNIP3 are associated with more aggressive disease suggests that a function in tumor cell survival via autophagy or mitophagy is a more likely mechanistic scenario than a role in cell death, in contexts of BNIP3 overexpression. Autophagy has documented roles in KRAS-driven lungCitation27,28 and pancreaticCitation40,41 cancer progression, and overactive RAS has been shown to upregulate BNIP3.Citation42 Further investigation of autophagy status in RAS-driven tumors with BNIP3 overexpression may reveal a mechanistic link between BNIP3 and tumor cell survival.
Uterine corpus endometrial carcinomas
Of 6 cancers showing significantly mutated AA genes (), UCEC had the highest number of significantly mutated genes (SMGs), including nearly all significantly mutated core autophagy genes (ATG4C, ULK4, RB1CC1/FIP200), as well as a high frequency of MTOR mutation (0.105) that included previously identified hotspot mutations recently characterized as MTOR pathway activity boosters (C1483F and S2215Y).Citation18,19 Histological UCEC subtypes include Type I endometrioid tumors that typically have better prognosis and are associated with high frequency PTEN mutation (among other lesions), while Type II serous tumors have worse prognosis and are classified by high frequency TP53 mutation.Citation13 Almost all AA SMGs identified in this study were found in patients with endometrioid UCEC, including 20 patients that were double-mutant for MTOR and PTEN. MTOR is thought to be overactive in endometrial cancers with inactive PTEN,Citation43 and as MTOR is a major posttranslational inhibitor of autophagy, it is tempting to speculate that autophagy may be less active in type I endometrioid tumors. It is noteworthy that we observed a recurrent truncating mutation (R1321*) in autophagy induction complex member RB1CC1 in 3 UCEC patients, along with a predicted damaging substitution (S93L) in 2 additional patients, suggesting autophagy induction may be compromised in these endometrioid UCEC patients. In contrast, more aggressive type II serous tumors showed a dramatic mRNA increase of an autophagy inducer, CDKN2A (; Table S5), previously reported to distinguish serous from endometrioid carcinomas by high protein expressionCitation13,44 and to be associated with migration and invasion.Citation45 Both protein products of CDKN2A (ARF and INK4a) induce autophagyCitation46,47 and a recent IHC analysis of 360 UCEC patient samples shows higher counts of MAP1LC3A/LC3A ‘stone-like structures’ that are associated with poor prognosis subtypes,Citation48 including: serous papillary, clear cell, and high-grade endometrioid. Further study of the autophagy status of less aggressive type I endometrioid tumors compared to more aggressive type II serous tumors may reveal a role for autophagy in the pathogenesis of endometrial carcinomas.
Head and neck squamous cell carcinoma
HNSCs are associated with smoking, alcohol use, and human papillomavirus (HPV) infection. HPV positive (+) HNSC is associated with better prognosis,Citation49 while TP53 mutation and CDKN2A deletion are associated with HPV-negative (−) HNSC.Citation50 Unsupervised clustering on 211 AA gene expression levels grouped HPV+ patients with patients showing the most favorable survival and HPV− patients with TP53 and CDKN2A mutant patients (); therefore, it seems that distinct HNSC phenotypes are detectable as perturbed AA transcript abundance. HNSC-G1 patients showed very poor survival and included 8/14 patients with mutated NFE2L2/NRF2 (Table S1), an important transcription factor in the oxidative stress response pathway. Activating NFE2L2 mutations have been identified in various cancers and are thought to lead to the constitutive activation of oxidative stress pathway genes that benefit tumor cell survival,Citation17,51 including the autophagy cargo receptor SQSTM1/p62. Overexpression of SQSTM1/p62 sets up a positive feedback loop that further activates NFE2L2 through competitive binding of the NFE2L2 inhibitor KEAP1. Three of 4 HNSC-G2 patients mutant for NFE2L2 harbored previously characterized E79 or E82 activating changesCitation21 and we found median SQSTM1/P62 mRNA levels to be higher in HNSC NFE2L2 mutants versus nonmutants (), suggesting that increased SQSTM1/p62-mediated autophagic degradation of KEAP1 could occur in NFE2L2 mutant HNSC patients with poor OS.
A smaller group of 24 patients (HNSC-G3) also showed poor OS, enrichment for metastasis in single lymph nodes (n2a), and a striking increase in TNFSF10/TRAIL gene expression. TNFSF10 can trigger cell survival and proliferation through NFKB/nuclear factor kappa B and/or MAPK8 (mitogen-activated protein kinase 8)-mediated cytoprotective autophagy.Citation52 Further investigation of the TNFSF10/TRAIL-MAPK8–autophagy axis in alveolar ridge tumors may reveal a cell-type dependent role for autophagy in the pathogenesis of these rare but aggressive tumors.
Acute myeloid leukemia
Diagnosis and prognosis of acute myeloid leukemia (LAML) is defined by specific combinations of cytogenetic and molecular risk factors, including transcription-factor fusions and recurrent mutations that tend toward mutual exclusivity.Citation53 OS stratification by unsupervised clustering of LAML patients on AA transcript abundance revealed 3 relative levels of OS among 4 patient groups (), each enriched for distinct, canonical cytogenetic or molecular pathologies. LAML-G3 patients with the most favorable OS showed enrichment for PML-RARA fusions and CEBPA mutations, alterations associated with better prognosis in LAML,Citation54 whereas LAML-G4 patients with the poorest OS were enriched for TP53 mutations and monosomal (MK) and complex karyotypes (CK). TP53 alteration in LAML patients with CK is associated with dismal outcome;Citation55 therefore, our cluster result suggests that AA genes show perturbed expression reflective of distinct combinations of diagnostic and prognostic alterations in LAML.
Leukemic cells often have increased lysosomal mass and biogenesis.Citation56 LAML-G2 patients overexpressed TFEB, a transcription factor that induces both lysosome biogenesis and autophagosome biogenesis. TFEB drives expression of AA genesCitation57 and ATG3, ATG7, and GABARAP all showed increased mRNA levels in LAML-G2 patients with median RPKM FC > 1.5. Further, LAML-G2 was enriched for patients with MLL fusions and M4 and M5 FAB subtypes that had increased transcript levels of additional AA genes, including: ATG14, MAP1LC3A, CEBPB, PRKAR1A, PRKAR2B, PRKACA, RAB24, and TNFSF10 (Table S5). M4 and M5 subtypes are defined by monocyte precursor overproliferation and are often associated with MLL fusions that may interfere with cellular differentiation.Citation54 Autophagy has been shown to maintain survival of monocytes during normal macrophage differentiation.Citation58 Perhaps patients with both MLL fusions and higher TFEB expression have levels of autophagy that can support the prolonged survival of blast cells with defective differentiation programs.
PML-RARA fusions are highly oncogenic and diagnostic of acute promyelocytic leukemia (APL) and the M3 subtype; however, APL patients show better OS than other LAML subtypes due to a favorable response to all-trans retinoic acid (ATRA) treatment.Citation54 Both LAML-G1 and LAML-G3 were enriched for PML-RARA fusions and the M3 subtype, and showed increased DNM1 mRNA compared to other groups (). High DNM1 expression is part of a gene expression signature specific to M3 patients and leukemic promyelocytes,Citation23 and is also required for mitophagy in yeast, where it recruits the fission complex to degrading mitochondria.Citation59 ATG13 transcript levels were particularly high in M3 patients (Table S5), while LAML-G1 patients showed increased ULK1 and ATG4D gene expression (; Table S5); all 3 core genes have recently been implicated in mammalian mitophagy.Citation60,61 Contradictory roles for autophagy in APL with PML-RARA fusions are described in the literature. Some studies implicate autophagy in basal and ATRA-induced degradation of PML-RARA fusions that may be beneficial for APL patients,Citation62 while others report constitutive autophagy activation in PML-RARA transplanted leukemic mice that supports leukemic cell growth and apoptotic resistance in vitro, following etoposide challenge.Citation63 It would be interesting to investigate whether high DNM1 expression increases mitophagy in M3 patients compared to other subtypes, and whether ATG4D, ULK1 and/or ATG13 are required for mitophagy in this context.
Clear cell renal carcinoma
The role of autophagy may change during kidney cancer progression depending on disease stage, as both inhibited and induced autophagy is hypothesized to contribute to tumorigenesis in TSC2-related hereditary kidney cancer syndromes.Citation64-66 Suppressed autophagy is implicated in the progression of polycystic kidney disease (PKD), where it promotes the growth of kidney cysts,Citation67 and patients with acquired cystic kidney disease are at a higher risk for KIRC.Citation68 Hypoxia also plays an important role in cyst expansion in PKD through the upregulation of HIF1A in cyst epithelium;Citation69 however, HIF1A has been shown to increase autophagic flux in a rat proximal tubular cell line.Citation70 Therefore, it may be that in the context of stabilized HIF1A, induced autophagy contributes to KIRC disease progression. KIRC-G2 patients showed poor OS, increased HIF1A mRNA levels compared to all other groups (), and a near complete lack of alterations in the 8 most mutated genes in KIRC, including VHL. Loss-of-function VHL mutations in KIRC lead to HIFA stabilization and the transcriptional activation of tumor-promoting hypoxia responsive genes; however, TCGA recently found that in the absence of VHL alteration (which occurred in 20% of the TCGA KIRC cohort) other molecular alterations exist that may act to stabilize HIFA.Citation14 Further investigation of the interplay between hypoxia, VHL status, autophagy status, and tumorigenesis in KIRC models may help define the complex role of autophagy dysregulation in kidney cancer.
Lung cancer: Adenocarcinoma and squamous cell carcinoma
Adenocarcinoma (LUAD) and squamous cell carcinoma (LUSC) are the 2 major subtypes of non-small cell lung carcinoma and are classified by morphology.Citation71 However, LUAD and LUSC also differ at the molecular level. For example, activating mutations in tyrosine kinase signaling pathway genes (e.g., EGFR, ALK, RET, ERBB2) are common in LUAD, while NFE2L2 mutations are a distinguishing feature of LUSC.Citation72 Autophagy has been shown to support tumorigenesis in lung cancer, particularly in KRAS-driven lung cancer cell lines and animal models that may be addicted to high levels of autophagy,Citation73,74 and autophagy inactivation in KRas-driven mouse models of lung cancer impairs cell growth, proliferation, and tumor progression.Citation27,28 We stratified gene expression of all 211 AA genes in both LUAD and LUSC by known recurrent somatic disease-specific alterations, including EGFR and KRAS alterations, and did not find differences in core gene expression between altered and wild-type patients; however, LUSC patients with EGFR amplification had increased mRNA levels of NFE2L2 (median FC > 2; Table S5). Both overactive and impaired autophagy are hypothesized to support oncogenic NFE2L2 activity in cancer,Citation75 through increased degradation of the NFE2L2 sequestering protein KEAP1Citation51 or through reduced degradation of KEAP1 binding protein SQSTM1/p62,Citation76 respectively. We confirmed that both KEAP1 and NFE2L2 are significantly mutated in TCGA lung cancer patientsCitation11 (). KEAP1 mutations predicted to be LOF (Table S1) were found in both subtypes, while NFE2L2 mutations were found only in LUSC, as well as in HNSC and UCEC. Several LUSC and HNSC patients had previously characterized E79 NFE2L2 activating mutations,Citation21 while activating E82 mutations were identified only in UCEC patients (Table S1). SQSTM1 is a target gene for transcriptional upregulation by NFE2L2,Citation77 and KEAP1 mutants in both LUAD and LUSC had significantly higher SQSTM1 mRNA levels compared to wild-type patients (; Table S5); therefore, as previously suggested, a positive-feedback loop may exist in patients with overactive NFE2L2/NRF2 (through NFE2L2 and/or KEAP1 mutation), whereby transcriptionally upregulated levels of SQSTM1 further stabilize NFE2L2 protein levels, through increased SQSTM1-mediated autophagic degradation of KEAP1.Citation77
A recent paper suggests that autophagy suppresses lung tumor development in a KRas-driven mouse model in early oncogenesis, while later supporting progression of established tumors within the same animals.Citation28 Patient samples of gene expression may harbor both tumor-suppressive and tumor-promoting AA gene expression signatures left from various time points during the natural history of each tumor. In this study, 2 known tumor-suppressors that can also induce autophagy were mutated or had decreased mRNA in lung tumor samples compared to normal tissues, STK11/LKB1Citation78 and DRAM1,Citation24 respectively, while a novel negative-regulator of both autophagy and chaperone-mediated autophagy, LRRK2,Citation79,80 also had decreased mRNA levels in both LUAD and LUSC. Decreased STK11 or DRAM1 suggests a possible decrease in autophagy in these tumors, while the striking loss of a negative-regulator of autophagy suggests a possible increase in autophagy. Therefore, it would seem that more elaborate studies of the cumulative effect on autophagy status of the function of multiple autophagy regulators are necessary to more accurately reflect the molecular environment in human tumors.
Breast invasive carcinoma
CDK5R1/p35, a positive regulator of the neuronal kinase CDK5, was overexpressed in all 4 cancer types for which we performed a tumor versus normal tissue comparison (BRCA, KIRC, LUAD, LUSC); however, CDK5 itself was overexpressed only in BRCA. CDK5 has previously been shown to be overexpressed in patients with breast cancer, pancreatic cancer, medullary thyroid carcinoma, prostate cancer, lung cancer, and glioblastoma,Citation81,82 and is an autophagy inducer in neurons in in vitro models of Parkinson disease.Citation83 We found that stratification of expression of our gene set by the alteration status of molecular changes previously implicated in breast cancer (Table S5) revealed increased mRNA levels of ATG5 and FOXO3 in KRAS-amplified BRCA patients. Further, both ATG3 and the autophagy inducer LAPTM4B had increased mRNA levels in BRCA patients with EGFR amplification and in patients with PAM50-defined ‘basal-like’ BRCA, while LAPTM4B was also increased in ‘HER2-enriched’ and ‘Luminal-B’ subtypes. These results support the idea that oncogene-induced autophagy is relevant in human breast cancer.Citation84
Conclusion
This is the first study to investigate the alteration status of an extensive set of autophagy-associated genes in cancer patient sequence data. Consistent with our current understanding of tumor heterogeneity, our analyses suggest that autophagy status, as far as it is inferable from DNA sequence and gene expression changes, is highly disease specific. Further research is required to determine whether the sequence alterations and gene expression changes identified here lead to concomitant changes in protein expression and/or function, and to examine whether alterations that lead to true functional changes benefit tumor cells through modulated autophagy or through other, autophagy-independent processes. Nevertheless, our study provides the first comprehensive resource of recurrently altered autophagy-associated genes found in patient samples, and highlights specific cancer-related phenotypes and genetic backgrounds where perturbed autophagy may be particularly relevant to patient overall survival. As sequence data for additional cancer types and subtypes becomes available, future studies will provide further insight into the targeting of autophagy-associated genes for alteration in human cancer.
Materials and Methods
Gene set curation
We curated 211 AA genes from the literature (for a complete list with references see Table S1) with which to query cancer patient sequence data provided by TCGA. The gene set included all human orthologs and paralogs of 31 ATG genes first identified in yeast, which we term core autophagy genes. To capture cancer associated alterations targeting AA genes in the wider autophagy interaction network7(AIN), we further included non-overlapping genes from 65 bait genes used by Behrends and colleagues in their mass spectrometry-based immuno-precipitation proteomic screen that originally defined the AIN, as well as prominent autophagy regulators, adaptors and cargo receptors described in the literature (Table S1). A schematic of the gene set () was generated using the Reactome Functional Interaction database Plugin 2 for Cytoscape 2.8.0Citation85,86 to illustrate high-confidence known and predicted human PPIs, between 162 members of the gene set that had manually-curated PPIs available. Using the Reactome Cluster FI network algorithm,Citation9,85 we further grouped PPIs identified within the gene set into modules with higher than expected intra-cluster connectivity versus inter-cluster connectivity. To highlight important cellular pathways reported to affect autophagy status, we annotated modules with significantly enriched (FDR < 0.01) GO biological process terms of member AA proteins.
Somatic mutations and selective pressure analysis
We collected all level-3 somatic mutations identified by TCGA in our tumor types and tested all genes for mutational patterns indicative of selective pressure in our gene set. We can infer selective pressure in a gene when its mutational profile deviates from the tumor's background mutation rate. The methods described by Greenman et al.Citation87 aim to identify genes with statistically significant deviations from the background profile. To accomplish this, we first classify the somatic mutations by type as either synonymous, nonsynonymous, truncating, nonstop or canonical splice site mutations using all transcripts in the Ensembl database. To simplify the model, truncating and non-stop mutations were considered equivalent. The type of mutation was obtained by determining the effect of each position in the isoform with the longest reading frame for a given gene. We further split each group by its nucleotide change (A>C, A>G, A>T, C>G, C>T, G>T); stratifying mutations in this manner allows the model to consider the effect of a tumor's mutational mechanism on the background profile. Under the assumption that synonymous mutations do not directly affect the encoded protein and thus do not confer an advantage to the gene, we use them to calculate a tumor's background mutation profile; that is, mutations appearing by chance through a tumor's mutational mechanism. Given this profile and nucleotide composition of a transcript, we can determine the expected type of mutations under no selection. By comparing the observed mutations to expected mutations in a gene, we can calculate a score statistic describing the level of selective pressure on the gene. The statistical significance of this score is obtained by comparing against an empirical distribution built through a 100,000-run Monte Carlo simulation under the null-hypothesis of no selective pressure. Finally, we obtain the type and strength of the selective pressure with the methods by Greenman et al.Citation87 a value less than, equal to or higher than 1 representing negative, null or positive selective pressure respectively.
We ran this analysis for a total of 211 genes across 11 cancer types (BRCA, COAD, GBM, HNSC, KIRC, LAML, LUAD, LUSC, OV, READ, UCEC). Due to the low number of mutations in our genes of interest, the background mutation rate was obtained by pooling mutations found in other genes not in this set. Doing so allowed us to build a more comprehensive mutational background. After correcting P-values for multiple-testing using Benjamini-Hochberg, SMGs were defined as having an FDR < 0.05 (; Table S2).
Differential expression analysis
To compare gene expression levels of tumor versus adjacent normal tissue, we downloaded RNA-seq read counts provided by TCGA for all genes across the genome for 4 cancers. Differentially expressed genes were identified using the LIMMA voom algorithm for RNA-seqCitation88 on TMM normalized read counts converted to log2 counts-per-million. We defined differentially expressed as a significant median fold change > 2 of log2 transformed tumoral gene expression (Benjamini Hoechberg adjusted P < 0.01) compared to matched normal. In addition, we required that a gene also possess a significant Wilcoxon rank sum test of tumor versus normal transcript abundance (reads-per-kilobase-per-million-mapped reads or RPKM; P < 0.01). To determine whether tumor and normal samples could be distinguished based on gene expression differences of differentially expressed genes identified by LIMMA, we performed unsupervised hierarchical clustering (using Cluster 3.0) on log2 transformed, median-centered RPKM transcript abundance (differentially expressed AA genes only) of combined tumor and normal samples, using complete linkage clustering and the Spearman rank correlation coefficient as similarity metric. To determine whether copy-number alterations (CNA) could contribute to differentially expressed AA genes, we first obtained amplification and homozygous deletion status of patients from cBioPortalCitation89 for all differentially expressed AA genes identified in our tumor versus normal analysis. For any focal CNAs with patient count > 5, we stratified differentially expressed AA transcript abundance by alteration status and applied a Wilcoxon rank sum test (P < 0.05) on altered versus wild-type expression per gene, and looked for significant increases or decreases of expression for amplified versus wild-type patients (potentially underlying increased differentially expressed AA gene expression) or homozygously deleted versus wild-type patients (potentially underlying decreased differentially expressed AA gene expression). We performed a similar analysis to determine whether AA transcript abundance is affected by patient genetic background or clinical phenotype, by obtaining (from cBioPortal) the alteration status of patients for molecular alterations and disease phenotypes reported in literature to have diagnostic or prognostic value, and for significant mutation identified in this study. For alterations or clinical manifestations with patient count > 5, we stratified transcript abundance for all 211 AA genes by alteration status and applied a Wilcoxon rank sum test (P < 0.05) on altered versus wild-type expression per gene, and looked for significant increases or decreases of AA mRNA in altered patients. We report AA genes with significant changes in gene expression in altered patients with a median FC (RPKM) > 1.5 (; Table S5). We tested AA gene expression changes in the context of known and previously reported mutation and/or CNA of the following genes: (in BRCA) AFF2, AKT1, ATM, BRCA1, BRCA2, BRIP1, CDH1, ERBB2, EGFR, FGFR1, FGFR2, FOXA1, GATA3, IGF1R, KMT2C, MAP2K4, MAP3K1, MYC, NBN, NF1, PIK3CA, PIK3R1, PTEN, PTPRD, RAD51C, RB1, RUNX1, SF3B1, TBX3, TP53; (in HNSC) CCND1, CDKN2A, EGFR, HRAS, NFE2L2, NOTCH1, PIK3CA, SOX2, TP53, TP63; (in KIRC) ARID1A, BAP1, KDM5C, MTOR, PBRM1, PTEN, SETD2, SMARCA4, TP53, VHL; (in LAML) CEBPA, DNMT3A, FLT3, IDH1 or IDH2, KRAS or NRAS, MLL, NPM1, PML-RARA, PTPN11, RUNX1, t(15;17), TET2, TP53, WT1, X11q23; (in LUAD) ALK, CDKN2A, KEAP1, KRAS, SMARCA4, STK11, TP53; (in LUSC) AKT2, APC, BAI3, BCL2L1, CCND1, CDKN2A, CREBBP, CUL3, EGFR, EYS, FBXW7, FGFR1, GRM8, KEAP1, KIT, MTOR, MUC16, MYC, NF1, NFE2L2, NOTCH1, NOTCH2, PIK3CA, PTEN, RB1, RUNX1T1, SMARCA4, SOX2, TP53, TP63; (in UCEC) ATG4C, BIRC6, CDK5RAP2, DNM1L, LRRK2, MAPK8, MTMR7, MTOR, NFE2L2, PPP2R1A, PRKACG, RABGAP1, RB1CC1, TAB3, TRAPPC8, ULK4, WDR45.
Unsupervised consensus clustering, clinical covariate analysis, and changes in gene expression between patient groups
To identify patient groups with shared patterns of AA gene expression in tumors, we employed a non-negative matrix factorization algorithmCitation22,90 to perform unsupervised consensus clustering of patient samples, per cancer type, on the RPKM values of all 211 AA genes in our gene set. We defined a robust clustering solution as having a cluster number that: generated a cophenetic correlation coefficient > 0.95 (in a comparison of K = 2 through K = 15 clusters per cancer type) over 200 iterations of clustering, maximized the silhouette averageCitation91 of all groups (> 0.7), and predicted groups with sample sizes of 10 or more patients. To identify changes in AA gene expression associated with differences in patient outcome, we focused on cluster solutions that defined patient groups with significantly different Kaplan-Meier overall survival curves (Log-rank test P < 0.05). We defined significant increases or decreases of transcript abundance (RPKM) between groups as a median fold change in expression > 1.5 (Wilcoxon rank sum test P < 0.05) for a single patient group compared to all other patient groups combined. In addition, we identified significantly enriched clinical phenotypes within each patient group (Fisher exact test P < 0.05) from disease-specific clinical metadata available from TCGA.
Disclosure of Potential Conflicts of Interest
No potential conflicts of interest were disclosed.
Supplemental Material
Supplemental data for this article can be accessed on the publisher's website.
1067362_supplemental_files.zip
Download Zip (343.7 KB)Acknowledgments
The results published here are in whole or part based upon data generated by the TCGA Research Network: http://cancergenome.nih.gov/. The authors thank Elizabeth Chun, Emilia Lim, Mario Jardon, Svetlana Bortnik, and Suganthi Chittaranjan for helpful discussions and/or comments on the manuscript.
Funding
Canadian Institutes of Health Research (New Investigator award and team grant GPG102167 to SMG).
References
- Leone RD, Amaravadi RK. Autophagy: a targetable linchpin of cancer cell metabolism. Trends Endocrinol Metab 2013; 24:209-17; PMID:23474062; http://dx.doi.org/10.1016/j.tem.2013.01.008
- Yang ZJ, Chee CE, Huang S, Sinicrope FA. The role of autophagy in cancer: therapeutic implications. Mol Cancer Ther 2011; 10:1533-41; PMID:21878654; http://dx.doi.org/10.1158/1535-7163.MCT-11-0047
- Lebovitz CB, Bortnik SB, Gorski SM. Here, there be dragons: charting autophagy-related alterations in human tumors. Clin Cancer Res Off J Am Assoc Cancer Res 2012; 18:1214-26; PMID:22253413; http://dx.doi.org/10.1158/1078-0432.CCR-11-2465
- Vogelstein B, Papadopoulos N, Velculescu VE, Zhou S, Diaz LA, Kinzler KW. Cancer genome landscapes. Science 2013; 339:1546-58; PMID:23539594; http://dx.doi.org/10.1126/science.1235122
- Rung J, Brazma A. Reuse of public genome-wide gene expression data. Nat Rev Genet 2013; 14:89-99; PMID:23269463; http://dx.doi.org/10.1038/nrg3394
- Mwenifumbo JC, Marra MA. Cancer genome-sequencing study design. Nat Rev Genet 2013; 14:321-32; PMID:23594910; http://dx.doi.org/10.1038/nrg3445
- Behrends C, Sowa ME, Gygi SP, Harper JW. Network organization of the human autophagy system. Nature 2010; 466:68-76; PMID:20562859; http://dx.doi.org/10.1038/nature09204
- Matthews L, Gopinath G, Gillespie M, Caudy M, Croft D, de Bono B, Garapati P, Hemish J, Hermjakob H, Jassal B, et al. Reactome knowledgebase of human biological pathways and processes. Nucleic Acids Res 2009; 37:D619-22; PMID:18981052; http://dx.doi.org/10.1093/nar/gkn863
- Newman MEJ. Modularity and community structure in networks. Proc Natl Acad Sci U S A 2006; 103:8577-82; PMID:16723398; http://dx.doi.org/10.1073/pnas.0601602103
- Greenman C, Stephens P, Smith R, Dalgliesh GL, Hunter C, Bignell G, Davies H, Teague J, Butler A, Stevens C, et al. Patterns of somatic mutation in human cancer genomes. Nature 2007; 446:153-8; PMID:17344846; http://dx.doi.org/10.1038/nature05610
- TCGA Research Network. Comprehensive genomic characterization of squamous cell lung cancers. Nature 2012; 489:519-25; PMID:22960745; http://dx.doi.org/10.1038/nature11404
- TCGA Sees Heterogeneity in Head and Neck Cancers. Cancer Discov 2013; 3:475-6; http://dx.doi.org/10.1158/2159-8290.CD-NB2013-049
- The Cancer Genome Atlas Research Network. Integrated genomic characterization of endometrial carcinoma. Nature 2013; 497:67-73; PMID:23636398; http://dx.doi.org/10.1038/nature12113
- The Cancer Genome Atlas Research Network. Comprehensive molecular characterization of clear cell renal cell carcinoma. Nature 2013; 499:43-9; PMID:23792563; http://dx.doi.org/10.1038/nature12222
- Spaans VM, Trietsch MD, Crobach S, Stelloo E, Kremer D, Osse EM, Haar NT, van Eijk R, Muller S, van Wezel T, et al. Designing a high-throughput somatic mutation profiling panel specifically for gynaecological cancers. PLoS ONE 2014; 9:e93451; PMID:24671188; http://dx.doi.org/10.1371/journal.pone.0093451
- Gao Y-B, Chen Z-L, Li J-G, Hu X-D, Shi X-J, Sun Z-M, Zhang F, Zhao Z-R, Li Z-T, Liu Z-Y, et al. Genetic landscape of esophageal squamous cell carcinoma. Nat Genet 2014; 46:1097-102; PMID:25151357; http://dx.doi.org/10.1038/ng.3076
- Shibata T, Kokubu A, Saito S, Narisawa-Saito M, Sasaki H, Aoyagi K, Yoshimatsu Y, Tachimori Y, Kushima R, Kiyono T, et al. NRF2 Mutation Confers Malignant Potential and Resistance to Chemoradiation Therapy in Advanced Esophageal Squamous Cancer. Neoplasia 2011; 13:864-73; PMID:21969819; http://dx.doi.org/10.1593/neo.11750
- Sato T, Nakashima A, Guo L, Coffman K, Tamanoi F. Single amino-acid changes that confer constitutive activation of mTOR are discovered in human cancer. Oncogene 2010; 29:2746-52; PMID:20190810; http://dx.doi.org/10.1038/onc.2010.28
- Grabiner BC, Nardi V, Birsoy K, Possemato R, Shen K, Sinha S, Jordan A, Beck AH, Sabatini DM. A diverse array of cancer-associated MTOR mutations are hyperactivating and can predict rapamycin sensitivity. Cancer Discov 2014; 4:554-63; PMID:24631838; http://dx.doi.org/10.1158/2159-8290.CD-13-0929
- Hast BE, Cloer EW, Goldfarb D, Li H, Siesser PF, Yan F, Walter V, Zheng N, Hayes DN, Major MB. Cancer-derived mutations in KEAP1 impair NRF2 degradation but not ubiquitination. Cancer Res 2014; 74:808-17; PMID:24322982; http://dx.doi.org/10.1158/0008-5472.CAN-13-1655
- Lo S-C, Li X, Henzl MT, Beamer LJ, Hannink M. Structure of the Keap1:Nrf2 interface provides mechanistic insight into Nrf2 signaling. EMBO J 2006; 25:3605-17; PMID:16888629; http://dx.doi.org/10.1038/sj.emboj.7601243
- Brunet J-P, Tamayo P, Golub TR, Mesirov JP. Metagenes and molecular pattern discovery using matrix factorization. Proc Natl Acad Sci U S A 2004; 101:4164-9; PMID:15016911; http://dx.doi.org/10.1073/pnas.0308531101
- Payton JE, Grieselhuber NR, Chang L-W, Murakami M, Geiss GK, Link DC, Nagarajan R, Watson MA, Ley TJ. High throughput digital quantification of mRNA abundance in primary human acute myeloid leukemia samples. J Clin Invest 2009; 119:1714-26; PMID:19451695; http://dx.doi.org/10.1172/JCI38248
- Liu EY, Ryan KM. Autophagy and cancer – issues we need to digest. J Cell Sci 2012; 125:2349-58; PMID:22641689; http://dx.doi.org/10.1242/jcs.093708
- Chandra V, Bhagyaraj E, Parkesh R, Gupta P. Transcription factors and cognate signalling cascades in the regulation of autophagy. Biol Rev 2015; PMID:25651938; http://dx.doi.org/10.1111/brv.12177
- Macneil LT, Walhout AJM. Gene regulatory networks and the role of robustness and stochasticity in the control of gene expression. Genome Res 2011; 21:645-57; PMID:21324878; http://dx.doi.org/10.1101/gr.097378.109
- Guo JY, Karsli-Uzunbas G, Mathew R, Aisner SC, Kamphorst JJ, Strohecker AM, Chen G, Price S, Lu W, Teng X, et al. Autophagy suppresses progression of K-ras-induced lung tumors to oncocytomas and maintains lipid homeostasis. Genes Dev 2013; 27:1447-61; PMID:23824538; http://dx.doi.org/10.1101/gad.219642.113
- Rao S, Tortola L, Perlot T, Wirnsberger G, Novatchkova M, Nitsch R, Sykacek P, Frank L, Schramek D, Komnenovic V, et al. A dual role for autophagy in a murine model of lung cancer. Nat Commun 2014; 5:3056; PMID:24445999; http://dx.doi.org/10.1038/ncomms4056
- Vernier-Magnin S, Nemos C, Mansuy V, Tolle F, Guichard L, Delage-Mourroux R, Jouvenot M, Fraichard A. Analysis of the guinea-pig estrogen-regulated gec1/GABARAPL1 gene promoter and identification of a functional ERE in the first exon. Biochim Biophys Acta 2005; 1731:23-31; PMID:16153720; http://dx.doi.org/10.1016/j.bbaexp.2005.05.002
- Nemos C, Mansuy V, Vernier-Magnin S, Fraichard A, Jouvenot M, Delage-Mourroux R. Expression of gec1/GABARAPL1 versus GABARAP mRNAs in human: predominance of gec1/GABARAPL1 in the central nervous system. Mol Brain Res 2003; 119:216-9; PMID:14625090; http://dx.doi.org/10.1016/j.molbrainres.2003.09.011
- Berthier A, Seguin S, Sasco AJ, Bobin JY, De Laroche G, Datchary J, Saez S, Rodriguez-Lafrasse C, Tolle F, Fraichard A, et al. High expression of gabarapl1 is associated with a better outcome for patients with lymph node-positive breast cancer. Br J Cancer 2010; 102:1024-31; PMID:20197771; http://dx.doi.org/10.1038/sj.bjc.6605568
- Kroemer G, Jäättelä M. Lysosomes and autophagy in cell death control. Nat Rev Cancer 2005; 5:886-97; PMID:16239905; http://dx.doi.org/10.1038/nrc1738
- Mathew R, White E. Autophagy, stress, and cancer metabolism: what doesn't kill you makes you stronger. Cold Spring Harb Symp Quant Biol 2011; 76:389-96; PMID:22442109; http://dx.doi.org/10.1101/sqb.2012.76.011015
- Lee J, Giordano S, Zhang J. Autophagy, mitochondria and oxidative stress: cross-talk and redox signalling. Biochem J 2012; 441:523-40; PMID:22187934; http://dx.doi.org/10.1042/BJ20111451
- Li L, Wei XH, Pan YP, Li HC, Yang H, He QH, Pang Y, Shan Y, Xiong FX, Shao GZ, et al. LAPTM4B: A novel cancer-associated gene motivates multidrug resistance through efflux and activating PI3K/AKT signaling. Oncogene 2010; 29:5785-95; PMID:20711237; http://dx.doi.org/10.1038/onc.2010.303
- Li Y, Zhang Q, Tian R, Wang Q, Zhao JJ, Iglehart JD, Wang ZC, Richardson AL. Lysosomal transmembrane protein LAPTM4B promotes autophagy and tolerance to metabolic stress in cancer cells. Cancer Res 2011; 71:7481-9; PMID:22037872; http://dx.doi.org/10.1158/0008-5472.CAN-11-0940
- Zhang J, Ney PA. Role of BNIP3 and NIX in cell death, autophagy, and mitophagy. Cell Death Differ 2009; 16:939-46; PMID:19229244; http://dx.doi.org/10.1038/cdd.2009.16
- Karpathiou G, Sivridis E, Koukourakis M, Mikroulis D, Bouros D, Froudarakis M, Bougioukas G, Maltezos E, Giatromanolaki A. Autophagy and Bcl-2/BNIP3 death regulatory pathway in non-small cell lung carcinomas. APMIS Acta Pathol Microbiol Immunol Scand 2013; 121:592-604; PMID:23216071; http://dx.doi.org/10.1111/apm.12026
- Vijayalingam S, Pillai SG, Rashmi R, Subramanian T, Sagartz JE, Chinnadurai G. Overexpression of BH3-Only protein BNIP3 leads to enhanced tumor growth. Genes Cancer 2010; 1:964-71; PMID:21779475; http://dx.doi.org/10.1177/1947601910386110
- Blum R, Kloog Y. Metabolism addiction in pancreatic cancer. Cell Death Dis 2014; 5:e1065; PMID:24556680; http://dx.doi.org/10.1038/cddis.2014.38
- Rosenfeldt MT, O'Prey J, Morton JP, Nixon C, MacKay G, Mrowinska A, Au A, Rai TS, Zheng L, Ridgway R, et al. p53 status determines the role of autophagy in pancreatic tumour development. Nature 2013; 504:296-300; PMID:24305049; http://dx.doi.org/10.1038/nature12865
- An H-J, Maeng O, Kang K-H, Lee J-O, Kim Y-S, Paik S-G, Lee H. Activation of Ras Up-regulates Pro-apoptotic BNIP3 in Nitric Oxide-induced Cell Death. J Biol Chem 2006; 281:33939-48; PMID:16954213; http://dx.doi.org/10.1074/jbc.M605819200
- Cheng H, Liu P, Zhang F, Xu E, Symonds L, Ohlson CE, Bronson RT, Maira S-M, Di Tomaso E, Li J, et al. A genetic mouse model of invasive endometrial cancer driven by concurrent loss of pten and Lkb1 is highly responsive to mtor inhibition. Cancer Res 2013; 74:15-23.
- Bartosch C, Manuel Lopes J, Oliva E. Endometrial carcinomas: a review emphasizing overlapping and distinctive morphological and immunohistochemical features. Adv Anat Pathol 2011; 18:415-37; PMID:21993268; http://dx.doi.org/10.1097/PAP.0b013e318234ab18
- Jung A, Schrauder M, Oswald U, Knoll C, Sellberg P, Palmqvist R, Niedobitek G, Brabletz T, Kirchner T. The invasion front of human colorectal adenocarcinomas shows co-localization of nuclear beta-catenin, cyclin D1, and p16INK4A and is a region of low proliferation. Am J Pathol 2001; 159:1613-7; PMID:11696421; http://dx.doi.org/10.1016/S0002-9440(10)63007-6
- Budina-Kolomets A, Hontz RD, Pimkina J, Murphy ME. A conserved domain in exon 2 coding for the human and murine ARF tumor suppressor protein is required for autophagy induction. Autophagy 2013; 9; PMID:23939042; http://dx.doi.org/10.4161/auto.25831
- Jiang H, Martin V, Gomez-Manzano C, Johnson DG, Alonso M, White E, Xu J, McDonnell TJ, Shinojima N, Fueyo J. The RB-E2F1 pathway regulates autophagy. Cancer Res 2010; 70:7882-93; PMID:20807803; http://dx.doi.org/10.1158/0008-5472.CAN-10-1604
- Sivridis E, Giatromanolaki A, Liberis V, Koukourakis MI. Autophagy in endometrial carcinomas and prognostic relevance of “stone-like” structures (SLS): What is destined for the atypical endometrial hyperplasia? Autophagy 7:74-82; PMID:21099253; http://dx.doi.org/10.4161/auto.7.1.13947
- Kimple RJ, Smith MA, Blitzer GC, Torres AD, Martin JA, Yang RZ, Peet CR, Lorenz LD, Nickel KP, Klingelhutz AJ, et al. Enhanced radiation sensitivity in HPV-positive head and neck cancer. Cancer Res 2013; 73:4791-800; PMID:23749640; http://dx.doi.org/10.1158/0008-5472.CAN-13-0587
- Lechner M, Frampton G, Fenton T, Feber A, Palmer G, Jay A, Pillay N, Forster M, Cronin MT, Lipson D. Targeted next-generation sequencing of head and neck squamous cell carcinoma identifies novel genetic alterations in HPV+ and HPV-tumors. Genome Med 2013; 5:49; PMID:23718828; http://dx.doi.org/10.1186/gm453
- Taguchi K, Fujikawa N, Komatsu M, Ishii T, Unno M, Akaike T, Motohashi H, Yamamoto M. Keap1 degradation by autophagy for the maintenance of redox homeostasis. Proc Natl Acad Sci U S A 2012; 109:13561-6; PMID:22872865; http://dx.doi.org/10.1073/pnas.1121572109
- He W, Wang Q, Xu J, Xu X, Padilla MT, Ren G, Gou X, Lin Y. Attenuation of TNFSF10/TRAIL-induced apoptosis by an autophagic survival pathway involving TRAF2- and RIPK1/RIP1-mediated MAPK8/JNK activation. Autophagy 2012; 8:1811-21; PMID:23051914; http://dx.doi.org/10.4161/auto.22145
- The Cancer Genome Atlas Research Network. Genomic and epigenomic landscapes of adult de novo acute myeloid leukemia. N Engl J Med 2013; 368:2059-74; PMID:23634996; http://dx.doi.org/10.1056/NEJMoa1301689
- Marchesi F, Annibali O, Cerchiara E, Tirindelli MC, Avvisati G. Cytogenetic abnormalities in adult non-promyelocytic acute myeloid leukemia: A concise review. Crit Rev Oncol Hematol 2011; 80:331-46; PMID:21123080; http://dx.doi.org/10.1016/j.critrevonc.2010.11.006
- Rücker FG, Schlenk RF, Bullinger L, Kayser S, Teleanu V, Kett H, Habdank M, Kugler C-M, Holzmann K, Gaidzik VI, et al. TP53 alterations in acute myeloid leukemia with complex karyotype correlate with specific copy number alterations, monosomal karyotype, and dismal outcome. Blood 2012; 119:2114-21; PMID:22186996; http://dx.doi.org/10.1182/blood-2011-08-375758
- Sukhai MA, Prabha S, Hurren R, Rutledge AC, Lee AY, Sriskanthadevan S, Sun H, Wang X, Skrtic M, Seneviratne A, et al. Lysosomal disruption preferentially targets acute myeloid leukemia cells and progenitors. J Clin Invest 2013; 123:315-28; PMID:23202731; http://dx.doi.org/10.1172/JCI64180
- Settembre C, Di Malta C, Polito VA, Arencibia MG, Vetrini F, Erdin S, Erdin SU, Huynh T, Medina D, Colella P, et al. TFEB links autophagy to lysosomal biogenesis. Science 2011; 332:1429-33; PMID:21617040; http://dx.doi.org/10.1126/science.1204592
- Zhang Y, Morgan MJ, Chen K, Choksi S, Liu Z. Induction of autophagy is essential for monocyte-macrophage differentiation. Blood 2012; 119:2895-905; PMID:22223827; http://dx.doi.org/10.1182/blood-2011-08-372383
- Mao K, Wang K, Liu X, Klionsky DJ. The scaffold protein Atg11 recruits fission machinery to drive selective mitochondria degradation by autophagy. Dev Cell 2013; 26:9-18; PMID:23810512; http://dx.doi.org/10.1016/j.devcel.2013.05.024
- Betin VMS, MacVicar TDB, Parsons SF, Anstee DJ, Lane JD. A cryptic mitochondrial targeting motif in Atg4D links caspase cleavage with mitochondrial import and oxidative stress. Autophagy 2012; 8:664-76; PMID:22441018; http://dx.doi.org/10.4161/auto.19227
- Joo JH, Dorsey FC, Joshi A, Hennessy-Walters KM, Rose KL, McCastlain K, Zhang J, Iyengar R, Jung CH, Suen D-F, et al. Hsp90-Cdc37 chaperone complex regulates Ulk1- and Atg13-mediated mitophagy. Mol Cell 2011; 43:572-85; PMID:21855797; http://dx.doi.org/10.1016/j.molcel.2011.06.018
- Isakson P, Bjørås M, Bøe SO, Simonsen A. Autophagy contributes to therapy-induced degradation of the PML/RARA oncoprotein. Blood 2010; 116:2324-31; PMID:20574048; http://dx.doi.org/10.1182/blood-2010-01-261040
- Huang Y, Hou J-K, Chen T-T, Zhao X-Y, Yan Z-W, Zhang J, Yang J, Kogan SC, Chen G-Q. PML-RARα enhances constitutive autophagic activity through inhibiting the Akt/mTOR pathway. Autophagy 2011; 7:1132-44; PMID:21673516; http://dx.doi.org/10.4161/auto.7.10.16636
- Linehan WM, Srinivasan R, Schmidt LS. The genetic basis of kidney cancer: a metabolic disease. Nat Rev Urol 2010; 7:277-85; PMID:20448661; http://dx.doi.org/10.1038/nrurol.2010.47
- Parkhitko A, Myachina F, Morrison TA, Hindi KM, Auricchio N, Karbowniczek M, Wu JJ, Finkel T, Kwiatkowski DJ, Yu JJ, et al. Tumorigenesis in tuberous sclerosis complex is autophagy and p62/sequestosome 1 (SQSTM1)-dependent. Proc Natl Acad Sci 2011; 108:12455-60; PMID:21746920; http://dx.doi.org/10.1073/pnas.1104361108
- Zhang J, Kim J, Alexander A, Cai S, Tripathi DN, Dere R, Tee AR, Tait-Mulder J, Di Nardo A, Han JM, et al. A tuberous sclerosis complex signalling node at the peroxisome regulates mTORC1 and autophagy in response to ROS. Nat Cell Biol 2013; 15:1186-96; PMID:23955302; http://dx.doi.org/10.1038/ncb2822
- Ravichandran K, Edelstein CL. Polycystic kidney disease: a case of suppressed autophagy? Semin Nephrol 2014; 34:27-33; PMID:24485027; http://dx.doi.org/10.1016/j.semnephrol.2013.11.005
- Chen Y-B, Tickoo SK. Spectrum of preneoplastic and neoplastic cystic lesions of the kidney. Arch Pathol Lab Med 2012; 136:400-9; PMID:22458902; http://dx.doi.org/10.5858/arpa.2011-0485-RA
- Belibi F, Zafar I, Ravichandran K, Segvic AB, Jani A, Ljubanovic DG, Edelstein CL. Hypoxia-inducible factor-1α (HIF-1α) and autophagy in polycystic kidney disease (PKD). Am J Physiol Renal Physiol 2011; 300:F1235-43; PMID:21270095; http://dx.doi.org/10.1152/ajprenal.00348.2010
- Jiang M, Liu K, Luo J, Dong Z. Autophagy is a renoprotective mechanism during in vitro hypoxia and in vivo ischemia-reperfusion injury. Am J Pathol 2010; 176:1181-92; PMID:20075199; http://dx.doi.org/10.2353/ajpath.2010.090594
- Rekhtman N, Ang DC, Sima CS, Travis WD, Moreira AL. Immunohistochemical algorithm for differentiation of lung adenocarcinoma and squamous cell carcinoma based on large series of whole-tissue sections with validation in small specimens. Mod Pathol 2011; 24:1348-59; PMID:21623384; http://dx.doi.org/10.1038/modpathol.2011.92
- Kim YR, Oh JE, Kim MS, Kang MR, Park SW, Han JY, Eom HS, Yoo NJ, Lee SH. Oncogenic NRF2 mutations in squamous cell carcinomas of oesophagus and skin. J Pathol 2010; 220:446-51; PMID:19967722; http://dx.doi.org/10.1002/path.2653
- Sakuma Y, Matsukuma S, Nakamura Y, Yoshihara M, Koizume S, Sekiguchi H, Saito H, Nakayama H, Kameda Y, Yokose T, et al. Enhanced autophagy is required for survival in EGFR-independent EGFR-mutant lung adenocarcinoma cells. Lab Investig J Tech Methods Pathol 2013; 93:1137-46; PMID:23938604; http://dx.doi.org/10.1038/labinvest.2013.102
- Guo JY, Chen H-Y, Mathew R, Fan J, Strohecker AM, Karsli-Uzunbas G, Kamphorst JJ, Chen G, Lemons JMS, Karantza V, et al. Activated Ras requires autophagy to maintain oxidative metabolism and tumorigenesis. Genes Dev 2011; 25:460-70; PMID:21317241; http://dx.doi.org/10.1101/gad.2016311
- Taguchi K, Motohashi H, Yamamoto M. Molecular mechanisms of the Keap1–Nrf2 pathway in stress response and cancer evolution. Genes Cells 2011; 16:123-40; PMID:21251164; http://dx.doi.org/10.1111/j.1365-2443.2010.01473.x
- Inami Y, Waguri S, Sakamoto A, Kouno T, Nakada K, Hino O, Watanabe S, Ando J, Iwadate M, Yamamoto M, et al. Persistent activation of Nrf2 through p62 in hepatocellular carcinoma cells. J Cell Biol 2011; 193:275-84; PMID:21482715; http://dx.doi.org/10.1083/jcb.201102031
- Jain A, Lamark T, Sjøttem E, Larsen KB, Awuh JA, Øvervatn A, McMahon M, Hayes JD, Johansen T. p62/SQSTM1 is a target gene for transcription factor NRF2 and creates a positive feedback loop by inducing antioxidant response element-driven gene transcription. J Biol Chem 2010; 285:22576-91; PMID:20452972; http://dx.doi.org/10.1074/jbc.M110.118976
- Ji H, Ramsey MR, Hayes DN, Fan C, McNamara K, Kozlowski P, Torrice C, Wu MC, Shimamura T, Perera SA, et al. LKB1 modulates lung cancer differentiation and metastasis. Nature 2007; 448:807-10; PMID:17676035; http://dx.doi.org/10.1038/nature06030
- Orenstein SJ, Kuo S-H, Tasset I, Arias E, Koga H, Fernandez-Carasa I, Cortes E, Honig LS, Dauer W, Consiglio A, et al. Interplay of LRRK2 with chaperone-mediated autophagy. Nat Neurosci 2013; 16:394-406; PMID:23455607; http://dx.doi.org/10.1038/nn.3350
- Manzoni C, Mamais A, Dihanich S, Abeti R, Soutar MPM, Plun-Favreau H, Giunti P, Tooze SA, Bandopadhyay R, Lewis PA. Inhibition of LRRK2 kinase activity stimulates macroautophagy. Biochim Biophys Acta 2013; 1833:2900-10; PMID:23916833; http://dx.doi.org/10.1016/j.bbamcr.2013.07.020
- Pozo K, Castro-Rivera E, Tan C, Plattner F, Schwach G, Siegl V, Meyer D, Guo A, Gundara J, Mettlach G, et al. The role of Cdk5 in neuroendocrine thyroid cancer. Cancer Cell 2013; 24:499-511; PMID:24135281; http://dx.doi.org/10.1016/j.ccr.2013.08.027
- Liang Q, Li L, Zhang J, Lei Y, Wang L, Liu D-X, Feng J, Hou P, Yao R, Zhang Y, et al. CDK5 is essential for TGF-β1-induced epithelial-mesenchymal transition and breast cancer progression. Sci Rep 2013; 3:2932; PMID:24121667
- Wong ASL, Lee RHK, Cheung AY, Yeung PK, Chung SK, Cheung ZH, Ip NY. Cdk5-mediated phosphorylation of endophilin B1 is required for induced autophagy in models of Parkinson's disease. Nat Cell Biol 2011; 13:568-79; PMID:21499257; http://dx.doi.org/10.1038/ncb2217
- Jain K, Paranandi KS, Sridharan S, Basu A. Autophagy in breast cancer and its implications for therapy. Am J Cancer Res 2013; 3:251; PMID:23841025
- Croft D, O'Kelly G, Wu G, Haw R, Gillespie M, Matthews L, Caudy M, Garapati P, Gopinath G, Jassal B, et al. Reactome: a database of reactions, pathways and biological processes. Nucleic Acids Res 2011; 39:D691-7; PMID:21067998; http://dx.doi.org/10.1093/nar/gkq1018
- Shannon P, Markiel A, Ozier O, Baliga NS, Wang JT, Ramage D, Amin N, Schwikowski B, Ideker T. Cytoscape: a software environment for integrated models of biomolecular interaction networks. Genome Res 2003; 13:2498-504; PMID:14597658; http://dx.doi.org/10.1101/gr.1239303
- Greenman C, Wooster R, Futreal PA, Stratton MR, Easton DF. Statistical analysis of pathogenicity of somatic mutations in cancer. Genetics 2006; 173:2187-98; PMID:16783027; http://dx.doi.org/10.1534/genetics.105.044677
- Law CW, Chen Y, Shi W, Smyth GK. Voom: precision weights unlock linear model analysis tools for RNA-seq read counts. Genome Biol 2014; 15:R29; PMID:24485249; http://dx.doi.org/10.1186/gb-2014-15-2-r29
- Gao J, Aksoy BA, Dogrusoz U, Dresdner G, Gross B, Sumer SO, Sun Y, Jacobsen A, Sinha R, Larsson E, et al. Integrative analysis of complex cancer genomics and clinical profiles using the cBioPortal. Sci Signal 2013; 6:pl1; PMID:23550210; http://dx.doi.org/10.1126/scisignal.2004088
- R-Forge: NMF - Nonnegative Matrix Factorization: R Development Page [Internet]. [cited 2012 Mar 1]; Available from: https://r-forge.r-project.org/R/?group_id=649
- Rousseeuw PJ. Silhouettes: A graphical aid to the interpretation and validation of cluster analysis. J Comput Appl Math 1987; 20:53-65; http://dx.doi.org/10.1016/0377-0427(87)90125-7