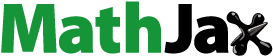
Abstract
Programmed cell death (PCD) is a critical biological process involved in many important processes, and defects in PCD have been linked with numerous human diseases. In recent years, the protein architecture in different PCD subroutines has been explored, but our understanding of the global network organization of the noncoding RNA (ncRNA)-mediated cell death system is limited and ambiguous. Hence, we developed the comprehensive bioinformatics resource (ncRDeathDB, www.rna-society.org/ncrdeathdb) to archive ncRNA-associated cell death interactions. The current version of ncRDeathDB documents a total of more than 4600 ncRNA-mediated PCD entries in 12 species. ncRDeathDB provides a user-friendly interface to query, browse and manipulate these ncRNA-associated cell death interactions. Furthermore, this resource will help to visualize and navigate current knowledge of the noncoding RNA component of cell death and autophagy, to uncover the generic organizing principles of ncRNA-associated cell death systems, and to generate valuable biological hypotheses.
Abbreviations
ATG10 | = | autophagy-related 10 |
ATG12 | = | autophagy-related 12 |
ATG16L1 | = | autophagy-related 16-like 1 |
ATG3 | = | autophagy-related 3 |
ATG4B | = | autophagy-related 4B, cysteine peptidase |
ATG5 | = | autophagy- related 5 |
BAX | = | BCL2-associated X protein |
BCL2 | = | B-cell CLL/lymphoma 2 |
CASP3 | = | caspase 3, apoptosis-related cysteine peptidase |
lncRNA | = | long non-coding RNA |
MAP1LC3B | = | microtubule-associated protein 1 light chain 3 beta |
MCL1 | = | myeloid cell leukemia 1 |
miRNA | = | microRNA |
ncRNA | = | noncoding RNA |
NPI | = | ncRNA-protein interaction |
PCD | = | programmed cell death |
PPI | = | protein-protein interaction |
snoRNA | = | small nucleolar RNA. |
Introduction
Noncoding RNAs (ncRNAs) are RNA species that are expressed in cells under certain conditions but do not code for proteins.Citation1 Currently, microRNAs (miRNAs) are the most studied type of ncRNAs, but novel classes of ncRNAs, such as long noncoding RNAs (lncRNAs) and snoRNAs, continue to be identified, and many studies have begun to focus on the role of ncRNA in programmed cell death.Citation2-5 Convincing evidence has been found for the involvement of microRNA,Citation6,7 lncRNACitation8 and snoRNACitation3 in common cell death subroutines such as apoptosis and autophagy. Despite this progress, unsolved questions remain; for example, while the outline of protein crosstalk between different PCD subroutines is relatively well characterized,Citation9,10 much less is known concerning the network organization of ncRNA-mediated cell death subroutines and how cell death-associated ncRNAs bridge various cell death subroutines and functional modules. Perhaps more importantly, it is unclear how the ncRNA-mediated cell death system interacts with canonical protein-protein interaction networks (PPI) networks and ncRNA-protein interaction (NPI) networks within processes of global signal transduction. Finally, lack of knowledge regarding pivotal ncRNAs and their protein targets, among the hundreds of ncRNAs found in PCD, hinders the development of ncRNA-based drug targets for clinical use.
To address these issues, we collected a large amount of published data describing the roles of diverse ncRNAs in PCD to develop and update the database ncRDeathDB (www.rna-society.org/ncrdeathdb) according to our previous miRDeathDBCitation11 for the purpose of archiving comprehensive ncRNA-associated cell death interactions. The current version of ncRDeathDB provides an all-inclusive bioinformatics resource on information detailing the ncRNA-mediated cell death system and includes 4615 (including 1817 predicted entries) ncRNA-mediated PCD entries involving 12 species. Furthermore, based on this comprehensive repository, we implemented several bioinformatics network techniques to systematically analyze the hierarchical and topologic characteristics of the ncRNA-mediated cell death system (). Then, we investigated its centrality and its functional role in the global signal transduction cascade by mapping it to the PPI and NPI networks. Finally, we identified several key ncRNAs players in both an experimentally verified autophagy module and a cell death network (including both autophagy and apoptosis modules) that together with their protein targets mediate intra- and inter-module regulation.
Results
Content of the ncRDeathDB database
According to our previous miRDeathDB, we connected the dot to build the miRNA-mediated cell death network.Citation12 However, with the increasing amount of data demonstrating important functions for ncRNA molecules in apoptosis and autophagy, we continuously curate the literature data and update it. At the same time, we realized the important role of other ncRNA (such as lncRNA and snoRNA) in apoptosis and autophagy. Hence, based on our reference retrieval, we recently updated miRDeathDBCitation11 to version 2.0 (ncRDeathDB, www.rna-society.org/ncrdeathdb), which allows users to browse, search, and analyze PCD-associated ncRNAs. Compared with its first release (miRDeathDB), ncRDeathDB not only enriches ncRNA types by including lncRNA and snoRNA with updated annotations, but also integrates a variety of useful tools for analyzing RNA-RNA and RNA-protein binding sites and for network visualization. The current version of ncRDeathDB documents 4615 (including 1817 predicted entries) ncRNA-associated cell death entries, including 2403 apoptosis-associated entries, 2205 autophagy-associated entries and 7 necrosis-associated entries, leading to a nearly 20-fold content enrichment compared to the initial miRDeathDB release in 2012. These entries were manually annotated from 1495 references, from 12 species, mainly including Homo sapiens, Mus musculus, and Rattus norvegicus (). Each entry contains detailed information, including annotation of ncRNAs, interacting genes, species, PMID, and detailed description. In the current version of ncRDeathDB, human, mouse, and rat account for 58.07%, 10.40% and 6.50% ncRNA-associated cell death entries, respectively. Notably, the human ncRNA-mediated cell death system consists of 2680 entries, including 2054 apoptosis, 619 autophagy-associated (340 experimentally validated entries and 279 predicted entries) and 7 necrosis-associated entries. Apoptosis-associated entries account for 358 miRNAs, 71 lncRNAs, 6 snoRNA, and 558 proteins, while autophagy-associated entries account for 199miRNAs, 4 lncRNA, and 99 proteins.
Table 1. Statistics of ncRNA-associated cell death entries in ncRDeathDB
ncRDeathDB also provides 4 options on the “Help” page to provide instructions for using the database. These include “Tutorial:” procedure and illustrations of the database; “Source:” sources of ncRNAs and gene information, and tools used in the database; “Parameter:” details the parameters for tools used for binding site prediction and ‘Error Report’. In the “Download & API” page, users can download all data in Microsoft Excel and TXT format or access the application programming interface (API) using scripts. In the ‘Submit’ page, ncRDeathDB invites users to submit novel ncRNA-associated cell death interactions.
Data querying, searching, browsing, and visualization
ncRDeathDB provides an interface for convenient retrieval of all interactions. Users can search each ncRNA-associated cell death interaction through 3 paths (), including ‘By keyword’: browse the ncRNA-associated cell death entries by inputting the keywords (any ncRNA, Gene Symbol, Death Pathway, Species) and fuzzy search supported, ‘By Death Pathway’: represent all ncRNA-associated cell death entries by associated option of species and death pathway, and ‘By Advanced Search’: represent the accurate search results by associated option of species, death pathway and ncRNA/Gene symbols exist in ncRDeathDB. Brief details of search results are presented as a table in the ‘Results’ page, while more detailed descriptions such as PubMed ID and description of the reference are displayed in the ‘Detail’ page. The ‘Browse page’ enables users to browse the database in 3 different ways: ‘Browse by ncRNA category’, ‘Browse by species’ and ‘Browse by cell death pathway’. All of the ncRNA-associated cell death interaction information is presented by selecting on each entry.
Figure 2. A flowchart for retrieving ncRNA-associated cell death interaction entry. (A) Three searching paths for retrieving the ncRNA-mediated cell death system. (B) The result of a representative database entry. (C) The detailed information for an ncRNA-associated cell death entry. In the results and detail pages, ncRDeathDB linked each ncRNA/protein to their corresponding detail pages and PMID to NCBI PubMed database.
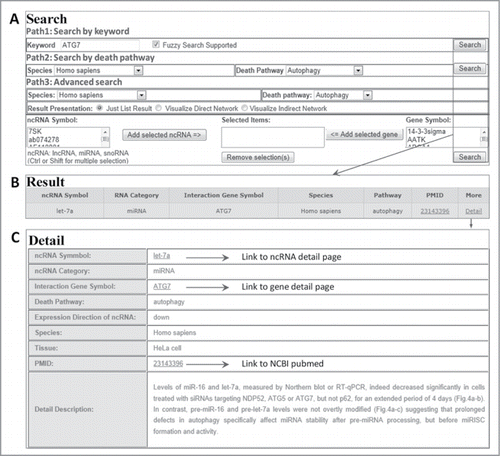
Topological characterization of the human ncRNA-mediated cell death system
In the current version of ncRDeathDB, nearly 60% of the entries included were for human ncRNA-associated cell death subroutines. Hence, in this work, we mainly focus on the largest human ncRNA-mediated cell death system by using experimentally validated entries to investigate the generic organizing principles of ncRNA-mediated cell death systems. According to experimentally validated entries, the human ncRNA-mediated cell death system consists of 1715 interactions and 951 nodes, including 1453 apoptosis and 262 autophagy-associated interactions. Using several bioinformatics network techniques, we first identified the hub and bottleneck nodes of the network (, Table S1). Typical examples, such as the hub nodes BCL2 and MIR21 and the bottleneck node MIR181A (reported in our previous work,Citation10,12) were validated for the current network. In addition, we have identified several newly discovered partner genes of MIR181A, which include apoptotic genes (CASP3, CASP9, BAX, FAS, etc) and autophagic genes (ATG10, BECN1, ATG16L1, ATG12, UVRAG, etc). This finding has further strengthened the role of MIR181A in mediating the crosstalk between apoptosis and autophagy.Citation12
Figure 3. Schematic diagram for network measurements used to decipher the ncRNA-mediated cell death system. (A) A schematic diagram represents one bottleneck node, 2 hub nodes and one pivot node. Hubs are highly connected to other nodes in the network and act as the center point within the modules or subnetworks. Evidence from model organisms indicates that hub proteins tend to be encoded by essential genes, and the deletion of hub nodes leads to amount of diseases. Bottlenecks are defined as proteins with a high betweenness that are central to many paths in the network, and play a key role in controlling network signaling, and also like to be essential proteins, and often function as key genes in the development of disease progression. Pivots correspond to significantly shared members of 2 different pathways or subnetworks, which potentially have a role as molecular switches between these pathways or subnetworks, and further sheds light on the organization of the cellular machinery. (B) Schematic description of the 3-core decomposition of a network. k-core is a subnetwork defined by iteratively removing the nodes with a degree lower than k and their incident links. The inner k-cores were shown to be global hubs and enriched with lethal genes, which may potentially act as the evolutionarily conserved backbone of ncRNA-mediated cell death system.
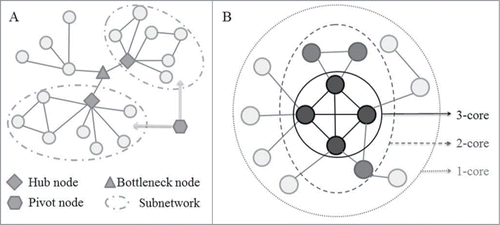
Furthermore, novel hubs have been identified, including MCL1, a member of the BCL2 family located in mitochondria, the different isoforms of which play a role in the regulation of apoptosis.Citation13,14 In the human ncRNA-associated cell death system, MCL1 interacts with 25 ncRNAs, including important hub ncRNAs such as MIR181A and MIR125B. TP53 is another interesting hub; in addition to interacting with several miRNA partners, it interacts with multiple lncRNAs, including H19, PANDAR, LINC-ROR and MEG3, suggesting that it plays a key role in linking lncRNAs with other ncRNAs.Citation15-18 Furthermore, we identified several novel ncRNAs as bottlenecks that bridge different cell death subroutines (Table S1). For example, MIR34A not only regulates key apoptotic genes such as BCL2, TP53, and members of the CDK family, but also interacts with ATG9, which is involved in autophagy.Citation19-22
Hierarchy of human ncRNA-mediated cell death system
In nature, hierarchical network organization has been found in both prokaryotic and eukaryotic regulatory networks.Citation23-25 Hence, we applied the k-core algorithm to decipher whether the human ncRNA-mediated cell death system is also hierarchically ordered (). This network is parsed into 5 detailed layers, and the nodes contained in each layer are 951 (layer 1), 394 (layer 2), 225 (layer 3), 150 (layer 4) and 73 (layer 5). Several established key cell death protein clusters, such as the autophagy-associated ‘ATG10 ATG7 ATG5 ATG12 ATG3 MAP1LC3B ATG4B ATG16L1’ cluster and the apoptosis-associated ‘MOAP1 BCL2L1 BCL2 BAX PMAIP1 MCL1 BID BBC3 BIK BAK1 BMF ERN1’ cluster appear in the innermost layer (4 and 5-layer), suggesting that the components in this layer are essential for normal cellular function.
To investigate the role of ncRNAs in the cell death system, we determined the fraction of ncRNAs at each layer, 345/951 (36.28%) in layer 1, 193/394 (48.98%) in layer 2, 127/225 (56.44%) in layer 3, 85/150 (56.67%) in layer 4, and 40/73 (54.79%) in layer 5. Some ncRNAs and proteins can interact with both canonical apoptotic and autophagy partners. Such dual functional molecules may play a signal transmission role in the cell death system. Interestingly, our results have shown that the number of dual ncRNAs and proteins within each layer is 158/951 (16.61%) in layer 1, 128/394 (32.49%) in layer 2, 94/225 (41.78%) in layer 3, 71/150 (47.33%) in layer 4, and 38/73 (52.05%) in layer 5, and that the percentage of dual ncRNAs and proteins increases dramatically with the k-value. Due to the low statistical power of detecting significant differences in the relatively rareness nodes (small samples) in 4- and 5-layer, thus, we define layer 1 as the outmost layer, the combined layers 2 and 3 as the middle layer and layers 4 and 5 as the core layer. According to hypergeometric test, our results showed that the percentage of dual ncRNAs and proteins increases significantly with an increasing k-value in the outmost layer vs. the middle layer (P < 0.01) and the middle layer vs. the core layer (P < 0.01),suggesting that many dual ncRNAs and proteins are located in the core layer of the cell death system and therefore play a vital role. These data also validated the existence of a tight crosstalk between apoptosis and autophagy at the molecular level. Furthermore, this crosstalk was mainly executed by the backbone of the cell death system rather than other molecules playing auxiliary roles.
The ncRNA-mediated cell death system in human protein-protein and ncRNA-protein networks
To investigate the biological significance of the ncRNA-mediated cell death system in the context of the global human regulation network, we mapped cell death-associated ncRNAs and proteins to the ncRNA-protein interaction (NPI) network and the human protein-protein interaction (PPI) network. represents the degree distribution of cell death-associated ncRNAs and proteins in both the NPI and PPI networks. It is clear that the cell death-associated ncRNAs and proteins especially the dual nodes have a significantly higher degree distribution (P < 0.01, hypergeometric test) than that of the whole nodes in the NPI and PPI networks, suggesting that cell death-associated ncRNAs and proteins act as hubs in these global networks. Dual ncRNAs and proteins have the highest degree distribution, reflective of not only the key role played by dual functional molecules in the crosstalk between cell death subroutines, but also their roles as core elements in the human PPI and NPI network and as the foundation of signal transduction.
Figure 4. Distribution of degrees of apoptosis and autophagy-associated ncRNAs and proteins in the global human NPI and PPI network. The boxplot represents the degree distribution. The cell death-associated ncRNAs and proteins especially the dual nodes have a significantly higher degree distribution than that of the whole nodes in the NPI and PPI network.
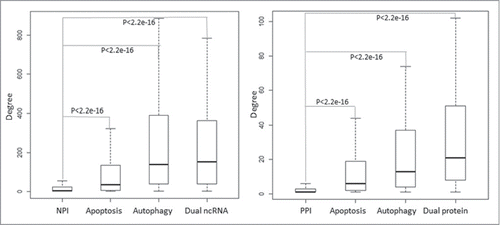
Global human NPI and PPI networks are also hierarchically structured.Citation26-28 As shown in , our results show that the percent of cell death-associated ncRNAs and proteins at each layer increased as k increased, and the dual proteins and ncRNAs involved in both autophagy and apoptosis increased the fastest. These results suggest that cell death-associated ncRNAs and proteins, especially dual-functional ncRNAs and proteins, mainly localized to the core layer of both the NPI and PPI networks. In summary, our results demonstrated that cell death-associated ncRNAs and proteins were involved in the basic construction of NPI and PPI networks.
Figure 5. Analysis of network centricity of cell death-associated ncRNA and protein in global the human NPI and PPI network. The percent of both cell death-associated ncRNAs and proteins in k-cores of the NPI and PPI network significantly increased as k increased, and the dual proteins and ncRNAs involved in both autophagy and apoptosis increased the fastest.
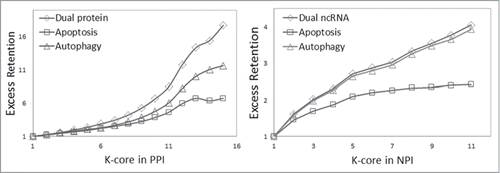
Identifying the pivot ncRNAs regulating the human autophagy subnetwork
One recent study extracted a high-confidence candidate “Autophagy-Interaction Network” (AIN) using the Comparative Proteomics Analysis Software Suite (CompPASS).Citation29 This protein autophagy network, which is composed of 409 candidate interacting proteins with 751 interactions and 8 subnetworks, has provided a resource for the mechanistic analysis of autophagy. Because of the sparseness of autophagy-associated genes with defined regulation by ncRNAs, some of genes defined in these subnetworks cannot be found in our data. However, several important autophagy subnetworks, such as the ULK1 kinase network, the Human network of orthologs and paralogs of yeast Atg8, the UBL conjugation system and the ATG2-WIPI subnetwork, can be mapped to our ncRNA-mediated cell death system. To identify the ncRNAs regulating these subnetworks, we performed a hypergeometric test for each subnetwork and identified 13 miRNAs and 1 lncRNA that have significant P values (). A total of 9 miRNAs (MIR519A, MIR374A, MIR20A, MIR630, MIR30C1, MIR181A, MIR130A, MIR30D, and MIRLET7A1) tightly control the UBL conjugation system through regulating some autophagic genes, such as ATG5, ATG12, and ATG16L1. Similarly, 3 miRNAs (MIR26B, MIR30A and MIR335) tightly control the human network of orthologs and paralogs of yeast Atg8, through regulating some autophagic genes, such as MAP1LC3B. More interesting, BANCR as a lncRNA was also involved in this network by targeting the MAP1LC3A and MAP1LC3B.
Table 2. ncRNAs are significant for the regulation of human protein autophagy subnetworks
Identifying pivot ncRNAs that regulate the human cell death modules
To extract pivot ncRNAs from the global cell death network (), the human cell death protein interaction network was extracted based on the PPI network, and both nodes of each interaction were documented in ncRDeathDB, consisting of 365 proteins and 1172 edges. Then, we used the ClusterONE plugin in Cytoscape to delineate potential modules in the cell death protein interaction network and identified 17 such modules (Table S2). Some of these modules indeed coincided with several experimentally validated protein complexes.Citation30 For example, the module ‘FASN FADD CASP8AP2 CASP8 TNFRSF10B FAF1 PEA15’ significantly contains the protein complex ‘Death-inducing signaling complex DISC associated with type I cells’ and the ‘FAS-FADD-CASP8-CASP10 complex’ (P<0.01, hypergeometric test), while the module ‘MOAP1 BCL2L1 BCL2 BAX PMAIP1 MCL1 BID BBC3 BIK BAK1 BMF ERN1’ mostly consisted of BCL2 family proteins, which play an influential role in both apoptosis and autophagy. Furthermore, some of the modules are highly consistent with the subnetworks defined in the ‘Autophagy-Interaction Network’. For example, the protein cluster “ATG10 ATG7 ATG5 ATG12 ATG3 MAP1LC3B ATG4B ATG16L1” is part of the ‘UBL conjugation system’ defined in AIN (P<0.01, hypergeometric test). All of these lines of evidence indicate that the modules identified by ClusterONE overlap significantly with existing protein complexes in the cell death subnetwork. Based on this module construction, we also uncovered a panel of ncRNAs that are extensively linked with these modules via a hypergeometric test (, and Table S3).Our results showed that these ncRNAs can easily be classified into 2 categories, one of which is located within a given module and regulates most of the proteins in the module. For example, MDM2-AS, MIR197 and MIR320A only target the BCL2-associated module, while MIR374A, MIR30A and MIR519A only target the module ‘ATG10 ATG7 ATG5 ATG12 ATG3 MAP1LC3B ATG4B ATG16L1’. Another category of ncRNAs acts as a bottleneck to bridging the different modules. The most significant discovery is that MIR181A simultaneously regulate modules ‘ATG10 ATG7 ATG5 ATG12 ATG3 MAP1LC3B ATG4B ATG16L1’ and ‘MOAP1 BCL2L1 BCL2 BAX PMAIP1 MCL1 BID BBC3 BIK BAK1 BMF ERN1’; while, the first module as the member of ‘UBL conjugation system,’ plays an important role in regulating autophagy, the latter consists of key proteins involved in apoptosis, such as BCL2, MCL1 and BIK. What's more, MIR181A is located in the centermost subnetwork of the cell death network, suggesting that MIR181A plays a critical role in the regulation of cell death by functioning in both apoptosis and autophagy.
Figure 6. Example of a module significantly regulated by ncRNAs identified in the human cell death protein interaction network. Pink and blue nodes represent apoptosis and autophagy, respectively. Yellow nodes represent the ncRNAs and proteins that play dual roles in apoptosis and autophagy. Triangular, arrowhead-shaped and circular nodes represent cell death-associated miRNAs, lncRNAs and proteins, respectively.

Table 3. Statistics of top 10 significant ncRNAs regulating human protein cell death modules
Finally, significant regulatory relationships between ncRNAs and their target modules are shown in . Apart from miRNA modulators, the novel lncRNA MALAT1 was also found to play an important role in cell death. It targets the module “MOAP1 BCL2L1 BCL2 BAX PMAIP1 MCL1 BID BBC3 BIK BAK1 BMF ERN1” and module “BCL2L1 BCL2 CASP3 CTNNB1 CASP8 PSEN1 TFAP2A APAF1,” which have a high overlap with the apoptotic protein complex ‘MCL1-BAK1 complex’ and “MRIT complex” respectively (P<0.01, hypergeometric test) (, and Table S3). These ncRNAs seem to act as messengers or coordinators in mediating the crosstalk between the different subroutines of PCD.
Discussion
In recent years, great efforts have been made to uncover new forms of mammalian programmed cell death.Citation1,31-34 Emerging work has focused on the network organization of cell death systems with the ultimate goal of exploring potential organization mechanisms from a global view of the Protein-Protein Interaction (PPI) network.Citation29,35 Consequently, accumulated ncRNAs and proteins have been identified in a variety of cell death subroutines.Citation10,29,36-38 It is now widely accepted that both ncRNAs and proteins are intimately connected with each other in a global cell death network. Of course, the PPI network in different cell death subroutines is only part of the story. Hence, 2 and half y ago, we established the miRDeathDB database to archive these resources and provide a basis for the analysis of miRNA-mediated cell death systems.Citation11,12 With the rapid development of this field, this is the perfect time to integrate recent findings and update information. We therefore developed ncRDeathDB (www.rna-society.org/ncrdeathdb), which documents by nearly 20-fold the data of the previous miRDeathDB database and includes diverse ncRNA-mediated cell death interactions, such as those involving miRNA, lncRNA, and snoRNA. ncRDeathDB is a good starting point for searching the web resources centered on diverse ncRNAs involved in PCD. More importantly, ncRDeathDB provides a valuable resource to manipulate, visualize, and analyze these ncRNA-associated cell death interactions. By integrating the ncRNA-associated cell death interactions into a global network, this resource will help to visualize and navigate current knowledge of the noncoding RNA component of cell death and autophagy, to uncover the generic organizing principles of ncRNA-associated cell death systems, and to generate valuable biological hypothesis. In ncRDeathDB, we present a systematic ncRNA-associated analysis of the human cell death systems by using experimentally validated entries. Such analysis has provided another view of the global organization of the ncRNA-associated cell death system and the potential mechanisms involved in the cell death process. First, we presented a network of 1715 human ncRNA-associated interactions (1453 apoptosis- and 262 autophagy-associated interactions) and 951 nodes (300 miRNAs, 40 lncRNAs, 1 snoRNA and 610 proteins) with extensive connectivity among different modules.Similarly, our previously reported hubs (BCL2 and MIR21) and bottleneck (MIR181A) were validated in this extended network with newly discovered partner ncRNAs and proteins, thus strengthening their roles in mediating the crosstalk between apoptosis and autophagy. More importantly, novel key hubs and bottlenecks have been explored that interact with diverse and important ncRNAs, especially multiple lncRNAs, to regulate communication with intra- and inter- autophagic and apoptotic modules. Hence, these diverse ncRNA dependent mechanisms may represent a basic mechanism for the conversation between autophagic and apoptotic death modules, leading to cellular homeostasis upon the presentation of external stimuli.
Next, to investigate the global center of ncRNAs and proteins involved in PCD, the ncRNA-associated cell death system was hierarchically divided into 5 fine layers using the k-core algorithm. Our results explored the idea that both dual functional ncRNAs and proteins tended to reside in the most inner layer of this system and that these molecules were tightly connected with each other and acted as the evolutionary backbone of the proteome. Furthermore, we investigated the relationship between the ncRNA-mediated cell death system and the canonical signal transduction process. For both protein-protein (PPI) and ncRNA-protein (NPI) interaction networks, our results demonstrated that the number of cell death-associated ncRNAs and proteins was significantly larger in the inner layer than in the outer layer. Dual proteins and ncRNAs involved in both autophagy and apoptosis displayed this tendency, suggesting that cell death-associated ncRNAs and proteins were encoded into the basic function of cells. Under stress or other urgent conditions, these dual proteins and ncRNAs could reprogram cell fate by choosing different apoptosis or autophagy pathways to adapt to the environment. Finally, in an experimentally verified autophagy module, we observed that some cell death-associated ncRNAs tightly control different autophagy subnetworks by regulating shared key genes. Similarly, in a cell death network (including both autophagy and apoptosis modules), our results identified several key ncRNA players and their protein targets, which mediate communication between different cell death modules. The aforementioned ncRNA-based intra- and inter-modulators are likely to be good candidates for pharmaceutical development in the near future.
In summary, our studies provide insight into the organization of the human ncRNA-mediated cell death system and decipher diverse ncRNA-dependent mechanisms as a basic component of the crosstalk between apoptosis and autophagy. In the future, with the identification of more necrosis-associated ncRNA, our database will serve as a more comprehensive ncRNA-associated resource for further mechanistic analysis of programmed cell death.
Materials and Methods
Data collection and web interface implementation
To collect all available ncRNA-associated entries involved in programmed cell death, we have integrated information on 3 major types of ncRNAs: long noncoding RNA (lncRNA) symbols from lncRNAdbCitation39 and Ensembl,Citation40 miRNA symbols from mirBase database,Citation41 and small nucleolar RNA (snoRNA) symbols from sno/scaRNAbaseCitation42 and snoRNA-LBME-db.Citation43 Because the research on other categories of ncRNAs is still in its infancy and there is no unified nomenclature, we instead use these ncRNA category names to replace specific ncRNA symbols. All abstracts and articles in the PubMed database were screened using the following keyword combinations: (each ncRNA symbol or ncRNA category) and (each cell death subroutines); for example, “malat1 and apoptosis.” The relevant hits were downloaded and prepared systematically for data curation by further manual inspection. Apart from the information regarding ncRNAs and the corresponding interacting genes, species and detailed description compiled from the references, we incorporate several useful tools to predict the potential binding sites of these ncRNA-associated cell death interactions. For ncRNA-RNA interactions, the binding sites and scores are predicted according to miRandaCitation44 and RISearch.Citation45 For ncRNA-protein interactions, RNA-binding residues and scores are computed by bindN+Citation46 and RNAbindR.Citation47 In addition, to help users interactively analyze the ncRNA-mediated cell death system online, ncRDeathDB provides an embedded Cytoscape web tool (cytoscapeweb.cytoscape.org/).
The ncRDeathDB database is implemented in the HTML and PHP languages with a MySQL server. The interface component consists of webpages designed and implemented in HTML/CSS in a Microsoft Windows environment. It has been tested in Google Chrome, Firefox and Internet Explorer web browsers.
Autophagy-associated entry prediction
Autophagy-associated miRNAs and their protein targets were predicted by using homologs search based on the experimentally validated autophagy-associated entries in ncRDeathDB. The conserved miRNAs families were identified by TargetScan.Citation48 The homological genes of human autophagy-associated genes were downloaded from NCBI.Citation49 Then, the interactions between conserved miRNAs and homological genes verified by TargetScanCitation48,50,51 were filtered as the potential autophagy-associated miRNA-protein pairs and assigned for other species.
Protein-protein interaction network and RNA-associated network construction
In this work, the global human protein-protein interaction(PPI) network was retrieved from the Human Protein Reference Database (HPRD),Citation52 and the human ncRNA protein interaction network(NPI) was retrieved from our RNA-Associated Interaction Database (RAID)Citation53 and miRTarBase,Citation54 in which the interactions have been validated by experiments and covered diverse ncRNA species, such as lncRNA and miRNA.
Degree and betweenness analysis of the human interaction network
The network was analyzed based on topological parameters such as betweenness and degree using a Cytoscape plug-in called ‘Network Analyzer.’Citation55 In a biological network, degree indicates the number of edges linked to a given node (). Betweenness measures the total number of nonredundant shortest paths going through a certain node or an edge, reflecting the bottleneck of the network () The nodes with a top 5% highest degree and betweenness were chosen as the hubs and bottlenecks in this work (Table S1). The hub and bottleneck nodes in the network usually play a key role in connecting the intra- and inter-modules or pathways.Citation56
K-core analysis of interaction network
The k-core of a network is defined as the subnetwork in which all of its node degrees are greater than k after recursively removing the nodes with a smaller degree than k ().Citation28 One can iteratively apply this ‘peeling’ procedure to divide the whole network into different layers, and the genes in layer k of the network are defined as the intersection between core k and core k+1. In this work, the human ncRNA-mediated cell death system has been parsed into 5 layers according to this ‘peeling’ procedure.
To measure the centrality of cell death-associated ncRNAs and proteins, the Excess Retention (ER) was calculated for each k-core layer of the NPI and PPI networks. ER is defined as the proportion of genes with a certain property A in the ‘k-core’ compared with that in the whole gene groups.Citation57 For instance, in a k-core with a total of Nk nodes, the proportion of protein with a property A is defined as Obviously, the fraction of proteins with property A in the whole network with a total N nodes is defined aserA = nA/N, and the ER of k-core is defined as
. In this work, we set the cell death-associated ncRNAs and proteins as the property A in ER, and then counted the percent of cell death-associated ncRNAs and proteins in each layer of the NPI and PPI networks. Thus, the ER value reflects the centrality of cell death-associated ncRNAs and proteins in human PPI and NPI networks.
Module identification and pivot ncRNA recognition
In this work, the human cell death protein interaction network was extracted based on the PPI network, all nodes of which were documented in ncRDeathDB. To identify functional modules in the human cell death protein interaction network, the network was clustered with the ClusterONE algorithmsCitation58 available in Cytoscape.Citation59 ClusterONE is a method of detecting the protein complex module based on the PPI network by iteratively adding or removing the most valuable incident and boundary nodes to locally optimize the cluster quality measure cohesiveness.Citation58
The pivot ncRNAs (), which significantly regulate different modules and subnetworks, are recognized by a hypergeometric test.Citation60 The P value of the test statistic is calculated as:
Where N is the total number of proteins contained in the human cell death protein interaction network, n represents the number of interacted genes of a certain ncRNA. M represents the number of genes belonging to a module, while i represents the genes in the modules interacted with ncRNA.
Disclosure of Potential Conflicts of Interest
No potential conflicts of interest were disclosed.
1089375_Supplemental_Material.zip
Download Zip (92.1 KB)Acknowledgment
We are grateful to Dr. Daniel J. Klionsky for help in editing the manuscript.
Supplemental Material
Supplemental data for this article can be accessed on the publisher's website
Funding
This work was supported by the National High Technology Research and Development Program of China (2014AA021102), the Major State Basic Research Development Program of China (2014CB910504), the National Natural Science Foundation of China (U1304311), the Natural Science Foundation of Heilongjiang Province of China (C2015027), the Scientific Research Fund of Heilongjiang Provincial Education Department (12541426).
References
- Ambros V. The functions of animal microRNAs. Nature 2004; 431:350-5; PMID:15372042; http://dx.doi.org/10.1038/nature02871
- DeOcesano-Pereira C, Amaral MS, Parreira KS, Ayupe AC, Jacysyn JF, Amarante-Mendes GP, Reis EM, Verjovski-Almeida S. Long non-coding RNA INXS is a critical mediator of BCL−XS induced apoptosis. Nucleic Acids Res 2014; 42:8343-55; PMID:24992962; http://dx.doi.org/10.1093/nar/gku561
- Michel CI, Holley CL, Scruggs BS, Sidhu R, Brookheart RT, Listenberger LL, Behlke MA, Ory DS, Schaffer JE. Small nucleolar RNAs U32a, U33, and U35a are critical mediators of metabolic stress. Cell Metab 2011; 14:33-44; PMID:21723502; http://dx.doi.org/10.1016/j.cmet.2011.04.009
- Sun M, Liu XH, Lu KH, Nie FQ, Xia R, Kong R, Yang JS, Xu TP, Liu YW, Zou YF, et al. EZH2-mediated epigenetic suppression of long noncoding RNA SPRY4-IT1 promotes NSCLC cell proliferation and metastasis by affecting the epithelial-mesenchymal transition. Cell Death Dis 2014; 5:e1298; PMID:24967960; http://dx.doi.org/10.1038/cddis.2014.256
- Wang Y, Chen L, Chen B, Li X, Kang J, Fan K, Hu Y, Xu J, Yi L, Yang J, et al. Mammalian ncRNA-disease repository: a global view of ncRNA-mediated disease network. Cell Death Dis 2013; 4:e765; PMID:23928704; http://dx.doi.org/10.1038/cddis.2013.292
- Gibbings D, Mostowy S, Voinnet O. Autophagy selectively regulates miRNA homeostasis. Autophagy 2013; 9:781-3; PMID:23422216; http://dx.doi.org/10.4161/auto.23694
- Hwang HW, Mendell JT. MicroRNAs in cell proliferation, cell death, and tumorigenesis. Br J Cancer 2007; 96 Suppl:R40-4; PMID:17393584
- Hung T, Wang Y, Lin MF, Koegel AK, Kotake Y, Grant GD, Horlings HM, Shah N, Umbricht C, Wang P, et al. Extensive and coordinated transcription of noncoding RNAs within cell-cycle promoters. Nat Genet 2011; 43:621-9; PMID:21642992; http://dx.doi.org/10.1038/ng.848
- Eisenberg-Lerner A, Bialik S, Simon HU, Kimchi A. Life and death partners: apoptosis, autophagy and the cross-talk between them. Cell Death Differ 2009; 16:966-75; PMID:19325568; http://dx.doi.org/10.1038/cdd.2009.33
- Xu J, Wang Y, Tan X, Jing H. MicroRNAs in autophagy and their emerging roles in crosstalk with apoptosis. Autophagy 2012; 8:873-82; PMID:22441107; http://dx.doi.org/10.4161/auto.19629
- Xu J, Li YH. miRDeathDB: a database bridging microRNAs and the programmed cell death. Cell Death Differ 2012; 19:1571; PMID:22743998; http://dx.doi.org/10.1038/cdd.2012.87
- Li Y, Zhuang L, Wang Y, Hu Y, Wu Y, Wang D, Xu J. Connect the dots: a systems level approach for analyzing the miRNA-mediated cell death network. Autophagy 2013; 9:436-9; PMID:23322033; http://dx.doi.org/10.4161/auto.23096
- Inuzuka H, Shaik S, Onoyama I, Gao D, Tseng A, Maser RS, Zhai B, Wan L, Gutierrez A, Lau AW, et al. SCF(FBW7) regulates cellular apoptosis by targeting MCL1 for ubiquitylation and destruction. Nature 2011; 471:104-9; PMID:21368833; http://dx.doi.org/10.1038/nature09732
- Day CL, Chen L, Richardson SJ, Harrison PJ, Huang DC, Hinds MG. Solution structure of prosurvival Mcl−1 and characterization of its binding by proapoptotic BH3-only ligands. J Biol Chem 2005; 280:4738-44; PMID:15550399; http://dx.doi.org/10.1074/jbc.M411434200
- Kim K, Jutooru I, Chadalapaka G, Johnson G, Frank J, Burghardt R, Kim S, Safe S. HOTAIR is a negative prognostic factor and exhibits pro-oncogenic activity in pancreatic cancer. Oncogene 2013; 32:1616-25; PMID:22614017; http://dx.doi.org/10.1038/onc.2012.193
- Yang F, Bi J, Xue X, Zheng L, Zhi K, Hua J, Fang G. Up-regulated long non-coding RNA H19 contributes to proliferation of gastric cancer cells. FEBS J 2012; 279:3159-65; PMID:22776265; http://dx.doi.org/10.1111/j.1742-4658.2012.08694.x
- Zhang A, Zhou N, Huang J, Liu Q, Fukuda K, Ma D, Lu Z, Bai C, Watabe K, Mo YY. The human long non-coding RNA-RoR is a p53 repressor in response to DNA damage. Cell Res 2013; 23:340-50; PMID:23208419; http://dx.doi.org/10.1038/cr.2012.164
- Zhou Y, Zhang X, Klibanski A. MEG3 noncoding RNA: a tumor suppressor. J Mol Endocrinol 2012; 48:R45-53; PMID:22393162; http://dx.doi.org/10.1530/JME-12-0008
- Di Martino MT, Leone E, Amodio N, Foresta U, Lionetti M, Pitari MR, Cantafio ME, Gullà A, Conforti F, Morelli E, et al. Synthetic miR-34a mimics as a novel therapeutic agent for multiple myeloma: in vitro and in vivo evidence. Clin Cancer Res 2012; 18:6260-70; PMID:23035210; http://dx.doi.org/10.1158/1078-0432.CCR-12-1708
- Hu QL, Jiang QY, Jin X, Shen J, Wang K, Li YB, Xu FJ, Tang GP, Li ZH. Cationic microRNA-delivering nanovectors with bifunctional peptides for efficient treatment of PANC-1 xenograft model. Biomaterials 2013; 34:2265-76; PMID:23298779; http://dx.doi.org/10.1016/j.biomaterials.2012.12.016
- Rucker FG, Russ AC, Cocciardi S, Kett H, Schlenk RF, Botzenhardt U, Langer C, Krauter J, Fröhling S, Schlegelberger B, et al. Altered miRNA and gene expression in acute myeloid leukemia with complex karyotype identify networks of prognostic relevance. Leukemia 2013; 27:353-61; PMID:22810507; http://dx.doi.org/10.1038/leu.2012.208
- Frankel LB, Lund AH. MicroRNA regulation of autophagy. Carcinogenesis 2012; 33:2018-25; PMID:22902544; http://dx.doi.org/10.1093/carcin/bgs266
- Cosentino Lagomarsino M, Jona P, Bassetti B, Isambert H. Hierarchy and feedback in the evolution of the Escherichia coli transcription network. Proc Natl Acad Sci U S A 2007; 104:5516-20; PMID:17372223; http://dx.doi.org/10.1073/pnas.0609023104
- Gerstein MB, Kundaje A, Hariharan M, Landt SG, Yan KK, Cheng C, Mu XJ, Khurana E, Rozowsky J, Alexander R, et al. Architecture of the human regulatory network derived from ENCODE data. Nature 2012; 489:91-100; PMID:22955619; http://dx.doi.org/10.1038/nature11245
- Yu H, Gerstein M. Genomic analysis of the hierarchical structure of regulatory networks. Proc Natl Acad Sci U S A 2006; 103:14724-31; PMID:17003135; http://dx.doi.org/10.1073/pnas.0508637103
- Cheng C, Yan KK, Hwang W, Qian J, Bhardwaj N, Rozowsky J, Lu ZJ, Niu W, Alves P, Kato M, et al. Construction and analysis of an integrated regulatory network derived from high-throughput sequencing data. PLoS Comput Biol 2011; 7:e1002190; PMID:22125477; http://dx.doi.org/10.1371/journal.pcbi.1002190
- Ravasz E, Barabasi AL. Hierarchical organization in complex networks. Phys Rev E Stat Nonlin Soft Matter Phys 2003; 67:026112; PMID:12636753; http://dx.doi.org/10.1103/PhysRevE.67.026112
- Wuchty S, Almaas E. Peeling the yeast protein network. Proteomics 2005; 5:444-9; PMID:15627958; http://dx.doi.org/10.1002/pmic.200400962
- Behrends C, Sowa ME, Gygi SP, Harper JW. Network organization of the human autophagy system. Nature 2010; 466:68-76; PMID:20562859; http://dx.doi.org/10.1038/nature09204
- Ruepp A, Waegele B, Lechner M, Brauner B, Dunger-Kaltenbach I, Fobo G, Frishman G, Montrone C, Mewes HW. CORUM: the comprehensive resource of mammalian protein complexes–2009. Nucleic Acids Res 2010; 38:D497-501; PMID:19884131; http://dx.doi.org/10.1093/nar/gkp914
- Bartel DP. MicroRNAs: genomics, biogenesis, mechanism, and function. Cell 2004; 116:281-97; PMID:14744438; http://dx.doi.org/10.1016/S0092-8674(04)00045-5
- Matera AG, Terns RM, Terns MP. Non-coding RNAs: lessons from the small nuclear and small nucleolar RNAs. Nat Rev Mol Cell Biol 2007; 8:209-20; PMID:17318225; http://dx.doi.org/10.1038/nrm2124
- Mercer TR, Dinger ME, Mattick JS. Long non-coding RNAs: insights into functions. Nat Rev Genet 2009; 10:155-9; PMID:19188922; http://dx.doi.org/10.1038/nrg2521
- Wang KC, Chang HY. Molecular mechanisms of long noncoding RNAs. Mol Cell 2011; 43:904-14; PMID:21925379; http://dx.doi.org/10.1016/j.molcel.2011.08.018
- Kang R, Zeh HJ, Lotze MT, Tang D. The Beclin 1 network regulates autophagy and apoptosis. Cell Death Differ 2011; 18:571-80; PMID:21311563; http://dx.doi.org/10.1038/cdd.2010.191
- Galluzzi L, Vitale I, Abrams JM, Alnemri ES, Baehrecke EH, Blagosklonny MV, Dawson TM, Dawson VL, El-Deiry WS, Fulda S, et al. Molecular definitions of cell death subroutines: recommendations of the Nomenclature Committee on Cell Death 2012. Cell Death Differ 2012; 19:107-20; PMID:21760595; http://dx.doi.org/10.1038/cdd.2011.96
- Marino G, Niso-Santano M, Baehrecke EH, Kroemer G. Self-consumption: the interplay of autophagy and apoptosis. Nat Rev Mol Cell Biol 2014; 15:81-94; PMID:24401948; http://dx.doi.org/10.1038/nrm3735
- Vanden Berghe T, Linkermann A, Jouan-Lanhouet S, Walczak H, Vandenabeele P. Regulated necrosis: the expanding network of non-apoptotic cell death pathways. Nat Rev Mol Cell Biol 2014; 15:135-47; PMID:24452471; http://dx.doi.org/10.1038/nrm3737
- Quek XC, Thomson DW, Maag JL, Bartonicek N, Signal B, Clark MB, Gloss BS, Dinger ME. lncRNAdb v2.0: expanding the reference database for functional long noncoding RNAs. Nucleic Acids Res 2015; 43:D168-73; PMID:25332394; http://dx.doi.org/10.1093/nar/gku988
- Flicek P, Amode MR, Barrell D, Beal K, Billis K, Brent S, Carvalho-Silva D, Clapham P, Coates G, Fitzgerald S, et al. Ensembl 2014. Nucleic Acids Res 2014; 42:D749-55; PMID:24316576; http://dx.doi.org/10.1093/nar/gkt1196
- Kozomara A, Griffiths-Jones S. miRBase: annotating high confidence microRNAs using deep sequencing data. Nucleic Acids Res 2014; 42:D68-73; PMID:24275495; http://dx.doi.org/10.1093/nar/gkt1181
- Xie J, Zhang M, Zhou T, Hua X, Tang L, Wu W. Sno/scaRNAbase: a curated database for small nucleolar RNAs and cajal body-specific RNAs. Nucleic Acids Res 2007; 35:D183-7; PMID:17099227; http://dx.doi.org/10.1093/nar/gkl873
- Lestrade L, Weber MJ. snoRNA-LBME-db, a comprehensive database of human H/ACA and C/D box snoRNAs. Nucleic Acids Res 2006; 34:D158-62; PMID:16381836; http://dx.doi.org/10.1093/nar/gkj002
- John B, Enright AJ, Aravin A, Tuschl T, Sander C, Marks DS. Human MicroRNA targets. PLoS Biol 2004; 2:e363; PMID:15502875; http://dx.doi.org/10.1371/journal.pbio.0020363
- Wenzel A, Akbasli E, Gorodkin J. RIsearch: fast RNA-RNA interaction search using a simplified nearest-neighbor energy model. Bioinformatics 2012; 28:2738-46; PMID:22923300; http://dx.doi.org/10.1093/bioinformatics/bts519
- Wang L, Huang C, Yang MQ, Yang JY. BindN+ for accurate prediction of DNA and RNA-binding residues from protein sequence features. BMC Syst Biol 2010; 4 Suppl 1:S3; PMID:20522253; http://dx.doi.org/10.1186/1752-0509-4-S1-S3
- Terribilini M, Sander JD, Lee JH, Zaback P, Jernigan RL, Honavar V, Dobbs D. RNABindR: a server for analyzing and predicting RNA-binding sites in proteins. Nucleic Acids Res 2007; 35:W578-84; PMID:17483510; http://dx.doi.org/10.1093/nar/gkm294
- Lewis BP, Shih IH, Jones-Rhoades MW, Bartel DP, Burge CB. Prediction of mammalian microRNA targets. Cell 2003; 115:787-98; PMID:14697198; http://dx.doi.org/10.1016/S0092-8674(03)01018-3
- Coordinators NR. Database resources of the National Center for Biotechnology Information. Nucleic Acids Res 2015; 43:D6-17; PMID:25398906; http://dx.doi.org/10.1093/nar/gku1130
- Grun D, Wang YL, Langenberger D, Gunsalus KC, Rajewsky N. microRNA target predictions across seven Drosophila species and comparison to mammalian targets. PLoS Comput Biol 2005; 1:e13; PMID:16103902; http://dx.doi.org/10.1371/journal.pcbi.0010013
- Lin CC, Mitra R, Zhao Z. A tri-component conservation strategy reveals highly confident microRNA-mRNA interactions and evolution of microRNA regulatory networks. PloS One 2014; 9:e103142; PMID:25054916; http://dx.doi.org/10.1371/journal.pone.0103142
- Keshava Prasad TS, Goel R, Kandasamy K, Keerthikumar S, Kumar S, Mathivanan S, Telikicherla D, Raju R, Shafreen B, Venugopal A, et al. Human Protein Reference Database–2009 update. Nucleic Acids Res 2009; 37:D767-72; PMID:18988627; http://dx.doi.org/10.1093/nar/gkn892
- Zhang X, Wu D, Chen L, Li X, Yang J, Fan D, Dong T, Liu M, Tan P, Xu J, et al. RAID: a comprehensive resource for human RNA-associated (RNA-RNA/RNA-protein) interaction. RNA 2014; 20:989-93; PMID:24803509; http://dx.doi.org/10.1261/rna.044776.114
- Hsu SD, Tseng YT, Shrestha S, Lin YL, Khaleel A, Chou CH, Chu CF, Huang HY, Lin CM, Ho SY, et al. miRTarBase update 2014: an information resource for experimentally validated miRNA-target interactions. Nucleic Acids Res 2014; 42:D78-85; PMID:24304892; http://dx.doi.org/10.1093/nar/gkt1266
- Assenov Y, Ramirez F, Schelhorn SE, Lengauer T, Albrecht M. Computing topological parameters of biological networks. Bioinformatics 2008; 24:282-4; PMID:18006545; http://dx.doi.org/10.1093/bioinformatics/btm554
- Yu H, Kim PM, Sprecher E, Trifonov V, Gerstein M. The importance of bottlenecks in protein networks: correlation with gene essentiality and expression dynamics. PLoS Computat Biol 2007; 3:e59; PMID:17447836; http://dx.doi.org/10.1371/journal.pcbi.0030059
- Wuchty S. Evolution and topology in the yeast protein interaction network. Genome Res 2004; 14:1310-4; PMID:15231746; http://dx.doi.org/10.1101/gr.2300204
- Nepusz T, Yu H, Paccanaro A. Detecting overlapping protein complexes in protein-protein interaction networks. Nat Methods 2012; 9:471-2; PMID:22426491; http://dx.doi.org/10.1038/nmeth.1938
- Shannon P, Markiel A, Ozier O, Baliga NS, Wang JT, Ramage D, Amin N, Schwikowski B, Ideker T. Cytoscape: a software environment for integrated models of biomolecular interaction networks. Genome Res 2003; 13:2498-504; PMID:14597658; http://dx.doi.org/10.1101/gr.1239303
- Ulitsky I, Shamir R. Pathway redundancy and protein essentiality revealed in the Saccharomyces cerevisiae interaction networks. Mol Syst Biol 2007; 3:104; PMID:17437029; http://dx.doi.org/10.1038/msb4100144