ABSTRACT
Exposure to particulate matter (PM) is recognized as a major health hazard, but molecular responses are still insufficiently described. We analyzed the epigenetic impact of ambient PM2.5 from biomass combustion on the methylome of primary human bronchial epithelial BEAS-2B cells using the Illumina HumanMethylation450 BeadChip. The transcriptome was determined by the Affymetrix HG-U133 Plus 2.0 Array. PM2.5 induced genome wide alterations of the DNA methylation pattern, including differentially methylated CpGs in the promoter region associated with CpG islands. Gene ontology analysis revealed that differentially methylated genes were significantly clustered in pathways associated with the extracellular matrix, cellular adhesion, function of GTPases, and responses to extracellular stimuli, or were involved in ion binding and shuttling. Differential methylations also affected tandem repeats. Additionally, 45 different miRNA CpG loci showed differential DNA methylation, most of them proximal to their promoter. These miRNAs are functionally relevant for lung cancer, inflammation, asthma, and other PM-associated diseases. Correlation of the methylome and transcriptome demonstrated a clear bias toward transcriptional activation by hypomethylation. Genes that exhibited both differential methylation and expression were functionally linked to cytokine and immune responses, cellular motility, angiogenesis, inflammation, wound healing, cell growth, differentiation and development, or responses to exogenous matter. Disease ontology of differentially methylated and expressed genes indicated their prominent role in lung cancer and their participation in dominant cancer related signaling pathways. Thus, in lung epithelial cells, PM2.5 alters the methylome of genes and noncoding transcripts or elements that might be relevant for PM- and lung-associated diseases.
Introduction
Exposure to ambient particulate matter (PM) is recognized as a major health hazard, associated with acute respiratory infections, lung cancer, and chronic respiratory or cardiovascular diseases.Citation1,Citation2 Whereas industrial and traffic-related pollutants have been extensively investigated, the number of studies evaluating the impact of air pollution resulting from burning biomass is limited.
Worldwide, biomass fuel represents about 10% of direct human energy consumption and will remain the main source of energy for most of humanity.Citation3-6 According to the World Health Organization, more than 3 billion people are affected by emissions from biomass fuels, claiming globally over 4 million deaths per year.Citation7 Epidemiologic and exposure studies have associated wild land fires, agricultural burning, and residential wood combustion with a variety of adverse respiratory health effects, including asthma, chronic obstructive lung disease, and acute lower respiratory tract infections, but also with cardiovascular effects and lung cancer.Citation3,Citation6 Thus, emissions from residential energy use have the largest impact on premature mortality worldwide.Citation5 The use of biomass fuels and wood burning is still expected to rise due to high fossil energy costs, the desire to use renewable, CO2-neutral energy sources, the rise in population, need for cropland, and climate changes.Citation3 Even in developed countries, residential biomass combustion is a major source of particle emissions.Citation3
Emissions from industrial or traffic related sources are essentially under legislation, but residential combustion of biomass fuels, agricultural burning, and forest fires are largely uncontrolled and may result in particle concentration up to 3 mg/m3 or even higher.Citation3,Citation8 Particles less than 2.5 μm (fine particles; PM2.5), are considered to be particularly harmful, as they efficiently evade the mucociliary defense system and are deposited in the peripheral airways, where they can exert their pathophysiological mechanisms, linking exposure to ambient fine particles to diseases.Citation9
Emissions from biomass fuels usually contain this harmful size range. Their chemical composition substantially depends on fuel composition and combustion characteristics and is different from industrial or traffic-related particles on which most health studies have focused.Citation3 However, molecular studies have demonstrated that particulate matter from different sources may all induce oxidative stress and cellular inflammatory responses, potential mechanisms linking PM to respiratory or cardiovascular diseases and lung cancer.Citation10-13
Adaption to environmental changes occurs by epigenetic chromatin modifications resulting in durable alterations in gene expression.Citation14 Indeed, for most of PM-associated diseases, epigenetic aberrations have been described.Citation15 Correspondingly, changes in the DNA methylation pattern have been observed in the presence of ambient PM. Initially, studies determining methylation changes and its disease relevance have mainly focused on specific candidate target genes or repetitive elements in blood DNA, e.g., after exposure to black carbon, cigarette condensate, or traffic-related particles.Citation16-18 Only lately, global methylation changes were determined upon high-occupational PM2.5 exposure.Citation19 Despite the significant impact of PM from biomass combustion on health, comprehensive genome-wide methylation studies are still missing.
In our study, we generated profiles of the human methylome and transcriptome by microarray technologies in the presence of PM2.5 from biomass combustion using human airway epithelial cells (BEAS-2B) that represent the first barrier to inhaled extraneous particles. Exposure to PM2.5 from biomass combustion resulted in genome-wide changes of the methylome including repetitive elements and noncoding transcripts that may be relevant for lung cancer, inflammation, asthma, and other PM-associated diseases. Additionally, we filtered 152 differentially expressed genes, which were either hyper- or hypomethylated. From these genes, 66 were related to lung diseases, especially to lung cancer. Our results provide first insights on how changes in the methylation pattern of genes induced by chronic exposure to PM from biomass combustion may especially influence the development of various lung diseases.
Results
Characterization of PM2.5 from biomass combustion
PM2.5 was obtained from a biomass power plant. Mineralogical analysis revealed that the particles mainly consisted of crystalline arcanite (K2SO4) or amorphous components (for detailed composition see Table S1 and Dornhof et al.).Citation20
Methylome of PM2.5-exposed BEAS-2B cells
To analyze whether PM affects the human methylome, human bronchial epithelial BEAS-2B cells were persistently exposed to PM2.5 from biomass combustion to accumulate DNA methylation changes. Cytotoxic effects were excluded by the absence of caspase-3/7 activity and DNA fragmentation (Fig. S1). Genome-wide DNA methylation was determined by using the Illumina Infinium HumanMethylation450 BeadChip (450K) Array. After 5 weeks, differential DNA methylation was observed in PM2.5-exposed BEAS-2B cells at 1.29% of the annotated sites (5501 CpGs) (). The majority of alterations in DNA methylation were related to hypomethylation (decrease in Δβ > 0.02; 4234 CpGs; ). Some individual differentially methylated CpGs were annotated to several different sites in relation to gene region or distance to CpG island ().
Figure 1. Methylome of BEAS-2B cells exposed to PM2.5 for 5 weeks. (A) Percentage of differentially methylated CpG sites in untreated BEAS-2B cells vs. PM2.5-exposed cells (change in Δβ>0.02). (B) Percentage of hypermethylation and hypomethylation. (C) Functional genomic distribution of hypomethylated CpGs (upper pie chart) or in relation to CpG island regions (lower pie chart). (D) Functional genomic distribution of hypermethylated CpGs (upper pie chart) or in relation to CpG island regions (lower pie chart).
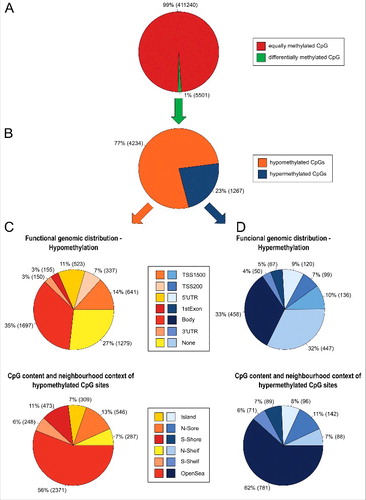
CpG hypo- and hypermethylation events in PM2.5-exposed BEAS-2B cells occurred frequently in the body of genes or unrelated to any gene region. Because methylation changes at CpG island-associated promoter sites have high relevance for gene expression,Citation14 these sites were further investigated. The promoter region, including CpGs located in the 5′UTR, the TSS200, and the TSS1500, was hypomethylated at 35.45% (1501 CpGs) and hypermethylated at 28.02% (355 CpGs) of the differentially methylated sites (). However, most of the hypo- and hypermethylation events, observed in the proximal promoters of PM2.5-exposed cells occurred in promoters not associated with CpG islands (Figs. S2/S3). Only 9.6% (144 CpGs) of the hypomethylated sites and 15.21% (54 CpGs) of the hypermethylated sites in the promoter region were associated with CpG islands.
Additionally, the number of the differentially methylated CpG sites in the regions island, N-shelf, N-shore, open sea, S-shelf, and S-shore were compared with the expected number of CpG sites, assuming that methylation changes appear equally distributed in all regions. However, it became evident that CpG sites located in islands appeared less frequently (negative enrichment, p<0.05) compared with a relatively high number of differentially methylated CpG sites (positive enrichment, p<0.05) located in the shelf or open sea region (Table S2).
Chromosomal distribution of DNA methylation of PM2.5-exposed BEAS-2B cells
Next, the chromosomal distribution of PM2.5 dependent DNA methylation was studied (, Table S3). DNA methylation changes were distributed on all analyzed chromosomes. Most methylation changes were detected on chromosome 1 (≥10% of either the hypo- or hypermethylated sites). In relation to the total amount of annotated CpGs per chromosome, hypomethylation was observed more frequently on chromosomes 1, 16, 17, and 22 (each ≥1.25%; enrichment tested by χ2-test, p < 0.05), whereas hypermethylation was enriched on chromosome 5 (≥0.4%; p < 0.05). Moreover, differential methylation was observed in both G- and R-bands. The fraction of hypomethylated CpGs compared with hypermethylated CpGs was significantly higher in R-bands (2868 hypomethylated CpGs; 746 hypermethylated CpGs) than in G-bands, which stain positive for Giemsa (1353 hypomethylated CpGs; 507 hypermethylated CpGs; χ2-test, p < 0.05). R-bands are known for their high gene density.
Figure 2. Ideogram of the human karyotype. R-bands are shown in white, G-bands in gray; the centromere and the coding region for rRNA are shown in red, regions of hypomethylation in blue, and regions of hypermethylation in yellow. The gender-specific XY-chromosomes were not further analyzed, because BEAS-2B cells originate from various individuals.
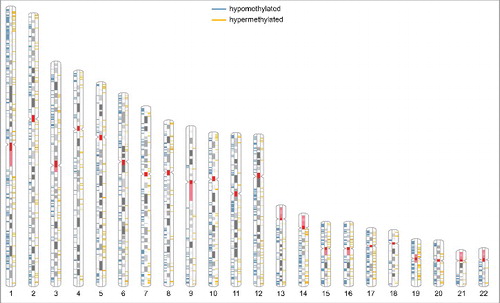
Functional clustering of differentially methylated genes
Differently methylated genes were functionally clustered by a gene ontology analysis, using the Enrichr web application.Citation21 Genes with hypomethylated CpGs were most significantly enriched in pathways associated with GTPase activity, regulatory signal transduction to growth factor stimuli and acidic chemicals, or coded for proteins involved in the architecture of the extracellular matrix (p <1.0e-6; ). Genes with hypermethylated CpG sites were significantly enriched in pathways connected to cellular adhesion and pathways responsible for ion binding, transport, or channel activity (p < 1.0e-5; ). The affected genes are listed in Tables S4/S5.
PM2.5 treatment affects tandem repeats and the miRNA-specific methylome
Differential methylation of repetitive DNA by environmental pollutants might have serious consequences for the structural integrity of the genome and on transcriptional activity, consequently affecting human health.Citation22 Therefore, the methylation profile of these tandem repeats was also analyzed. Interspersed repeats (long interspersed nuclear elements, LINEs; short interspersed nuclear elements, SINEs; long-terminal repeats, LTRs) satellite DNA (simple sequence repeats, SSRs; satellite) and low complexity regions (LCRs) were included in the study (). Reduced methylation of tandem repeats was prominent, but also some DNA loci were hypermethylated, indicating potentially impaired functionality of these sites.
Table 1. PM-dependent methylation changes of tandem repeats.
Furthermore, the differential methylation of noncoding transcripts (miRNAs, miRs) was analyzed using positional data from the miRBase database (http://mirbase.org). Persistent exposure to PM2.5 significantly modified the methylation profile at 45 CpG loci annotated to 43 miRs. A large part of these CpGs were located proximal to the promoter and were hypomethylated, indicating a potential mechanism for their transcriptional activation ().
Table 2. Disease relevance of miR with a PM-dependent, differential DNA methylation pattern.
The direct disease association of miR was examined by the human microRNA Disease Database v.2.0 (http://cuilab.cn/hmdd). We found that miRs with a distinct methylation pattern were prominently involved in lung and PM-associated diseases, such as lung cancer and neoplasms, asthma, interstitial lung disease, or pulmonary embolism (). Moreover, miRs were linked to different types of cancer, regulation of inflammation and viral responses, which may also adversely affect lung physiology. A high proportion of miRs could be allocated to heart diseases. Several miRs contribute to the metabolic syndrome, and affect hypertension, diabetes, or lipid metabolism. All these diseases can be related to high ambient PM exposure.Citation1,Citation2,Citation23
Effect of gene methylation on gene transcription
To determine the biologic significance of differential DNA methylation, gene expression profiling of PM2.5-exposed BEAS-2B cells was performed using the Human Genome U133 Plus 2.0 Array. Subsequent correlation of the transcriptome with changes in methylation resulted in 152 individual genes that show significant congruence (p-value threshold ≤ 0.05; Tables S6/S7). Mapping of expression vs. CpG methylation of these genes indicated a trend toward transcriptional activation by hypomethylation (). Accordingly, 78.42% of the hypomethylated CpGs were positioned on upregulated genes and located in the upper left quadrant. However, a correlation of hypermethylation and gene repression was not evident (lower right quadrant, 51.28% of hypermethylated CpGs). This trend did not change significantly, when only CpG island or promoter-associated alterations in the methylation pattern were included in the analysis (data not shown).
Figure 4. Mapping of gene expression vs. DNA methylation (p-value threshold ≤ 0.05). Each dot represents a single CpG, located on a gene that is both differentially methylated and expressed upon persistent exposure to 100 µg/ml PM2.5 for 5 weeks. Hypomethylated CpGs located on genes that cluster in the upper left quadrant were upregulated, whereas hypermethylated CpGs located on genes that were downregulated clustered in the lower right quadrant. The number of CpGs in each quadrant is indicated (UL, upper left quadrant; UR, upper right quadrant; LL, lower left quadrant; LR, lower right quadrant).
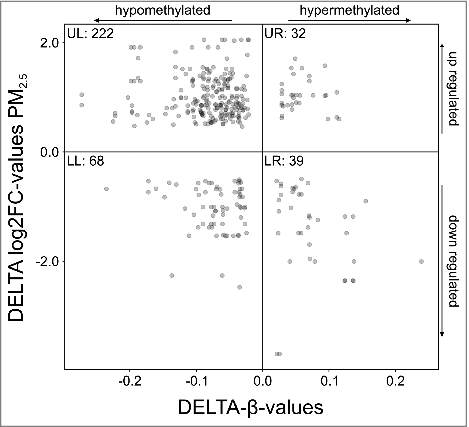
Gene ontology analysis using the Enrichr web application allocated genes that show both differential CpG methylation and transcription to functional properties (, Tables S8/S9). Functional enrichment of downregulated genes revealed that PM2.5 largely affected common pathways related to cytokine signaling, cell motility, differentiation, and development. Upregulated genes were largely involved in biologic responses to extracellular stimuli, e.g., chemotaxis, cell growth, wound healing, inflammation, angiogenesis, and responses to foreign particles or molecules.
Figure 5. Functional enrichment of genes that show both differential methylation and transcription following persistent exposure to 100 µg/ml PM2.5 for 5 weeks identified by the Enrichr web server. The top 14 of the most significant functionally linked pathways, p-values, combined score, and number of clustered genes are indicated.
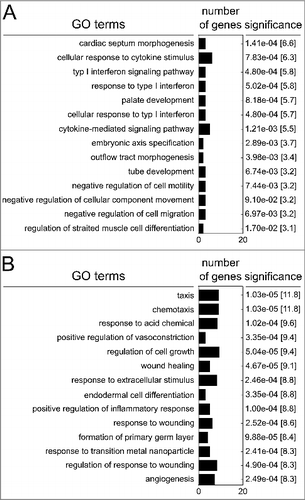
PM2.5-associated changes in the methylome and transcriptome are linked to human disease markers
Exposure to PM2.5 has been epidemiologically related to pulmonary and cardiovascular disease, metabolic disorders, and lung cancer.Citation1,Citation2,Citation23 Therefore, a disease ontology approach was used to find a causal relationship between alterations in the methylome and transcriptome of PM2.5-exposed BEAS-2B cells and diseases known to be exacerbated by PM. The 152 genes that showed both exposure-associated alterations in DNA methylation and gene expression were included in the analysis. Biocomputational profiling using the human disease methylation database (http://bioinfo.hrbmu.edu.cn/diseasemeth), the Dragon Database for Methylated Genes in Diseases (http://cbrc.kaust.edu.sa/ddmgd/), and the Human Gene Database GeneCards® (http://genecards.org) revealed 66 molcular regulators mainly associated with lung diseases, in particular with lung cancer (). Extracted genes involved in lung independent diseases are listed in Table S9.
Table 3. Differentially methylated and expressed genes and allocated lung-specific and lung-associated diseases.
Next, the cellular and molecular relevance of the genes associated with lung cancer was investigated by a Medline search in more detail (data are included in , for references see Table S10). A significant number of these genes are upregulated in tumors and modulated by methylation. Some of these genes are implicated in tumor suppression and improved prognosis, whereas other genes were found to be largely involved in cellular transformation, proliferation and cell growth, tumor progression, metastasis, angiogenesis, inhibition of apoptosis, and therapeutic resistance. These tumor-promoting genes are prominently associated with Wnt, Akt, growth factor or hypoxia-related signaling pathways, epithelial-mesenchymal transition (EMT), and the circadian system. All of these pathways are central in promoting cellular survival and tumorigenesis.Citation24-30
Discussion
This study demonstrates that long-term exposure to PM2.5 results in genome-wide alterations of the methylome and significantly alters gene expression. Predominantly, we observed hypomethylation of CpG sites, which is consistent with other studies, analyzing tandem repeats or individual candidate genes involved in cancer or cardiovascular disease upon exposure to polluted air.Citation18,Citation31 Extensive DNA hypomethylation can be induced by oxidative stress.Citation32 Correspondingly, we detected increased intracellular levels of reactive oxygen species in chronically PM2.5-exposed lung epithelial cells (Regina Dornhof, unpublished observation).
Although methylation changes were also found in the promoter region, only a minor part of those were located within CpG islands. The methylation pattern of CpG islands within the promoter region seems to be most critical for gene expression,Citation14 but methylation outside this region may also be functionally relevant, because these CpG sites are evolutionarily conserved and not subject to negative selection. Accordingly, it was shown that changes in the methylation pattern of non-CpG island promoters have almost an identical impact on gene expression and disease relevance as changes in CpG island promoters.Citation33
PM-mediated variations in DNA methylation were distributed among most chromosomes, but accumulated at regions with high gene density. The fraction of hypomethylated to hypermethylated CpG sites was enriched in the transcriptionally competent R-bands compared with G-bands and hypomethylation was most prominent on the chromosomes 17 and 19, which contain the highest gene density in the genome. The observed intra-chromosomal clustering of DNA methylation changes may be a specific biologic response to PM2.5. However, it may also demonstrate that changes in methylation is enriched at loci of high gene density or simply is a matter of technical bias by annotation of variable CpG sites to these regions.
Gene ontology enrichment analysis revealed that hypomethylated genes prominently affected pathways involved in G-protein signaling, cellular structure and extracellular interaction. Correspondingly, we observed PM-dependent cytoskeletal changes, such as the formation of stress fibers,Citation20 which might progress to epithelial barrier disruption in the respiratory tract, systemic spread of inhaled particles and lung-independent diseases. Hypermethylated genes were also associated with pathways involving cellular adhesion, indicating that these pathways are a dominant field of epigenetic regulation by PM. Loss of cellular adhesion by repressive hypermethylation might promote cancer cell progression, permeability of epithelial barriers, and metastasis. Furthermore, hypermethylation also clustered for pathways responsible for ion binding and shuttling, which might represent a biologic response to the high salt content in PM2.5.
Hypomethylation was evident at repetitive element-associated DNA. Likewise, exposure to ambient air pollution or cigarette smoke induces hypomethylation of interspersed elements (LINE-1) or tandem repeats.Citation18,Citation31,Citation34 Reduced methylation of interspersed repeats is associated with genomic instability, overexpression of oncogenes, and a higher risk of developing and dying from cancer.Citation35,Citation36 It has been proposed that hypomethylation of repetitive elements may also be used as a marker for many other human diseases, including metabolic disorders, lung injury, cardiovascular diseases, and associated risk factors.Citation16,Citation37-39 Thus, genome-wide hypomethylation of repetitive elements might represent a major mechanism to promote PM-associated diseases.
miRs posttranscriptionally affect gene expression and their deregulation may be involved in the pathogenesis of human disease.Citation40 Transcriptional activation of miRs can be regulated epigeneticallyCitation41 and exposure to environmental pollutants or cigarette smoke may result in differential expression of miRs.Citation42,Citation43 We found methylation changes in some of these miR loci but also identified others for which relationship to PM exposure has not yet been reported (miRs listed in ). Methylation changes were located near to the transcription start point suggesting functional relevance. A miR-specific disease database predicted a connection to PM-associated diseases. Almost all hits were related to the development or progression of cancer. miR-loci with a tumor suppressive allocation were frequently hypermethylated,Citation44-48 whereas some oncogenic miR-loci were hypomethylated.Citation49-55 However, the biologic functions of miRs are complex and most often tumor-type specific. Several miRs were also involved in the regulation of inflammation, response to pathogens, cardiovascular diseases, and associated risk factors, or various lung specific diseases (see ). Some of these miRs have been described as useful biomarkers, such as for early detection of non-small cell lung cancer (NSCLC),Citation56 COPD,Citation57 or type 2 diabetes,Citation58 indicating that their dysregulation might represent an early event in disease development. Furthermore, dysregulation of some of these miRs frequently correlates with outcome of cancer and is predictive for refractory therapy,Citation59-61 development or relapse of disease before clinical manifestation,Citation62 and poor prognosis.Citation50,Citation51,Citation63 Additionally, it may lead to hypertensionCitation64 and is also indicative of increased risk of death upon chronic heart diseaseCitation65 or post infarction.Citation66 However, further experiments are needed to prove that methylation changes affect miR and target gene expression, and thus represent a prominent mechanism for PM-associated diseases.
Hypomethylation of genes is associated with transcriptional activation.Citation14 Accordingly, most genes that were hypomethylated also showed increased gene expression. In contrast, only less than half of the hypermethylated genes showed transcriptional repression. Because hypermethylation of CpG islands within promoter regions has a high impact on transcriptional repession,Citation14 these genes were separately analyzed, but also showed no statistical bias for gene repression (data not shown). Controversially, increased gene body methylation or high methylation of shore and shelf regions, flanking demethylated CpG islands are associated with high gene expression, which might partly explain lack of correlation.Citation67,Citation68 Nevertheless, methylated CpG dinucleotides are considered to be mutational hot spots that cause genetic diseases and cancer through spontaneous deamination of 5-methylcytosine (5mC) and its transition to thymine.Citation14
We functionally clustered differentially methylated genes according to their up- or downregulated expression. Interestingly, most of the selected genes were repeatedly identified by different GO terms with potential impact on PM-associated diseases (genes listed in Tables S8/S9 and referenced in Table S10). Some antiviral genes were downregulated, whereas other genes, encoding viral docking sites, were upregulated. This may facilitate respiratory infections, frequently associated to ambient PM or cigarette smoke exposure.Citation69,Citation70 Furthermore, genes that promote respiratory or cardiovascular diseases were primarily upregulated, whereas suppressors of these diseases were transcriptionally repressed. Encoded proteins are known to be critically involved in the pathogenesis of acute and chronic inflammation, asthma, COPD, atherosclerosis, or lung injury, but also often have pleiotropic functions and thus may additionally contribute to epithelial-mesenchymal transition, chronic inflammation and, consequently, to a pro-tumorigenic microenvironment.Citation71,Citation72 Other allocated genes may directly promote tumorigenesis by facilitating proliferation, invasiveness, metastasis, angiogenesis, immune evasion or resistance and are associated with poor patient survival when upregulated in tumor cells, tumor stroma, or surrounding tissue (Tables S8/S9). Transcriptional and functional activation by cigarette smoke or airborne particulate matter and regulation by methylation have been independently confirmed for some of these genes (Tables S9/S10). In contrast, tumor suppressive genes were transcriptionally repressed in the presence of PM (Tables S8/S10). Interestingly, some upregulated genes contradictorily showed hypermethylation whereas some repressed genes were hypomethylated (Tables S6-S9). This indicates that these genes may not be exclusively epigenetically regulated, but that additional, unknown mechanisms might predominate for their expression. However, most of these genes were repeatedly identified by multiple gene ontology terms with highest significance highlighting their potential relevance. Because they represent central mediators of severe diseases, their reciprocal interaction and pathophysiological impact on adverse health effects by PM should be further examined.
In addition, the disease relevance of differentially methylated and expressed genes was confirmed by disease ontology. Some of the previously functionally clustered genes were allocated again and numerous additional genes were identified (). Genes, significant for cancer formation and progression were most highly enriched, indicating that exposure to PM may facilitate pro-tumorigenic conditions and transform lung epithelial cells to tumor cells. The cancer-associated molecular functions of these genes are referenced in Table S10. Again, tumor-promoting genes and genes associated with poor outcome in cancer patients were generally upregulated, whereas tumor-suppressive genes were often downregulated. However, the observed upregulation of some tumor-suppressive genes might represent an adaptive response to protect from cellular transformation. Independent studies have shown that many of these genes can be regulated by DNA methylation (see and Table S10) and, interestingly, most of the allocated genes were associated with the same dominant pro-tumorigenic pathways, such as EGF/TGF-β-signaling, EMT including downregulation of E-cadherin, b-catenin activation, and response to hypoxia. Since these pathways are substantially linked to malignant progression, metastasis, angiogenesis, therapy resistance, and poor clinical outcome,Citation25-29 it is crucial to further verify their activation and pathologic function during PM exposure.
In addition to cancer, dysregulation of some of these disease relevant genes may also exacerbate pulmonary inflammation, lung injury, severity of respiratory infections, and progression of COPD and ARDS (Table S10), but also lung-independent diseases, such as cardiovascular disease, hypertension, metabolic syndrome, neurologic disorders and chronic inflammation, or cancer in different tissue types (Table S11) and, thus, may contribute to other common pathological features symptomatic for PM exposure. However, here we used bronchial epithelial cells and therefore our discussion focuses on lung diseases. Whether PM2.5 induces similar biologic responses in different cell types needs further investigation.
Our results may open the door for preventive or therapeutic considerations, e.g., by using dietary compounds to reduce or reverse detrimental methylation changes when exposed to high PM2.5 concentrations. It has already been shown that folic acid can enhance methylation and that DNA methylation inhibitors can significantly reduce carcinogen-induced lung cancer development in animal models.Citation73,Citation74
However, the presented data may have some limitations by the use of a single fraction of PM from biomass combustion. Particle composition and cellular responses can vary depending on the type of biomass and combustion conditions.Citation75 Additionally, the concentration of PM and duration of exposure may have a direct impact on biologic responses. The given concentration (100 μg/ml), which had no significant impact on cell viability upon prolonged treatment, was also used in other studies investigating biocombustion.Citation11 Moreover, our approach for efficient identification of putative target sites for methylation changes is additionally supported by the fact that ambient PM concentrations from biomass combustion may significantly exceed industrial or traffic-related emissions,Citation3,Citation8 and that inhaled PM <2.5 μm may accumulate in the lung parenchyma.Citation9 Consistently, health effects are especially evident in regions with recurrent and intense pollution, but rarely after a single and short exposure.Citation1 Nevertheless, further studies will be necessary for targeted validation of the most important top hits. We also acknowledge that the use of an immortalized cell line and cell culture conditions may not reflect in vivo processes. Moreover, both 5mC and 5-hydroxymethylcytosine (5hmC) are interrogated by the Illumina 450K array. The 5hmC modification, which is an intermediate of 5mC demethylation by TET enzymes,Citation76 may be responsible for some of the activation, correlated with higher promoter methylation. Finally, the Illumina 450K array does not completely cover the entire epigenome.
In conclusion, using BEAS-2B cells as a model, we could observe genome wide DNA methylation changes at CpG sites residing in disease relevant genes, repetitive elements, and miRs. Consequently, DNA methylation analysis should be included in future efforts to understand the interactions between environmental exposures and PM-associated diseases. By identifying putative targets and their allocation to selective prominent disease relevant pathways, there is now an urgent need to independently confirm the biologic effects of methylation changes on gene expression, to clarify the interplay of the affected mediators, and to demonstrate how they contribute to adverse health effects. This might finally result in the establishment of new sensitive and reliable diagnostic biomarkers and therapeutic targets for the prevention and/or treatment of PM-associated diseases.
Materials and methods
Preparation of PM2.5
Preparation of PM2.5 has been described previously.Citation20 Briefly, bulk fly ash was collected from an electrostatic precipitator of a medium-scale biomass power plant with a nominal thermal output of 1.7 MW (Bürger Energie St. Peter eG, St. Peter, Schwarzwald, Germany), which exclusively combusts chips of soft wood (mainly spruce) derived from the local forests. The wood chips included debarked stem wood and branches with a minimum of 7 cm in diameter (merchantable wood) without leaves and twigs. The collected ash was subsequently size-fractionated by a cyclone with an aerodynamic cut-off diameter of 2.5 µm (Labor für Partikeltechnologie/Mechanische Verfahrenstechnik, Hochschule Konstanz, Technik, Wirtschaft und Gestaltung). The resultant size fraction (PM2.5) was then used for all biologic assays.
Cell culture and PM2.5 treatment
Immortalized bronchial epithelial cells (BEAS-2B; LGC Standards GmbH, #ATCC-CRL-9609) were maintained in Dulbecco's Modified Eagle Medium/F12 with stable Glutamine (GE Healthcare, #E15–889) containing 5% fetal bovine serum (Life Technologies, #10270–106) 100 IU streptomycin and 100 IU penicillin (Sigma-Aldrich, #11074440001) at 37°C in a humidified incubator. BEAS-2B cells were plated at a density of 0.7 × 106 cells per 75 cm2 cell culture flasks (Greiner bio-one, #658175) and were split twice a week after rinsing 2 times with phosphate buffered saline and subsequently using trypsin, 0.25% EDTA (Life Technologies, #25200056) for detachment. For the experiments, adherent cells were repeatedly treated with PM2.5 every first day after each passage. Particles were immersed in BEAS-2B growth medium at a concentration of 200 μg/ml and sonicated for 20 min (SONOREX RK510H, BANDELIN electronic GmbH & Co. KG, Berlin, Germany) immediately before dilution to the cells at a final concentration of 100 µg/ml PM2.5. The cells were maintained in the same PM2.5-containing medium until the next passage. For the last passage, cells were seeded in 6-well plates and were exposed on the next day with 100 µg/ml PM2.5 for a final time period of 48 h. Control cells were similarly treated in the absence of PM2.5. After 5 weeks of exposure, cells were harvested for DNA (DNeasy Blood & Tissue Kit, Qiagen, #69504) and RNA (RNeasy® Plus Mini Kit, Qiagen, #74136) extraction following the manufacturer's instructions. To avoid RNA contamination, 400 μg RNase A (Carl Roth, #7156.1) were added during cellular lysis for genomic DNA isolation. For each condition (control and PM2.5-treated cells) 3 independent replicates have been performed for DNA and for RNA isolation.
DNA methylation chip preparation and data analysis
The Illumina HumanMethylation450 BeadChip (450K) array was performed by AROS Applied Biotechnology A/S (Aarhus, Denmark) resulting in IDAT files containing the raw data. Analysis of Illumina 450K v1.2 data was performed with the R minfi package.Citation77 For the preprocessing of the raw data, the preprocessQuantile function was used. After calculation of β-values, 56,235 cross hybridizing probes were removed.Citation78 Differentially methylated positions were identified using the dmpFinder function and a False Discovery Rate (FDR) of less than 5% was obtained (q-value cutoff of 0.05). The differences of averaged β-values of the 4 replicates of each control and particular matter sample were defined with a cutoff for minimum difference of 0.02 (decrease in Δβ > 0.02, hypomethylated; increase in Δβ>0.02, hypermethylated). Probes were annotated using the getAnnotation function implemented in the minfi package. Enrichment calculations (Table S2) were based on the χ2-test (p < 0.05).
Gene expression and chip preparation
Affymetrix HG-U133 Plus 2.0 oligonucleotide microarrays (Affymetrix, #900467) were used to determine transcription levels of more than 54,000 transcripts and variants, representing over 20,000 genes. RNA was quality assessed (Agilent RNA 6,000 Nanokit, Agilent Technologies, #AGI 5067–1511; Agilent 2100 Bioanalyzer, Agilent Technologies, Waldbronn, Germany) and quantified (NanoDrop ND-1,000 spectrophotometer, Thermo Fisher Scientific, Berlin, Germany). The RNA quality revealed values of RIN ≥ 9.9 for all samples. After reverse transcription of 300 ng total RNA (Invitrogen™ Ambion™ RETROscript™ Reverse Transcription Kit, Thermo Fisher Scientific, #AM1710) and generation of cRNA probes (3′ IVT Plus Amplification Kit, Affymetrix, #902395), 50 μg/mL of biotinylated and fragmented cRNA was hybridized to HG-U133 Plus 2.0 arrays. After washing and fluorescence labeling (GeneChip Hybridization, Wash & Stain Kit, Affymetrix, #900720), arrays were scanned (Affymetrix GeneChip Scanner 3000, Affymetrix).
Gene expression analysis
Analysis of the raw expression data was performed using the simpleaffyCitation79 and the affy R packagesCitation80 for uploading and RMA normalization of the data. The lmFit, contrasts.fit, as well as the eBayes functions of the limma packageCitation81 were used to preprocess the data, and, finally, the topTable function was applied to identify differentially expressed genes, with a false discovery rate defined by the Benjamini-Hochberg method of smaller than 0.05 and each p-value smaller than 0.05. Annotation data of probes were obtained from the supplier's homepage (http:/www.affymetrix.com/support/technical/byproduct.affx?product = hg-u133-plus, file: “HG-u133_Plus_2.na35.annot.csv,” creation_date = 2014–10–06). If several probe sets were assigned to the same LocusID either all sets were kept individually since variances between those values might be a result of alternative splicing of the related transcript ()Citation82 or the average of the transcripts were calculated (, S6, and S7) for a clearer presentation.
Data plotting
Mean β values of the control probes were plotted against the β values of the PM-treated samples of all differentially methylated CpG sites using the ggplot2 package of R.Citation83
Transcription start site (TSS) positions were obtained from the UCSC website (http://hgdownload.soe.ucsc.edu/goldenPath/hg19/database/; file: “knownGene.txt.gz”) taking into account the strandedness of annotated genes. Distances of all differentially methylated CpG positions to their nearest TSSs were calculated with the help of the DistanceToNearest-function of the “GenomicRanges” package,Citation84 and plotted against their corresponding Dbvalue.
The phenogram shown in the manuscript is a collage of 3 different phenograms (for hypomethylated CpGs, hypermethylated CpGs, and Giemsa bands). Each phenogram was generated using the web application PhenoGram.Citation85 The input data table for the Giemsa-stained cytobands was obtained from the PhenoGram website (cytoBand-human.txt, http://visualization.ritchielab.psu.edu/phenograms/document).
The names of all differentially methylated or differentially expressed genes were converted with the help of the web application GeneCards (http://www.genecards.org/) and the db2db database (https://biodbnet-abcc.ncifcrf.gov/db/db2db.php), and subsequently analyzed for enrichment using the default parameters of the Enrichr platform.Citation21 Plotting of the tabular output of Enrichr was performed using Python's matplotlib module (http://matplotlib.org/).
Overlaps of all differentially methylated CpG positions with different kinds of repetitive elements (“LINE,” “SINE,” “LTR,” “Simple_repeat,” “Low_complexity,” “Satellite”) were counted using the GenomicRanges package in R.Citation84 The repetitive element positions were downloaded from the UCSC website (http://hgdownload.cse.ucsc.edu/goldenPath/hg19/database/, file: rmsk.txt.gz). Overlaps with miRs were counted and incorporated with the input data from the miRBase website (http://www.mirbase.org/ftp.shtml, file: hsa.gff3; gff-version 3 - miRBase v21, build GRCh38, hg38). The CpG positions were converted from hg19 to hg38 by using the liftOver tool (http://genome.ucsc.edu/cgi-bin/hgLiftOver).
Circos plots were generated using the default settings of related web application (http://mkweb.bcgsc.ca/tableviewer/).
Disclosure of potential conflicts of interest
No potential conflicts of interest were disclosed.
Authors contribution
Katharina Heßelbach provided biologic samples, was involved in data processing, their interpretation and assisted with manuscript preparation. Gwang-Jin Kim and Stefan Günther were responsible for bioinformatics data processing, statistical analysis and manuscript preparation. Stephan Flemming established the bioinformatics data processing and was involved in the interpretation of results. Thomas Häupl and Marc Bonin conducted the Affymetrix HG-U133 Plus 2.0 transcriptome analysis. Regina Dornhof provided biologic samples. Irmgard Merfort and Matjaz Humar were responsible for study design, sample preparation, data analysis, interpretation and presentation of results and manuscript preparation.
kepi_a_1356555_supporting_information.docx
Download MS Word (2.7 MB)Funding
This work was supported by the EU Interreg IV Programm “Oberrhein” (project C35 BIOCOMBUST) and the German Research Foundation (DFG, CRC992, Medical Epigenetics).
References
- Anderson JO, Thundiyil JG, Stolbach A. Clearing the air: a review of the effects of particulate matter air pollution on human health. J Med Toxicol. 2012;8:166-75. doi:10.1007/s13181-011-0203-1. PMID:22194192
- Raaschou-Nielsen O, Andersen ZJ, Beelen R, Samoli E, Stafoggia M, Weinmayr G, Hoffmann B, Fischer P, Nieuwenhuijsen MJ, Brunekreef B, et al. Air pollution and lung cancer incidence in 17 European cohorts: prospective analyses from the European Study of Cohorts for Air Pollution Effects (ESCAPE). Lancet Oncol. 2013;14:813-22. doi:10.1016/S1470-2045(13)70279-1. PMID:23849838
- Naeher LP, Brauer M, Lipsett M, Zelikoff JT, Simpson CD, Koenig JQ, Smith KR. Woodsmoke health effects: a review. Inhal Toxicol. 2007;19:67-106. doi:10.1080/08958370600985875. PMID:17127644
- Programme UND. World energy assesment. New York:UNPD; 2004.
- Lelieveld J, Evans JS, Fnais M, Giannadaki D, Pozzer A. The contribution of outdoor air pollution sources to premature mortality on a global scale. Nature. 2015;525:367-71. doi:10.1038/nature15371. PMID:26381985
- Kim KH, Jahan SA, Kabir E. A review of diseases associated with household air pollution due to the use of biomass fuels. J Hazard Mater. 2011;192:425-31. doi:10.1016/j.jhazmat.2011.05.087. PMID:21705140
- WHO. Household air pollution and health. WHO Media Centre; 2016. : Fact sheet N°292.
- Rumchev K, Zhao Y, Spickett J. Health Risk Assessment of Indoor Air Quality, Socioeconomic and House Characteristics on Respiratory Health among Women and Children of Tirupur, South India. Int J Environ Res Public Health. 2017;14:E429. doi:10.3390/ijerph14040429. PMID:28420188
- Valavanidis A, Fiotakis K, Vlachogianni T. Airborne particulate matter and human health: toxicological assessment and importance of size and composition of particles for oxidative damage and carcinogenic mechanisms. J Environ Sci Health C Environ Carcinog Ecotoxicol Rev. 2008;26:339-62. doi:10.1080/10590500802494538. PMID:19034792
- Maatta J, Luukkonen R, Husgafvel-Pursiainen K, Alenius H, Savolainen K. Comparison of hardwood and softwood dust-induced expression of cytokines and chemokines in mouse macrophage RAW 264.7 cells. Toxicology. 2006;218:13-21. doi:10.1016/j.tox.2005.09.001. PMID:16202497
- Danielsen PH, Moller P, Jensen KA, Sharma AK, Wallin H, Bossi R, Autrup H, Molhave L, Ravanat JL, Briede JJ, et al. Oxidative stress, DNA damage, and inflammation induced by ambient air and wood smoke particulate matter in human A549 and THP-1 cell lines. Chem Res Toxicol. 2011;24:168-84. doi:10.1021/tx100407m. PMID:21235221
- Ovrevik J, Refsnes M, Lag M, Brinchmann BC, Schwarze PE, Holme JA. Triggering Mechanisms and Inflammatory Effects of Combustion Exhaust Particles with Implication for Carcinogenesis. Basic Clin Pharmacol Toxicol. 2016. PMID:28001342.
- Lodovici M, Bigagli E. Oxidative stress and air pollution exposure. J Toxicol. 2011;2011:487074. doi:10.1155/2011/487074. PMID:21860622
- Li E, Zhang Y. DNA methylation in mammals. Cold Spring Harb Perspect Biol. 2014;6:a019133. doi:10.1101/cshperspect.a019133. PMID:24789823
- Heerboth S, Lapinska K, Snyder N, Leary M, Rollinson S, Sarkar S. Use of epigenetic drugs in disease: an overview. Genet Epigenet. 2014;6:9-19. PMID:25512710.
- Bellavia A, Urch B, Speck M, Brook RD, Scott JA, Albetti B, Behbod B, North M, Valeri L, Bertazzi PA, et al. DNA hypomethylation, ambient particulate matter, and increased blood pressure: findings from controlled human exposure experiments. J Am Heart Assoc. 2013;2:e000212. doi:10.1161/JAHA.113.000212. PMID:23782920
- Bruniquel D, Schwartz RH. Selective, stable demethylation of the interleukin-2 gene enhances transcription by an active process. Nat Immunol. 2003;4:235-40. doi:10.1038/ni887. PMID:12548284
- Guo L, Byun HM, Zhong J, Motta V, Barupal J, Zheng Y, Dou C, Zhang F, McCracken JP, Diaz A, et al. Effects of short-term exposure to inhalable particulate matter on DNA methylation of tandem repeats. Environ Mol Mutagen. 2014;55:322-35. doi:10.1002/em.21838. PMID:24436168
- Panni T, Mehta AJ, Schwartz JD, Baccarelli AA, Just AC, Wolf K, Wahl S, Cyrys J, Kunze S, Strauch K, et al. Genome-Wide Analysis of DNA Methylation and Fine Particulate Matter Air Pollution in Three Study Populations: KORA F3, KORA F4, and the Normative Aging Study. Environ Health Perspect. 2016;124:983-90. doi:10.1289/ehp.1509966. PMID:26731791
- Dornhof R, Maschowski C, Osipova A, Gieré R, Seidl M, Merfort I, Humar M. Stress fibers, autophagy and necrosis by persistent exposure to PM2.5 from biomass combustion. PLoS One. 2017;12(7):e0180291. doi:10.1371/journal.pone.0180291. PMID:28671960
- Kuleshov MV, Jones MR, Rouillard AD, Fernandez NF, Duan Q, Wang Z, Koplev S, Jenkins SL, Jagodnik KM, Lachmann A, et al. Enrichr: a comprehensive gene set enrichment analysis web server 2016 update. Nucleic Acids Res. 2016;44:W90-7. doi:10.1093/nar/gkw377
- Ross JP, Rand KN, Molloy PL. Hypomethylation of repeated DNA sequences in cancer. Epigenomics. 2010;2:245-69. doi:10.2217/epi.10.2. PMID:22121873
- Coogan PF, White LF, Jerrett M, Brook RD, Su JG, Seto E, Burnett R, Palmer JR, Rosenberg L. Air pollution and incidence of hypertension and diabetes mellitus in black women living in Los Angeles. Circulation. 2012;125:767-72. doi:10.1161/CIRCULATIONAHA.111.052753. PMID:22219348
- Xu CX, Jin H, Shin JY, Kim JE, Cho MH. Roles of protein kinase B/Akt in lung cancer. Front Biosci (Elite Ed). 2010;2:1472-84. PMID:20515818
- Lindsey S, Langhans SA. Epidermal growth factor signaling in transformed cells. Int Rev Cell Mol Biol. 2015;314:1-41. PMID:25619714
- Miyazono K, Ehata S, Koinuma D. Tumor-promoting functions of transforming growth factor-beta in progression of cancer. Ups J Med Sci. 2012;117:143-52. doi:10.3109/03009734.2011.638729. PMID:22111550
- Schmalhofer O, Brabletz S, Brabletz T. E-cadherin, beta-catenin, and ZEB1 in malignant progression of cancer. Cancer Metastasis Rev. 2009;28:151-66. doi:10.1007/s10555-008-9179-y. PMID:19153669
- Tiwari N, Gheldof A, Tatari M, Christofori G. EMT as the ultimate survival mechanism of cancer cells. Semin Cancer Biol. 2012;22:194-207. doi:10.1016/j.semcancer.2012.02.013. PMID:22406545
- Semenza GL. Defining the role of hypoxia-inducible factor 1 in cancer biology and therapeutics. Oncogene. 2010;29:625-34. doi:10.1038/onc.2009.441. PMID:19946328
- Arellanes-Licea E, Caldelas I, De Ita-Perez D, Diaz-Munoz M. The circadian timing system: a recent addition in the physiological mechanisms underlying pathological and aging processes. Aging Dis. 2014;5:406-18. PMID:25489492
- Chen R, Meng X, Zhao A, Wang C, Yang C, Li H, Cai J, Zhao Z, Kan H. DNA hypomethylation and its mediation in the effects of fine particulate air pollution on cardiovascular biomarkers: A randomized crossover trial. Environ Int. 2016;94:614-9. doi:10.1016/j.envint.2016.06.026. PMID:27397927
- Valinluck V, Tsai HH, Rogstad DK, Burdzy A, Bird A, Sowers LC. Oxidative damage to methyl-CpG sequences inhibits the binding of the methyl-CpG binding domain (MBD) of methyl-CpG binding protein 2 (MeCP2). Nucleic Acids Res. 2004;32:4100-8. doi:10.1093/nar/gkh739. PMID:15302911
- Han H, Cortez CC, Yang X, Nichols PW, Jones PA, Liang G. DNA methylation directly silences genes with non-CpG island promoters and establishes a nucleosome occupied promoter. Hum Mol Genet. 2011;20:4299-310. doi:10.1093/hmg/ddr356. PMID:21835883
- Liu F, Killian JK, Yang M, Walker RL, Hong JA, Zhang M, Davis S, Zhang Y, Hussain M, Xi S, et al. Epigenomic alterations and gene expression profiles in respiratory epithelia exposed to cigarette smoke condensate. Oncogene. 2010;29:3650-64. doi:10.1038/onc.2010.129. PMID:20440268
- Zhu ZZ, Sparrow D, Hou L, Tarantini L, Bollati V, Litonjua AA, Zanobetti A, Vokonas P, Wright RO, Baccarelli A, et al. Repetitive element hypomethylation in blood leukocyte DNA and cancer incidence, prevalence, and mortality in elderly individuals: the Normative Aging Study. Cancer Causes Control. 2011;22:437-47. doi:10.1007/s10552-010-9715-2. PMID:21188491
- Lamprecht B, Walter K, Kreher S, Kumar R, Hummel M, Lenze D, Kochert K, Bouhlel MA, Richter J, Soler E, et al. Derepression of an endogenous long terminal repeat activates the CSF1R proto-oncogene in human lymphoma. Nat Med. 2010;16:571-9, 1p following 9. doi:10.1038/nm.2129. PMID:20436485
- Baccarelli A, Wright R, Bollati V, Litonjua A, Zanobetti A, Tarantini L, Sparrow D, Vokonas P, Schwartz J. Ischemic heart disease and stroke in relation to blood DNA methylation. Epidemiology. 2010;21:819-28. doi:10.1097/EDE.0b013e3181f20457. PMID:20805753
- Martin-Nunez GM, Rubio-Martin E, Cabrera-Mulero R, Rojo-Martinez G, Olveira G, Valdes S, Soriguer F, Castano L, Morcillo S. Type 2 diabetes mellitus in relation to global LINE-1 DNA methylation in peripheral blood: a cohort study. Epigenetics. 2014;9:1322-8. doi:10.4161/15592294.2014.969617. PMID:25437047
- Nzabarushimana E, Prior S, Miousse IR, Pathak R, Allen AR, Latendresse J, Olsen RH, Raber J, Hauer-Jensen M, Nelson GA, et al. Combined exposure to protons and (56)Fe leads to overexpression of Il13 and reactivation of repetitive elements in the mouse lung. Life Sci Space Res (Amst). 2015;7:1-8. doi:10.1016/j.lssr.2015.08.001. PMID:26553631
- Osman A. MicroRNAs in health and disease-basic science and clinical applications. Clin Lab. 2012;58:393-402. PMID:22783567
- Xi S, Xu H, Shan J, Tao Y, Hong JA, Inchauste S, Zhang M, Kunst TF, Mercedes L, Schrump DS. Cigarette smoke mediates epigenetic repression of miR-487b during pulmonary carcinogenesis. J Clin Invest. 2013;123:1241-61. doi:10.1172/JCI61271. PMID:23426183
- Vrijens K, Bollati V, Nawrot TS. MicroRNAs as potential signatures of environmental exposure or effect: a systematic review. Environ Health Perspect. 2015;123:399-411. PMID:25616258
- Rodosthenous RS, Coull BA, Lu Q, Vokonas PS, Schwartz JD, Baccarelli AA. Ambient particulate matter and microRNAs in extracellular vesicles: a pilot study of older individuals. Part Fibre Toxicol. 2016;13:13. doi:10.1186/s12989-016-0121-0. PMID:26956024
- Sun CC, Li SJ, Li DJ. Hsa-miR-134 suppresses non-small cell lung cancer (NSCLC) development through down-regulation of CCND1. Oncotarget. 2016;7:35960-78. doi:10.18632/oncotarget.8482. PMID:27166267
- Wang C, Liu P, Wu H, Cui P, Li Y, Liu Y, Liu Z, Gou S. MicroRNA-323-3p inhibits cell invasion and metastasis in pancreatic ductal adenocarcinoma via direct suppression of SMAD2 and SMAD3. Oncotarget. 2016;7:14912-24. doi:10.18632/oncotarget.7482. PMID:26908446
- Zhou B, Song J, Han T, Huang M, Jiang H, Qiao H, Shi J, Wang Y. MiR-382 inhibits cell growth and invasion by targeting NR2F2 in colorectal cancer. Mol Carcinog. 2016;55(12):2260-67. https://doi.org/10.1002/mc.22466
- Mou X, Liu S. MiR-485 inhibits metastasis and EMT of lung adenocarcinoma by targeting Flot2. Biochem Biophys Res Commun. 2016;477:521-6. doi:10.1016/j.bbrc.2016.04.043. PMID:27262438
- Sun LL, Wang J, Zhao ZJ, Liu N, Wang AL, Ren HY, Yang F, Diao KX, Fu WN, Wan EH, et al. Suppressive role of miR-502-5p in breast cancer via downregulation of TRAF2. Oncol Rep. 2014;31:2085-92. PMID:24677135
- Li X, Liu X, Xu W, Zhou P, Gao P, Jiang S, Lobie PE, Zhu T. c-MYC-regulated miR-23a/24-2/27a cluster promotes mammary carcinoma cell invasion and hepatic metastasis by targeting Sprouty2. J Biol Chem. 2013;288:18121-33. doi:10.1074/jbc.M113.478560. PMID:23649631
- Qu WQ, Liu L, Yu Z. Clinical value of microRNA-23a upregulation in non-small cell lung cancer. Int J Clin Exp Med. 2015;8:13598-603. PMID:26550300
- Zhao G, Liu L, Zhao T, Jin S, Jiang S, Cao S, Han J, Xin Y, Dong Q, Liu X, et al. Upregulation of miR-24 promotes cell proliferation by targeting NAIF1 in non-small cell lung cancer. Tumour Biol. 2015;36:3693-701. doi:10.1007/s13277-014-3008-4. PMID:25725584
- Cristobal I, Madoz-Gurpide J, Rojo F, Garcia-Foncillas J. Potential therapeutic value of miR-425-5p in metastatic colorectal cancer. J Cell Mol Med. 2016;20:2213-4. doi:10.1111/jcmm.12902. PMID:27396018
- Tong XD, Liu TQ, Wang GB, Zhang CL, Liu HX. MicroRNA-570 promotes lung carcinoma proliferation through targeting tumor suppressor KLF9. Int J Clin Exp Pathol. 2015;8:2829-34. PMID:26045791
- Zhang L, Yang L, Liu X, Chen W, Chang L, Chen L, Loera S, Chu P, Huang WC, Liu YR, et al. MicroRNA-657 promotes tumorigenesis in hepatocellular carcinoma by targeting transducin-like enhancer protein 1 through nuclear factor kappa B pathways. Hepatology. 2013;57:1919-30. doi:10.1002/hep.26162. PMID:23175432
- Wang J, Zhao YC, Lu YD, Ma CP. Integrated bioinformatics analyses identify dysregulated miRNAs in lung cancer. Eur Rev Med Pharmacol Sci. 2014;18:2270-4. PMID:25219825
- Zhu W, Zhou K, Zha Y, Chen D, He J, Ma H, Liu X, Le H, Zhang Y. Diagnostic Value of Serum miR-182, miR-183, miR-210, and miR-126 Levels in Patients with Early-Stage Non-Small Cell Lung Cancer. PLoS One. 2016;11:e0153046. doi:10.1371/journal.pone.0153046. PMID:27093275
- Wang M, Huang Y, Liang Z, Liu D, Lu Y, Dai Y, Feng G, Wang C. Plasma miRNAs might be promising biomarkers of chronic obstructive pulmonary disease. Clin Respir J. 2016;10:104-11. doi:10.1111/crj.12194. PMID:25102970
- Yan S, Wang T, Huang S, Di Y, Huang Y, Liu X, Luo Z, Han W, An B. Differential expression of microRNAs in plasma of patients with prediabetes and newly diagnosed type 2 diabetes. Acta Diabetol. 2016;53:693-702. doi:10.1007/s00592-016-0837-1. PMID:27039347
- Zheng D, Dai Y, Wang S, Xing X. MicroRNA-299-3p promotes the sensibility of lung cancer to doxorubicin through directly targeting ABCE1. Int J Clin Exp Pathol. 2015;8:10072-81. PMID:26617714
- Guo L, Liu Y, Bai Y, Sun Y, Xiao F, Guo Y. Gene expression profiling of drug-resistant small cell lung cancer cells by combining microRNA and cDNA expression analysis. Eur J Cancer. 2010;46:1692-702. doi:10.1016/j.ejca.2010.02.043. PMID:20371173
- Li J, Wang Y, Song Y, Fu Z, Yu W. miR-27a regulates cisplatin resistance and metastasis by targeting RKIP in human lung adenocarcinoma cells. Mol Cancer. 2014;13:193. doi:10.1186/1476-4598-13-193. PMID:25128483
- Philipone E, Yoon AJ, Wang S, Shen J, Ko YC, Sink JM, Rockafellow A, Shammay NA, Santella RM. MicroRNAs-208b-3p, 204-5p, 129-2-3p and 3065-5p as predictive markers of oral leukoplakia that progress to cancer. Am J Cancer Res. 2016;6:1537-46. PMID:27508095
- Chen SW, Wang TB, Tian YH, Zheng YG. Down-regulation of microRNA-126 and microRNA-133b acts as novel predictor biomarkers in progression and metastasis of non small cell lung cancer. Int J Clin Exp Pathol. 2015;8:14983-8. PMID:26823832
- Zou X, Wang J, Chen C, Jose PA, Zeng C. Secreted Monocyte miR-27a Causes Hypertension by Reducing Mas Receptor Expression and Function in the Artery. J Am Soc Hypertens. 2016;10Suppl 1:e3. doi:10.1016/j.jash.2016.06.010. PMID:27677133
- Qiang L, Hong L, Ningfu W, Huaihong C, Jing W. Expression of miR-126 and miR-508-5p in endothelial progenitor cells is associated with the prognosis of chronic heart failure patients. Int J Cardiol. 2013;168:2082-8. doi:10.1016/j.ijcard.2013.01.160. PMID:23465244
- Matsumoto S, Sakata Y, Nakatani D, Suna S, Mizuno H, Shimizu M, Usami M, Sasaki T, Sato H, Kawahara Y, et al. A subset of circulating microRNAs are predictive for cardiac death after discharge for acute myocardial infarction. Biochem Biophys Res Commun. 2012;427:280-4. doi:10.1016/j.bbrc.2012.09.039. PMID:22995291
- Edgar R, Tan PP, Portales-Casamar E, Pavlidis P. Meta-analysis of human methylomes reveals stably methylated sequences surrounding CpG islands associated with high gene expression. Epigenetics Chromatin. 2014;7:28. doi:10.1186/1756-8935-7-28. PMID:25493099
- Lou S, Lee HM, Qin H, Li JW, Gao Z, Liu X, Chan LL, Kl Lam V, So WY, Wang Y, et al. Whole-genome bisulfite sequencing of multiple individuals reveals complementary roles of promoter and gene body methylation in transcriptional regulation. Genome Biol. 2014;15:408. doi:10.1186/s13059-014-0408-0. PMID:25074712
- Logan J, Chen L, Gangell C, Sly PD, Fantino E, Liu K. Brief exposure to cigarette smoke impairs airway epithelial cell innate anti-viral defence. Toxicol In Vitro. 2014;28:1430-5. doi:10.1016/j.tiv.2014.07.012. PMID:25111775
- Kelly FJ, Fussell JC. Air pollution and airway disease. Clin Exp Allergy. 2011;41:1059-71. doi:10.1111/j.1365-2222.2011.03776.x. PMID:21623970
- Thiery JP, Acloque H, Huang RY, Nieto MA. Epithelial-mesenchymal transitions in development and disease. Cell. 2009;139:871-90. doi:10.1016/j.cell.2009.11.007. PMID:19945376
- Vendramini-Costa DB, Carvalho JE. Molecular link mechanisms between inflammation and cancer. Curr Pharm Des. 2012;18:3831-52. doi:10.2174/138161212802083707. PMID:22632748
- Gonda TA, Kim YI, Salas MC, Gamble MV, Shibata W, Muthupalani S, Sohn KJ, Abrams JA, Fox JG, Wang TC, et al. Folic acid increases global DNA methylation and reduces inflammation to prevent Helicobacter-associated gastric cancer in mice. Gastroenterology. 2012;142:824-33 e7. PMID:22248660. doi:10.1053/j.gastro.2011.12.058
- Belinsky SA, Klinge DM, Stidley CA, Issa JP, Herman JG, March TH, Baylin SB. Inhibition of DNA methylation and histone deacetylation prevents murine lung cancer. Cancer Res. 2003;63:7089-93. PMID:14612500
- Cassee FR, Heroux ME, Gerlofs-Nijland ME, Kelly FJ. Particulate matter beyond mass: recent health evidence on the role of fractions, chemical constituents and sources of emission. Inhal Toxicol. 2013;25:802-12. doi:10.3109/08958378.2013.850127. PMID:24304307
- Ito S, Shen L, Dai Q, Wu SC, Collins LB, Swenberg JA, He C, Zhang Y. Tet proteins can convert 5-methylcytosine to 5-formylcytosine and 5-carboxylcytosine. Science. 2011;333:1300-3. doi:10.1126/science.1210597. PMID:21778364
- Aryee MJ, Jaffe AE, Corrada-Bravo H, Ladd-Acosta C, Feinberg AP, Hansen KD, Irizarry RA. Minfi: a flexible and comprehensive Bioconductor package for the analysis of Infinium DNA methylation microarrays. Bioinformatics. 2014;30:1363-9. doi:10.1093/bioinformatics/btu049. PMID:24478339
- Chen EY, Dobrinski KP, Brown KH, Clagg R, Edelman E, Ignatius MS, Chen JY, Brockmann J, Nielsen GP, Ramaswamy S, et al. Cross-species array comparative genomic hybridization identifies novel oncogenic events in zebrafish and human embryonal rhabdomyosarcoma. PLoS Genet. 2013;9:e1003727. doi:10.1371/journal.pgen.1003727. PMID:24009521
- Wilson CL, Miller CJ. Simpleaffy: a BioConductor package for Affymetrix Quality Control and data analysis. Bioinformatics. 2005;21:3683-5. doi:10.1093/bioinformatics/bti605. PMID:16076888
- Gautier L, Cope L, Bolstad BM, Irizarry RA. affy-analysis of Affymetrix GeneChip data at the probe level. Bioinformatics. 2004;20:307-15. PMID:14960456. doi:10.1093/bioinformatics/btg405
- Ritchie ME, Phipson B, Wu D, Hu Y, Law CW, Shi W, Smyth GK. limma powers differential expression analyses for RNA-sequencing and microarray studies. Nucleic Acids Res. 2015;43:e47. doi:10.1093/nar/gkv007. PMID:25605792
- Stalteri MA, Harrison AP. Interpretation of multiple probe sets mapping to the same gene in Affymetrix GeneChips. BMC Bioinformatics. 2007;8:13. doi:10.1186/1471-2105-8-13. PMID:17224057
- Wickham H. ggplot2: Elegant Graphics for Data Analysis. Springer Science & Business Media; 2009.
- Lawrence M, Huber W, Pages H, Aboyoun P, Carlson M, Gentleman R, Morgan MT, Carey VJ. Software for computing and annotating genomic ranges. PLoS Comput Biol. 2013;9:e1003118. doi:10.1371/journal.pcbi.1003118. PMID:23950696
- Wolfe D, Dudek S, Ritchie MD, Pendergrass SA. Visualizing genomic information across chromosomes with PhenoGram. BioData Min. 2013;6:18. doi:10.1186/1756-0381-6-18. PMID:24131735