ABSTRACT
In acute myeloid leukemia (AML) DNA hypermethylation of gene promoters is frequently observed and often correlates with a block of differentiation. Treatment of AML patients with DNA methyltransferase inhibitors results in global hypomethylation of genes and, thereby, can lead to a reactivation of the differentiation capability. Unfortunately, after termination of treatment both hypermethylation and differentiation block return in most cases. Here, we apply, for the first time, a computational model of epigenetic regulation of transcription to: i) provide a mechanistic understanding of the DNA (de-) methylation process in AML and; ii) improve DNA demethylation treatment strategies. By in silico simulation, we analyze promoter hypermethylation scenarios referring to DNMT dysfunction, decreased H3K4me3 and increased H3K27me3 modification activity, and accelerated cell proliferation. We quantify differences between these scenarios with respect to gene repression and activation. Moreover, we compare the scenarios regarding their response to DNMT inhibitor treatment alone and in combination with inhibitors of H3K27me3 histone methyltransferases and of H3K4me3 histone demethylases. We find that the different hypermethylation scenarios respond specifically to therapy, suggesting that failure of remission originates in patient-specific deregulation. We observe that inappropriate demethylation therapy can result even in enforced deregulation. As an example, our results suggest that application of high DNMT inhibitor concentration can induce unwanted global gene activation if hypermethylation originates in increased H3K27me3 modification. Our results underline the importance of a personalized therapy requiring knowledge about the patient-specific mechanism of epigenetic deregulation.
Introduction
There is increasing evidence that tumors can originate from deregulation of the epigenetic machinery, which subsequently induce changes of the chromatin structure and gene expression. Hallmarks of epigenetic deregulation during tumor development are changes in DNA methylation and histone modifications. In different tumor types, e.g., leukemia,Citation1 colon cancer,Citation2 and glioblastoma,Citation3 different tumor subclasses have been identified based on DNA methylation patterns. These patterns often correlate with prognosis. Here, we focus on DNA methylation changes observed in acute myeloid leukemia (AML) and on strategies to reverse these changes.
In AML, only a few mutations are found. However, among the most frequently mutated genes, many, such as IDH1/2, TET2, or Dnmt3a, are related to aberrant DNA methylation patterns.Citation4 Based on such patterns, 16 AML subtypes have been classified.Citation1 A typical DNA methylation pattern of AML shows both hypermethylation and hypomethylation of CpGs. AML hypermethylated CpGs are mostly located in gene promoters. In contrast, AML hypomethylated CpGs are mainly detected in gene bodies. Hypermethylation of promoter CpG islands of tumor suppressor genes often leads to downregulation of these genes. This process is probably one of the best studied epigenetic changes in tumors.Citation5-7 To revert this kind of gene repression, inhibitors of the maintenance DNA methyltransferase DNMT, have been applied for a long time in therapies.Citation8 Most of these clinical applications focused on hematological malignancies such as AML. Nevertheless, DNMT1 inhibitors have been tested also for solid tumors.Citation9
The most frequently used DNMT inhibitors are 5-azacitidine (AZA) and 5-aza-2′deoxycytidine (DAC). Both agents affect human AML cell lines by inducing: i) DNMT methyltransferase degradation;Citation10,11 ii) DNA hypomethylation;Citation12 and iii) DNA damage.Citation13 The disadvantage of these epigenetic drugs is that they act globally. Moreover, previous investigations have shown that after termination of demethylation treatment with AZA or DAC, hypermethylation of gene promoters is restored after short times. In fact, genes that become activated during the treatment are silenced again in 2–4 weeks after treatment.Citation14 Thus, although typically 15–20% of patients receiving these drugs reach complete remission (CR),Citation15 a prolonged remission requires continuous cycles of administration. Here, we aim at understanding why only such a limited number of patients reach CR. We ask whether inhibitor dosage and timing actually play an important role in DNMT inhibitor treatment and whether the optimization of treatment protocols can improve outcome. Eventually, we aim at identifying strategies that enable sustained treatment effects.
One promising approach to improve outcome appears to be the design of therapies that combine DNMT inhibitors and modulators of histone methylation. A rationale for this concept is the crosstalk between DNA and histone methylation.Citation16 Histone marks can either suppress DNA methylation or contribute its recruitment.Citation17 Effectors of histone marks have been already tested experimentally. An example is 3-Deazaneplanocin-A (DZNep), an inhibitor of the methionine metabolism, which has the capability to reactivate genes that are repressed by tri-methylation of histone H3 at lysine 27 (H3K27me3). By combining AZA with DZNep, the time for re-occurrence of the hypermethylated state can be retarded.Citation18 Here, we aim at a mechanistic understanding of such effects. For this purpose, we apply in silico simulation of demethylation therapy.
Recently, we have developed a single cell-based computational model that allows analyzing the crosstalk between gene expression, H3K4me3 of nucleosomes associated with the gene promoter, and DNA methylation of promoter CpGs by means of simulation studies. We have successfully applied our model to explain the aging processes of: i) haematopoietic stem cells in their in vivo nicheCitation19 and ii) mesenchymal stem cells during prolonged in vitro expansion.Citation20
H3K4me3 is a transcription activating histone modification. As an extension of our model, we here include the histone modification H3K27me3, which is associated with gene repression.Citation21 If both histone modifications are present at the nucleosomes of a promoter a bivalent state is established, where the gene is more or less repressed. Both H3K4me3 and H3K27me3 at gene promoters are repressed by DNA methylation. We here study by in silico simulation how the interplay between DNA and histone modification is involved in DNA hypermethylation scenarios and how it shapes the response to DNA demethylation therapies applying Dnmt1 inhibitors alone or combining DNMT1 inhibitors and histone modifying drugs.
Methods
For simulation of DNA hypermethylation scenarios and DNA demethylation treatment, we build on a multi-scale model of epigenetic regulation of transcription.Citation19,22,23 The model allows simulation of normal and disturbed epigenetic and transcriptional states of cells over time. It describes a population of cells in which all cells carry the same artificial genome (AG) consisting of 100 kb and encoding for up to 100 genes. The transcription of these genes is controlled by the transcription factor network they encode (containing both activators and repressors) and by the histone modifications H3K4me3 and H3K27me3 at the promoter of the genes. The histone methyltransferases (HMT) setting these modifications interact with methylated CpGs within the promoter region. A brief overview of the basic model assumptions can be found in Supplement A.
In , the regulatory network used throughout this study is shown. H3K4me3 forms a positive feedback loop with transcription (RNA Polymerase II binding). Thus, increased H3K4me3 levels at the gene promoter increase the transcription of the associated geneCitation24 and increased transcription increases the H3K4me3 level.Citation25 H3K27me3 forms a negative feedback loop with transcription (RNA Polymerase II binding). Thus, increased H3K27me3 levels decrease the transcription of the associated geneCitation26 and increased transcription decreases H3K27me3.Citation27 DNA methylation impacts transcription only indirectly. It is assumed to weaken the binding of H3K4me3Citation28 as well as H3K27me3Citation27 HMTs to the promoter regions. On the other hand binding of de novo DNMTs is assumed to be repressed by H3K4me3Citation29 and facilitated by H3K27me3.Citation30
Figure 1. Regulatory network considered in the model. H3K4me3 modification and transcription form a positive feedback loop, while H3K27me3 modification and transcription form a negative one. Both loops implicate interaction between the marks and RNA polymerase II (Pol II) binding at the gene promoter. DNA methylation (DNAme) suppresses both H3K4me3 and H3K27me3 by suppressing recruitment of the respective HMTs. Recruitment of DNMTs is repressed by H3K4me3 but strengthened by H3K27me3.
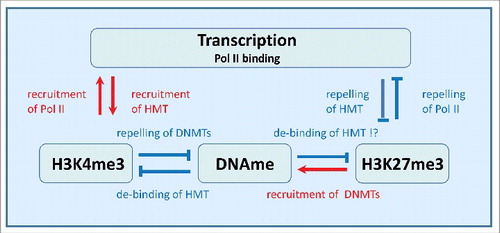
In our model, DNA methylation can change subsequent to cell division only. It is regulated by the maintenance DNA methyltransferase DNMT1 and by the de novo DNA methyltransferases DNMT3A/B, which ensure inheritance of existing methylation and confer setting of new methylation, respectively. Histone methylation underlies permanent (de-)modification. In addition, it becomes diluted after cell division, as modified histones of the mother cell are randomly distributed onto the daughter cells. Thereby, spontaneous de-modification can occur in case of bistable histone modification states.Citation31 In case of H3K4me3 loss, this will enable DNA methylation of formerly un-methylated promoters. However, even if the H3K4me3 modification of the mother cell was restored, the transient de-modification would open a time window for DNA methylation of the gene promoter. We have demonstrated that this behavior potentially results in epigenetic drifts, including progressive DNA hypermethylation at certain gene promoters, a process that is observed during aging.Citation19
Our model contains parameters, such as rates for histone (de-) modification and for maintenance and de novo DNA methylation, that characterize the histone modification and DNA methylation machinery. In addition, parameters such as ‘maximum promoter activity’ characterize the transcription machinery and parameters as the ‘cell cycle time’ specify cell properties. In our simulations, we consider all CpGs to be initially un-methylated, and all nucleosomes to be modified with both H3K4me3 and H3K27me3. Transcription of each gene is initialized by an equally distributed random number between 0 and 1. We start each simulation with 100 cells. The individual cells perform growth steps (ΔV) with a probability r per time step (Δt) and divide if the initial cell volume V0 is doubled. To keep the size of the cell population limited, cells undergo apoptosis with probability (a*Nt) per Δt, where Nt is the number of cells at time t. We started recording the systems behavior after 2000 simulated time steps Δt, referring to 20 d. All simulations have been performed for different realizations of the AG to exclude biases related to a specific realization. Model equations and the parameter set used in this study are given in Supplement A and Table A1, respectively.
Results
Different origins of promoter hypermethylation
In the following, we investigate 4 possible promoter hypermethylation scenarios. We consider hypermethylation caused by: S1) accelerated cell proliferation, which affects DNA methylation via destabilization of H3K4me3 and H3K27me3; S2) increased de novo DNA methylation probability; S3) decreased activity of the H3K4me3 HMT and; S4) decreased activity of the H3K27me3 histone demethylase (HDM). The latter 2 facilitating DNMT3A binding/recruitment to the promoter.
In AML, the scenarios S1–3 can originate in loss (or gain) of function mutations of cell cycle genes (S1), such as FLT3,Citation32 the gene encoding DNMT3ACitation33 (S2), or genes encoding H3K4me3 HMTs (S3), such as MLL.Citation34 A mutation of H3K27me3 HDMs (S4), e.g., UTX, has not been reported in AML. However, all scenarios can also be a consequence of transcriptional and metabolic deregulation due to genomic instability in general.Citation35 Gene repression by H3K27me3, as associated with S4, has been shown to be required for specific AML subtypes.Citation36
In our model, accelerated proliferation (S1) is simulated assuming a decreased average cell cycle time (−50%), i.e., decreasing the growth steps from 20 to 10, 1 d instead of 2 d, being at the lower end of measured values.Citation37 A higher de novo DNA methyltransferase activity (S2) is simulated assuming an increased modification probability by DNMT3A/B (+50%). Decreased H3K4me3 HMT activity (S3) is described by decreasing the H3K4me3 modification rate (−50%); decreased H3K27me3 HDM activity (S4) is described by decreasing the H3K27me3 de-modification rate (−50%). All the assumed enzymatic changes are rather moderate (see, e.g., HMT activity of H3K4me3 HMT variantsCitation38). The effects of scenarios S1–4 on the epigenetic and transcriptional states, i.e., the regulatory states of the cells, are shown in .
Figure 2. Changes of regulatory states following the induction of hypermethylation. A) Heatmaps of the time development of these changes (differences) for all genes of the simulated network averaged over all cells. Compared are the hypermethylation scenarios S1–4. The averaged cell cycle time was set to 2 d (1 d for the accelerated proliferation in S1). The onset of simulated hypermethylation is at t = 30 d in all scenarios. Colors are normalized to minimum and maximum values in each of the 16 heatmaps, separately. B) Hypermethylation scenarios (red: S1, blue: S2, green: S3, cyan: S4) increase the variance of CpG methylation compared with the control (R). Shown are averaged variances of DNA methylation from all single CpGs.
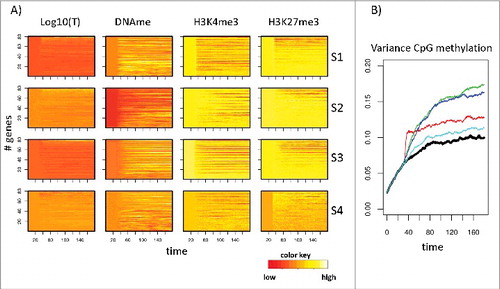
Simulation results for the changes (differences) of these states for all 4 scenarios are compared with each other (absolute values are shown in Supplement B, Fig. B1). Here, and in the following figures, transcription is provided as the logarithm of the simulated promoter activity, while histone modifications and DNA methylation are quantified as fractions of the modified histones and the fraction of methylated CpGs associated with the promoter of the gene, respectively. As reference system (R), we used a normal cell population showing age-related promoter hypermethylation but having not been subject to a specific hypermethylation scenario.
After onset of the scenarios at time point t = 30 d, changes in the epigenetic states are obvious for all scenarios, while changes in the transcriptional states are in general small. Nevertheless, expression of selected genes becomes deregulated (details see below). shows averages for the individual genes over all cells of the simulated population. However, individual cells undergo individual regulation and, thus, may differ in their regulatory states. Such variance has been shown to affect AML progression.Citation39 To quantify this kind of heterogeneity, we calculated the inter-cell variance of DNA methylation for all scenarios. We observed significant differences between S1-S4, with the highest variance being present following increased de novo DNMT activity (S2) and decreased activity of H3K4me3 HMT (S3).
Hypermethylation scenarios can be distinguished by the induced regulatory states
Next, we asked whether the regulatory states induced by scenarios S1–4 could be distinguished applying an unsupervised cluster algorithm. Thus, we simulated 3 replicates for each scenario by repeating the simulations with different random number seeds. Afterwards, we compared the regulatory states of scenarios S1–4 and of the reference scenario R applying a cluster algorithm to all state variables, i.e., transcription, DNA methylation, and trimethylation of H3K4 and H3K27. shows the results of this comparison. Although the parameter changes associated with the respective scenario are rather moderate (± 50% of the reference value), for each variable all simulated replicates of all individual scenarios cluster together. Thus, moderate parameter changes are sufficient to distinguish S1–4 among each other and from the reference scenario R.
Figure 3. Hierarchical clustering of hypermethylation scenarios. The scenarios S1–4 together with the reference scenario R have been clustered according to transcription, DNA methylation, H3K4me3, and H3K27me3 profiles. All 4 regulatory states have been measured at t = 70 d, i.e., 40 d after onset of the scenarios. For each scenario, 3 in silico replicates have been simulated. The replicates of all scenarios cluster together, independently of the underlying type of data. A total of 1500 CpGs were used for the clustering of DNA methylation. All genes and all nucleosomes are used otherwise.
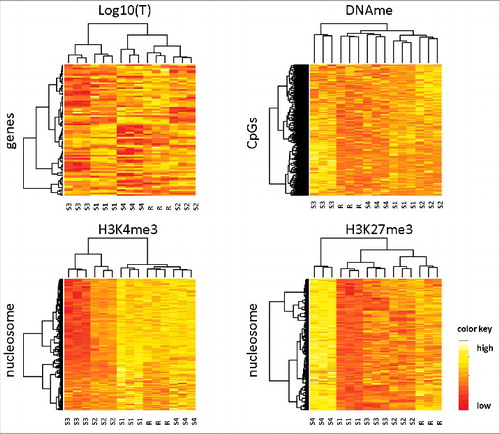
Transcriptional changes of genes during hypermethylation depend on the scenario and their prior histone states
Our clustering results suggest that different hypermethylation scenarios affect different subsets of genes. To strengthen this hypothesis, we analyzed their effects on individual genes in more detail. For each realization of a scenario, we identified genes that become transcriptionally activated or repressed. We selected genes that changed transcription at least by 30% (ΔT = ±0.3T), when measured and averaged over all time points between day 40 and 90. Subsequently, we analyzed changes of the epigenetic states in these 2 subgroups of genes separately and quantified the distribution of these changes. shows results of this analysis. Obviously, scenarios S1–4 all show different characteristics, although there are also some common features. In scenarios S1–3, downregulated genes often lose H3K4me3 and most of these genes also lose H3K27me3. In these cases, transcriptional downregulation is often associated with increasing DNA methylation leading to a destabilization of both H3K4me3 and H3K27me3 (see Supplement B, Fig. B2). In scenario S4, in contrast, most of the downregulated genes do not change histone modification. Here, transcriptional downregulation is an effect of the TF network. Upregulation of genes in scenarios S1–3 is frequently an effect of the TF network as well and not associated with changes in the histone modifications. Somewhat contra-intuitively, upregulated genes in scenarios S1–3 alternatively lose both H3K4me3 and H3K27me3, similar to the described effects in downregulated genes. Detailed inspection of these genes revealed that whether they become up- or downregulated is dependent on their histone modification state before onset of hypermethylation (see Supplement B, Fig. B2).
Figure 4. Effects of hypermethylation on histone modification states. Shown are percent of genes repressed (top row, ΔT = −0.3T) or activated (bottom row, ΔT = 0.3T) in S1-S4 (5 different genome realizations, 344 genes in total). Genes are classified according to changes in H3K4me3 (up, down, no). For each class, the fraction of genes where H3K27me3 is changed in a defined manner (up, down, no) is provided.
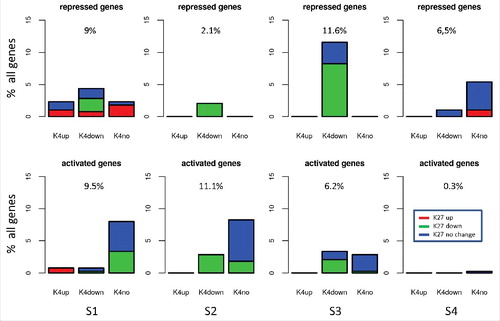
In case the fraction of H3K4me3 modified histones at a gene promoter is larger than the fraction of H3K27me3 modified histones, loss of modifications is more pronounced for H3K4me3 and the gene becomes downregulated. This occurs mainly for activated genes. For the opposite case, loss of modifications is more pronounced for H3K27me3 and the gene becomes upregulated. This occurs mainly for repressed genes. Notably, for scenario S4, upregulation is not a characteristic event and is seen only in a few genes.
In summary, our analyses for moderate to strong transcriptional changes (ΔT = ± 0.3T) demonstrate that, indeed, different hypermethylation scenarios affect different genes. Nevertheless, considering all transcriptional changes (ΔT<>0), we observed also a small subgroup of genes showing common changes for S1–3 (see supplement B, Fig. B3). This is in agreement with experimental findings showing that a small group of genes is similarly regulated in all AML cases.Citation1
Simulated demethylation treatment: Transient effects and long-term epigenetic deregulation
Next, we aimed at examining the effects of drug-based treatment strategies targeting hypermethylation. For this purpose, we simulated treatment by DNMT1 inhibitors (such as AZA or DAC), H3K27me3 inhibitors (such as DZNEP, D9)Citation40 and H3K4me3 HDM inhibitors (such as LSD1 inhibitor SP2509Citation41), and combinations of these inhibitors. The effect of DNMT1 inhibitors was modeled assuming degradation of the DNMT1 proteins over a defined time window and simulated decreasing the probability of inheritance of the CpG methylation from 0.95 to 0.90, 0.85, 0.80, 0.75, and 0.70. This decrease resulted in a reduction of the equilibrium promoter DNA methylation from about 27% down to 5% (see Supplement B, Fig. B4). This mimics the concentration-dependent effect of DAC on HEK293 cells observed in vitro.Citation42 Increasing DAC from 0.25 to 2.0 µM reduces the global DNA methylation level from about 60% down to less than 20%.
AZA is incorporated in both, RNA and DNA,Citation43 while DAC is incorporated in DNA only.Citation11 However, in trials, both confer similar meaningful clinical activity.Citation15 Thus, we modeled AZA and DAC treatment identically. The effect of H3K27me3 HMT inhibitors was simulated assuming a globally reduced H3K27me3 HMT activity, i.e., considering H3K27me3 modification rate reduced by a factor of 2. Analogously, H3K4me3 HDM inhibitors are simulated, considering H3K4me3 de-modification rate reduced by a factor of 2. We applied these changes between the time points t = 70 d and t = 80 d, i.e., for 10 d, as applied in real trials.Citation44 This refers to 10 cell cycles in S1 (accelerated proliferation) and 5 cell cycles otherwise. At the time point of onset of the therapy, the hypermethylation scenarios are already active for 40 d.
show the regulatory states averaged over all cells and all genes for 3 selected treatment scenarios. An overview over all simulated treatment scenarios is provided in Supplement C, Fig. C1). As expected, strong DNA demethylation changes are observed after treatment with DNMT1 inhibitor. In these cases, the treatment-induced overall demethylation is much stronger than the global hypermethylation accumulated until its onset. Thus, an effective hypomethylation is induced. Nevertheless, after termination of the treatment, DNA methylation is re-established. It reaches levels of normal aging (scenario R) after about 20 d and pre-treatment levels after 40 d. This roughly agrees with experimentally observed re-methylation times of 2–4 weeks.Citation14
Figure 5. Simulated regulatory states during demethylation treatment. Shown are results for transcription, DNA methylation, as well as H3K4me3 and H3K27me3 levels for scenarios S1–4 and the reference scenario R. Averages are taken over all cells and all genes at each time point. The treatment starts at t = 70 d and is terminated at t = 80 d. Selected scenarios: A) System without treatment; B) DNMT1 inhibitor treatment; C) H3K27me3 HMT inhibitor treatment; D) Combined DNMT1 and H3K27me3 HMT inhibitor treatment. DNMT1 inhibition was simulated with Dmain = 0.7.
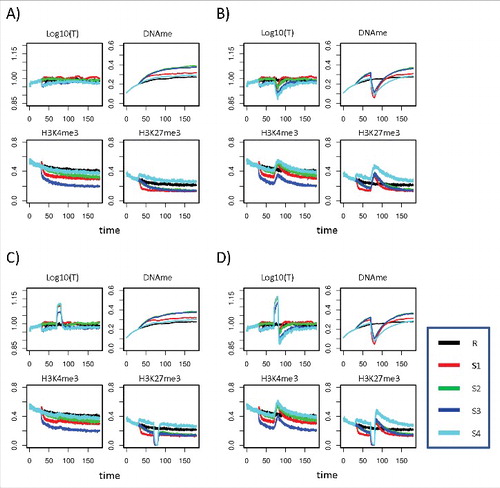
Changes of H3K4me3 and H3K27me3 induced by the treatment vary. Strikingly, treatment with DNMT1 inhibitors can increase H3K27me3 to levels higher than those observed in reference cells for more than 50 d (S4; see , ). Such behavior was observed for different realizations of the AG. Thus, our model suggests that treatment can induce unexpected long-term epigenetic deregulation. In general, different hypermethylation scenarios respond differently to treatment.
Strategies for optimizing demethylation treatment
In agreement with experimental observations, in our model, treatment by AZA/DAC or by combinations of these agents with histone modifying ones confers only transient DNA demethylation. Thus, preservation of the induced demethylation requires repeated application of the therapy. In clinical trials, treatment is repeated dependent on response and toxicityCitation45 (e.g., 10 d AZA repeated after 28 d, 6–24 cycles).Citation44 To estimate the effects of repeated treatment on transcriptional regulation, we simulated repeated application of DNMT1 inhibitors, H3K27me3 HMT inhibitors, H3K4me3 HDM inhibitors, and combinations.
Our simulations show relapse of hypermethylation within 20–40 d after a treatment phase of 10 d and thus suggest a treatment cycle of 30–50 d in accordance with the choice made in clinical trials. However, treatment over 10 d induced very strong demethylation effects (see , ). Thus, we considered shorter treatment phases of 4 d similar to ongoing studiesCitation46 (5 d, repeated after 28 d, >6 cycles). This shorter treatment phase clearly induces weaker DNA demethylation effects, which are reversed at shorter times. In simulation series, we found that effects of 4 d treatments are reversed within about 16 d. Thus, we considered a treatment cycle of 20 d. In each simulation, 4 treatment cycles have been followed up. We performed simulations with different concentrations of the DNMT1 inhibitor modeled by reducing the DNA maintenance probability from 0.95 to 0.90, 0.85, 0.80, 0.75, and 0.70. As criterion for optimal treatment we chose a minimal standard deviation (SD) between reference and treated transcription profiles ().
Figure 6. Effects of repeated treatment. A) Comparison of the DNA methylation kinetics following 10 d treatment (dotted green curve) and 4 times repeated 4 d treatment (cycle length 20 d, solid green curve) for S2. For comparison, DNA methylation without treatment is shown as well (black line). B) Regulatory states during DNMT1 inhibitor treatment (Dmain = 0.7). C) Standard deviations of the transcriptional states of all genes from the reference scenario (R). Shown are the results for all treatment types and all hypermethylation scenarios. S1-S4 are compared with the reference system not being subjected to any treatment (gray bar). Left panel: Moderate repression of DNMT1 modification probability from 0.95 to 0.90. Right panel: Strong repression of DNMT1 modification probability from 0.95 to 0.70.
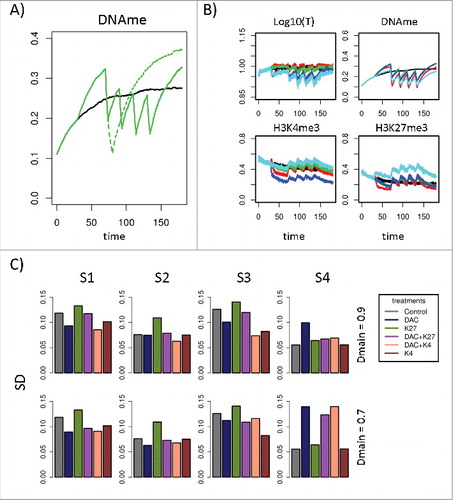
shows regulatory states during treatment with DNMT1 inhibitor only. Focusing on the epigenetic states only and, in particular, on DNA methylation, under this treatment, best results appear to be observed for hypermethylation scenario S1 (red). However, in terms of an optimal treatment, SD of the transcriptional states from the reference (R) are smaller for scenarios S2-S4. In , all treatments are compared with respect to this SD. For comparison, the SD of an untreated system is shown as well. Without treatment, the strongest SDs are observed for scenarios S1 and S3, while the deviations for scenarios S2 and S4 are rather weak. The best treatment suggested by the model is DNMT1 inhibition alone or in combination with H3K4me3 stabilization at moderate DNMT1 inhibitor concentration. For higher inhibitor concentrations, the treatment is inappropriate for scenarios S3 and S4. An alternative treatment appears to be H3K4me3 stabilization alone. H3K27me3 inhibition alone appears to be not appropriate as it increases the SD of transcription from the reference state for scenarios S1–3. Combination of DNMT1 and H3K27me3 HMT inhibition is not appropriate because it does not correct transcription at moderate DNMT1 inhibitor concentration and for higher concentrations; it even increases deviations in case of scenario S4. This behavior was consistently observed for different realizations of the AG.
Considering the differences between the treatment results for scenarios S1–4, our results suggest that an effective treatment should either be patient specific or be capable of reversing gene expression for different hypermethylation scenarios. Regarding the latter, treatment with moderate DNMT1 inhibitor concentrations alone, as practiced in clinics, indeed appears to be a good choice.
Combined hypermethylation scenarios in AML
To gain insight into the mechanisms that might actually dominate hypermethylation in AML, we reanalyzed matched DNA methylation and gene expression data together with mutation and French-American-British (FAB) classification data provided by the TCGA consortium.Citation4 We applied a SOM-method (oposSOMCitation47) to define AML subgroups based on the TCGA expression data. We identified 5 groups, AML1–5, with 24, 33, 32, 38, and 64 members, respectively (Supplement C, Table C1). The groups nicely separate as demonstrated by their group expression portraits () and a correlation network plot (Supplement C, Fig. C2A). Group membership of the individual samples was defined based on the average activation of all genes associate with all metagenes of the group-specific overexpression spots (, red spots). As a threshold, we used the simple standard deviation from mean expression. Although the classification is based on expression data only, specific DNA methylation portraits are observed for all groups (). Moreover, the groups show specific enrichment for different mutations frequently found in AML, among them, mutations of NPM1, DNMT3A, and FLT3. Formation of the fusion proteins PML-RARA and MYH11-CBFB is exclusively seen in group AML4 and 5, respectively (Supplement C, Fig. C2B). In addition, groups AML4 and 5 comprise nearly all samples of FAB class M3 and M4, respectively (Supplement C, Fig. C2C). Notably, all groups show a similar age distribution. For all AML groups, we calculated the average expression of a set of 27 epigenetic modifiers including HMTs, HDMs, and DNMTs (). The groups show specific expression of H3K4me3 HDMs (e.g., KDM5B) and HMTs (e.g., KMT2F), of H3K27me3 HDMs (e.g., KDM6B), and of regulators of DNA methylation (e.g., DNMT3A/B). These results support our assumptions of scenarios with differentially regulated expression of epigenetic modifiers. However, all groups show deregulation of several modifiers in parallel. We found, for example, expression of DNMT3A and KDM5B to be positively correlated, suggesting combined effects in individual patients (). In addition, expression of these genes is correlated with that of cell cycle genes, such as CCND2 ().
Figure 7. Combined hypermethylation effects. A) SOM portraits of the AML subgroups AML1–5 (TCGA data, 20 × 20 metagenes). All groups show characteristic expression [log(E)] and DNA methylation patterns (β-values). Red: overexpression/methylation; Blue: underexpression/methylation. Shown are averages over all genes referring to the respective metagene. B) Average expression of epigenetic modifiers in the subgroups AML1–5. They show differential expression of H3K4 HMT and HDM, as well as of DNMT and TET genes (P values: green border: <0.05; red border: <0.01; black border: <0.001; t-test). C, D) Correlation between KDM5B and DNMT3A (C) and between CCND2 and DNMT3A (D) expression for all patients. Regardless of a considerable variance a clear correlations are observed (lines: linear regression).
![Figure 7. Combined hypermethylation effects. A) SOM portraits of the AML subgroups AML1–5 (TCGA data, 20 × 20 metagenes). All groups show characteristic expression [log(E)] and DNA methylation patterns (β-values). Red: overexpression/methylation; Blue: underexpression/methylation. Shown are averages over all genes referring to the respective metagene. B) Average expression of epigenetic modifiers in the subgroups AML1–5. They show differential expression of H3K4 HMT and HDM, as well as of DNMT and TET genes (P values: green border: <0.05; red border: <0.01; black border: <0.001; t-test). C, D) Correlation between KDM5B and DNMT3A (C) and between CCND2 and DNMT3A (D) expression for all patients. Regardless of a considerable variance a clear correlations are observed (lines: linear regression).](/cms/asset/9e63976c-6596-48be-9b75-14c89c4e47af/kepi_a_1361090_f0007_c.jpg)
Motivated by these findings, we performed simulations of a combined hypermethylation scenario. We assumed a superposition of S1, S2, and S3 and simulated treatment of this combined scenario with a DNMT1 inhibitor alone. Here, we observed a clear benefit for longer treatment phases, leading to stronger DNA demethylation and larger time intervals of corrected gene expression (Supplement C, Fig. C3).
Discussion
Aberrant DNA methylation in AML can originate from different mutation scenarios. In our study, we demonstrate that such differences are sufficient to induce also different DNA methylation patterns. Actually, measured differences in DNA methylation pattern have been used for AML sub-classification. However, such classification has not rigorously been used to unravel the cause of patient-specific deregulation. Consequently, the cause of several patterns remains speculative.Citation48 We demonstrated that such knowledge is essential for decisions about applicability and design of AML demethylation therapies.
We analyzed promoter DNA hypermethylation scenarios that are driven either directly by activated DNMTs or indirectly by aberrant H3K4 and H3K27 trimethylation. Our simulation results suggest that, among these cases, patients with deregulated DNA methylation due to decreased H3K4me3 HMT activity or accelerated proliferation, both de-stabilizing H3K4me3, show strongest deregulation. As general effective DNA demethylation therapies, we identified treatment with DNMT1 or H3K4me3 HDM inhibitors alone. Thereby, our criterion for optimal treatment was overall corrected gene expression, instead of successful DNA demethylation or overall potential for gene activation.Citation18 The latter criterion suggests that application of H3K27me3 HMT inhibitors (in combination with DNMT1 inhibitors) is an effective treatment. This treatment strategy appears to be obvious, as strong repressors of proliferation and stem cell maintenance are themselves controlled by H3K27me3-mediated repression.Citation36 However, the gene activation gained by downregulation of H3K27me3 is global and thus associated with uncontrolled risks, as suggested by other tumor models.Citation49 In addition, our study suggests that particular effects of deregulation might even become reinforced by this type of treatment. Considering that in most cases the cause of the hypermethylation is unknown, these results suggest that treatment surveillance is important to avoid related side effects. Recent study protocols do already include such surveillance (surveillance after cycle 2, 4, and 6).Citation46
After termination of treatment, hypermethylation is re-established in most experimental AML models. There is a single report on imprinted hypomethylation if applied to non-cancer cell types.Citation42 In our simulations, we see re-establishment of DNA methylation in general, although the time required might strongly vary. Thus, our simulations suggest that longer-term DNA demethylation and thus longer-term complete remission (CR) in AML can only be a result of a competitive advantage of wild type vs. AML clones under treatment, resulting in eradication of the AML clones. At least one reduction has been monitored recently following epigenetic therapy.Citation50 It was found not to be necessarily correlated with blast content, i.e., with the phenotype currently relevant for stratification of outcome. Moreover, even in cases that show CR, reduction of mutation burden was not mandatory; however, they were more frequent. Thus, it appears that genetic and epigenetic development in AML is partially uncoupled. First, systematic reports on AML epi-clones support this hypothesis.Citation39 Consequently, in agreement with the original idea of demethylation therapy, the important point is whether therapy corrects gene expression in such a way that tumor suppressors become activated and can induce apoptosis in mutant cells. We here suggest that this will be hindered by differential response of different hypermethylation scenarios to therapy. Notably, alternative therapy effects of DNMT1 inhibitors have been suggested recently.Citation51,52
As suggested by the reanalyzed TCGA data, particular strong hypermethylation in AML can originate in combined effects of DNA, histone modifying enzymes, and activated proliferation. This is in agreement with recent findings of enforced DNMT3A expression in proliferation activated AML cells.Citation53 Our simulations suggest that 10 d treatment with DNMT1 inhibitor, while inducing strong demethylation effects, might also correct for such strong hypermethylation. This might explain the observed clinical benefit of longer compared with shorter treatment phases. Another problem with short treatment phases is that slowly cycling cells might not be targeted. This can relate, for example, to cells stopped at cell cycle check point or specifically silenced cells, such as AML blasts under hypoxia.Citation54 We expect that these cells are responsible for fast relapse after termination of treatment.Citation50 As a consequence, stimulation of proliferation of these cells could be beneficial, as suggested for CML treatment.Citation55,56
In the present study, we investigated scenarios of CpG-rich promoter DNA hypermethylation. In AML, mechanisms of more gene specific deregulation of DNA methylation are also active. For example, mutations of the TET2 gene can induce hypermethylated states at specific promoters where, under reference conditions, binding of the encoded protein results in active DNA demethylation.Citation48 In these scenarios, hypermethylation is not a consequence of deregulated DNMTs or pre-existent deregulation of histone marks. Such scenarios are outside of the focus of our study and require detailed knowledge about active DNA demethylation and its targets.
DNA hypermethylation of CpG-rich promoters, as discussed in the present study, has been recently characterized as being a consequence of AML progression—in particular, of enforced proliferation—and not its cause.Citation53 Thus, demethylation therapy might in general suppress progression of AML only and further treatment might be required to eradicate its stem.
Conclusions
Our study demonstrates that deregulation of different epigenetic enzymes results in specific DNA hypermethylation scenarios and that cells that are subject to these different scenarios respond differently to demethylation therapy. We suggest that an understanding of these differences is essential to design optimal therapy protocols. These protocols should be either focused on patient specifics or include drugs capable of correcting gene expression for a broad class of hypermethylation scenarios.
Disclosure of potential conflicts of interest
No potential conflicts of interest were disclosed.
Authors contribution
J.P. developed the modeling ideas, the source code, performed all simulations, analyzed data and contributed to writing. L.H. calculated the SOM and analyzed the AML subgroups. M.Lü. supervised the study for clinical DNA demethylation treatments. M.Loe supervised the study on HSCs and contributed with the writing. J.G. supervised the study, wrote the article, contributed ideas, and analyzed data. All authors read and approved the final manuscript.
Supplementary_materials.docx
Download MS Word (1.5 MB)Acknowledgments
The authors like to thank the Deutsche Forschungsgemeinschaft (DFG) SPP1463 (grant number: GA637/4–1) for financial support of this work.
References
- Figueroa ME, Lugthart S, Li Y, Erpelinck-Verschueren C, Deng X, Christos PJ, Schifano E, Booth J, van Putten W, Skrabanek L, et al. DNA methylation signatures identify biologically distinct subtypes in acute myeloid leukemia. Cancer Cell. 2010;17:13-27. doi:10.1016/j.ccr.2009.11.020. PMID:20060365
- Hinoue T, Weisenberger DJ, Lange CP, Shen H, Byun HM, Van Den Berg D, Malik S, Pan F, Noushmehr H, van Dijk CM, et al. Genome-scale analysis of aberrant DNA methylation in colorectal cancer. Genome Res. 2012;22:271-82. doi:10.1101/gr.117523.110. PMID:21659424
- Sturm D, Witt H, Hovestadt V, Khuong-Quang DA, Jones DT, Konermann C, Pfaff E, Tonjes M, Sill M, Bender S, et al. Hotspot mutations in H3F3A and IDH1 define distinct epigenetic and biological subgroups of glioblastoma. Cancer Cell. 2012;22:425-37. doi:10.1016/j.ccr.2012.08.024. PMID:23079654
- CGARN. Cancer Genome Atlas Research Network: Genomic and epigenomic landscapes of adult de novo acute myeloid leukemia. N Engl J Med. 2013;368:2059-74. doi:10.1056/NEJMoa1301689. PMID:23634996
- Jones PA, Baylin SB. The epigenomics of cancer. Cell. 2007;128:683-92. doi:10.1016/j.cell.2007.01.029. PMID:17320506
- Esteller M. Epigenetics in cancer. N Engl J Med. 2008;358:1148-59. doi:10.1056/NEJMra072067. PMID:18337604
- Baylin SB, Jones PA. A decade of exploring the cancer epigenome - biological and translational implications. Nat Rev Cancer. 2011;11:726-34. doi:10.1038/nrc3130. PMID:21941284
- Saiki JH, McCredie KB, Vietti TJ, Hewlett JS, Morrison FS, Costanzi JJ, Stuckey WJ, Whitecar J, Hoogstraten B. 5-azacytidine in acute leukemia. Cancer. 1978;42:2111-4. doi:10.1002/1097-0142(197811)42:5. PMID:82472<2111::AID-CNCR2820420505>3.0.CO;2-I
- Nervi C, De Marinis E, Codacci-Pisanelli G. Epigenetic treatment of solid tumours: A review of clinical trials. Clin Epigenetics. 2015;7:127. doi:10.1186/s13148-015-0157-2. PMID:26692909
- Ghoshal K, Datta J, Majumder S, Bai S, Kutay H, Motiwala T, Jacob ST. 5-Aza-deoxycytidine induces selective degradation of DNA methyltransferase 1 by a proteasomal pathway that requires the KEN box, bromo-adjacent homology domain, and nuclear localization signal. Mol Cell Biol. 2005;25:4727-41. doi:10.1128/MCB.25.11.4727-4741.2005. PMID:15899874
- Stresemann C, Bokelmann I, Mahlknecht U, Lyko F. Azacytidine causes complex DNA methylation responses in myeloid leukemia. Mol Cancer Ther. 2008;7:2998-3005. doi:10.1158/1535-7163.MCT-08-0411. PMID:18790780
- Flotho C, Claus R, Batz C, Schneider M, Sandrock I, Ihde S, Plass C, Niemeyer CM, Luebbert M. The DNA methyltransferase inhibitors azacitidine, decitabine and zebularine exert differential effects on cancer gene expression in acute myeloid leukemia cells. Leukemia. 2009;23:1019-28. doi:10.1038/leu.2008.397. PMID:19194470
- Kiziltepe T, Hideshima T, Catley L, Raje N, Yasui H, Shiraishi N, Okawa Y, Ikeda H, Vallet S, Pozzi S, et al. 5-Azacytidine, a DNA methyltransferase inhibitor, induces ATR-mediated DNA double-strand break responses, apoptosis, and synergistic cytotoxicity with doxorubicin and bortezomib against multiple myeloma cells. Mol Cancer Ther. 2007;6:1718-27. doi:10.1158/1535-7163.MCT-07-0010. PMID:17575103
- Wodarz D, Boland CR, Goel A, Komarova NL. Methylation kinetics and CpG-island methylator phenotype status in colorectal cancer cell lines. Biol Direct. 2013;8:14. doi:10.1186/1745-6150-8-14. PMID:23758948
- Cruijsen M, Luebbert M, Wijermans P, Huls G. Clinical results of hypomethylating agents in AML treatment. J Clin Med. 2014;4:1-17. doi:10.3390/jcm4010001. PMID:26237015
- D'Alessio AC, Szyf M. Epigenetic tête-à-tête: The bilateral relationship between chromatin modifications and DNA methylation. Biochem Cell Biol. 2006;84:463-76. doi:10.1139/o06-090. PMID:16936820
- Kouzarides T. Chromatin modifications and their function. Cell. 2007;128:693-705. doi:10.1016/j.cell.2007.02.005. PMID:17320507
- Momparler RL, Cote S, Momparler LF, Idaghdour Y. Epigenetic therapy of acute myeloid leukemia using 5-aza-2′-deoxycytidine (decitabine) in combination with inhibitors of histone methylation and deacetylation. Clin Epigenetics. 2014;6:19. doi:10.1186/1868-7083-6-19. PMID:25313314
- Przybilla J, Rohlf T, Loeffler M, Galle J. Understanding epigenetic changes in aging stem cells–a computational model approach. Aging Cell. 2014;13:320-8. doi:10.1111/acel.12177. PMID:24428552
- Hamidouche Z, Rother K, Przybilla J, Krinner A, Clay D, Hopp L, Fabian C, Stolzing A, Binder H, Charbord P, Galle, J. Bistable epigenetic states explain age-dependent decline in mesenchymal stem cell heterogeneity. Stem Cells. 2017 Mar;35(3):694-704. doi:10.1002/stem.2514. Epub 2016 Nov 8. PMID:27734598
- Cui K, Zang C, Roh T-Y, Schones DE, Childs RW, Peng W, Zhao K. Chromatin signatures in multipotent human hematopoietic stem cells indicate the fate of bivalent genes during differentiation. Cell Stem Cell. 2009;4:80-93. doi:10.1016/j.stem.2008.11.011. PMID:19128795
- Przybilla J, Buske P, Binder H, Galle J. Histone modifications control DNA methylation profiles during ageing and tumour expansion. Front Life Sci. 2013;7:31-43. doi:10.1080/21553769.2013.854279.
- Przybilla J, Rohlf T, Galle J. Towards a mechanistic understanding of epigenetic dynamics. In: Teschendorff AE, editors. Computational and statistical epigenomics.. Springer; 2015. p. 141-60.
- Vermeulen M, Mulder KW, Denissov S, Pijnappel WW, van Schaik FM, Varier RA, Baltissen MP, Stunnenberg HG, Mann M, Timmers HT. Selective anchoring of TFIID to nucleosomes by trimethylation of histone H3 lysine 4. Cell. 2007;131:58-69. doi:10.1016/j.cell.2007.08.016. PMID:17884155
- Buratowski S, Kim T. The role of cotranscriptional histone methylations. Cold Spring Harb Symp Quant Biol. 2010;75:95-102. doi:10.1101/sqb.2010.75.036. PMID:21447819
- Pasini D, Malatesta M, Jung HR, Walfridsson J, Willer A, Olsson L, Skotte J, Wutz A, Porse B, Jensen ON, et al. Characterization of an antagonistic switch between histone H3 lysine 27 methylation and acetylation in the transcriptional regulation of Polycomb group target genes. Nucleic Acids Res. 2010;38:4958-69. doi:10.1093/nar/gkq244. PMID:20385584
- Jermann P, Hoerner L, Burger L, Schubeler D. Short sequences can efficiently recruit histone H3 lysine 27 trimethylation in the absence of enhancer activity and DNA methylation. Proc Natl Acad Sci U S A. 2014;111:E3415-21. doi:10.1073/pnas.1400672111. PMID:25092339
- Thomson JP, Skene PJ, Selfridge J, Clouaire T, Guy J, Webb S, Kerr AR, Deaton A, Andrews R, James KD, et al. CpG islands influence chromatin structure via the CpG-binding protein Cfp1. Nature. 2010;464:1082-6. doi:10.1038/nature08924. PMID:20393567
- Ooi SK, Qiu C, Bernstein E, Li K, Jia D, Yang Z, Erdjument-Bromage H, Tempst P, Lin SP, Allis CD, et al. DNMT3L connects unmethylated lysine 4 of histone H3 to de novo methylation of DNA. Nature. 2007;448:714-7. doi:10.1038/nature05987. PMID:17687327
- Rush M, Appanah R, Lee S, Lam LL, Goyal P, Lorincz MC. Targeting of EZH2 to a defined genomic site is sufficient for recruitment of Dnmt3a but not de novo DNA methylation. Epigenetics. 2009;4:404-14. doi:10.4161/epi.4.6.9392. PMID:19717977
- Binder H, Steiner L, Przybilla J, Rohlf T, Prohaska S, Galle J. Transcriptional regulation by histone modifications: Towards a theory of chromatin re-organization during stem cell differentiation. Phys Biol. 2013;10:026006. doi:10.1088/1478-3975/10/2/026006. PMID:23481318
- Schnerch D, Yalcintepe J, Schmidts A, Becker H, Follo M, Engelhardt M, Wasch R. Cell cycle control in acute myeloid leukemia. Am J Cancer Res. 2012;2:508-28. PMID:22957304
- Im AP, Sehgal AR, Carroll MP, Smith BD, Tefferi A, Johnson DE, Boyiadzis M. DNMT3A and IDH mutations in acute myeloid leukemia and other myeloid malignancies: Associations with prognosis and potential treatment strategies. Leukemia. 2014;28:1774-83. doi:10.1038/leu.2014.124. PMID:24699305
- Huang G, Zhao X, Wang L, Elf S, Xu H, Sashida G, Zhang Y, Liu Y, Lee J, Menendez S, et al. The ability of MLL to bind RUNX1 and methylate H3K4 at PU.1 regulatory regions is impaired by MDS/AML-associated RUNX1/AML1 mutations. Blood. 2011;118:6544-52. doi:10.1182/blood-2010-11-317909. PMID:22012064
- Prasad P, Ronnerblad M, Arner E, Itoh M, Kawaji H, Lassmann T, Daub CO, Forrest AR, Lennartsson A, Ekwall K. High-throughput transcription profiling identifies putative epigenetic regulators of hematopoiesis. Blood. 2014;123:e46-57. doi:10.1182/blood-2013-02-483537. PMID:24671951
- Neff T, Sinha AU, Kluk MJ, Zhu N, Khattab MH, Stein L, Xie H, Orkin SH, Armstrong SA. Polycomb repressive complex 2 is required for MLL-AF9 leukemia. Proc Natl Acad Sci U S A. 2012;109:5028-33. doi:10.1073/pnas.1202258109. PMID:22396593
- Preisler HD, Raza A, Gopal V, Ahmad S, Bokhari J. Distribution of cell cycle times amongst the leukemia cells within individual patients with acute myelogenous leukemia. Leuk Res. 1995;19:693-8. doi:10.1016/0145-2126(95)98846-P. PMID:7500644
- Weirich S, Kudithipudi S, Jeltsch A. Somatic cancer mutations in the MLL1 histone methyltransferase modulate its enzymatic activity and dependence on the WDR5/RBBP5/ASH2L complex. Mol Oncol. 2017;11:373-87. doi:10.1002/1878-0261.12041. PMID:28182322
- Li S, Garrett-Bakelman FE, Chung SS, Sanders MA, Hricik T, Rapaport F, Patel J, Dillon R, Vijay P, Brown AL, et al. Distinct evolution and dynamics of epigenetic and genetic heterogeneity in acute myeloid leukemia. Nat Med. 2016;22:792-9. doi:10.1038/nm.4125. PMID:27322744
- Jiang X, Lim CZ, Li Z, Lee PL, Yatim SM, Guan P, Li J, Zhou J, Pan J, Chng WJ, et al. Functional characterization of D9, a novel deazaneplanocin A (DZNep) Analog, in targeting acute myeloid leukemia (AML). PLoS One. 2015;10:e0122983. doi:10.1371/journal.pone.0122983. PMID:25928216
- Fiskus W, Sharma S, Shah B, Portier BP, Devaraj SG, Liu K, Iyer SP, Bearss D, Bhalla KN. Highly effective combination of LSD1 (KDM1A) antagonist and pan-histone deacetylase inhibitor against human AML cells. Leukemia. 2014;28:2155-64. doi:10.1038/leu.2014.119. PMID:24699304
- Ramos MP, Wijetunga NA, McLellan AS, Suzuki M, Greally JM. DNA demethylation by 5-aza-2′-deoxycytidine is imprinted, targeted to euchromatin, and has limited transcriptional consequences. Epigenetics Chromatin. 2015;8:11. doi:10.1186/s13072-015-0004-x. PMID:25806086
- Li LH, Olin EJ, Buskirk HH, Reineke LM. Cytotoxicity and mode of action of 5-azacytidine on L1210 leukemia. Cancer Res. 1970;30:2760-9. PMID:5487063
- Prebet T, Sun Z, Figueroa ME, Ketterling R, Melnick A, Greenberg PL, Herman J, Juckett M, Smith MR, Malick L, et al. Prolonged administration of azacitidine with or without entinostat for myelodysplastic syndrome and acute myeloid leukemia with myelodysplasia-related changes: Results of the US Leukemia Intergroup trial E1905. J Clin Oncol. 2014;32:1242-8. doi:10.1200/JCO.2013.50.3102. PMID:24663049
- Blum W, Garzon R, Klisovic RB, Schwind S, Walker A, Geyer S, Liu S, Havelange V, Becker H, Schaaf L, et al. Clinical response and miR-29b predictive significance in older AML patients treated with a 10-day schedule of decitabine. Proc Natl Acad Sci U S A. 2010;107:7473-8. doi:10.1073/pnas.1002650107. PMID:20368434
- Grishina O, Schmoor C, Dohner K, Hackanson B, Lubrich B, May AM, Cieslik C, Mueller MJ, Luebbert M. DECIDER: Prospective randomized multicenter phase II trial of low-dose decitabine (DAC) administered alone or in combination with the histone deacetylase inhibitor valproic acid (VPA) and all-trans retinoic acid (ATRA) in patients >60 years with acute myeloid leukemia who are ineligible for induction chemotherapy. BMC Cancer. 2015;15:430. doi:10.1186/s12885-015-1432-5.. PMID:26008690
- Loffler-Wirth H, Kalcher M, Binder H. oposSOM: R-package for high-dimensional portraying of genome-wide expression landscapes on bioconductor. Bioinformatics. 2015;31:3225-7. doi:10.1093/bioinformatics/btv342. PMID:26063839
- Schoofs T, Berdel WE, Muller-Tidow C. Origins of aberrant DNA methylation in acute myeloid leukemia. Leukemia. 2014;28:1-14. doi:10.1038/leu.2013.242. PMID:23958917
- Oittinen M, Popp A, Kurppa K, Lindfors K, Mäki M, Kaikkonen MU, Viiri K. Polycomb Repressive Complex 2 Enacts Wnt Signaling in Intestinal Homeostasis and Contributes to the Instigation of Stemness in Diseases Entailing Epithelial Hyperplasia or Neoplasia. Stem Cells. 2017 Feb;32(2):445–457. doi:10.1002/stem.2479. Epub 2016 Sep 13
- Uy GL, Duncavage EJ, Chang GS, Jacoby MA, Miller CA, Shao J, Heath S, Elliott K, Reineck T, Fulton RS, Fronick CC, O'Laughlin M, Ganel L, Abboud CN, Cashen AF, DiPersio JF, Wilson RK, Link DC, Welch JS, Ley TJ, Graubert TA, Westervelt P, Walter MJ. Dynamic changes in the clonal structure of MDS and AML in response to epigenetic therapy. Leukemia. 2017 Apr;31(4):872–881. doi:10.1038/leu.2016.282. Epub 2016 Oct 14. PMID:27740633
- Licht JD. DNA methylation inhibitors in cancer therapy: The immunity dimension. Cell. 2015;162:938-9. doi:10.1016/j.cell.2015.08.005. PMID:26317460
- Chiappinelli KB, Strissel PL, Desrichard A, Li H, Henke C, Akman B, Hein A, Rote NS, Cope LM, Snyder A, et al. Inhibiting DNA methylation causes an interferon response in cancer via dsRNA including endogenous retroviruses. Cell. 2015;162:974-86. doi:10.1016/j.cell.2015.07.011. PMID:26317466
- Spencer DH, Russler-Germain DA, Ketkar S, Helton NM, Lamprecht TL, Fulton RS, Fronick CC, O'Laughlin M, Heath SE, Shinawi M, et al. CpG island hypermethylation mediated by DNMT3A is a consequence of AML progression. Cell. 2017;168:801-16 e13. doi:10.1016/j.cell.2017.01.021. PMID:28215704
- Drolle H, Wagner M, Vasold J, Kutt A, Deniffel C, Sotlar K, Sironi S, Herold T, Rieger C, Fiegl M. Hypoxia regulates proliferation of acute myeloid leukemia and sensitivity against chemotherapy. Leuk Res. 2015;39:779-85. doi:10.1016/j.leukres.2015.04.019. PMID:25982178
- Roeder I, Horn M, Glauche I, Hochhaus A, Mueller MC, Loeffler M. Dynamic modeling of imatinib-treated chronic myeloid leukemia: Functional insights and clinical implications. Nat Med. 2006;12:1181-4. doi:10.1038/nm1487. PMID:17013383
- Horn M, Glauche I, Muller MC, Hehlmann R, Hochhaus A, Loeffler M, Roeder I. Model-based decision rules reduce the risk of molecular relapse after cessation of tyrosine kinase inhibitor therapy in chronic myeloid leukemia. Blood. 2013;121:378-84. doi:10.1182/blood-2012-07-441956. PMID:23175686