ABSTRACT
When used during pregnancy, analgesics and psychotropics pass the placenta to enter the foetal circulation and may induce epigenetic modifications. Where such modifications occur and whether they disrupt normal foetal developme nt, are currently unanswered questions. This field of prenatal pharmacoepigenetics has received increasing attention, with several studies reporting associations between in utero medication exposure and offspring epigenetic outcomes. Nevertheless, no recent systematic review of the literature is available. Therefore, the objectives of this review were to (i) provide an overview of the literature on the association of prenatal exposure to psychotropics a nd analgesics with epigenetic outcomes, and (ii) suggest recommendations for future studies within prenatal pharmacoepigenetics. We performed systematic literature searches in five databases. The eligible studies assessed human prenatal exposure to psychotropics or analgesics, with epigenetic analyses of offspring tissue as an outcome. We identified 18 eligible studies including 4,419 neonates exposed to either antidepressants, antiepileptic drugs, paracetamol, acetylsalicylic acid, or methadone. The epigenetic outcome in all studies was DNA methylation in cord blood, placental tissue or buccal cells. Although most studies found significant differences in DNA methylation upon medication exposure, almost no differences were persistent across studies for similar medications and sequencing methods. The reviewed studies were challenging to compare due to poor transparency in reporting, and heterogeneous methodology, design, genome coverage, and statistical modelling. We propose 10 recommendations for future prenatal pharmacoepigenetic studies considering both epidemiological and epigenetic perspectives. These recommendations may improve the quality, comparability, and clinical relevance of such studies. PROSPERO registration ID: CRD42020166675.
Background
Every day, pregnant women use medications for which the scientific evidence on foetal safety is limited or inconclusive. As most medications pass both the placental and blood-brain barriers during gestation [Citation1–4], common medications such as analgesics and psychotropics may exhibit pharmaceutical effects in the foetus and potentially disrupt normal foetal development. This reasoning is based on the Developmental Origins of Health and Disease (DOHaD) hypothesis, which is a conceptual framework linking prenatal environmental exposures to health and disease in later life [Citation5–9]. Indeed, many studies have reported a variety of adverse developmental outcomes as sociated with in utero medication exposure, including developmental delays and abnormalities (comprehensively reviewed in [Citation10–18] and the textbook by Schaefer et al. [Citation19]).
T he mechanisms by which prenatal exposure to medications impacts foetal development remain largely unknown. One suggested mechanism is the direct or indirect influence of epigenetic modifications in the developing foetus [Citation9,Citation20]. Epigenetics encompasses regulatory mechanisms that can impact genome stability and gene transcription, such as histone modifications and DNA methylation (DNAm) of cytosine-phosphate-guanine sites (CpGs). Such modifications are reversible and can be influenced by both genetics and environmental factors, such as medications [Citation21], making epigenetic changes plausible mediators of the prenatal environmental impact on developmental outcomes [Citation9,Citation20].
The research on epigenetic modifications in neonates exposed to medications in utero, hereafter referred to as prenatal pharmacoepigenetics, has gained increasing attention in recent years. Although the literature on prenatal pharmacoepigenetics is growing, only one systematic review summarizing the findings on medications that potentially interfere with foetal development is available [Citation22]. However, this review only included studies on antidepressants. Therefore, the primary aim of the current review is to provide an overview of the literature on the association of prenatal exposure to psychotropics and analgesics with epigenetic outcomes. In addition, by evaluating the eligible studies from both epidemiological and epigenetic perspectives, this review also aims to provide recommendations for future prenatal pharmacoepigenetic research to improve the overall quality, comparability, and clinical relevance of prenatal pharmacoepigenetic association studies.
Methods
Search strategy
Literature searches were performed in the MEDLINE, EMBASE, PsycINFO, Scopus, and Web of Science databases. The searches were first completed on 19 January 2020, and any new studies meeting the eligibility criteria, published before 1 September 2020, were included in the final review. In addition, the reference lists of the eligible articles and references of 35 relevant reviews were screened to ensure complete coverage of the literature. Prior to performing the literature searches, a detailed search strategy and vocabulary were developed with support from experienced librarians in medicine, pharmacy, and psychology. We included studies investigating (i) prenatal exposure to (ii) psychotropics and analgesics with (iii) an epigenetic outcome. The search terms for these three criteria are listed in Supplementary Table S1. Supplementary Table S2 provides an example of a search in EMBASE. The review is reported in adherence to the Preferred Reporting Items for Systematic Reviews and Meta-Analyses (PRISMA) guidelines [Citation23], and the protocol and search strategy are available in the PROSPERO database (registration ID: CRD42020166675) [Citation24,Citation25].
Inclusion criteria
The studies included in this review were selected based on the participants, intervention/exposure, comparison group, outcome, and study design (PICOS) criteria [Citation26]. Participants were defined as children (<18 y old) prenatally exposed to psychotropics or analgesics for which epigenetic data were available. Anatomical Therapeutic Chemical (ATC) codes were used to identify medication groups in accordance with the World Health Organization ATC index [Citation27]. The exposure was defined as use of antidepressants (ATC code: N06A), psycholeptics (N05), antiepileptic drugs (AEDs; N03), analgesics (N02), or non-steroidal anti-inflammatory drugs (NSAIDs; M01A) during pregnancy. We specifically selected analgesics and psychotropics, based on the expertise of our research group, biological plausibility, and the emerging number of pharmacoepigenetic studies on analgesics and psychotropics. The comparison group included children of mothers who did not use the medication of interest during pregnancy. The outcome was epigenetic measurements in tissue samples from exposed and unexposed children (<18 y old). If the study also included data on immediate or long-term developmental outcomes in the children, we reported these as well. Studies investigating the same data sets were all eligible if they reported on different exposures and/or outcomes. Only original articles with the study designs case-control, cohort, or randomized controlled trial were included. No limitations were applied regarding the time of publication, but only articles in English or Scandinavian languages were eligible.
Data extraction
After searching and retrieving the results from the databases, any duplicates were removed in EndNote X8.2 and the remaining records uploaded to the online systematic review data management platform Rayyan [Citation28]. Two reviewers (KG and EWO) independently screened the titles and abstracts, excluding studies that did not meet the inclusion criteria. If the eligibility of a paper was unclear based on the title and abstract, it was included for the next round of screening. In the second screening, the full-text versions of all papers were read and the final exclusion of papers performed. Any disagreement between the two reviewers was resolved by a third reviewer (HMEN).
Results
Outcomes of the screening and selection process
The initial searches yielded a total of 2,159 records: 488 records in MEDLINE, 880 records in EMBASE, 88 records in PsycINFO, 194 records in Scopus, and 509 records in Web of Science (). A total of 871 duplicated records were removed, leaving 1,288 unique articles to screen the titles and abstracts in Rayyan [Citation28]. After the first screening, 1,262 papers were excluded due to being non-original studies (n = 605) or failing to meet the defined PICOS criteria (n = 657). After reading the complete texts of the 26 records remaining from the first round, we excluded 11 records due to wrong exposure according to our criteria (could not differentiate medication exposure across groups; n = 5), wrong population according to our criteria (participants were too old upon exposure or sampling; n = 3), or wrong comparison groups according to our criteria (did not include a non-medicated group; n = 3). By screening the reference lists of the 15 remaining records and 35 relevant reviews, we identified 1 additional article. Two additional studies meeting the eligibility criteria were published during the revision process (before 1 September 2020) and were also included. Consequently, a total of 18 records were included in the final review.
Figure 1. Flow chart of article screening and selection based on the template from PRISMA [Citation23]. ‘Second search’ refers to eligible studies published during the manuscript revision process.
![Figure 1. Flow chart of article screening and selection based on the template from PRISMA [Citation23]. ‘Second search’ refers to eligible studies published during the manuscript revision process.](/cms/asset/0d901c8e-7430-4121-be9a-69d033d02454/kepi_a_1903376_f0001_oc.jpg)
Overview of the eligible studies
All of the eligible articles were based on data from single birth cohorts, except for one study validating results in an independent cohort [Citation29], and one study being a randomized controlled trial [Citation30]. Of the eligible articles, nine were epigenome-wide association studies (EWASs; median sample size 241 neonates [interquartile range; IQR: 284]), and eleven were candidate gene studies (median sample size 115 neonates [IQR: 168]). Hence, two studies, including 46 and 58 neonates, combined epigenome-wide and candidate gene approaches [Citation31,Citation32]. The medications included were the psychotropics antidepressants (12 studies; median sample size 201 neonates [IQR: 354]), and AEDs (2 studies; 18 and 201 neonates), and the analgesics paracetamol (2 studies; 281 and 384 neonates), acetylsalicylic acid (1 study; 358 neonates), and methadone (1 study; 53 neonates). The epigenetic outcome investigated in all papers was DNAm, in cord blood (13 studies; median sample size 201 neonates [IQR: 341]), placental tissue (5 studies; median sample size 236 neonates [IQR: 38]), and/or buccal cells (2 studies; 236 and 53 neonates). The neonatal tissues were sampled within 72 h after birth in all studies, except by Cardenas et al. (2019), who also collected blood from children aged 3–5 y and 7–11 y [Citation29]. All studies adjusted for potential covariates and/or confounders in their statistical analyses or by design, but the number of variables under consideration differed greatly (Supplementary Table S3). The covariates most frequently accounted for were maternal age (n = 16), smoking during pregnancy (n = 13), infant sex (n = 12), gestational age (n = 10), and folate use in pregnancy (n = 10).
In addition to the epigenetic outcomes, several studies reported phenotypic outcomes in children, specifically poor foetal growth (n = 1) [Citation33], birth weight (n = 2) [Citation31,Citation34], severity of neonatal abstinence syndrome (n = 1) [Citation34], ADHD (n = 1) [Citation35], stress reactivity (n = 2) [Citation36,Citation37], and soothability (n = 1) [Citation38] (Supplementary Table S4). One study performed a mediation analysis of medication exposure, epigenetic modification, and neonatal phenotypic outcome [Citation37]. This study assessed whether the DNAm of a CpG in the placental NR3C2 gene acted as a mediator of the effect of maternal depressive symptoms on cortisol reactivity in 12-month-old infants [Citation37]. The effects of maternal depression on cortisol levels were decomposed into direct effects and DNAm-mediated indirect effects, finding that, although the indirect effect of DNAm was positive, it did not overcome the larger negative direct effect of depressive symptoms on infant cortisol levels [Citation37]. However, the analysis demonstrated an increased DNAm at the NR3C2 CpG upon in utero antidepressant exposure, suggesting that maternal antidepressant use during pregnancy enhances the indirect effect of NR3C2 DNAm on the infant stress response [Citation37].
All EWASs used the Illumina platform [Citation39] to assess DNAm with the MethylationEPIC (n = 2), HumanMethylation 450 (n = 3), or HumanMethylation 27 (n = 4) bead chips. To assess the association between CpG DNAm and medication exposure, the majority of the EWASs used linear regression models (n = 6). In most of the EWASs, a result was considered significant if the false discovery rate (FDR) adjusted p-value was <0.05 (n = 8), except for one study that used an FDR adjusted p < 0.1 [Citation32].
In the candidate gene studies, several methods were used to investigate DNAm: the Illumina platform (n = 1) [Citation33], the SEQUENOM MassARRAY EpiTYPER platform (n = 3), and the PyroMark system (n = 7). The studies reported the methylation percentages (n = 6), mean methylation percentages of triplicates (n = 4) or the β value from the Illumina microarray (n = 1) [Citation33]. Various statistical tests were applied to assess differential DNAm. For these tests, three studies used FDR adjusted p-values (p < 0.25 in one study; p < 0.05 in two studies), two studies used the Bonferroni-corrected p-value, and the last six studies applied an unadjusted p < 0.05. Further details on the studies are available in . For excellent discussions of statistical approaches in epigenetic studies, we recommend the recently published reviews by Teschendorff and Relton [Citation40], van Rooij et al. [Citation41], and Mansell et al. [Citation42].
Table 1. Overview of the studies included in the literature review
Table 2. Overview of the methodology and statistical analysis
Results of prenatal medication exposure and neonatal DNA methylation
The most examined medication group in prenatal pharmacoepigenetics was antidepressants, investigated in 12 studies of 3,320 neonates (2 EWASs, 8 candidate gene studies, and 2 studies combining an epigenome-wide and a candidate gene approach). In the most recent EWAS, Cardenas et al. [Citation29] discovered 130 differentially methylated CpGs in cord blood samples collected from neonates exposed to antidepressants in utero. One of these sites that mapped to ZNF575 was replicated in an independent cohort [Citation29]. Schroeder et al. [Citation43] found that the exposed neonates had two differentially methylated CpGs in TNFRSF21 and CHRNA4. However, the authors disregarded these findings as false positives considering the small effect sizes (DNAm changes of 1–3%) [Citation43]. In the EWAS conducted by Gurnot et al. [Citation31], three CpGs were differentially methylated in neonates prenatally exposed to serotonin reuptake inhibitors (SRIs; CYP2E1, EVA1, and SLMAP). However, in the EWAS by Non et al. [Citation32], no CpGs were significantly different in neonates exposed to selective serotonin reuptake inhibitors (SSRIs) in utero.
The candidate gene studies investigated CpGs in a total of 32 different genes (Supplementary Table S6). Most of the included genes were chosen based on their suggested association with psychiatric disorders (e.g., the serotonin transporter gene SLC6A4) [Citation32,Citation38,Citation44], stress reactivity (e.g., the glucocorticoid and mineralocorticoid receptor genes NR3C1 and NR3C2) [Citation32,Citation36,Citation37,Citation45], or adverse early life events (e.g., the brain-derived neurotrophic factor gene BDNF) [Citation32,Citation44]. In the studies combining epigenome-wide and candidate gene approaches [Citation31,Citation32], the candidate gene investigation was used to verify the epigenome-wide results. However, except for the verification of CYP2E1 DNAm by Gurnot et al. [Citation31], neither of the significant genes in either of the candidate gene studies were also significant in the EWASs of antidepressants.
Four genes involved in neurotransmitter receptor or transporter activity (NR3C1, SLC6A4, and FKBP5) or neuronal differentiation (BDNF) were investigated across several studies (). The DNAm of neither NR3C1 nor BDNF was associated with prenatal exposure to antidepressants in any of the studies investigating these genes [Citation32,Citation36,Citation37,Citation45]. For SLC6A4, the results were contradictory. Although Gartstein et al. [Citation38] found an increase in DNAm at six CpGs in cord blood upon prenatal SSRI exposure, Non et al. [Citation32] reported a decrease in DNAm at one CpG in cord blood upon prenatal SSRI exposure when examined by pyrosequencing but not in the epigenome-wide approach. Finally, Devlin et al. [Citation44] found no association between in utero exposure to SSRIs or serotonin and noradrenaline reuptake inhibitors (SNRIs), and DNAm of SLC6A4 in cord blood. A CpG in FKBP5, which encodes a co-regulator of the glucocorticoid receptor, was negatively associated with in utero SSRI exposure in cord blood [Citation32], but not in the placenta [Citation33]. In summary, the results of studies on prenatal antidepressant exposure and DNAm are largely inconsistent.
Table 3. Overview of genes examined in more than one candidate gene study on antidepressants
Prenatal AED exposure was investigated in two EWASs [Citation46,Citation47], which reported discrepant results. Emes et al. [Citation46] found no global DNAm differences in the cord blood of neonates exposed to AEDs in utero, whereas Smith et al. [Citation47] observed decreased global DNAm in the cord blood of neonates prenatally exposed to AEDs and no global DNAm differences in placental tissue. Furthermore, Emes et al. [Citation46] reported differential DNAm at 662 CpGs when comparing cord blood from neonates that were exposed and not exposed to AEDs in utero, whereas Smith et al. [Citation47] found 14 CpGs with significantly reduced DNAm in the same tissue, including three CpGs that were also significant in placentae.
Two EWASs examined the association between in utero paracetamol exposure and DNAm in placentae [Citation48] or cord blood [Citation35]. Addo et al. [Citation48] reported 24 differentially methylated CpGs in placental tissue when comparing exposed and unexposed pregnancies. Using a different study design, Gervin et al. [Citation35] compared DNAm in long-term paracetamol-exposed children with ADHD to short-term-exposed children with ADHD (2,089 differentially methylated CpGs), unexposed children with ADHD (192 differentially methylated CpGs), and unexposed children without ADHD (6,211 differentially methylated CpGs). Although the studies report vastly different numbers of significant CpGs, both Gervin et al. [Citation35] and Addo et al. [Citation48] concluded that prenatal paracetamol exposure may be associated with DNAm in cord blood from susceptible individuals or placentae, respectively.
Yeung et al. [Citation30] investigated the association between prenatal acetylsalicylic acid exposure and DNAm in cord blood. In the randomized controlled trial, women were randomly assigned to receive 81 mg of acetylsalicylic acid or placebo every day until conception (within six menstrual cycles) and during pregnancy [Citation49]. The DNAm of one CpG (3,500 base pairs upstream of the POU4F1 promoter) in cord blood was significantly associated with prenatal exposure to acetylsalicylic acid [Citation30]. However, Yeung et al. concluded that the association of prenatal acetylsalicylic acid exposure with DNAm in cord blood is negligible, as only one CpG with a minor effect size (1% increase in CpG DNAm) was discovered in their association study [Citation30].
In the EWAS on prenatal methadone exposure, McLaughlin et al. [Citation34] reported a significant increase in buccal cell DNAm of ABCB1, CYP2D6, and OPRM1 in neonates of mothers who were methadone-maintained during pregnancy. The authors argued that their results demonstrated that opioids interact with epigenetic mechanisms, and that the altered DNAm of the opioid metabolism-related genes may have a functional significance that needs further investigation [Citation34].
Discussion
In this review, we have systematically summarized the literature investigating associations between prenatal medication exposure and epigenetic differences in neonates. We included a total of 18 studies on DNAm, examining in utero exposure to antidepressants, AEDs, paracetamol, acetylsalicylic acid, or methadone. We found substantial inconsistency across studies, including heterogeneity in methodology, materials, design, genome coverage, and statistical modelling, making the interpretation of findings and cross-study comparisons challenging. The novelty of the field combining epidemiological and pharmacoepigenetic methods may partly explain this heterogeneity due to a lack of consensus on how to perform analyses and report findings. Therefore, we discuss the results of the reviewed studies with respect to both epidemiological and epigenetic considerations, and suggest 10 recommendations for future studies in prenatal pharmacoepigenetics, as summarized in .
Box 1. (1)HYPOTHESIS: candidate gene studies should use a plausible hypothesis to guide the study design Hypotheses should be defined prior to designing a candidate gene study, and be guided by principles of teratology, knowledge of pharmacological mechanisms, and epidemiological and biological observations. Hypothesis-free EWASs are also important as the field of prenatal pharmacoepigenetic studies is still emerging.(2)MEDICATION SELECTION: investigate individual medications rather than medication classes Unless the pharmacological and epigenetic mechanisms of action of medications are expected to be similar across the medication class, medications should be analysed on an individual substance level.(3)STATISTICAL POWER: ensure sufficient sample sizes to detect relevant DNAm differences To detect biologically relevant DNAm associations and to ensure valid interpretation of the results, tools developed for power assessments in epigenetic studies should be used when planning such studies.(4)STUDY DESIGN: include a disease comparison group to disentangle medication from indicationStudies should include a disease comparison group to better differentiate the effects of exposure to medication from the underlying maternal disease. This may reduce the impact of confounding by indication.(5)SYSTEMATIC ERROR: assess selection bias, information bias, and confounding Selection bias should be assessed by comparing characteristics of study samples to the target population. The validity of medication exposure, neonatal phenotype, and other covariates should be reported, and information bias and misclassification addressed. Measured confounders of the exposure–outcome association(s) are to be adjusted for and residual confounding investigated. Importantly, cell type heterogeneity should be considered a confounding factor in epigenetic studies.(6)TISSUE SELECTION: biomarkers and extrapolation of DNAm patterns across tissues If the research aim is not only to report a tissue-independent biomarker, but to extrapolate results to other target tissues, the limitations of such translation should be recognized,, and reduced using software applications or data sets on cross-tissue correlations of modifications.(7)LONGITUDINAL PERSPECTIVE: assess persistence of DNAm patterns throughout childhoodThe follow-up of epigenetic patterns later in childhood is essential to assess the relevance of these changes over time, as they may suggest a long-term impact on the phenotypic outcome. (8)DATA INTEGRATION: integrate epigenetic data with complementary omics dataIntegration of complementary omics data, such as genomic and transcriptomic data, can strengthen functional and causal inferences of the findings. (9) CAUSAL INFERENCE: provides a framework for interpreting exposure-outcome associations Causal inference methods, such as two-step Mendelian randomization, may support the inference of causation from exposure–outcome associations, including how medication may impact phenotypic outcome via DNAm changes. Importantly, the underlying assumptions of causal methods are often untestable and, therefore, such methods should be used carefully.(10)REPLICATION: replicate findings using different methods and independent cohorts Replication both across methods and in independent cohorts is essential to increase the validity of the findings and the generalizability of the results to enhance clinical relevance..
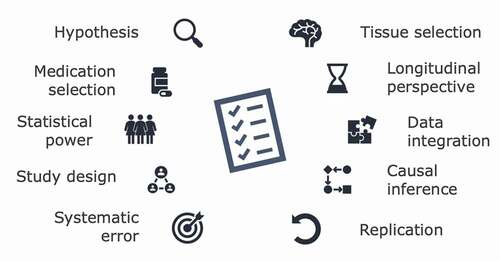
Prenatal pharmacoepigenetic candidate gene studies should have a clearly defined hypothesis guided by teratological principles [Citation50] and pharmacological, epidemiological, and biological knowledge (pt. 1, Box 1). Founding the research question on a well-informed hypothesis is fundamental for a transparent and well-designed prenatal pharmacoepigenetic study. This was mostly done in the candidate gene studies included in this review, which provided a rationale for selecting the genes being studied, such as the gene being related to psychiatric disorders (the serotonin transporter gene SLC6A4) [Citation32,Citation38,Citation44] or stress reactivity (the glucocorticoid and mineralocorticoid receptor genes NR3C1 and NR3C2) [Citation32,Citation36,Citation37,Citation45].
Small molecular and structural differences between drugs are known to cause variations in toxicity and teratogenicity [Citation50]. Although the reviewed studies on analgesics focused on one specific medication [Citation30,Citation34,Citation35,Citation48], the studies on psychotropics investigated the effect of medication classes on neonatal DNAm [Citation29,Citation31–33,Citation36–38,Citation43–47,Citation51,Citation52]. In the two studies on AEDs [Citation46,Citation47], several medications were investigated, which may be too broad considering the various different pharmacological [Citation53,Citation54] and epigenetic mechanisms of action of AEDs [Citation21,Citation55,Citation56]. For example, Smith et al. [Citation47] jointly analysed seven different AEDs among 53 women, but also performed a stratified analysis of carbamazepine monotherapy (36 women). In contrast, Emes et al. [Citation46] jointly analysed valproate, lamotrigine, and carbamazepine among nine women, and did not stratify their analyses on individual medications. Analyses on a medication class level may mask effects or give heterogeneous results that are difficult to interpret. Consequently, prenatal pharmacoepigenetic studies should aim to investigate individual medications rather than medication classes and ensure sufficient study power to do this (pt. 2 & pt. 3, Box 1).
The median sample size of the reviewed studies was 201 (IQR of 289), with sample sizes as low as 18 [Citation46] and 23 [Citation31]. None of the studies reported a power assessment to justify the selected sample size. The power of an EWAS depends on many variables, including the significance level, effect size, sample size, array technology, tissue type, and distribution of DNAm differences [Citation57]. Therefore, power calculations are challenging, but simulation studies for power estimation [Citation42,Citation58,Citation59], as well as power assessment tools [Citation42,Citation57], may support the investigation of power in epigenetic studies. In epigenetic epidemiology, the effect sizes are expected to be small, ranging from 0.05 to 0.1 and upwards [Citation60,Citation61], as evidenced in studies on prenatal smoking exposure, with effect sizes commonly ranging from 0.02 to 0.1 [Citation62,Citation63]. We recommend that future prenatal pharmacoepigenetic studies perform and report power assessments in order to ensure sufficient power to detect genuine epigenetic differences between comparison groups (pt. 3, Box 1).
The indication for medication use is an important potential confounder in prenatal pharmacoepigenetic studies, as the observed outcome may be associated with the underlying maternal illness and not the medication used to treat it [Citation64,Citation65]. Among the 14 studies on psychotropics in this review, only eight studies included such a comparison group [Citation29,Citation32,Citation33,Citation36,Citation37,Citation44,Citation51,Citation52]. Notably, seven of these studies found an association between the underlying maternal depression and DNAm in the neonate [Citation29,Citation32,Citation33,Citation36,Citation37,Citation44,Citation51], emphasizing the importance of including this comparison group in future prenatal pharmacoepigenetic studies (pt. 4, Box 1).
When defining medication-exposed comparison groups, more than half of the reviewed studies relied partly or entirely on self-reported medication use during pregnancy [Citation29,Citation35,Citation37,Citation38,Citation43,Citation45–48,Citation51]. This measure does not necessarily reflect the actual medication use [Citation66–68] and is vulnerable to recall bias if reported retrospectively [Citation69]. In five studies, medication exposure was assessed at birth using maternal and/or neonatal blood concentrations of the medication [Citation31,Citation34,Citation37,Citation47,Citation51]. Although informative at birth, this measure does not reflect medication use in earlier stages of pregnancy. Similarly, the eight studies investigating the association between DNAm and neonatal phenotypic outcomes included various outcome definitions without assessing the validity of the measurement [Citation31,Citation33–38,Citation46]. In five studies, data were measured objectively (birth weight [Citation31,Citation33,Citation46] and cortisol levels [Citation36,Citation37]), whereas two studies relied on diagnoses by specialists [Citation34,Citation35], and one study used parent reports on infant temperament [Citation38]. An assessment of medication exposure, neonatal phenotype, and covariates is crucial to avoid misclassification. Therefore, we recommend that future prenatal pharmacoepigenetic studies perform sensitivity analyses to assess the robustness of the findings, taking into account the validity of the measures (pt. 5, Box 1). For example, methods to quantify the impact of exposure and outcome misclassifications, such as probabilistic bias analysis [Citation70], are highly recommended [Citation64].
A wide range of different covariates were considered in the studies (Supplementary Table S3). Selecting an appropriate set of confounders to control for is critical to avoid systematic bias (pt. 5, Box 1). Ten studies selected confounders by assessing associations between covariates, exposure, and outcome [Citation31,Citation33,Citation34,Citation36–38,Citation45,Citation46,Citation51,Citation52]. We suggest to control for covariates that are assumed to be confounders, i.e., covariates that are not part of the causal path, and that are both a cause of the exposure and the outcome. Therefore, the covariates to be accounted for should occur upstream of the prenatal exposure, while mediators (which are part of the causal pathway) should not be accounted for when investigating the total effect of the exposure on the outcome. The specific covariates to be taken into account need to be assessed for each individual study, as the relevance of the covariates depends on several factors (e.g., study design and tissue type), and include both technical covariates related to laboratory procedures and biological covariates. Examples of biological covariates to be evaluated are maternal age, smoking during pregnancy, infant sex, gestational age, and folate use in pregnancy, which were the covariates most commonly accounted for in the studies included in this review (Supplementary Table S3). To this end, future prenatal pharmacoepigenetic studies may also benefit from implementing causal inference tools, such as directed acyclic graphs (DAGs) [Citation71], to identify a sufficient set of confounders for adjustments [Citation64]. Such investigations can be complemented by assessing whether the selected confounders largely capture the model variability, as was performed by surrogate variable analysis in Gervin et al. [Citation35], and by principal component analyses in Addo et al. [Citation48] and Cardenas et al. [Citation29]. For an excellent overview of a general approach to identify relevant confounders in observational studies, please refer to the review by VanderWeele [Citation72].
The majority of the reviewed studies were based on cord blood, which consists of cells exhibiting cell type-specific DNAm patterns [Citation40]. Therefore, prenatal pharmacoepigenetic studies should consider whether DNAm differences associated with medication exposure reflect variation in constituent cell types, which are known to mediate or confound the exposure associations [Citation40,Citation73]. When investigating cell-type proportions as a mediator, in order to assess the direct effect of medication exposure on associated DNAm differences, it may be necessary to adjust for estimated or measured cell-type composition (see, e.g., Liu et al. [Citation74] and Gervin et al. [Citation75]). However, if the total effect of medication exposure on DNAm is more interesting, for instance when searching for potential biomarkers of a phenotypic outcome, the cell-type composition should not be accounted for, as it may remove relevant DNAm–phenotypic outcome associations (see, e.g., Ollikainen et al. [Citation76]). Cell type composition may act as a confounder when assessing the extent to which DNAm mediate the effect of drug exposure on a phenotypic outcome, and should in such instances be accounted for [Citation73]. In summary, variation in cell-type composition confers an important covariate in epigenetic studies, and should be appropriately evaluated [Citation40,Citation60,Citation73,Citation77]. Surprisingly, only six of the reviewed studies considered cell-type composition in their analyses [Citation29,Citation30,Citation35,Citation45,Citation48,Citation52], emphasizing the need for increased awareness among prenatal pharmacoepigenetic researchers to evaluate cell types in future studies (pt. 5, Box 1). There are several different methods to determine and account for the cell-type composition in tissue samples, and these are extensively described in the excellent recent review by Teschendorff and Relton [Citation40].
Among the reviewed records, eight studies hypothesized an association between DNAm and neonate phenotypes [Citation31,Citation33–38,Citation46], including five studies concerning brain-related phenotypic outcomes [Citation34–38]. However, DNAm in peripheral surrogate tissues does not necessarily resemble DNAm in the target tissue [Citation78–80], which challenges the accuracy of the extrapolation of the findings. Although this limitation was acknowledged in most studies [Citation29–32,Citation35–38,Citation43–45,Citation47,Citation48,Citation51], only one study attempted to reduce the constraint by including a correlation analysis of select CpGs across adult whole blood and brain tissue [Citation29]. Importantly, investigation of peripheral tissues is still considered valuable, since biomarkers of maternal disease or child developmental outcomes do not need to be from the relevant tissue (i.e., do not need to be tissue-specific). However, to realize the ultimate aim of prenatal pharmacoepigenetics of gaining direct mechanistic insights into how medication exposure impacts the foetus with potential phenotypic consequences, future studies should validate tissue extrapolation by, for example, investigating cross-tissue correlations in available databases [Citation61,Citation81–85] (pt. 6, Box 1). Yet, current databases are mostly available on adult tissues, limiting the relevance to prenatal pharmacoepigenetic studies. Researchers have been calling for initiatives to develop biobanks of foetal and child brain specimens, while also taking into account the ethical issues of building such biobanks [Citation86].
Only one of the reviewed studies investigated DNAm patterns longitudinally during childhood, finding that DNAm at a CpG in ZNF575 persisted into early childhood [Citation29]. Though investigating the DNAm at birth provides information on the immediate impact of prenatal medication exposure, the follow-up of epigenetic patterns later in childhood is valuable to assess the persistence over time and increase the clinical relevance of the findings (pt. 7, Box 1).
The clinical relevance of prenatal pharmacoepigenetic research may also be strengthened by functional and causal interpretations of the results. Using a multi-omics approach with integration of omics data (e.g., genomics, epigenomics, and transcriptomics data) [Citation87] could substantiate the epigenetic findings (pt. 8, Box 1). However, the reviewed studies only used single omics data (i.e., epigenomics). Although single omics data are potentially useful both as biomarkers and in providing insight into biological pathways, this is limited to correlations or associations often reflecting reactive, rather than causative, processes. We recommend that future studies include additional omics data, as this may enable (i) investigation of the functional consequences of DNAm on gene expression [Citation40], (ii) adjustment for the genetic variation associated with DNAm variation [Citation88–90], and (iii) utilization of genomic methylation quantitative trait loci (mQTLs) to implement causal inference methods, such as two-step Mendelian randomization [Citation40,Citation91] (pt. 9, Box 1). There are several openly accessible resources making omics data available for integration, as thoroughly reviewed by Walton, Relton and Caramaschi [Citation92].
Causal modelling and reasoning are increasingly being applied in genetic epidemiology to strengthen the ability to make causal inferences about associations, but it is still new to the field of pharmacoepigenetics [Citation40,Citation64]. For example, two-step Mendelian randomization [Citation91,Citation93–95] has been used to assess how DNAm can mediate an association between prenatal exposure and phenotypic outcomes in children [Citation96,Citation97]. Notably, only one reviewed study attempted to make causal inferences about an association between prenatal antidepressant exposure, cord blood DNA, and infant stress reactivity in a mediation analysis [Citation37]. We foresee important advances in future prenatal pharmacoepigenetic studies using the causal inference framework (pt. 9, Box 1). Importantly, the causal models rely on assumptions that need to be met for them to be valid [Citation98]. As these assumptions are often untestable [Citation98], careful use of the causal inference framework in pharmacoepigenetic studies is essential.
Lastly, to validate findings, replication using a different technology and in an independent cohort is essential, both to determine the robustness of the associations and to assess the level of technical and biological variation. Notably, only two of the reviewed studies applied more than one method to assess DNAm [Citation31,Citation32], and only one study attempted to validate their results in an independent cohort [Citation29], emphasizing the need for an increased focus on replication in prenatal pharmacoepigenetics (pt. 10, Box 1). Several multi-cohort consortia to enable replication of studies are already in place, such as the Pregnancy and Childhood Epigenetics consortium (PACE) [Citation99].
Conclusion
Investigating the potential effects of pharmacological treatment in pregnancy is essential to establish foetal epigenetic safety, understand the underlying mechanisms, and recognize the clinical consequences for the offspring. However, studies on prenatal medication exposure and epigenetic changes are largely heterogeneous and inconsistent. To improve the quality, comparability, and interpretability of future prenatal pharmacoepigenetic studies, we propose 10 recommendations bridging the fields of prenatal epidemiology and epigenetics. Epidemiological approaches and causal inference frameworks will reduce systematic bias and improve our ability to interpret exposure–outcome associations, including how medications may impact phenotypic outcomes via changes in DNAm. Furthermore, it is essential to consider the persistence of DNAm patterns over time and the potential for cross-tissue extrapolation when assessing the biological relevance of the epigenetic contribution. Importantly, integrating more omics data and implementing two-step Mendelian randomization can strengthen the functional and causal inferences of the findings. In conclusion, a consensus on how to perform and report prenatal pharmacoepigenetic studies will fuel the development of the field and contribute to future high-quality studies of clinical relevance.
List of Abbreviations
ADHD | = | Attention-deficit/hyperactivity disorder |
AED | = | Antiepileptic drug |
ATC | = | Anatomical Therapeutic Chemical |
CpG | = | 5ʹ–Cytosine–phosphate–guanine–3ʹ site |
DAG | = | Directed acyclic graph |
DOHaD | = | Developmental Origins of Health and Disease |
DNAm | = | DNA methylation |
EWAS | = | Epigenome-wide association study |
FDR | = | False discovery rate |
IQR | = | Interquartile range |
mQTL | = | Methylation quantitative trait locus |
NSAID | = | Non-steroidal anti-inflammatory drug |
SNRI | = | Serotonin and noradrenaline reuptake inhibitor |
SRI | = | Serotonin reuptake inhibitor |
SSRI | = | Selective serotonin reuptake inhibito |
Author’s contribution
EWO, HMEN, and KG conceived the idea of the systematic literature review. EWO, HMEN, and KG planned the searches. EWO performed the searches. EWO and KG performed the screening and selection of records. EWO extracted the data and drafted the first version of the paper. EWO, HMEN, and KG all revised the paper. All authors read and approved the final manuscript.
Ethics approval and consent to participate
Not applicable.
Supplemental Material
Download Zip (360.7 KB)Acknowledgments
We thank Christian Page and Mollie Wood for their critical appraisal of the article. We also thank the librarians at the Library of Medicine and Science of the University of Oslo, particularly Bente Kathrine Rasch and Hilde Strømme, for excellent support in developing the search strategy for the review.
Disclosure statement
No potential conflict of interest was reported by the authors.
Supplementary material
Supplemental data for this article can be accessed here.
Additional information
Funding
References
- Griffiths SK, Campbell JP. Placental structure, function and drug transfer. Contin Educ Anaesthesia Crit Care Pain. 2015;15(2):84–89.
- Tetro N, Moushaev S, Rubinchik-Stern M, et al. The placental barrier: the gate and the fate in drug distribution. Pharm Res. 2018;35(4):1–16.
- Goasdoué K, Miller SM, Colditz PB, et al. Review: the blood-brain barrier; protecting the developing fetal brain. Placenta. 2017;54:111–116.
- Ek CJ, Dziegielewska KM, Habgood MD, et al. Barriers in the developing brain and Neurotoxicology. Neurotoxicology. 2012;33:586–604.
- Barker DJP. The origins of the developmental origins theory. J Intern Med. 2007;261(5):412–417.
- Gillman MW. Developmental origins of health and disease. N Engl J Med. 2005;353(17):1848–1850.
- Gluckman PD, Hanson MA, Pinal C. The developmental origins of adult disease. Matern Child Nutr. 2005;1(3):130–141.
- Godfrey K. The ‘developmental origins’ hypothesis: epidemiology. In: Gluckman PD, Hanson MA, editors. Dev Orig Heal Dis. Cambridge: Cambridge University Press; 2006. p. 6–32.
- Godfrey KM, Lillycrop KA, Burdge GC, et al. Epigenetic mechanisms and the mismatch concept of the developmental origins of health and disease. Pediatr Res. 2007;61(5 Part 2):5–10.
- Hjorth S, Bromley R, Ystrom E, et al. Use and validity of child neurodevelopment outcome measures in studies on prenatal exposure to psychotropic and analgesic medications – A systematic review. PLoS One. 2019;14(7):e0219778.
- Bromley R, Weston J, Adab N, et al. Treatment for epilepsy in pregnancy: neurodevelopmental outcomes in the child. Cochrane Database Syst Rev J. 2014.
- Weston J, Bromley R, Jackson CF, et al. Monotherapy treatment of epilepsy in pregnancy: congenital malformation outcomes in the child. Cochrane Database Syst Rev. 2016;11(11).
- El Marroun H, White T, Verhulst FC, et al. Maternal use of antidepressant or anxiolytic medication during pregnancy and childhood neurodevelopmental outcomes: a systematic review. Eur Child Adolesc Psychiatry. 2014;23(10):973–992.
- Prady SL, Hanlon I, Fraser LK, et al. A systematic review of maternal antidepressant use in pregnancy and short- and long-term offspring’s outcomes. Arch Womens Ment Health. 2018;21(2):127–140.
- Tosato S, Albert U, Tomassi S, et al. A systematized review of atypical antipsychotics in pregnant women: balancing between risks of untreated illness and risks of drug-related adverse effects. J Clin Psychiatry. 2017;78(5):e477–89. Physicians Postgraduate Press Inc.
- Bauer AZ, Kriebel D, Herbert MR, et al. Prenatal paracetamol exposure and child neurodevelopment: a review. Horm Behav. Academic Press Inc. 2018;101:125–147. .
- Yazdy M, Desai R, Brogly S. Prescription opioids in pregnancy and birth outcomes: a review of the literature. J Pediatr Genet. 2015;04(2):056–70. Georg Thieme Verlag KG.
- Ornoy A, Weinstein-Fudim L, Ergaz Z. Antidepressants, antipsychotics, and mood stabilizers in pregnancy: what do we know and how should we treat pregnant women with depression. Birth Defects Res. 2017;109(12):933–956. John Wiley and Sons Inc.
- Schaefer C, Peters PWJ, Miller RK, editors. Drugs during pregnancy and lactation: treatment options and risk assessment. 3rd ed. Academic Press, London, UK; 2014.
- Barouki R, Melén E, Herceg Z, et al. Epigenetics as a mechanism linking developmental exposures to long-term toxicity. Environ Int. 2018;114:77–86.
- Lötsch J, Schneider G, Reker D, et al. Common non-epigenetic drugs as epigenetic modulators. Trends Mol Med. 2013;19(12):742–753.
- Viuff ACF, Pedersen LH, Kyng K, et al. Antidepressant medication during pregnancy and epigenetic changes in umbilical cord blood: a systematic review. Clin Epigenetics. 2016;8(1). DOI:https://doi.org/10.1186/s13148-016-0262-x
- Moher D, Liberati A, Tetzlaff J, et al. Preferred reporting items for systematic reviews and meta-analyses: the PRISMA statement. PLoS Med. 2009;6(7):e1000097.
- Centre for Reviews and Dissemination University of York; National Institute for Health Research. PROSPERO [Internet].
- Page MJ, Shamseer L, Tricco AC. Registration of systematic reviews in PROSPERO: 30,000 records and counting. Syst Rev. 2018;7(1):32.
- Counsell C. Formulating questions and locating primary studies for inclusion in systematic reviews. Ann Intern Med. 1997;127(5):380–387.
- WHO Collaborating Centre for Drug Statistics Methodology. ATC/DDD Index 2020 [Internet].
- Ouzzani M, Hammady H, Fedorowicz Z, et al. Rayyan – a web and mobile app for systematic reviews. Syst Rev. 2016;5(1). DOI:https://doi.org/10.1186/s13643-016-0384-4
- Cardenas A, Faleschini S, Cortes Hidalgo A, et al. Prenatal maternal antidepressants, anxiety, and depression and offspring DNA methylation: epigenome-wide associations at birth and persistence into early childhood. Clin Epigenetics. 2019;11(1). DOI:https://doi.org/10.1186/s13148-019-0653-x.
- Yeung EH, Guan W, Zeng X, et al. Cord blood DNA methylation reflects cord blood C-reactive protein levels but not maternal levels: a longitudinal study and meta-analysis. Clin Epigenetics. 2020;12(1). DOI:https://doi.org/10.1186/s13148-020-00852-2.
- Gurnot C, Martin-Subero I, Mah SM, et al. Prenatal antidepressant exposure associated with CYP2E1 DNA methylation change in neonates. Epigenetics. 2015;10(5):361–372.
- Non AL, Binder AM, Kubzansky LD, et al. Genome-wide DNA methylation in neonates exposed to maternal depression, anxiety, or SSRI medication during pregnancy. Epigenetics. 2014;9(7):964–972.
- Ciesielski TH, Marsit CJ, Williams SM. Maternal psychiatric disease and epigenetic evidence suggest a common biology for poor fetal growth. BMC Pregnancy Childbirth. 2015;15(1). DOI:https://doi.org/10.1186/s12884-015-0627-8
- McLaughlin P, Mactier H, Gillis C, et al. Increased DNA methylation of ABCB1, CYP2D6, and OPRM1 genes in newborn infants of methadone-maintained opioid-dependent mothers. J Pediatr. 2017;190:180–184.
- Gervin K, Nordeng H, Ystrom E, et al. Long-term prenatal exposure to paracetamol is associated with DNA methylation differences in children diagnosed with ADHD. Clin Epigenetics. 2017;9(1). DOI:https://doi.org/10.1186/s13148-017-0376-9
- Oberlander TF, Weinberg J, Papsdorf M, et al. Prenatal exposure to maternal depression, neonatal methylation of human glucocorticoid receptor gene (NR3C1) and infant cortisol stress responses. Epigenetics. 2008;3(2):97–106.
- Galbally M, Watson SJ, van Ijzendoorn M, et al. The role of glucocorticoid and mineralocorticoid receptor DNA methylation in antenatal depression and infant stress regulation. Psychoneuroendocrinology. 2020;115:104611.
- Gartstein MA, Hookenson KV, Brain U, et al. Sculpting infant soothability: the role of prenatal SSRI antidepressant exposure and neonatal SLC6A4 methylation status. Dev Psychobiol. 2016;58(6):745–758.
- Weisenberger DJ, van den Berg D, Pan F, et al. Comprehensive DNA Methylation Analysis on the Illumina® Infinium® Assay Platform. Appl Note, Illumina.
- Teschendorff AE, Relton CL. Statistical and integrative system-level analysis of DNA methylation data. Nat Rev Genet. 2018;19:129–147.
- van Rooij J, Mandaviya PR, Claringbould A, et al. Evaluation of commonly used analysis strategies for epigenome- and transcriptome-wide association studies through replication of large-scale population studies. Genome Biol. 2019;20(1):235. BioMed Central Ltd.
- Mansell G, Gorrie-Stone TJ, Bao Y, et al. Guidance for DNA methylation studies: statistical insights from the Illumina EPIC array. BMC Genomics. 2019;20(1):366. BioMed Central Ltd.
- Schroeder JW, Smith AK, Brennan PA, et al. DNA methylation in neonates born to women receiving psychiatric care. Epigenetics. 2012;7(4):409–414.
- Devlin AM, Brain U, Austin J, et al. Prenatal exposure to maternal depressed mood and the MTHFR C677T variant affect SLC6A4 methylation in infants at birth. PLoS One. 2010;5(8):e12201.
- Mansell T, Vuillermin P, Ponsonby AL, et al. Maternal mental well-being during pregnancy and glucocorticoid receptor gene promoter methylation in the neonate. Dev Psychopathol. 2016;28(4pt2):1421–1430.
- Emes RD, Clifford H, Haworth KE, et al. Antiepileptic drugs and the fetal epigenome. Epilepsia. 2013;54(1):e16–9.
- Smith AK, Conneely KN, Newport DJ, et al. Prenatal antiepileptic exposure associates with neonatal DNA methylation differences. Epigenetics. 2012;7:458–463.
- Addo KA, Bulka C, Dhingra R, et al. Acetaminophen use during pregnancy and DNA methylation in the placenta of the extremely low gestational age newborn (ELGAN) cohort. Environ Epigenetics. 2019;5(2). DOI:https://doi.org/10.1093/eep/dvz010.
- Schisterman EF, Silver RM, Lesher LL, et al. Preconception low-dose aspirin and pregnancy outcomes: results from the EAGeR randomised trial. Lancet. 2014;384(9937):29–36.
- Friedman JM. The principles of teratology: are they still true? Birth Defects Res A Clin Mol Teratol. 2010;88(10):766–768.
- Galbally M, Ryan J, van Ijzendoorn M, et al. Maternal depression, antidepressant use and placental oxytocin receptor DNA methylation: findings from the MPEWS study. Psychoneuroendocrinology. 2018;90:1–8.
- Soubry A, Murphy S, Huang Z, et al. The effects of depression and use of antidepressive medicines during pregnancy on the methylation status of the IGF2 imprinted control regions in the offspring. Clin Epigenetics. 2011;3(1):2.
- Cook AM, Bensalem-Owen MK. Mechanisms of action of antiepileptic drugs. Therapy. 2011;8(3):307–313.
- White HS, Smith MD, Wilcox KS. Mechanisms of action of antiepileptic drugs. Int Rev Neurobiol. 2007;81:85–110.
- Monti B, Polazzi E, Contestabile A. Biochemical, molecular and epigenetic mechanisms of valproic acid neuroprotection. Curr Mol Pharmacol. 2010;2(1):95–109.
- Göttlicher M, Minucci S, Zhu P, et al. Valproic acid defines a novel class of HDAC inhibitors inducing differentiation of transformed cells. Embo J. 2001;20(24):6969–6978.
- Graw S, Henn R, Thompson JA, et al. PwrEWAS: a user-friendly tool for comprehensive power estimation for epigenome wide association studies (EWAS). BMC Bioinformatics. 2019;20(1). DOI:https://doi.org/10.1186/s12859-019-2804-7
- Tsai PC, Bell JT. Power and sample size estimation for epigenome-wide association scans to detect differential DNA methylation. Int J Epidemiol. 2015;44(4):1429–1441.
- Saffari A, Silver MJ, Zavattari P, et al. Estimation of a significance threshold for epigenome-wide association studies. Genet Epidemiol. 2018;42(1):20–33. Wiley-Liss Inc.
- Heijmans BT, Commentary: MJ. The seven plagues of epigenetic epidemiology. Int J Epidemiol. 2012;41(1):74–78.
- Mill J, Heijmans BT. From promises to practical strategies in epigenetic epidemiology. Nat Rev Genet. 2013;14(8):585–594.
- Breton CV, Marsit CJ, Faustman E, et al. Small-magnitude effect sizes in epigenetic end points are important in children’s environmental health studies: the children’s environmental health and disease prevention research center’s epigenetics working group. Environ Health Perspect. 2017;125(4):511–526. Public Health Services, US Dept of Health and Human Services.
- Joubert BR, Felix JF, Yousefi P, et al. DNA methylation in newborns and maternal smoking in pregnancy: genome-wide consortium meta-analysis. Am J Hum Genet. 2016;98(4):680–696. Cell Press.
- Wood ME, Lapane KL, van Gelder MMHJ, et al. Making fair comparisons in pregnancy medication safety studies: an overview of advanced methods for confounding control. Pharmacoepidemiol Drug Saf. 2018;27(2):140–147.
- Medicines Agency E The European Network of Centres for Pharmacoepidemiology and Pharmacovigilance (ENCePP) Guide on Methodological Standards in Pharmacoepidemiology [Internet].
- Skurtveit S, Selmer R, Odsbu I, et al. Self-reported data on medicine use in the Norwegian mother and child cohort study compared to data from the Norwegian prescription database. Nor Epidemiol. 2014;24:209–216.
- Joseph RM, van Staa TP, Lunt M, et al. Exposure measurement error when assessing current glucocorticoid use using UK primary care electronic prescription data. Pharmacoepidemiol Drug Saf. 2019;28(2):179–186.
- Gnjidic D, Du W, Pearson SA, et al. Ascertainment of self-reported prescription medication use compared with pharmaceutical claims data. Public Heal Res Pract. 2017;27.
- Rothman KJ, Greenland S, Lash TL. Chapter 9: validity in Epidemiologic Studies. In: Mod Epidemiol. 3rd ed. Philadelphia, PA, USA: Lippincott Williams & Wilkins; 2008. p. 128–147.
- Lash TL, Fox MP, Maclehose RF, et al. Good practices for quantitative bias analysis. Int J Epidemiol. 2014;43(6):1969–1985. Oxford University Press.
- Textor J, Hardt J, Knüppel, S. DAGitty, Epidemiology: 2011;22(5):745). DOI: https://doi.org/10.1097/EDE.0b013e318225c2be
- VanderWeele TJ. Principles of confounder selection. Eur J Epidemiol. 2019;34(3):211–219. Springer Netherlands.
- Houseman EA, Kim S, Kelsey KT, et al. DNA methylation in whole blood: uses and challenges. Curr Environ Heal Reports. Springer. 2015;2(2):145–154.
- Liu Y, Aryee MJ, Padyukov L, et al. Epigenome-wide association data implicate DNA methylation as an intermediary of genetic risk in rheumatoid arthritis. Nat Biotechnol. 2013;31(2):142–147. Nature Publishing Group.
- Gervin K, Andreassen BK, Hjorthaug HS, et al. Intra-individual changes in DNA methylation not mediated by cell-type composition are correlated with aging during childhood. Clin Epigenetics. 2016;8(1):110. Springer Verlag.
- Ollikainen M, Ismail K, Gervin K, et al. Genome-wide blood DNA methylation alterations at regulatory elements and heterochromatic regions in monozygotic twins discordant for obesity and liver fat. Clin Epigenetics. 2015;7(1). DOI:https://doi.org/10.1186/s13148-015-0073-5.
- Jones MJ, Moore SR, Kobor MS. Principles and challenges of applying epigenetic epidemiology to psychology. Annu Rev Psychol. 2018;69(1):459–485.
- Liu H, Liu X, Zhang S, et al. Systematic identification and annotation of human methylation marks based on bisulfite sequencing methylomes reveals distinct roles of cell type-specific hypomethylation in the regulation of cell identity genes. Nucleic Acids Res. 2016;44(1):75–94.
- Lister R, Pelizzola M, Dowen RH, et al. Human DNA methylomes at base resolution show widespread epigenomic differences. Nature. 2009;462(7271):315–322.
- Gu J, Stevens M, Xing X, et al. Mapping of variable DNA methylation across multiple cell types defines a dynamic regulatory landscape of the human genome. G3 Genes, Genomes, Genet. 2016;6:973–986.
- Ollikainen M, Smith KR, Joo EJ-H, et al. DNA methylation analysis of multiple tissues from newborn twins reveals both genetic and intrauterine components to variation in the human neonatal epigenome. Hum Mol Genet. 2010;19(21):4176–4188.
- de Carli MM, Baccarelli AA, Trevisi L, et al. Epigenome-wide cross-tissue predictive modeling and comparison of cord blood and placental methylation in a birth cohort. Epigenomics. 2017;9(3):231–240.
- Ma B, Allard C, Bouchard L, et al. Locus-specific DNA methylation prediction in cord blood and placenta. Epigenetics. 2019;14(4):405–420.
- Herzog EM, Eggink AJ, Willemsen SP, et al. The tissue-specific aspect of genome-wide DNA methylation in newborn and placental tissues: implications for epigenetic epidemiologic studies. J Dev Orig Health Dis. 2020;12(1):113–123.
- Relton CL, Gaunt T, McArdle W, et al. Data resource profile: accessible resource for integrated epigenomic studies (ARIES). Int J Epidemiol. 2015;44(4):1181–1190.
- Abbott A. Tissue-bank shortage: brain child. Nature. 2011;478(7370):442–443. Nature.
- Conesa A, Beck S. Making multi-omics data accessible to researchers. Sci Data. 2019;6(1):251.
- van Dongen J, Nivard MG, Willemsen G, et al. Genetic and environmental influences interact with age and sex in shaping the human methylome. Nat Commun. 2016;7(1):1–13.
- Gaunt TR, Shihab HA, Hemani G, et al. Systematic identification of genetic influences on methylation across the human life course. Genome Biol. 2016;17(1). DOI:https://doi.org/10.1186/s13059-016-0926-z.
- Banovich NE, Lan X, McVicker G, et al. Methylation QTLs are associated with coordinated changes in transcription factor binding, histone modifications, and gene expression levels. PLoS Genet. 2014;10(9):e1004663. DOI: https://doi.org/10.1371/journal.pgen.1004663
- Relton CL, Davey Smith G. Two-step epigenetic Mendelian randomization: a strategy for establishing the causal role of epigenetic processes in pathways to disease. Int J Epidemiol. 2012;41(1):161–176.
- Walton E, Relton CL, Caramaschi D. Using openly accessible resources to strengthen causal inference in epigenetic epidemiology of neurodevelopment and mental health. Genes (Basel). 2019;10(3):193. MDPI AG.
- Kirkbride JB, Susser E, Kundakovic M, et al. Prenatal nutrition, epigenetics and schizophrenia risk: can we test causal effects? Epigenomics. 2012;4(3):303–315.
- Pingault JB, O’Reilly PF, Schoeler T, et al. Using genetic data to strengthen causal inference in observational research. Nat Rev Genet. 2018;19(9):566–580.
- Relton CL, Smith GD. Mendelian randomization: applications and limitations in epigenetic studies. Epigenomics. 2015;7(8):1239–1243.
- Caramaschi D, Sharp GC, Nohr EA, et al. Exploring a causal role of DNA methylation in the relationship between maternal vitamin B12 during pregnancy and child’s IQ at age 8, cognitive performance and educational attainment: a two-step Mendelian randomization study. Hum Mol Genet. 2017;26(15):3001–3013.
- Allard C, Desgagné V, Patenaude J, et al. Mendelian randomization supports causality between maternal hyperglycemia and epigenetic regulation of leptin gene in newborns. Epigenetics. 2015;10(4):342–351.
- Pearl J. An introduction to causal inference. Int J Biostat. 2010;6(2). DOI:https://doi.org/10.2202/1557-4679.1203
- Felix JF, Joubert BR, Baccarelli AA, et al. Cohort profile: pregnancy and childhood epigenetics (PACE) consortium. Int J Epidemiol. 2018;47(1):22–23.
- Addo KA, Bulka C, Dhingra R, et al. Acetaminophen use during pregnancy and DNA methylation in the placenta of the extremely low gestational age newborn (ELGAN) cohort [corrected]. Environ Epigenetics. 2020;6(1). DOI:https://doi.org/10.1093/eep/dvaa006.
- Gervin K, Salas LA, Bakulski KM, et al. Systematic evaluation and validation of reference and library selection methods for deconvolution of cord blood DNA methylation data. Clin Epigenetics. 2019;11(1). DOI:https://doi.org/10.1186/s13148-019-0717-y.
- Houseman EA, Accomando WP, Koestler DC, et al. DNA methylation arrays as surrogate measures of cell mixture distribution. BMC Bioinformatics. 2012;13(1). https://doi.org/10.1186/1471-2105-13-86.
- Gervin K, Page CM, Aass HCD, et al. Cell type specific DNA methylation in cord blood: a 450K-reference data set and cell count-based validation of estimated cell type composition. Epigenetics. 2016;11(9):690–698.
- Hayes AF. Beyond Baron and Kenny: statistical mediation analysis in the New Millennium. Commun Monogr. 2009;76(4):408–420.
- Hoyo C, Murtha AP, Schildkraut JM, et al. Methylation variation at IGF2 differentially methylated regions and maternal folic acid use before and during pregnancy. Epigenetics. 2011;6(7):928–936.