ABSTRACT
Objectives:
Sysmex® XN series hematopoietic progenitor cell (XN-HPC) is a sensitive, fast, and economic analytical method for predicting the yields of peripheral blood stem cell enumeration and products, and does not require a sophisticated or expensive workflow. However, various studies have shown that the characteristics of its diagnostic performance were non-uniform.
Methods:
We performed a systematic inquiry using PubMed, Embase, and Cochrane Library, to comprehensively search for studies published before November 21, 2021. The pooled specificity (SPE), sensitivity (SEN), negative likelihood ratio (NLR), positive likelihood ratio (PLR), diagnostic odds ratio (DOR) and receiver operating characteristic (ROC) curves were summarized to appraise the diagnostic merit of XN-HPC. A forest plot was used to research the sensitivities and specificities of XN-HPC performance. Subgroup analysis was performed to investigate heterogeneity where of importance.
Results:
Our research included four studies that assessed the diagnostic performance of XN-HPC in hematopoietic progenitor cell collection. The pooled accuracy was 95.4% (95% CI, 94.3–96.3), SPE was 0.81 (95% CI, 0.71–0.88), SEN was 0.95 (95% CI, 0.75-0.99), NLR was 0.06 (95% CI, 0.01–0.37), PLR was 5.0 (95% CI, 3.0–8.5), DOR was 78 (95% CI, 9–707) and the summary of the area under the ROC was 0.90 (95% CI, 0.87–0.92). Forest plot of sensitivities and specificities from XN-HPC test accuracy studies indicated the existence of high heterogeneity. We deduced that the patients were the source of heterogeneity via subgroup analysis.
Conclusions:
XN-HPC is an excellent diagnostic marker for quantitative detection of peripheral blood hematopoietic progenitor cells.
Introduction
Peripheral blood stem cells (PBSCs) have gradually replaced bone marrow (BM) as the main source of hematopoietic stem cells for the treatment of various haematological malignancies [Citation1, Citation2]. An adequate dose of hematopoietic stem cells is essential to promote hematopoietic reconstitution, thereby improving the efficiency of transplantation, and reducing the need for supportive blood transfusion and risk of infection [Citation3, Citation4]. The window for the best collection time is narrow, which needs to be determined accurately by the clinician. This is the key for final successful transplantation [Citation5, Citation6].
According to the International Society of Hematotherapy and Graft Engineering (ISHAGE), current detection methods for circulating hematopoietic stem cells and the decision to start apheresis are based on the CD34+ cell count determined through flow cytometry [Citation7, Citation8]. Despite the high degree of standardization, this method is expensive, has a long turnaround-time, is laborious, and requires sophisticated equipment and skilled staff [Citation9, Citation10]. In contrast, the XN stem cell counting technique requires less than 200 μL of peripheral blood or apheresis products for four separate counts without the need for sample pre-treatment, cleaning, or manual gating [Citation11, Citation12]. Moreover, to evaluate the cell’s characteristics, the immature myeloid information (IMI) channels play an important role, by which the HPC is observed in a distinct area of the blast region [Citation11, Citation12]. The counts, which include absolute hematopoietic progenitor cells, and percentage of total leukocytes are reported within five minutes [Citation13]. Compared with previous HPC counts measured using the XE analyzer, that using the Sysmex® XN analyzer (Sysmex Corporation, Kobe, Japan, XN-HPC) showed a significant correlation with the CD34 + cell count via flow cytometry [Citation10, Citation14]. HPCs can be measured quickly, economically, and can be included in routine operation, using an automatic blood analyzer for blood cell count analysis with the lowest additional cost.
The Sysmex® XN series hematopoietic progenitor cell (XN-HPC) is a sensitive, fast, and economic analytical method for predicting the yields of peripheral blood stem cell enumeration and products, and does not require a sophisticated or expensive workflow. However, the diagnostic performance characteristics were non-uniform in various studies, even unilateral and contradictory. In this study, we systematically and comprehensively evaluated the diagnostic performance of the published literature with sufficient statistical methods.
Materials and methods
This analysis was based on the preferred reporting items for systematic review and meta-analysis of the diagnostic test accuracy studies the statement of the preferred report item (PRISMA-DTA). Prior to the commencement of the study, the research program was registered and approved by the international systematic review forward-looking register PROSPERO (Reference No. CRD42022323431).
Literature search strategy and eligibility criteria
Search items included controlled terms (MesH in PubMed and Emtree in Embase) and free text terms. The target condition of this review was ‘Cell, Hematopoietic Stem’ OR ‘Cells, Hematopoietic Stem’ OR ‘Hematopoietic Stem Cell’ OR ‘Stem Cell, Hematopoietic’ OR ‘Hematopoietic Progenitor Cells’ OR ‘Cell, Hematopoietic Progenitor’ OR ‘Cells, Hematopoietic Progenitor’ OR ‘Hematopoietic Progenitor Cell’ OR ‘Progenitor Cell, Hematopoietic’ OR ‘Stem Cells, Hematopoietic’ OR ‘Hematopoietic Colony-Forming Unit’ OR ‘Hematopoietic Colony-Forming Units’ OR ‘CD34+ stem cell’ OR ‘HPC’ OR ‘XN HPC’ AND ‘Sysmex’ OR ‘Sysmex XN’. Studies were selected if they met the following criteria: (1) Patients: availability of basic information, such as age and gender, and has undergone autologous and/or allogeneic transplantation; (2) Diagnostic tool: XN-HPC; (3) Outcomes: sufficient data for a two-by-two table; (4) Study design: irrespective of study design. Exclusion criteria were: (1) duplicate records; (2) types of manuscript: comments, letters, reviews, and conference abstracts; (3) diagnostic tools: other than XN-10, XN-20, XN-1000, or XN-9000; and (4) data insufficient for reconstruction.
Data extraction and quality assessment
The authors (LH and YX) independently extracted relevant information from each study, including the first author, country, year of publication, male ratio, sample size, number of allogeneic transplantation donors, average age of allogeneic transplantation donors, diseases of autotransplantation patients, number of patients, mean age of patients, CD34 counting guidelines, HPC model, sample type, mobilization scheme, and True Positive (TP), True Negative (TN), False Negative (FN), and False Positive (FP). The extracted information was checked by a third author (GZ). Both authors re-examined the differences to ensure the accuracy of the database. The quality of the included study was assessed using the revised Quality Assessment of Diagnostic Accuracy Studies (QUADAS-2) tool, to estimate the risk of bias and applicability.
Statistical analysis
We extracted a 2 × 2 table from each eligible study (i.e. TP, FP, FN, and TN). The pooled sensitivity (SEN), specificity (SPE), positive likelihood ratio (PLR), negative likelihood ratio (NLR), diagnostic odds ratio (DOR), and corresponding 95% confidence interval (CI) were analysed using the bivariate random effects regression model. The summary receiver operating characteristic curve (SROC) was constructed to assess the existence of threshold effect, and the area under curve (AUC) was measured to evaluate the diagnostic accuracy of the test. An excellent diagnostic evaluation had an affecting value up to 1.0, whereas an AUC ≤ 0.5 indicated poor analysis quality. We used the I-square (I²) test to study the heterogeneities. P-values for pooled results were two-sided, and a P-value of <0.05 was considered statistically significant. Statistical analyses were performed using the Revman 5.3 and STATA software (version 13.1; Stata Corporation, College Station, TX, U.S.A.).
Results
Study selection and study characteristics
A total of 117 articles were explored, including 82 citations from PubMed, 35 from Embase, and 0 from Cochrane Library. shows the protocol of study inclusion and selection. A total of 17 duplicate records were eliminated. According to the inclusion and exclusion protocol, we excluded 83 studies by evaluating their titles and abstracts. The rest of the studies were further reviewed by reading the full text. Finally, this meta-analysis included only three studies, one of which included two independent types of institutional studies, which resulted in a total of four studies. summarizes the characteristics of these studies. Only one study from Francesco et al. (2019) did not mention the age and sex of the recorded population, resulting in a failure to conduct the subgroup analysis of age or sex. In addition, except for the study of Francesco et al. (2019), no other included studies have proposed a detailed mobilization plan for reference. No autologous transplant population was mentioned by Kim et al. (2021). The bar chart of the quality assessment is shown in , indicating that the risk mostly comes from the patient selection ().
Figure 3. Forest plot of sensitivities and specificities on the diagnostic accuracy studies of XN-HPC.
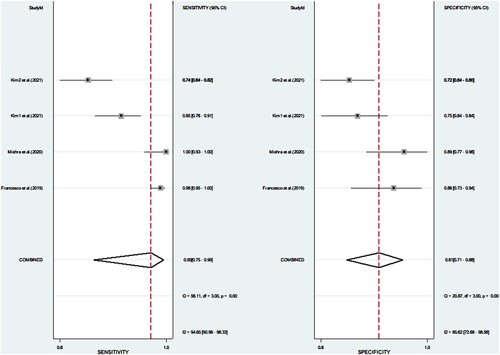
Table 1. Main characteristics of four studies included in the meta-analysis for the diagnosis of XN-HPC.
Meta-analysis outcomes
Our research included four studies that assessed the diagnosis performance of XN-HPC in a hematopoietic progenitor cell collection. The pooled accuracy was 95.4% (95% CI, 94.3–96.3), SEN was 0.95 (95% CI, 0.75–0.99), SPE was 0.81 (95% CI, 0.71–0.88), PLR was 5.0 (95% CI, 3.0–8.5), NLR was 0.06 (95% CI, 0.01–0.37), DOR was 78 (95% CI, 9–707), and the summary of the area under the ROC was 0.90 (95% CI 0.87–0.92; ).
Validation of meta-analysis results
Verification of meta-analysis results in the four studies Francesco et al. (2019), Mishra et al. (2020), Kim et al. (2021a), and Kim et al. (2021b), revealed that the pooled SEN and PLR were 0.93, 31.68, and 61.23 respectively, whereas the pooled SPE and NLR were only 0.81 and 0.52, respectively. In the forest map, the P-value of the Q test of sensitivity was <0.01, indicating that the heterogeneity included in the study was statistically significant. I2 statistics were 94.65%, indicating that the heterogeneity was relatively large (I2 > 50%: the heterogeneity is more apparent). The Q test of the same kind of specificity shows that the heterogeneity included in the study was statistically significant, whereas the I2 value was 85.62%, indicating a high degree of heterogeneity ( ).
Figure 5. Forest plot of multiple univariable meta-regression and subgroup analysis for sensitivities and specificities of XN-HPC.
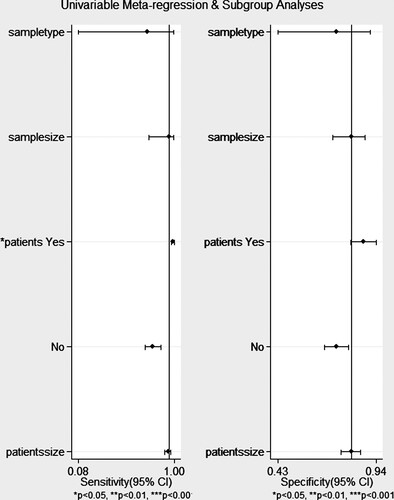
To explore the potential heterogeneity, meta-regression and subgroup meta-analysis were performed. In general, the performance of the trial was affected by the type of sample accepted, number of samples, type of disease, and number of patients. Our small sample size study shows that the inclusion of the type of diseases of patients affects the heterogeneity of the study. In other words, autologous and allogeneic grafts should not be treated equally with predicted yields via XN-HPC. In order to explore the source of heterogeneity, we included the criteria in Furundarena et al. (2019) using sensitivity and specific forest maps. We found that the sensitivity of the I2 value decreased from 94.65 to 92.37, and specificity I2 statistics decreased by 10% (unpresention).
Discussion
Peripheral blood stem cell transplantation is an acceptable and preferred approach, especially for high-risk hematologic malignancies, a better approach for the prevention of chronic GVHD, and a source of hematopoietic progenitor cells for transplantation from HLA-matched related donors [Citation1, Citation15, Citation16]. Additionally, it is a convenient and economical method. In recent years, peripheral blood stem cell transplantation has witnessed the emergence of XN-HPC as a viable candidate for predicting the number of CD34 cells. Sysmex® XN-HPC could also be considered a reliable system for apheresis for stem cell enumeration. A greater degree of standardization could be achieved due to its efficiency, ease of implementation, and the possibility of simultaneously performing a complete blood count. Furthermore, it also reduces the mental and physical strain on the patients, as well as the burden of cell processing on clinical engineers and medical staff [Citation5, Citation6, Citation17].
It has been seven years since the earliest application of the XN series to study the diagnostic performance of HPC in peripheral blood stem cell collections. Some studies have proved the accuracy of XN-HPC tests in the diagnosis of hematopoietic progenitor cells, with a reported SEN of 73.5–100% and SPE of 72–100% [Citation11, Citation18–26]. XN-HPC does offer certain advantages. As a 24-hour online method to detect hematopoietic progenitor cells, XN-HPC has attracted attention from hematopoietic stem cell transplantation experts.
In this study, we focused on the pooled accuracy, specificity (SPE), sensitivity (SEN), negative likelihood ratio (NLR),positive likelihood ratio (PLR). The pooled accuracy was ≤95.4%. PLR was ≤5.0, which manifested when the value of XN-HPC was positive and the donor was receiving sufficient products. NLR was 0.06, indicating the time of re-mobilization and collection. The AUC was 0.90. Simultaneously, we showed that the type of collection donors included in the study was a factor that affected the heterogeneity of the study. We concluded that we may not be able to reflect the role of sample type, sample size, and patient number because of the small sample size.
The records included in this study exhibited the following characteristics. In the SMC and HSC groups studied by Kim et al. (2021) [Citation11, Citation18–26], the moderate correlations between PB-HPC and CD34+ were 0.71 and 0.66, respectively. Especially the patients with plasma cell tumors exhibited a weak correlation between XN-HPC count and CD34+ cell count, measured using flow cytometry. This indicates that XN-HPC counting should be used cautiously. Mishra et al. (2020) [Citation28] found that the correlation between XN-HPC and CD34+ cells was 0.887 in peripheral blood and 0.847 in harvested products. This showed that the adequacy of the predicted harvest product of HPC was equivalent to that of CD34+ cells. Francesco et al. (2019) [Citation21] started from precision and functional sensitivity. HPC replaced the CD34+ cell count during the PBSC harvest, thus significantly improving the single mining workflow. This analysis could predict the time of a single blood collection with 100% SPE and 100% PPV. Kong et al. [Citation29] used the XE-2100 model to study the best time for autologous PBSC collection in patients with lymphoma and multiple myeloma. They found that XN-HPC count can be used to monitor and optimize clinical PBSC collection times. When Kasane et al. [Citation24] studied the collection time of autologous peripheral blood stem cells, they found that XN-HPC was an economic, convenient, and rapid index test to evaluate the collection time of peripheral blood stem cells on the day of and day before collection. Jamal et al. [Citation26] conducted a verification experiment and found that the positive predictive value was 100% and the negative predictive value was 17% when the XN-HPC cut-off value was 1.845 × 106 cells/kg of the recipient’s body weight, which was used as a cost-effective and time-saving substitute for the CD34+ cell count. Furundarena et al. (2019) [Citation22] proposed a decision-making algorithm that could be used for clinical management of peripheral blood stem cell collection through a hierarchical design, based on HPC to determine low-zone exclusion combined with high-zone diagnosis and CD34+ cell algorithm. In another study, multivariate analysis showed that the earliest HPC count was a good indicator of the optimal timing of PBSCH. The influencing factors were threshold setting and age [Citation30].
Coincidentally, Söderström et al. [Citation25] found that the correlation coefficient between peripheral blood and CD34 based on XN-1000, XN-HPC was 0.73, but considering the poor consistency in the low range of clinical decision-making, it was recommended to carefully use the XN-HPC count as an alternative indicator of CD34+ cell count. Al Mamari et al. [Citation31] found that stem cell count in apheresis products was not recommended based on XN-2000, because the Pearson correlation coefficient between the median HPC count and number of CD34+ cells was only 0.58, while that in peripheral blood could reach 0.79, implicating the heterogeneity from the specimen type possibly.
In addition to the heterogeneity mentioned above, the limitations of our study should also be taken into account. First, considering the current practicability, the XN series was selected as the model for testing HPC in the inclusion standard, which lead to selection of only few studies. Second, the total number of eligible studies and patients included in the meta-analysis was small, in part because only a small number of published studies investigated the diagnostic ability. We suggest to establish a multi-center clinical prediction model to overcome these limitations.
To conclude, XN-HPC should be used as a marker for quantitative detection of peripheral blood hematopoietic progenitor cells due to excellent diagnostic performance.
Disclosure statement
No potential conflict of interest was reported by the author(s).
Data availability statement
There is not a data set associated with this submission.
Additional information
Funding
References
- Amouzegar A, Dey BR, Spitzer TR. Peripheral blood or bone marrow stem cells? Practical considerations in hematopoietic stem cell transplantation. Transfus Med Rev. 2019;33:43–50.
- Grommé M, Russcher H, Braakman E, et al. Multicenter study to evaluate a new enumeration method for hematopoietic stem cell collection management. Transfusion. 2017;57:1949–1955.
- Tanosaki R, Kumazawa T, Yoshida A, et al. Novel and rapid enumeration method of peripheral blood stem cells using automated hematology analyzer. Int J Lab Hematol. 2014;36:521–530.
- Körbling M, Freireich EJ. Twenty-five years of peripheral blood stem cell transplantation. Blood. 2011;117:6411–6416.
- Iftikhar R, Chaudhry QUN, Anwer F, et al. Allogeneic hematopoietic stem cell transplantation in aplastic anemia: current indications and transplant strategies. Blood Rev 2021;47:100772.
- Tian M, Li X, Liu F, et al. Efficacy of peripheral arterial access for peripheral blood stem cells collection. J Clin Apher. 2021;36:864–869.
- Steussy BW, Capper M, Krasowski MD, et al. Algorithms utilizing peripheral blood hematopoietic progenitor cell counts in lieu of some CD34+ cell counts predict successful peripheral blood stem cell collections with substantial time and cost savings. ISBT Sci Ser. 2016;11:153–162.
- Fatorova I, Blaha M, Lanska M, et al. Timing of peripheral blood stem cell yield: comparison of alternative methods with the classic method for CD34+ cell determination. Biomed Res Int. 2014;2014:1–13.
- Lefrère F, Zohar S, Beaudier S, et al. Evaluation of an algorithm based on peripheral blood hematopoietic progenitor cell and CD34+ cell concentrations to optimize peripheral blood progenitor cell collection by apheresis. Transfusion. 2007;47:1851–1857.
- Oelschlaegel U, Bornhaeuser M, Thiede C, et al. HPC enumeration with the Sysmex XE-2100 can guide further flow cytometric CD34+ measurements and timing of leukaphereses. Cytotherapy. 2003;5:414–419.
- Al Mamari S, Al Saadi A, Al Zaabi R, et al. Measurement of hematopoietic progenitor cells using XN2000 hematology analyzer. Int J Lab Hematol. 2022;44:82–87.
- Padmanabhan A, Reich-Slotky R, Jhang JS, et al. Use of the haematopoietic progenitor cell parameter in optimizing timing of peripheral blood stem cell harvest. Vox Sang 2009;97:153–159.
- Jamal A, Khan T, Zaidi U, et al. Highly specific functional equivalence of XN-HPC for optimum CD34+ cell count in harvested allogeneic bone marrow stem cell products. Hematology. 2022;27:232–238.
- Park SH, Park CJ, Kim MJ, et al. Evaluation of parameters obtained from the Sysmex XN-2000 for predicting the recovery of the absolute neutrophil count and platelets after hematopoietic stem cell transplantation. Int J Lab Hematol. 2016;38:198–208.
- Champlin RE, Schmitz N, Klein JP, et al. Blood stem cells compared with bone marrow as a source of hematopoietic cells for allogeneic transplantation. Blood. 2000;95:3702–3709.
- Melve GK, Ersvaer E, Eide GE, et al. Peripheral blood stem cell mobilization in healthy donors by granulocyte colony-stimulating factor causes preferential mobilization of lymphocyte subsets. Front Immunol. 2018;9:845–859.
- Kong JH, Hu Y, Kong SY, et al. Analysis of laboratory parameters for optimal autologous peripheral blood stem cell collection from lymphoma and myeloma patients. J Clin Apher. 2021;36:135–142.
- Kim SM, Kim HY, Kim SJ, et al. Correlation between peripheral blood automated hematopoietic progenitor cell counts and flow cytometric CD34+ cell counts differs according to diagnosis in patients undergoing autologous peripheral blood stem cell transplantation. J Clin Apher. 2021;36:737–749.
- Mishra S, Kulkarni U, Mathews N, et al. A study to compare Hematopoietic Progenitor Cell count determined on a next-generation automated cell counter with flow cytometric CD34 count in peripheral blood and the harvested peripheral blood stem cell graft from autologous and allogenic donors. Int J Lab Hematol. 2021;43:76–83.
- Kasane M, Kurosawa S, Kojima M, et al. Usefulness of hematopoietic progenitor cell monitoring to predict autologous peripheral blood stem cell harvest timing: a single-center retrospective study. Transfus Apher Sci. 2021;60:103150.
- Dima F, Barison E, Midolo M, et al. Assessment of haematopoietic progenitor cell counting with the Sysmex ® XN-1000 to guide timing of apheresis of peripheral blood stem cells. Blood Transfusion. 2020;18:67–76.
- Furundarena JR, Uranga A, Alkorta A, et al. Evaluation of the predictive value of the hematopoietic progenitor cell count using an automated hematology analyzer for CD34+ stem cell mobilization and apheresis product yield. Int J Lab Hematol. 2020;42:170–179.
- Grommé M, Russcher H, Braakman E, et al. Multicenter study to evaluate a new enumeration method for hematopoietic stem cell collection management. Transfusion. 2017;57:1949–1955.
- Kasane M, Kurosawa S, Kojima M, et al. Usefulness of hematopoietic progenitor cell monitoring to predict autologous peripheral blood stem cell harvest timing: A single-center retrospective study. Transfus Apher Sci. 2021;60:103150.
- Söderström A, Møller BK, Sørensen BS. Evaluation of the Sysmex XN automated hematopoietic progenitor cell enumeration for timing of peripheral blood stem cell harvest. Transfus Apher Sci. 2020;59:102683.
- Jamal A, Khan MT, Parveen S, et al. Peripheral blood stem cell harvest HPC count Is an effective surrogate marker for CD34+ cell count in allogeneic stem cell transplant setting. Transl Oncol. 2020;13:100788.
- Kim SM, Kim HY, Kim SJ, et al. Correlation between peripheral blood automated hematopoietic progenitor cell counts and flow cytometricCD34+ cell counts differs according to diagnosis in patients undergoing autologous peripheral blood stem cell transplantation. J Clin Apher. 2021;36:737–749.
- Mishra S, Kulkarni U, Mathews N, et al. A study to compare Hematopoietic Progenitor Cell count determined on a next-generation automated cell counter with flow cytometric CD34 count in peripheral blood and the harvested peripheral blood stem cell graft from autologous and allogenic donors. Int J Lab Hematol. 2021;43:76–83.
- Kong JH, Hu Y, Kong SY, et al. Analysis of laboratory parameters for optimal autologous peripheral blood stem cell collection from lymphoma and myeloma patients. J Clin Apher. 2021;36:135–142.
- Tanaka H, Ishii A, Sugita Y, et al. Impact of hematopoietic progenitor cell count as an indicator for optimal timing of peripheral stem cell harvest in clinical practice. J Clin Exp Hematopathol. 2017;56:150–159.
- Al Mamari S, Al Saadi A, Al Zaabi R, et al. Measurement of hematopoietic progenitor cells using XN2000 hematology analyzer. Int J Lab Hematol. 2022;44:82–87.