ABSTRACT
Background
The COVID-19 pandemic affected healthcare delivery globally, impacting care access and delivery of essential services.
Objectives
We investigated the pandemic’s impact on care for patients with type 2 diabetes and factors associated with care disruption in Kenya and Tanzania.
Methods
A cross-sectional study was conducted among adults diagnosed with diabetes pre-COVID-19. Data were collected in February–April 2022 reflecting experiences at two time-points, three months before and the three months most affected by the COVID-19 pandemic. A questionnaire captured data on blood glucose testing, changes in medication prescription and access, and healthcare provider access.
Results
We recruited 1000 participants (500/country). Diabetes care was disrupted in both countries, with 34.8% and 32.8% of the participants reporting change in place and frequency of testing in Kenya, respectively. In Tanzania, 12.4% and 17.8% reported changes in location and frequency of glucose testing, respectively. The number of health facility visits declined, 14.4% (p < 0.001) in Kenya and 5.6% (p = 0.001) in Tanzania. In Kenya, there was a higher likelihood of severe care disruption among insured patients (adjusted odds ratio [aOR] 1.56, 95% confidence interval [CI][1.05–2.34]; p = 0.029) and a lower likelihood among patients residing in rural areas (aOR, 0.35[95%CI, 0.22–0.58]; p < 0.001). Tanzania had a lower likelihood of severe disruption among insured patients (aOR, 0.51[95%CI, 0.33–0.79]; p = 0.003) but higher likelihood among patients with low economic status (aOR, 1.81[95%CI, 1.14–2.88]; p = 0.011).
Conclusions
COVID-19 disrupted diabetes care more in Kenya than Tanzania. Health systems and emergency preparedness should be strengthened to ensure continuity of service provision for patients with diabetes.
Paper Context
Main findings: The COVID-19 pandemic disrupted diabetes care in Kenya and Tanzania resulting in changes in place and frequency of blood glucose testing, medication prescribed (less oral hypoglycaemics and more insulin), fewer health facility visits and more difficulty accessing healthcare providers.
Added knowledge: This study quantifies the impact of the COVID-19 pandemic on diabetes care in Kenya and Tanzania, and describes the factors associated with care disruption in both countries.
Global health impact for policy and action: Evidence on diabetes care disruption is useful in making plans and policies responsive to the needs of diabetes patients during pandemics or related emergency situations.
Responsible Editor Jennifer Stewart Williams
Background
The outbreak of coronavirus disease 2019 (COVID-19), caused by the severe acute respiratory syndrome coronavirus 2, resulted in a global pandemic [Citation1]. The pandemic, responsible for an estimated 770 million confirmed infections and 7 million deaths as of 21 September 2023, affected healthcare delivery globally [Citation2,Citation3]. In Africa, 9.5 million infections were documented by the World Health Organization by 21 September 2023 [Citation2]. At the peak of the pandemic, routine care for chronic diseases was adversely affected worldwide, with diabetes, chronic obstructive pulmonary disease, and hypertension most affected [Citation4]. The World Health Organization introduced the COVID-19 Strategic Preparedness and Action Plan to provide public health support measures to respond to the pandemic and ensure continuity of essential services [Citation5]. African countries faced significant challenges, due to the fragile health systems with inadequate healthcare resources and personnel [Citation6,Citation7]. The shift of healthcare resources to the COVID-19 emergency response and measures instituted by governments to control the spread of the virus, such as lockdown and ‘stay-at-home’ measures disrupted care [Citation8,Citation9].
An efficient and functional healthcare system results in effective diabetes care. Type 2 diabetes, a chronic disease, requires continuity of care involving routine consultation, blood glucose monitoring, screening and management of complications and co-morbidities. Discontinuity of care results in poor control and an increased risk of complications [Citation10]. The COVID-19 pandemic did not spare patients with type 2 diabetes, with evidence showing an increase in the proportion of patients with poor treatment control in Ethiopia from 24.8% pre-COVID-19 to up to 36.% during the pandemic [Citation11] and experts highlighting the importance of timely and effective management [Citation12]. Also, having type 2 diabetes predisposes individuals to severe forms of COVID-19 [Citation13]. However, evidence of the impact of the pandemic on diabetes care is limited in the two East African countries of Kenya and Tanzania. According to estimates by the International Diabetes Federation, in 2021, 821,500 (3% prevalence) and 2,884,000 (10.3% prevalence) adults had diabetes in Kenya and Tanzania, respectively [Citation14]. In Kenya and Tanzania provide two interesting contexts for this assessment because of the quite different approaches deployed to manage the pandemic. Whereas Kenya instituted strict lockdowns and curfews at the peak of the pandemic, Tanzania opted for a less stringent approach without lockdowns and curfews. However, it is not known whether these two different approaches have led to different outcomes in terms of diabetes care in the two countries.
Knowledge of the impact of the COVID-19 pandemic on diabetes care is helpful in planning care for patients with type 2 diabetes and chronic diseases in pandemic situations. Such evidence will be essential to strengthen healthcare services and to make national plans and policies responsive to the needs of type 2 diabetes patients during pandemics or related emergency situations. Therefore, we investigated the extent of healthcare disruption resulting from the COVID-19 pandemic and associated factors among patients with type 2 diabetes in Kenya and Tanzania.
Methods
Study design and setting
This was a cross-sectional survey study conducted in urban and rural locations in Kenya and Tanzania in East Africa between February – April 2022. In Kenya surveys were conducted in four counties: Nairobi and Kiambu (predominantly urban); and Nyeri and Vihiga (rural). In Tanzania, surveys were conducted in Dar es Salaam (urban) and Morogoro (rural) regions.
Participants
Patients with type 2 diabetes receiving care at selected health facilities were invited to participate. Lists of type 2 diabetes patients receiving care from these health facilities were obtained from patient records. We included consenting adults (aged ≥18 years) of all gender, with or without co-morbidities (such as hypertension), who had commenced type 2 diabetes care before the COVID-19 pandemic declaration in Kenya and Tanzania (March 2020). Patients who were bedridden, pregnant or breastfeeding and those unable to comprehend study information were excluded.
Variables
The outcome variables measured were: (1) change in where testing took place; (2) change in frequency of blood glucose testing; (3) change in prescribed medication; (4) change in access to prescribed medication; (5) change in number of health facility visits in the preceding three months; (6) change in the cost of medication; and (7) change in access to a health provider. A ‘disruption’ variable was developed as an unweighted composite measure of the outcome variables and categorised into ‘no disruption’, ‘moderate disruption’, and ‘severe disruption’. Disruption was considered ‘severe’ if four or more of the above outcomes were experienced and ‘moderate’ if one to three outcomes were experienced. Socio-demographic and health-related variables, such as age, sex, location, marital status, occupation, education, economic status, health insurance coverage, family history of diabetes, presence of co-morbidities and known duration with type 2 diabetes were measured and considered as potential predictors of disruption. Socio-economic status was assessed subjectively using an economic ladder question (1–10 scale) because most of the participants did not have formal employment with regular income to estimate wealth and the ladder has been validated as a measure of socioeconomic status among such populations (graphically represented with 10 rungs) [Citation15].
Data sources/measurements
The study protocols and tools were aligned across countries to ensure consistency. Before the commencement of the surveys, the field teams were trained, and study tools piloted. In collaboration with healthcare providers, experienced, trained fieldworkers contacted study participants to make appointments. Informed consent was obtained from eligible participants and a questionnaire administered. With the questionnaire, data were collected on socio-demographic characteristics, medical history and the impact of the COVID-19 pandemic on diabetes care. Participants were asked to report on their experiences at two time-points, during the three months before COVID-19 was confirmed in both countries (March 2020) and the three months during the pandemic when they felt their care was most affected. Also, information was collected on reasons for any disruption of care. Interviews were conducted by telephone in Kenya and face-to-face in Tanzania, owing to the differing COVID-19 regulations in the two countries.
Sample size considerations
We planned to recruit 500 participants in each country (1000 participants). The sample size was estimated using the Cochran formula [Citation16], assuming 50% of patients with type 2 diabetes experienced disruption of care during COVID-19 [Citation17], considering a power of 80% at 95% confidence interval, 5% acceptable sampling error and a non-response rate of 30%.
Statistical analysis
All statistical analyses were done using STATA version 16 (College Station, Texas). Descriptive characteristics of individuals in Kenya were reported alongside those of Tanzania and presented as frequencies and percentages as well as mean and standard deviation for continuous variables. Differences in the individual disruption variables before and during the COVID-19 were assessed for statistically significant differences using the independent samples T-test. We described the levels of disruption (none, moderate and severe) graphically using bar charts. To identify predictors of disruption in three levels, we conducted a univariate ordered logistic regression and then, a multivariate ordered logit model including pre-selected co-variates was built. The selection of factors was made a priori and based on the WHO social determinants of health and wellbeing framework [Citation18]. Crude and Adjusted odds ratios, 95% confidence intervals and p-values are reported. A p-value of less than 0.05 was considered statistically significant. The analyses are country-specific and presented alongside each other.
Results
Between February and April 2022, 1000 participants (500 in each country) consented to participate in the study. In Kenya, most participants were recruited from Nairobi (276) and Kiambu (104) counties. In Tanzania, 300 and 200 participants were recruited from Dar es Salaam and Morogoro, respectively.
The characteristics of the study participants are shown in . The participants were predominantly female (66% [330/500] and 67.2% [336/500] in Kenya and Tanzania, respectively). In both countries, the participants were of similar age (Kenya 58.2 years [standard deviation (SD) 12.6] and Tanzania 56.8 years [SD 10.2]). However, in the above 70 category, Kenya had 18.2% while Tanzania had 6.2%. Most of the participants were of low socio-economic status (Kenya 89.4% (447/500), Tanzania 74.8% (374/500)). In Kenya, 55.2% of the participants had secondary education and higher while 33.6% had the same in Tanzania.
Table 1. Socio-demographic characteristics of type 2 diabetes patients who participated in the study in Kenya and Tanzania.
The proportions of participants who reported changes in diabetes care parameters due to the COVID-19 pandemic are shown in . Overall, a higher proportion of participants in Kenya compared to Tanzania reported changes across the parameters studied. In Kenya, blood glucose testing was the most disrupted component of care, with 34.8% (178/500) and 32.8% (164/500) changed place and frequency of testing in Kenya, respectively. In Tanzania, 12.4% (62/500) reported a change in place of testing and 17.8% (89/500) reported a change in the frequency of testing. In Kenya, there was a relative decline in testing at home (29.6% to 20%; p = 0.0019) and an increase in testing at health facilities (39.4–96.0%; p < 0.0001). In Tanzania, the reverse was observed with an increase in testing at home (2.6–7.2%; p < 0.001) and a decline in testing at health facilities (86.6–81.0%; p < 0.0001). Participants did less testing during the pandemic in Kenya as shown by a decline in testing at least once a day and an increase in testing at least twice a week. In both countries, there was a decline noted in the prescription of oral hypoglycaemics (Kenya, −2.6% [p < 0.0001]; Tanzania, −2.2% [p = 0.002]) and an increase noted for prescription of insulin (Kenya, 2.6% [p < 0.0019]; Tanzania, 2.2% [p = 0.002]) during the pandemic. There was a 14.4% (p < 0.0001) decline in the number of health facility visits in Kenya and a 5.6% (p = 0.001) decline in Tanzania.
Table 2. Diabetes care before and during the COVID-19 pandemic in Kenya and Tanzania.
A breakdown of the cumulative number of disruptions is shown in . Of the participants who experienced disruption, the majority experienced moderate disruption (Kenya 62.6%, Tanzania 47%; ).
Figure 1. Proportion of cumulative disruptions of diabetes care disruption due to COVID-19 in Kenya and Tanzania.
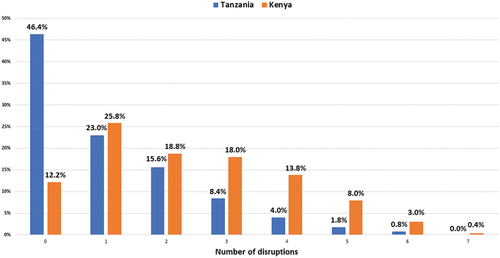
Figure 2. Levels of diabetes care disruption due to COVID-19 in Kenya and Tanzania.
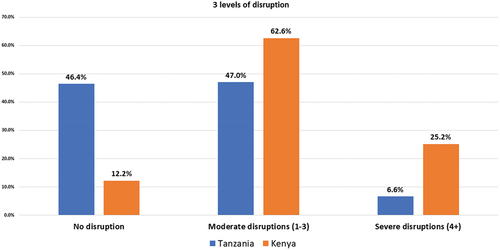
The factors associated with disruption are shown in . In the bivariate analysis (), rural living was associated with less disruption in Kenya. In Tanzania, rural living, being older (70+ years) and having health insurance were associated with less disruption. However, being of low socioeconomic status was associated with severe disruption.
Table 3. Predictors of severe disruption of diabetes care in Kenya and Tanzania (bivariate analysis).
Table 4. Predictors of severe disruption of diabetes care in Kenya and Tanzania (multivariate analysis).
In the multivariate analysis (), the results are as follows. In Kenya, there was a higher likelihood of severe disruption among insured participants compared to the uninsured (adjusted odds ratio [aOR] 1.56 95% confidence interval [CI] [1.05, 2.34] p = 0.029). There was a lower likelihood of severe disruption among participants residing in rural areas (aOR 0.35[0.22–0.58] p < 0.001). A weak association with less disruption was noted for participants who have lived with type 2 diabetes for less than six years (aOR 0.72[0.49–1.06] p = 0.093). In contrast, the factors associated with severe disruption in Tanzania were health insurance cover and economic status. Participants with health insurance were less likely to be severely disrupted (aOR 0.51[0.33, 0.79] p = 0.003). However, participants from the lower socio-economic stratum were more likely to be severely disrupted (aOR 1.81[1.14, 2.88] p = 0.011). Some borderline association (lower likelihood) with severe disruption was observed for older participants (70+ years) (aOR 0.37[0.12, 1.17] p = 0.092) and farmers (aOR 0.48[0.21, 1.09] p = 0.080).
Discussion
In this paper, we have shown that the COVID-19 pandemic disrupted diabetes care in Kenya and Tanzania. The disruption resulted in changes in place and frequency of blood glucose testing, medication prescribed (less oral hypoglycaemics and more insulin), fewer health facility visits and more difficulty accessing healthcare providers. Health insurance was a predictor of severe disruption in both countries but in different directions. In Kenya, having health insurance was positively associated with severe disruption, whereas there was an inverse association in Tanzania. Urban living and belonging to a lower economic status were associated with severe disruption in Kenya and Tanzania, respectively.
The disruption of diabetes care highlighted here has been reported elsewhere. For example, the pandemic resulted in an increase in poor control of diabetes and hypertension in Ethiopia [Citation11]. Patients with diabetes in Rwanda reported challenges with care access [Citation19]. Significant reductions were noted in outpatient visits and glycated haemoglobin (HbA1c) measurements among U.S. veterans in the first three months of the pandemic [Citation20] and in diabetic patients in Italy [Citation21]. There was disruption of care and a reduction in clinic visits by patients with diabetes in India [Citation22].
Diabetes care was disrupted in both Kenya and Tanzania despite the two countries deploying different approaches to combat the pandemic. Patients with type 2 diabetes are at a higher risk of developing severe forms of COVID-19 and death due to COVID-19 compared to individuals without co-morbidities [Citation13]. Patients were well informed about this vulnerability during the pandemic and fear of exposure to COVID-19 could potentially have deterred visits to healthcare facilities [Citation4]. Furthermore, travel restrictions in Kenya and loss of employment and income due to the pandemic in both countries may have impacted diabetes care seeking.
Disruption of care was not only limited to diabetes. The pandemic resulted in the reduction of general inpatient utilization in Kenya [Citation23]. More broadly, there was a reduction in service utilization of maternal, newborn and child health services in eastern Africa [Citation9,Citation24] and disruption of cancer, TB and HIV services [Citation3,Citation25,Citation26]. The pandemic also affected planned healthcare delivery and resource allocation for persons with chronic diseases [Citation27].
Our study reported a decline in prescriptions of oral hypoglycaemics and an increase in insulin prescriptions among patients with type 2 diabetes. The disruption of care may have resulted in poor blood sugar control, necessitating a prescription change. Also, the poor control could have resulted from limited access to healthy and affordable food, limited physical activity opportunities due to restrictions on movement, and increased stress. The pandemic increased stress and anxiety for many people, which can affect blood sugar control [Citation28].
Urban living was associated with severe disruption in Kenya. The pandemic impacted livelihoods and may have affected the urban areas more than the rural ones. Many people living in urban areas work in the informal sector and may have lost their livelihoods due to the pandemic. For example, lockdowns disrupted businesses and employment in Nairobi [Citation29], making it difficult for people to afford healthcare. Also, lockdown measures enforced in Kenya (including the ban of public transport) may have impacted urban and rural areas differently, considering that public transportation is a major mode of travel in urban areas, which impedes travel to healthcare facilities. Furthermore, we cannot rule out differences in baseline care in rural and urban areas. With less formalised and poorer baseline care, there could have been less disruption in rural areas compared to urban areas. In addition, fewer COVID-19 infections were reported in rural areas and there was the common belief that COVID-19 was an ‘urban’ disease. This may have resulted in lower risk perception of the disease in rural areas [Citation30].
Belonging to a lower economic status was associated with severe disruption in Tanzania. Low economic status can lead to more disruption of diabetes care during the COVID-19 pandemic by limiting access to healthcare facilities, medication, testing supplies, information, and education. People with low socio-economic status may live in areas with limited access to healthcare facilities, making it difficult to receive regular diabetes care. This problem can be exacerbated during the pandemic when movement restrictions and limited transport services make it difficult for people to travel to healthcare facilities. Also, there are challenges with affording medication and testing supplies for diabetes care. During the pandemic, these challenges were exacerbated by supply chain disruptions and economic hardships [Citation31,Citation32].
Having health insurance is expected to result in better healthcare access and outcomes [Citation33]. Health insurance can help individuals access diabetes care during the pandemic by reducing the financial burden of healthcare expenses. It was, therefore, surprising to find that health insurance was a predictor of severe diabetes care disruption in Kenya. Health insurance has been shown to have limited effects on quality of care in low-income countries [Citation34]. In some situations, health insurance could potentially disrupt diabetes care during the pandemic. Some health insurance plans in Kenya may not provide adequate coverage for diabetes services [Citation35]. Finally, health insurance may not effectively ensure access to diabetes care if healthcare systems are overwhelmed with COVID-19 patients. In such situations, even individuals with health insurance may face challenges accessing diabetes care due to diverted resources and limited healthcare capacity.
Our study had several strengths. Our data are from two countries that adopted quite different approaches to the pandemic, providing two different contexts to diabetes care disruption. Also, the study design was robust and the sample size adequate, ensuring that the results are valid, reliable and generalizable. The study had some limitations, which we acknowledge. The data collection was cross-sectional, leading to temporal ambiguity and also making it difficult to infer causation. This was mitigated by collecting data for two time points, before and during the pandemic’s peak. Because of the retrospective nature of data, we cannot rule out recall bias. We also acknowledge that conditions in Kenya and Tanzania are not the same, influenced by the different country contexts. Lastly, the selection of the study sites was purposive and not random. To ensure that nationally representative data were collected, urban and rural study sites were selected in both countries.
The disruption of diabetes care during the COVID-19 pandemic in Kenya and Tanzania highlights several important issues. Our findings demonstrate the need for strong healthcare infrastructure support to ensure continuity of care during pandemic or related emergency situations for people with type 2 diabetes to ensure access to regular check-ups, and medication. Both countries should learn from the disruptions in healthcare caused by the pandemic and invest in improving healthcare infrastructure, including increasing the number of healthcare workers and expanding access to healthcare facilities. Telemedicine had emerged as a vital tool during the pandemic, allowing healthcare providers to provide remote care and consultation to patients with diabetes. Some telemedicine initiatives have been deployed in Kenya [Citation36–38] and Tanzania [Citation39]. Investment in expanding access to telemedicine services for people with diabetes would be beneficial [Citation12,Citation40].
Conclusions
The pandemic has highlighted the importance of social safety nets and ensuring continuity of essential health services to protect vulnerable populations, including people with diabetes who may have lost their livelihoods due to the pandemic. Investing in social safety nets ensures that people with diabetes have access to affordable medication and testing supplies, even during economic hardship. Overall, the disruption of diabetes care during the COVID-19 pandemic in Kenya and Tanzania highlights the need for stronger healthcare infrastructure, more innovative approaches to diabetes care, and social safety nets to protect vulnerable populations. By learning from these disruptions, Kenya and Tanzania can work towards improving diabetes care and mitigating the impact of future pandemics on diabetes care.
Author contributions
Conception and design of the study: GA, SM and CMG
Data acquisition: RES, PB, CK, SFM, LK, PK, IM, GM
Data analysis: CK and PB
Data interpretation: RES, PB, CK, SFM, LK, PK, IM, GM, CB, MD, FSM, EG, CMG, SM, GA
Drafting of the first version of the manuscript: RES
Critical review of the manuscript: PB, CK, SFM, LK, PK, IM, GM, RES, CB, MD, FSM, EG, CMG, SM, GA
All authors read and approved the final manuscript.
Ethics and consent
Ethical approval for this work was obtained both in Kenya and Tanzania. In Kenya, approval was obtained from the African Medical and Research Foundation (AMREF) Health Africa’s Ethics and Scientific Research Committee (ref. no: AMREF-ESRC P900/2020) and the National Commission for Science, Technology and Innovation (licence no: NACOSTI/p22/14986). In Tanzania, the Ifakara Health Institute Ethics Committee (ref no: IHI/IRB/No: 38–2021) and the National Health Research Ethics Committee (ref no: NIMR/HQ/R.8a/Vol.X/3806) granted ethical approval. All participants provided informed consent.
Acknowledgments
The authors thank members of the field teams who participated in data collection in Kenya and Tanzania.
Disclosure statement
No potential conflict of interest was reported by the author(s).
Data availability statement
The datasets used and/or analyzed during the current study are available from the corresponding author on reasonable request.
Additional information
Funding
References
- World Health Organisation. Novel Coronavirus (2019-nCoV) situation report – 22; [ cited 2020]. Available from: https://www.who.int/docs/default-source/coronaviruse/situation-reports/20200211-sitrep-22-ncov.pdf?sfvrsn=fb6d49b1_2
- World Health Organisation. WHO coronavirus disease (COVID-19) dashboard; 2023 [cited 2023 Sept 25]. Available from: https://covid19.who.int
- Arsenault C, Gage A, Kim MK, Kapoor NR, Akweongo P, Amponsah F, et al. COVID-19 and resilience of healthcare systems in ten countries. Nature Med. 2022;28:1314–11. doi: 10.1038/s41591-022-01750-1. Epub 20220314. PubMed PMID: 35288697; PubMed Central PMCID: PMC9205770.
- Chudasama YV, Gillies CL, Zaccardi F, Coles B, Davies MJ, Seidu S, et al. Impact of COVID-19 on routine care for chronic diseases: a global survey of views from healthcare professionals. Diabetes Metab Syndr. 2020;14:965–967. doi: 10.1016/j.dsx.2020.06.042. Epub 20200623. PubMed PMID: 32604016; PubMed Central PMCID: PMC7308780.
- World Health Organization. COVID‑19 strategic preparedness and response plan: monitoring and evaluation framework, 11 May 2021. Geneva (Switzerland): World Health Organization; 2021.
- Amu H, Dowou RK, Saah FI, Efunwole JA, Bain LE, Tarkang EE. COVID-19 and health systems functioning in Sub-Saharan Africa using the “WHO building blocks”: the challenges and responses. Front Public Health. 2022;10:856397. doi: 10.3389/fpubh.2022.856397. Epub 20220404. PubMed PMID: 35444973; PubMed Central PMCID: PMC9013894.
- Tessema GA, Kinfu Y, Dachew BA, Tesema AG, Assefa Y, Alene KA, et al. The COVID-19 pandemic and healthcare systems in Africa: a scoping review of preparedness, impact and response. BMJ Glob Health. 2021;6:e007179. doi: 10.1136/bmjgh-2021-007179. PubMed PMID: 34853031; PubMed Central PMCID: PMC8637314.
- Delobelle PA, Abbas M, Datay I, De Sa A, Levitt N, Schouw D, et al. Non-communicable disease care and management in two sites of the Cape Town Metro during the first wave of COVID-19: a rapid appraisal. Afr J Prim Health Care Fam Med. 2022;14:e1–e7. doi: 10.4102/phcfm.v14i1.3443. Epub 20220118. PubMed PMID: 35144453; PubMed Central PMCID: PMC8831902.
- Amouzou A, Maïga A, Faye CM, Chakwera S, Melesse DY, Mutua MK, et al. Health service utilisation during the COVID-19 pandemic in Sub-Saharan Africa in 2020: a multicountry empirical assessment with a focus on maternal, newborn and child health services. BMJ Glob Health. 2022;7:e008069. doi: 10.1136/bmjgh-2021-008069. PubMed PMID: 35501068; PubMed Central PMCID: PMC9062456.
- Shah K, Tiwaskar M, Chawla P, Kale M, Deshmane R, Sowani A. Hypoglycemia at the time of Covid-19 pandemic. Diabetes Metab Syndr. 2020;14:1143–1146. doi: 10.1016/j.dsx.2020.07.003. Epub 20200710. PubMed PMID: 32668399; PubMed Central PMCID: PMC7347476.
- Ayele TA, Shibru H, Mequanent Sisay M, Melese T, Fentie M, Azale T, et al. The effect of COVID-19 on poor treatment control among ambulatory hypertensive and/or diabetic patients in Northwest Ethiopia. PLOS ONE. 2022;17:e0266421. doi: 10.1371/journal.pone.0266421. Epub 20220519. PubMed PMID: 35588110; PubMed Central PMCID: PMC9119481.
- Giorgino F, Bhana S, Czupryniak L, Dagdelen S, Galstyan GR, Janež A, et al. Management of patients with diabetes and obesity in the COVID-19 era: experiences and learnings from South and East Europe, the Middle East, and Africa. Diabet Res Clin Pract. 2021;172:108617. doi: 10.1016/j.diabres.2020.108617. Epub 20201210. PubMed PMID: 33310175; PubMed Central PMCID: PMC7728417.
- Vardavas CI, Mathioudakis AG, Nikitara K, Stamatelopoulos K, Georgiopoulos G, Phalkey R, et al. Prognostic factors for mortality, intensive care unit and hospital admission due to SARS-CoV-2: a systematic review and meta-analysis of cohort studies in Europe. Eur Respir Rev. 2022;31:220098. doi: 10.1183/16000617.0098-2022. Epub 20221102. PubMed PMID: 36323422.
- International Diabetes Federation. IDF diabetes atlas 2021. 10th ed. Brussels (Belgium): International Diabetes Federation; 2021.
- Operario D, Adler NE, Williams DR. Subjective social status: reliability and predictive utility for global health. Psychol Health. 2004;19:237–246. doi: 10.1080/08870440310001638098
- Cochran WG. Note on an approximate formula for the significance levels of z. Ann Math Stat. 1940;11:93–95. doi: 10.1214/aoms/1177731945
- Organization WH. Rapid assessment of service delivery for NCDs during the COVID-19 pandemic. Geneva: World Health Organization; 2020.
- Solar O, Irwin A. A conceptual framework for action on the social determinants of health. Geneva (Switzerland): WHO Document Production Services; 2010.
- Habineza JC, James S, Sibomana L, Klatman E, Uwingabire E, Maniam J, et al. Perceived impact of the COVID-19 pandemic on young adults with type 1 diabetes in Rwanda. Pan Afr Med J. 2021;40:252. doi: 10.11604/pamj.2021.40.252.28899. Epub 20211221. PubMed PMID: 35251446; PubMed Central PMCID: PMC8856971.
- Adhikari S, Titus AR, Baum A, Lopez P, Kanchi R, Orstad SL, et al. Disparities in routine healthcare utilization disruptions during COVID-19 pandemic among veterans with type 2 diabetes. BMC Health Serv Res. 2023;23:41. doi: 10.1186/s12913-023-09057-8. Epub 20230116. PubMed PMID: 36647113; PubMed Central PMCID: PMC9842402.
- Bellini B, Nreu B, Francesconi P, Mannucci E. Covid-19 pandemic, diabetes and glycemic control: results of a retrospective cohort study of 230.000 persons with diabetes in tuscany. Nutr Metab Cardiovasc Dis. 2022;32:2588–2593. doi: 10.1016/j.numecd.2022.07.017. Epub 20220806. PubMed PMID: 36064691; PubMed Central PMCID: PMC9356641.
- Khader MA, Jabeen T, Namoju R. A cross sectional study reveals severe disruption in glycemic control in people with diabetes during and after lockdown in India. Diabetes Metab Syndr. 2020;14:1579–1584. doi: 10.1016/j.dsx.2020.08.011. Epub 20200818. PubMed PMID: 32858476; PubMed Central PMCID: PMC7434486.
- Barasa E, Kairu A, Ng’ang’a W, Maritim M, Were V, Akech S, et al. Examining unit costs for COVID-19 case management in Kenya. BMJ Glob Health. 2021;6:e004159. doi: 10.1136/bmjgh-2020-004159. PubMed PMID: 33853843; PubMed Central PMCID: PMC8053308.
- Bekele C, Bekele D, Hunegnaw BM, Van Wickle K, Gebremeskel FA, Korte M, et al. Impact of the COVID-19 pandemic on utilisation of facility-based essential maternal and child health services from March to August 2020 compared with pre-pandemic March–August 2019: a mixed-methods study in North Shewa Zone, Ethiopia. BMJ Open. 2022;12:e059408. doi: 10.1136/bmjopen-2021-059408. Epub 20220603. PubMed PMID: 36437538.
- Dorward J, Khubone T, Gate K, Ngobese H, Sookrajh Y, Mkhize S, et al. The impact of the COVID-19 lockdown on HIV care in 65 South African primary care clinics: an interrupted time series analysis. Lancet HIV. 2021;8:e158–e65. doi: 10.1016/s2352-3018(20)30359-3. Epub 20210204. PubMed PMID: 33549166; PubMed Central PMCID: PMC8011055.
- SeyedAlinaghi S, Mirzapour P, Pashaei Z, Afzalian A, Tantuoyir MM, Salmani R, et al. The impacts of COVID-19 pandemic on service delivery and treatment outcomes in people living with HIV: a systematic review. AIDS Res Ther. 2023;20:4. doi: 10.1186/s12981-022-00496-7. Epub 20230106. PubMed PMID: 36609313; PubMed Central PMCID: PMC9821373.
- Ahoto AT, Wang W. Effects of COVID-19 outbreak on persons with chronic health conditions in Anglophone West Africa: a qualitative study involving key stakeholders. Inquiry. 2022;59:469580221092830. doi: 10.1177/00469580221092830. PubMed PMID: 35522190; PubMed Central PMCID: PMC9083033.
- García-Lara RA, Gómez-Urquiza JL, Membrive-Jiménez MJ, Velando-Soriano A, Granados-Bolivar ME, Romero-Béjar JL, et al. Anxiety, distress and stress among patients with diabetes during COVID-19 pandemic: a systematic review and meta-analysis. J Pers Med. 2022;12. doi: 10.3390/jpm12091412. Epub 20220830. PubMed PMID: 36143195; PubMed Central PMCID: PMC9506161.
- Joshi N, Lopus S, Hannah C, Ernst KC, Kilungo AP, Opiyo R, et al. COVID-19 lockdowns: employment and business disruptions, water access and hygiene practices in Nairobi’s informal settlements. Soc Sci Med. 2022;308:115191. doi: 10.1016/j.socscimed.2022.115191. Epub 20220706. PubMed PMID: 35930847; PubMed Central PMCID: PMC9258418.
- Akwaowo CD, Eyo CS, Umoh IO, Ekpin VI, Esubok NU. Perception and practices towards covid-19 amongst residents in Southern Nigeria. Niger Postgrad Med J. 2021;28:149–159. doi: 10.4103/npmj.npmj_554_21. PubMed PMID: 34708700.
- Ipagala P, Mlugu EM, Mwakalukwa R, Kagashe GA. Impact of COVID-19 on the supply chain of essential health commodities: a mixed method study, in Dar es Salaam, Tanzania. J Pharm Policy Pract. 2023;16:103. doi: 10.1186/s40545-023-00617-1. Epub 20230922. PubMed PMID: 37736737; PubMed Central PMCID: PMC10515070.
- Francis JR. COVID-19: implications for supply chain management. Front Health Serv Manage. 2020;37:33–38. doi: 10.1097/hap.0000000000000092. PubMed PMID: 32842087.
- Mugo MG. The impact of health insurance enrollment on health outcomes in Kenya. Health Econ Rev. 2023;13:42. doi: 10.1186/s13561-023-00454-7. Epub 20230816. PubMed PMID: 37584819; PubMed Central PMCID: PMC10428604.
- Osei Afriyie D, Kwesiga B, Achungura G, Tediosi F, Fink G. Effects of health insurance on quality of care in low-income countries: a systematic review. Public Health Rev. 2023;44:1605749. doi: 10.3389/phrs.2023.1605749. Epub 20230810. PubMed PMID: 37635905; PubMed Central PMCID: PMC10447888.
- Oyando R, Were V, Willis R, Koros H, Kamano JH, Naanyu V, et al. Examining the responsiveness of the National Health Insurance Fund to people living with hypertension and diabetes in Kenya: a qualitative study. BMJ Open. 2023;13:e069330. doi: 10.1136/bmjopen-2022-069330. Epub 20230704. PubMed PMID: 37407061; PubMed Central PMCID: PMC10335555.
- Onsongo S, Kamotho C, Rinke de Wit TF, Lowrie K. Experiences on the utility and barriers of telemedicine in healthcare delivery in Kenya. Int J Telemed Appl. 2023;2023:1487245. doi: 10.1155/2023/1487245. Epub 20230503. PubMed PMID: 37180825; PubMed Central PMCID: PMC10171985.
- Kim EJ, Moretti ME, Kimathi AM, Chan SY, Wootton R. Use of provider-to-provider telemedicine in Kenya during the COVID-19 pandemic. Front Public Health. 2022;10:1028999. doi: 10.3389/fpubh.2022.1028999. Epub 20221121. PubMed PMID: 36478732; PubMed Central PMCID: PMC9720268.
- Qin R, Dzombak R, Amin R, Mehta K. Reliability of a telemedicine system designed for rural Kenya. J Prim Care Community Health. 2013;4:177–181. doi: 10.1177/2150131912461797. Epub 20121003. PubMed PMID: 23799704.
- Adebayo PB, Jusabani A, Mukhtar M, Zehri AA. The changing trend of teleconsultations during COVID-19 era at a tertiary facility in Tanzania. Pan Afr Med J. 2020;35:125. doi: 10.11604/pamj.supp.2020.35.2.24977. Epub 20200728. PubMed PMID: 33282080; PubMed Central PMCID: PMC7687499.
- Baatiema L, Sanuade OA, Allen LN, Abimbola S, Hategeka C, Koram KA, et al. Health system adaptions to improve care for people living with non-communicable diseases during COVID-19 in low-middle income countries: a scoping review. J Glob Health. 2023;13:06006. doi: 10.7189/iogh.13.06006. Epub 20230303. PubMed PMID: 36862142; PubMed Central PMCID: PMC9980283.