Abstract
Costus igneus has been practiced to alleviate a range of human ailments and the substantial vision was to explore potential bio-active compounds of Costus igneus to better comprehend the underlying mechanism in the management of diabetes. In-silico approach using AutoDock and Desmond, the anti-diabetic efficacy of discovered phytochemicals was evaluated. The best-docked were chosen for simulation studies. The molecular dynamic modelling showed that the complexes of ligands with receptors were stable during a time of 100 ns, which further confirmed and enhanced their significant binding affinity. According to docking scores and simulation studies, these phytocompounds could be targeted to garner efficient preventive medications for diabetes from a biological source using contemporary technology, and further in vivo and in vitro research of these compounds may be encouraged in the search for a potent treatment for the disease.
Abbreviations: DPP-IV: dipeptidyl peptidase-IV; IR: insulin receptor; PPARγ: peroxisome proliferator-activated receptor-γ; IDF: International Diabetes Federation; ILP: insulin-like protein; GLP: glucagon-like peptide-1 receptor; GIP: gastric inhibitory peptide; HBD: hydrogen bond donor; HBA: hydrogen bond acceptor; QSAR: quantitative structure-activity relationship; ADMET: absorption; distribution; metabolism; excretion and toxicology; HIA: human intestinal absorption; BBB: blood–brain barrier.
1. Introduction
Diabetes mellitus is both a chronic and a metabolic condition. Diabetes now affects 422 million people worldwide, up from 108 million in 1980. It is characterized by high blood glucose levels, abnormal lipid metabolism, and enhanced oxidative stress caused by glucose auto-oxidation into free radicals [Citation1]. People are increasingly concerned about their health as a result of the prevalence of fatal diseases, as well as their eating habits, workloads, hypertension, food gaps, and several other everyday activities [Citation2]. The expense of synthetic medicines is increasing day by day as a result of globalization and modern medical science. Furthermore, despite considerable progress in biomedicine, with greater understanding and possibly successful therapeutic techniques to treat many diseases, diabetes therapy remains a major obstacle [Citation3].
A chronic endocrine-metabolic illness called diabetes mellitus causes increased blood glucose levels, which over time can seriously harm the eyes, heart, kidneys, blood vessels, and nerves. The most prevalent type of diabetes is type 2, which commonly impacts adults, and emerges when the body ceases to make enough insulin or becomes resistant to it. Type 2 diabetes has been much more common over the course of the past thirty years in nations of all income levels. Diabetes type 1 is a chronic illness in which the pancreas induces little or no insulin on its own. It was formerly referred to as juvenile diabetes or insulin-dependent diabetes. Access to affordable medical care, particularly insulin, is essential to the survival of people with diabetes [Citation4].
The World Health Organization's (WHO) statistics indicated that the prevalence of diabetes was about 9% in individuals in 2014, and it has been predicted that by 2030, diabetes may rank as the seventh most common cause of death worldwide [Citation5]. Currently, 1.5 million deaths per year are directly related to diabetes, which affects 422 million people globally, the majority of whom reside in nations with low or middle incomes. Over the past few decades, there has been a consistent rise in both the incidence and prevalence of diabetes. Despite this, the WHO states that by 2025, there will be a universally accepted goal to stop the exponential increase in diabetes and obesity. Diabetes statistics demonstrate the disease's increasing financial burden on people, families, and nations worldwide. According to the IDF (International Diabetes Federation) Diabetes Atlas (2021), 10.5% of adults (20–79 years old) have diabetes, with nearly half being unaware that they have it. IDF predictions indicate that by 2045, 1 in 8 individuals, or over 783 million people, will have diabetes, a 46% rise [Citation6].
At this time, type 1 diabetes cannot be prevented. Effective methods for controlling type 2 diabetes, as well as the complications and early death that can arise from all types of diabetes, are available, for example, frequent exercise, a healthy diet, quitting smoking, and maintaining healthy blood pressure and cholesterol levels are policies and practices that promote overall good health for all people, regardless of whether they have diabetes.
Irrespective of the category of diabetes a patient might possess, a series of inexpensive interventions can enhance their overall health. The management of elevated blood pressure and lipids to lower cardiovascular risk and other risks, periodic health screenings for impairment to the kidneys, eyes, and feet to ease prompt intervention, and controlling blood glucose are some of these measures [Citation7].
The current work addresses the mechanism underlying the hypoglycaemic activity of Costus igneus with a focus on molecular targets for type 2 diabetes mellitus.
The unpleasant side effects of numerous synthetic drugs have reignited interest in traditional diabetic treatment approaches. For the management of diabetes, over 400 indigenous species have been discovered, as well as several herbal therapies that are recommended for diabetes and diabetic complications, based on ethnobotanical knowledge. Natural compounds have recently reacquired prominence, but ample research is required to create relevant and potentially favourable bioactive leads that are found in nature that potentially be deployed to oversee diabetes by relying on specified molecular markers [Citation8]. Many secondary metabolites have been implicated in the diabetes-prevention properties of plants. Plant extracts carry a wide array of secondary metabolites that have been exhibited to govern glucose by nourishing or stimulating β-cells, significantly increasing insulin sensitivity, subverting gluconeogenesis, and arousing glycogenesis, among many other mechanisms [Citation9].
The Costaceae family includes Costus igneus as shown in Figure , commonly known as the Spiral Flag. It is found in eastern Brazil (Bahia and Espirito Santo states) and South America [Citation10]. It is a newbie to India and is cultivated as an elegant plant in Kerala [Citation11]. It thrives in a tropical environment. It has simple, alternating, whole, and oblong evergreen leaves that are 4–8 inches long and have parallel venation. Huge, glossy, dark green leaves spiral in a spiral pattern around the stems. It attains a height of around 60 cm, with the longest stems lying down. Captivating orange blossoms with a diameter of 2.5–12.5 cm bloom during the summer. Insulin plants are propagated by cutting their stems. It's a tasty dietary supplement because people used to eat 2–3 leaves twice a day for diabetes treatment, earning it the nickname “insulin plant” [Citation12].
The leaves of Costus igneus are rich in polyphenols, anthraquinones, alkaloids, flavonoids, saponins, and terpenoids. Using plant extracts and naturally occurring polyphenols having anti-diabetic, anti-inflammatory, anti-apoptotic, and anti-cancerous activities may be helpful in controlling diabetes mellitus. With polyphenols, the effects of other metabolic conditions like insulin resistance, hyperglycaemia, hyperlipidemia, obesity, and diabetes mellitus can be reduced [Citation4].
Different doses of the plant extract in streptozotocin-induced diabetic male albino rats showed recovery in all the biochemical parameters such as lipid peroxidation, reduced glutathione, superoxide dismutase, catalase, glucose-6-phosphate dehydrogenase, glutathione reductase, glutathione peroxidase, and glutathione-S transferase; ALT (alanine transaminase), AST (aspartate aminotransferase), and ALP (alkaline phosphatase); creatinine, urea, and uric acid; total cellular protein content in tissues, glycogen content, and serum protein content (albumin and globulin); and inflammatory cytokines [Citation4]. Methanolic leaf extract of Costus igneus at concentrations ranging from 100 to 500 µg/ml was used to find its inhibitory effect on α amylase and α glucosidase. The half-maximal inhibitory concentration (IC 50) value for α amylase and α glucosidase was found to be 273.99 and 298.03 µg/ml which was higher when compared to acarbose [Citation13].
Diabetes mellitus was induced with a single injection of streptozotocin in Swiss mice and was treated for 2 weeks with a single dose of ILP (Insulin-like protein) purified from the Costus igneus extract (14.3 mM/kg). The results indicated that the group treated with ILP did not show a reduction in the pancreatic islets, suggesting the pancreatic function was preserved, differently from the groups of untreated diabetic animals. This analysis indicated that ILP not only presents an insulin mimetic effect but also protected the islets from further damage in diabetic Swiss mice [Citation12]. In RIN5f cell lines that respond to insulin, increasing quantities of ILP were employed to assess the pure protein's hypoglycaemic properties, with human insulin serving as a control. Using the DNSA approach, doses of glucose that weren't already in the extracellular media were given every 30 min for 180 min. They discovered that the levels of glucose in the wells’ supernatant were rapidly decreasing. When compared to insulin, ILP was found to have a better reaction when used to mediate glucose transport. This suggests that ILP has an insulin-like activity and that glucose is taken up by the cells effectively. According to the investigation, ILP induces GLUT-4 to go to the plasma membrane and accumulate IRS-1 (insulin receptor), which makes it easier to absorb and utilize glucose and lowers blood glucose levels [Citation14].
The ethanolic leaf extract of Costus igneus has shown a potent anti-diabetic effect in alloxan-induced diabetic albino rats. The comparison was made between ethanolic extract of Costus igneus extract and a known antidiabetic drug Glibenclamide. An oral glucose tolerance test was performed. Standard drug Glibenclamide lowered the blood glucose level significantly whereas ethanolic extract significantly (P < 0.01) decreased fasting blood serum glucose in the diabetic rats at 1, 2, 3, 5, and 7hrs as compared to initial (0hrs) blood serum glucose levels. Also, orally administered ethanolic extract of Costus igneus significantly increased body weight by decreasing blood glucose levels in diabetic rats [Citation15].
Oxidative stress is well-sighted in the pathogenesis of various diseases like diabetes, neurodegenerative diseases, respiratory tract disorders, and cancer also plays a major role in the process of aging [Citation16,Citation17]. Phytochemical screening of Costus igneus leaves showed the presence of protein, iron, and antioxidant components such as ascorbic acid, α-tocopherol, β-carotene, terpenoids, steroids, and flavonoids. The methanolic extract was found to contain the highest number of phytochemicals such as carbohydrates, triterpenoids, proteins, alkaloids, tannins, saponins, and flavonoids [Citation10,Citation18]. Flavonoids are well-known for anti-diabetic activity and contain polyphenols, with a common structure of diphenyl propane (C6-C3-C6), consisting of two aromatic rings linked through three carbon atoms [Citation19].
Although oral medications for diabetes mellitus are available, individuals are still looking for a lasting solution for insulin induction. Herbal medications are those whose active components are derived from plant materials like leaves, roots, or flowers. However, just because something is “natural” doesn't necessarily imply it's safe for you to consume it. Herbal medicines have an impact on the body in a similar way to conventional drugs, and improper use may have negative effects. Therefore, they ought to be handled with the same caution and respect as traditional medications [Citation7,Citation20]. Screening plants to identify their therapeutic qualities and bioactive components has sparked renewed interest [Citation21]. The WHO's recommendations for evaluating the efficacy of plants in circumstances where we lack safe, modern medications have given a boost to the same [Citation22]. These concepts have sparked a passion for research into natural products with prevention of diabetes capabilities and few negative effects [Citation23]. In streptozotocin-induced diabetic albino rats, Costus igneus aqueous leaves extract demonstrated significant diabetes prevention activity. Plant extracts were shown to reduce blood glucose levels in 15 days when administered orally by gastric intubation with a force-feeding needle [Citation12]. The anti-hyperglycaemic effect of Costus igneus has been supported by numerous non-clinical trials [Citation24]. However, no research has been done on Costus igneus elements to comprehend how they function and how they constrain diabetes.
Bioinformatics attributes cost-effective and expedited work involved in designing experiments, executing them, and implementing laboratory trials. Physiochemical principles and intricate scientific computation techniques are combined in a novel way via molecular docking. Based on the typical interaction between the receptor and ligand/drug molecule, docking aids in medication development. It aids the industry in concentrating on substances having the greatest potential against a specific target [Citation25].
In this investigative work, we explored the phytoconstituents of Costus igneus and for this, an activator of Insulin receptor (IR), Dipeptidyl Peptidase-IV (DPP-IV) inhibitor, and an agonist of Peroxisome proliferator-activated receptor-gamma (PPAR-γ) were chosen as therapeutic molecular targets for diabetes mellitus.
1.2. Insulin receptor
Insulin facilitates glycogen biosynthesis and glucose transport in skeletal muscle while limiting protein catabolism. Insulin enhances lipogenesis and glucose uptake while restricting lipolysis in adipose tissue. It boosts lipogenesis and glycogen synthesis and inhibits fatty acid oxidation and glycogen synthesis in the liver, affecting metabolism indirectly in addition to its direct physiological actions [Citation26–28]. The mechanism behind how the insulin receptor activator works is shown in Figure a.
1.3. Dipeptidyl peptidase-IV
DPP-IV is vital for glucose homeostasis. It is required for incretin (Glucagon-like peptide-1 (GLP-1) and Gastric inhibitory polypeptide (GIP)) breakdown as incretins allow the body to produce more insulin when it desired and minimize the level of glucose the liver produces and its inhibitors act by boosting incretin levels, which elevate insulin secretion, impede glucagon release, lower blood sugar levels, and slow stomach emptying [Citation28,Citation29]. Figure b depicts the process through which the DPP-IV inhibitor operates.
1.4. Peroxisome proliferator-activated receptor-gamma
When the agonist is bound, PPARs construct heterodimers involving retinoid X receptors and engage with cofactors to accelerate the rate of gene transcription. PPARs play a key metabolic role as regulators of lipid metabolism and lipid sensors. Thyroid and steroid receptors are members of the PPAR superfamily of nuclear receptors. PPAR-γ is found in a variety of tissues (e.g. fat, skeletal muscle, and liver). Its stimulation increases the transcription of genes encoding glucose and lipid metabolism, adipocyte development, and insulin signalling [Citation28,Citation30]. Figure c shows how the PPAR-γ agonist works.
2. Materials and methods
2.1. Building a phytoconstituents library
Costus igneus, a member of the Costaceae family, has become increasingly appealing for advanced research due to its antihyperglycaemic activity, ingestion as a food source, and application in traditional medicine. The server DLAD4U (Disease List Automatically Derives For You) (dlad4u.org) was utilized for disease retrieval and prioritization. Costus igneus possess diabetes prevention properties, according to text mining. There have been several non-clinical investigations that show Costus igneus has diabetes management properties [31,12, Citation32,Citation33]. However, due to the multifaceted character of this condition, it is impossible to rely on a single experimental paradigm or treatment to overcome it. IR, DPP-IV, and PPAR-γ are instances of experimental models representing protein receptors involved in glucose regulation throughout the body. As a potential consequence of reviewing the scientific literature as well as the PubChem database (pubchem.ncbi.nlm.nih.gov) for diabetes management potency from Costus igneus, a bank of phytochemical compounds in SDF format was constructed [Citation34].
2.2. Predicting drug-likeness
Based on Lipinski's approach, the SwissADME tool was used to compute drug-likeness property. As per Lipinski's statistic [Citation35], “drug-like” entities must have a molecular weight less than 500, a LogP (lipophilicity) not greater than 4.15, no more than five hydrogen bond donors (HBD), and no more than ten hydrogen bond acceptors (HBA). Lipinski's rule has been employed to deduce putative ligands [Citation36,Citation37].
2.3. ADME and toxicity analysis
Quantitative structure–activity relationships (QSAR) analyses were carried out using the Molinspiration server (molinspiration.com) to determine the efficacy of screened phytochemicals as drug targets. The ADMETlab 2.0 and protox-II prediction algorithms were used to estimate the toxicity of the phytochemicals investigated. Human intestinal absorption (HIA), acute toxicity rate (LD50), carcinogen toxicity, and Blood–brain barrier (BBB) penetration have all been speculated utilizing the ADMETlab 2.0 and protox-II algorithm [Citation38].
2.4. Retrieval of 3D structures of receptor proteins
To find new diabetes preventive compounds, molecular targets were explored through PharmGkb (pharmgkb.org), DrugBank (go.drugbank.com), and the literature, which yielded various molecular targets. The protein targets’ X-ray crystallographic structures were retrieved via RCSB's Protein Data Bank (rcsb.org) and served as the receptor in the docking analysis [Citation36,Citation37,Citation39].
2.5. Optimization of receptor proteins
The Swiss PDB viewer tool was employed to refine receptor protein for the docking study. The receptor proteins were constructed and enhanced by omitting ligands and molecules of water. The receptor proteins were also energy minimized [Citation40].
2.6. Construction of ligands
The crystal structures of Costus igneus phytoconstituents and reference standard of selected therapeutic targets were extracted from PubChem as an SDF file, transcribed to PDB format, and energy minimized using Avogadro software (avogadro.cc/) [Citation41]. Metformin was employed as an IR reference ligand, Sitagliptin as a DPP-IV reference ligand, and Ciglitazone as a PPAR-γ reference ligand.
2.7. Molecular docking
Molecular docking research aims at finding the adequate agonists of IR and PPAR-γ, as well as repressors of DPP-IV. To carry out virtual screening, BiteNet–Skoltech iMolecule server was used to locate molecular targets’ active binding domains [Citation42]. Following that, the AutoDock software (Autodock tools1.5.7) was used to accomplish molecular docking. The refinement of the protein and ligand was achieved by the incorporation of polar hydrogen bonds, Kollman charges as well as Gasteiger charges. Around the protein's binding pocket, a grid box with dimensions of 60 × 60 × 60 and spacing of 0.375 was created. The search parameter was assigned to the genetic algorithm. The output was managed by Lamarckian GA run with the docking log file being retrieved for evaluation of binding energy. The optimal conformations were taken into account for binding energy (estimated in kcal/mol) at the culmination of the docking process. For further estimation, the complexes with minimal energy were used. Ligplot+ v.2.2 software (LigPlus/download.html) was used to quantify the complex's 2D associations, revealing hydrogen bonds and their bond lengths, whereas the UCSF Chimera tool (ucsf.edu/chimera) was used to depict the complex in 3D [Citation43,Citation44].
2.8. Molecular dynamics simulation
After docking, the top-scoring complex was attributed to a simulation modelling using the Desmond module of the Schrödinger suite 2022 [Citation45]. The software has advanced algorithms, and all default settings were applied to produce swift and reliable results. Utilizing an orthorhombic solvent box configured for a 10 Å buffer area, the complexes were solvated using OPLS3 as the force field and TIP4P as solvent model. The ideal potentials for liquid simulations-AA (OPLS-AA) 2005 force field were employed to align all of the atoms. In order to confine the moment of the atoms possessing covalent bonds, NVT was employed as the ensemble class using the SHAKE/RATTLE algorithm. The simulation commenced at 300 K and 1 bar of pressure and ran for 100 ns. The temperature was controlled by the Nose–Hoover chain approach, and pressure conditions were managed by the Martyna-Tobias Klein scheme's isotropic coupling style [Citation46]. While electrostatic interactions were handled using Particle Mesh Ewald, short-range interactions were given a cut-off of 9 Å. Prior to the molecular dynamics simulation, the models were relaxed and no positional restraints were used. The simulation's parameters were all combined using the RESPA integrator. To explore the interaction's dynamic nature and component stability, a trajectory lasting 100 ns was set to be displayed over 1000 frames [Citation47,Citation48].
3. Results and discussion
3.1. Constructing a phytoconstituents library
Through exploring academic data and PubChem, a repository of twenty-one phytoconstituents of Costus igneus was devised. The PubChem database has been used to obtain crystal structures of phytoconstituents [Citation10,Citation49,Citation50]. Table summarizes bioactive constituents reported for Costus igneus.
Table 1. Phytoconstituents’ dossier of physiochemical characteristics.
3.2. Predicting drug-likeness
Early preclinical research is aided by drug-likeness filters, which help to avoid expensive pre-clinical and clinical setbacks [Citation25,Citation51,Citation52]. Moreover, Lipinski’s rule of five has been employed to determine the drug-likeness of compounds under study [Citation53,Citation54,Citation55] as shown in Table .
It has been determined that 19 phytoconstituents have a drug-like molecular makeup and fall within the range where the Lipinski criterion is not violated. Their log P values are between the ranges of 0.79–4.54. Except for Epigallocatechin gallate and β-carotene, which revealed two breaches in terms of molecular weight, hydrogen bond acceptor, and hydrogen bond donor, all other compounds fall within the acceptable range. While β-carotene displayed violations in terms of molecular weight and the lop P value, epigallocatechin gallate displayed violations in terms of the quantity of hydrogen bond donors and acceptors.
3.3. ADMET results
The Molinspiration, ADMETlab2.0, and Protox-II servers evaluate pharmacokinetic parameters based on four criteria: absorption, distribution, metabolism, and excretion. Table displays the findings of the analysis. BBB penetration, HIA, carcinogen toxicity, and rat LD50 were all predicted using the methodology. The ADMET profiles of the phytoconstituents were within permissible bounds, indicating their efficacy as potential therapeutic candidates [Citation56].
All compounds showed good gastrointestinal absorption and presented moderate to high solubility. The high solubility of phytoconstituents is due to hydroxy groups that participate in hydrogen bonding with water molecules. The blood–brain barrier is a semipermeable border present within endothelial cells. It prevents the passage of pathogens and large or hydrophilic molecules into the extracellular fluid of the CNS (central nervous system) passage of small polar and hydrophobic molecules [Citation57]. BBB (blood–brain barrier) is the layer of endothelial cells that prevents the entry or exit of solutes from the bloodstream to the extracellular space. It may be easily evaluated by considering the compound’s concentration in the brain’s concentration in blood [Citation57]. In the central nervous system, active drugs and high penetrations of the blood–brain barrier are necessary. In contrast, in non-central nervous system active drugs, low penetration reduces the CNS side effects. The blood–brain barrier permeants are mentioned in Table .
ADMET properties of a useful drug-like compound accepted the following parameters: such as (i) non-toxic (ii) non-carcinogenic (iii) it must be absorbed in the human intestine with less penetration in the Blood–brain barrier [Citation58]. All the selected phytoconstituents are predicted to be not readily biodegradable but showed no carcinogenicity. But since these are purely computational details, further in-silico comparative analyses should be performed to rule out all the in-silico possibilities for metabolism.
3.4. Protein targets
IR (PDB ID–1IR3), DPP-IV (PDB ID–2OQV) as well as PPAR-γ (PDB ID–4CI5) were the three three-dimensional crystal structures retrieved from Protein Data Bank (3,27) and used as a target in the screening of selected phytoconstituents. In preparing the receptor co-crystallized ligands, solvent elements and water molecules were excluded, Polar hydrogen atoms were devised, nonpolar hydrogen atoms were integrated, and Kolmann charges were incorporated using the Autodock tool. PDB to PDBQT conversion was done on the protein receptor and Gasteiger charges were added for a protein using UCSF chimera [Citation59]. The grid size was set to 60 × 60 × 60 x.y.z points having a grid spacing of 0.375 Å was designated. All other receptor preparation options were left at their normal defaults [Citation27]. The NCBI conserved domain (https://www.ncbi.nlm.nih.gov/ Structure/cdd/wrpsb.cgi) database was used to show the conserved residues of the main protease structure as shown in Figure .
3.5. Insightful analysis of molecular docking
The docking process was carried out using AutoDock. The docking scores are graphically represented in Figure and the binding affinities of ligands are represented as kcal/mol in Table . Docking findings for 3 molecular targets IR, DPP-IV, and PPAR-γ, speculated that sixteen out of twenty-one presumptive phytoconstituents displayed larger binding affinities and modalities of binding than the reference molecules. The fluctuations in binding energy are thus more than –4.0 kcal/mol. The lower binding affinities with higher interactions were due to hydroxyl group Hydrogen bonds having an essential role in the stability and macromolecular recognition. Hydrogen bonds also play a crucial role in several biological processes; hence, hydrogen bonds have a primary role in determining pharmacological receptors [Citation60]. The presence of bulky methyl groups in Stigmasterol and β-sitosterol reduces the interactions and increases the binding energy.
Figure 4. Graphical depiction of the binding energy of phytoconstituents against Diabetes mellitus targeted therapies highlighting foremost docked compounds similar to their reference counterpart (a) IR (b) DPP-IV (c) PPAR-γ.
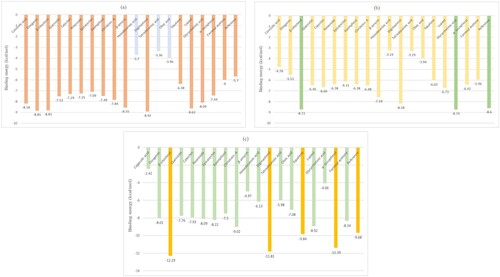
Table 2. The binding energy of phytoconstituents selected for docking towards diabetes mellitus targeted therapies.
Figure depicts a bar graph of several Costus igneus phytochemicals about therapeutic targets for Diabetes mellitus. The best binding energy for IR was shown to be Stigmasterol (−8.92 kcal/mol); Glycyrrhetnic acid (−8.74 kcal/mol) has the best binding energy for DPP-IV, while the best binding energy for PPAR-γ was discovered to be β-sitosterol (−12.29 kcal/mol). These phytochemicals’ binding energies with IR, DPP-IV, and PPAR-γ were ranging from –8.92 to –3.36 kcal/mol, –8.74 to –3.24 kcal/mol, and –12.29 to –2.42 kcal/mol, respectively as shown in Table and Figure .
Ligplot+ was used to study protein–ligand interactions for a given PDB file encrypting the docking [Citation41]. It helps in examining hydrogen bond amplitude, hydrogen-bonded interaction, as well as hydrophobic affinities with their respective receptors. The IR-Stigmasterol complex succeeded in establishing a hydrogen bond with amino acids GLU1047 and Asp1150, meanwhile, the DPP-IV-Glycyrrhetnic acid complex built a single hydrogen bond with the residue TYR662. In the aspect of PPAR-γ, the efficient phytochemical β-sitosterol displayed two hydrogen bond coupling with amino acid LYS at 263 and 265 positions. Figure illustrates the 3D and 2D illustrations of superlative docked complexes and the spectrum of hydrogen bond amplitude in diverse phytoconstituents along with varied residues. Residues forming hydrophobic affinity with phytoconstituents are represented by red sparking arcs, while the hydrogen bonds along with constraints were portrayed by dashed green lines. Protein residues in corresponding 3D positions are indicated by red circles and ellipses. The Ligplot+ program self-generated schematic 2D representations of the interfaces of protein–ligand complexes from standard PDB file input. The output was in the form of an informative representation of the intermolecular interactions and their strengths, including hydrogen bonds, hydrophobic contacts, and atom accessibilities [Citation61]. The type and amount of bonding exhibited by the phytocompounds in the protein’s active site showed their affinity toward the drug. The number and position of hydroxyl groups are essential in determining the interacting potential. In addition to conventional hydrogen bonds, other types of interactions like van der Waals, pi-sigma, pi-sulfur, pi-alkyl, and carbon–hydrogen bonds are also important in determining the potentiality of phytocompounds [Citation62].
3.6. 2d Depiction of the receptor–ligand interaction using ligplot+ algorithm
2D interactions of references and pre-eminent screened bioactive components were examined using the interacting motif of hydrogen bonding ties with the standards and hydrophobic associations with diverse residues for all three molecular targets as shown in Figure . For IR, the best-fitting ligand (Stigmasterol) in purple has a shared residue binding with the GLU1047 reference in green. The best-fitting ligand (Glycyrrhetinic acid) with DPP-IV, indicated in purple, has a shared residue binding with the reference, shown in yellow, at TYR662 and the best-fitting ligand (β-sitosterol) with PPAR-γ displayed in purple shares common residue binding with the reference shown in light blue at two residues, LYS263 and LYS265. Thus, best-docked ligands of respective receptors were discovered to be prospective diabetes management candidates based on molecular docking and ligplot+ interactions. For fit quality, all the chosen compounds have values within the stipulated range. We envisage that a fixed geometry ascertained due to cyclization in peptides could help it bind to receptors more effectively.
3.7. Molecular dynamics simulation
Desmond software was used to run molecular dynamics simulations on each receptor's top-performing docked ligand for 100 ns. Stigmasterol was chosen to simulate with IR, Glycyrrhetinic acid chosen with DPP-IV, while β-sitosterol chosen for PPAR-γ.
3.8. Structural deviation and compactness
Each simulation's receptor–ligand complex variations and stability were examined, and the final trajectory was created using the baseline root mean square deviation. It was found that throughout the simulation, the overall protein–ligand complex was steady. For IR and PPAR-γ, the trajectory was stable throughout the timeframe. However, for DPP-IV, the first 10 nanoseconds show some variations in the complex, but the following 90 nanoseconds complex was stable. Throughout the trajectory, little crust and troughs in Root Mean Square Deviation (RMSD) graph may be observed, but they are caused by the system's internal vibrations. All three IR, DPP-IV, and PPAR-y complexes exhibited average RMSD of 3.05 , 8.81 , and 4.77 Å respectively and these complexes exhibited 5–7 rotatable bonds. To improve the overall interactions, all three complexes may substantially deviate from their bound conformations during MD simulation. High RMSD with respect to the initial bound conformation may result from the ligands having a lot of rotatable bonds. Despite having significant RMSD values, these complexes’ local and overall structures looked to be fairly stable, as shown by analysis of their trajectories. Although the global shape was maintained and the MD simulations seemed to have converged, the visual trajectory analysis showed that the local shape surrounding the detected locations was quite flexible. The resulting Root Mean Square Fluctuation (RMSF) plot aided in determining the stability of the protein's residues. Beta-sheet and alpha-helix secondary structure packing residues confer less fluctuation and have lower RMSF, whereas terminal and loops residues exhibit larger RMSF. Figure reveals that the aforementioned phenomena are shared by the residues of all three complexes. However, it was found that the majority of the residues involved in DPP-IV binding to Glycyrrhetinic acid had greater RMSF values, indicating turbulence at the binding void. The entire complexes had an RMSF value ranges between 0.6 Å and 6.4 Å. A greater RMSF value suggested that the residue was more flexible than the average position. Polar amino acids with the most fluctuation are serine, glutamine, and asparagine. In order to ease strain on the protein, Figure shows that some bonds in each of the three ligands experience moderate rotations or torsions. For instance, Figure displays dial plots that illustrate how rotatable bonds in complexes changed their conformation during the duration of the simulation. On the dial plots, the corresponding colour designates each rotatable bond. Despite being a complex molecule, it is noticeable from the dial plots that it undergoes rotation to allow for protein adjustment. Given the significant variation of residues in the protein termini, non-structured areas, and loops, and in the ligands’root mean square fluctuations and torsion profile, DPP-IV has a greater RMSD than the other two [Citation63,Citation64].
3.9. Secondary structural analysis and interaction dynamics
Interaction analysis was executed using Desmond. The number and position of interacting bonds are essential in determining the superlative potential of that compound. There was a total of 20 ligand interactions with protein residues, ranging from LEU1002 to PRO1172 of the A chain and TYR10 of the B chain, when the interactions of the stigmasterol-IR complex were analysed. Residues created four different kinds of interactions: hydrogen bonds, hydrophobic attachments, ionic bonds, and water bridges. It was found that the ligand formed polar and hydrophobic dynamics with the active domains to achieve stability. Figure illustrates the graphical features of the two-dimensional interactions between ligand atoms and protein residues. It displayed the precise percentage of associations between the protein and ligand. 25% of the simulation time was spent with residues interacting with the ligand via hydrophobic interactions; other residues, such as SER1006 and TYR10, briefly established H-bonds.
A total of 37 ligand connections with protein residues were found by examining the interactions of the glycyrrhetinic acid-DPP-IV complex, specifically with LYS721 and ASP725 of the A chain of protein and from ASN119 to ALA747 of B chain. The actual frequency of associations between protein and its ligand is displayed in Figure . The ligand was held in the binding pocket by interactions with residues LYS122 and ASP739 that involved two types of bonds, notably H-bonds and water bridges, during the whole simulation. GLN123, TRP124, GLU191, TYR238, LYS250, ASP737, GLU738 and SER744 were other residues that briefly formed H-bonds.
As can be shown in Figure , 29 ligand connections with protein residues from SER225 to LEU452 were discovered by examining the interactions of the β-sitosterol–PPAR–γ complex. Three types of bonding, including H-bonds, ionic bonds, and water bridges, were used by residues GLU295 and SER225 to interact with the ligand for 30% and 25% of the simulation duration, respectively. Other residues, such as PRO227, LEU228, ALA292, and GLU343 formed H-bonds, although only briefly.
The greater stability of the ligand in the binding void can be accounted for by other forms of interactions that were also evident. The top panel displayed all of the contacts that all three complexes made during the course of the trajectory, while the bottom panel displayed particular residue-level interactions with the ligands during each trajectory frame (Figure (a)). Understanding the protein packing and folding features of the protein with various ligands can be done by computing and evaluating secondary structural assessment. The frequency of secondary structures in protein–ligand complexes is shown in Table as a percentage.
Figure 9. Protein–Ligand contact histogram and timeline representation (a) Stigmasterol–IR complex (b) Glycyrrhetinic acid–DPP-IV (c) β-sitosterol–PPAR-γ.
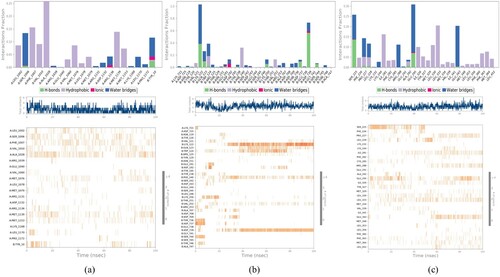
Table 3. Protein secondary structure elements (SSE) interpretation.
Comparing the three different mechanisms of diabetes mellitus’ receptors, it was hypothesized that stigmasterol, glycyrrhetinic acid, and β-sitosterol against the aforementioned reference ligand exhibit the best activity in terms of binding interaction with the insulin receptor, DPP-IV and PPAR-γ pathway respectively. However, among these three, glycyrrhetinic acid is the most effective ligand for employing the DPP-IV pathway against diabetes mellitus as it has the best binding interaction in terms of bond number, binding energy, and stability interactions.
In this context, several analogs of Costus igneus should be designed and screened in silico for the above-mentioned biological activities prior to in vitro studies. Thus, the in-silico experiments gave a clear insight into its potential as an activator of Insulin receptor (IR), Dipeptidyl Peptidase-IV (DPP-IV) inhibitor, and an agonist of Peroxisome proliferator-activated receptor-gamma (PPAR-γ)
4. Conclusion
We examined Costus igneus compounds for diabetes preventive properties in the literature and employed molecular docking against diabetes mellitus-targeted therapies in this work. Eventually, twenty-one phytochemicals were screened for drug-likeness and ADMET prognostication, with the observations demonstrating that just two compounds did not follow the criterion, the rest other follows. For simulation dynamics, Stigmasterol with IR, Glycyrrhetinic acid with DPP-IV, and β-sitosterol with PPAR-γ were chosen since they have the highest docking scores of all the molecules. The proposed binding sites could be used in silico rational design approaches for ligands aimed at diagnosis or therapy of diabetes mellitus. Thus, the above-listed compounds are promising diabetes preventive compounds, as per molecular docking analysis. These bioactive compounds have the greatest possible binding affinity for all of the hypoglycaemic therapeutic targets. Therefore, our observations conceptually facilitate Costus igneus as preventive diabetes properties, which are concurrent with both the clinical as well as non-clinical utilization of Costus igneus in diabetic sufferers. Hence, plant-derived natural bioactive components are very good alternatives to minimize the prevalence of diabetes-induced hepatorenal dysfunctions and other associated comorbidities. Nonetheless, further studies are required to isolate particular bioactive compounds and evaluation of its hepatorenal protective mechanisms at the cellular and molecular levels.
Acknowledgements
The authors thankfully acknowledge the facilities of Maharshi Dayanand University, Rohtak, Haryana, India.
Disclosure statement
No potential conflict of interest was reported by the author(s).
References
- Biswas K, Shaw S, Mishra S, et al. Nanotechnology in Biology and Medicine. 1st ed. 2019. p. 185–192. doi: 10.1201/9780429259333
- Sulakshana G., A.S.R.-W.J.P. Res, Undefined 2015. In vitro evaluation of antifungal activity in three different species of Costus, Wjpr.S3.Ap-South-1.Amazonaws.Com. 4 (2015). https://wjpr.s3.ap-south-1.amazonaws.com/article_issue/1441012271.pdf.
- Sharma P, Joshi T, Joshi T, et al. In silico screening of potential antidiabetic phytochemicals from Phyllanthus emblica against therapeutic targets of type 2 diabetes. Journal of Ethnopharmacology. 2020;248:112268. doi: 10.1016/j.jep.2019.112268
- Hajam YA, Kumar R, Reshi MS, … Ishtikhar M. Administration of Costus igneus Nak leaf extract improves diabetic-induced impairment in hepatorenal functions in male albino rats. J King Saud Univ Sci. 2022;34(4):101911. doi:10.1016/j.jksus.2022.101911
- Msopa E, Mwanakasale V. Identification of risk factors of diabetes mellitus in bank employees of selected banks in Ndola town. Diabetes & Metab Syndr: Clin Res Rev. 2019;13(2):1497–1504. doi:10.1016/j.dsx.2018.11.062
- https://www.who.int/health-topics/diabetes#tab=tab_1
- Srivastava S, He F, Huang Y, et al. A brief review on medicinal plants-at-arms against COVID-19. Interdiscip Perspect Infect Dis. 2023;2023:16.
- Ahmed D, Khan MI, Kaithwas G, et al. Molecular docking analysis and antidiabetic activity of Rifabutin against STZ-NA induced diabetes in albino wistar rats. Beni-Suef Univ J Basic Appl Sci. 2017;6:269–284. doi:10.1016/J.BJBAS.2017.04.010
- N. Gupta, T. Gudipati, G.P.-I.J.C.M.A. Sci, undefined 2018, Plant secondary metabolites of pharmacological significance in reference to diabetes mellitus: an update, Researchgate.Net. (n.d.). https://www.researchgate.net/profile/Neha-Gupta-42/publication/325306464_Plant_Secondary_Metabolites_of_Pharmacological_Significance_in_Reference_to_Diabetes_Mellitus_An_Update/links/5ba1da7e45851574f7d58d52/Plant-Secondary-Metabolites-of-Pharmacological-Significance-in-Reference-to-Diabetes-Mellitus-An-Update.pdf (accessed June 2, 2022).
- Hegde P, Rao H, Rao P. A review on Insulin plant (Costus igneus Nak). Pharmacogn Rev. 2014;8:67. doi:10.4103/0973-7847.125536
- M.S. Deogade, A. Wanjari, S.C. Lohakare, Pharmacognostical and Phytochemical study of NE Br leaf Costus igneus Original Article, (n.d.). doi:10.1007/978-3-540
- Joshi BN, Munot H, Hardikar M, et al. Orally active hypoglycemic protein from Costus igneus N. E. Br.: an in vitro and in vivo study. Biochem. Biophys. Res. Commun. 2013;436:278–282. doi:10.1016/J.BBRC.2013.05.093
- Suchitra MR, Srinithya N, Sivakumar R, et al. Inhibitory effect of Costus igneus Linn (Insulin Leaf) on alpha amylase, alpha glucosidase activity to facilitate antihyperglycemic effect in Type 2 Diabetes Mellitus. J Glob Trends Pharm Sci. 2021;12:9782–9786. https://www.jgtps.com/
- Costa IS, Medeiros AF, Piuvezam G, et al. Insulin-like proteins in plant sources: a systematic review. Diabetes Metab Syndr Obes. 2020;13:3421–3431. doi: 10.2147/DMSO.S256883
- Bhat V, Asuti N, Kamat A. M.S.-J. of P., undefined 2010. Antidiabetic activity of insulin plant (Costus igneus) leaf extract in diabetic rats, Cabdirect.Org. (n.d.). https://www.cabdirect.org/cabdirect/abstract/20103155496 (accessed June 2, 2022).
- Sadrzadeh SH, Nanji AA, Meydani M. Effect of chronic ethanol feeding on plasma and liver α-and γ-tocopherol levels in normal and vitamin E-deficient rats: relationship to lipid peroxidation. Biochem Pharmacol. 1994;47(11):2005–2010. doi:10.1016/0006-2952(94)90075-2
- Anderson D, Phillips BJ, Yu TW, et al. Effects of vitamin C supplementation in human volunteers with a range of cholesterol levels on biomarkers of oxygen radical-generated damage. Pure Appl Chem. 2000;72(6):973–983. doi:10.1351/pac200072060973
- F. Mathew, B.V.-I.J.P.S.R. Res, undefined 2019, A review on medicinal exploration of Costus igneus: the insulin plant, Researchgate.Net. (n.d.). https://www.researchgate.net/profile/Arvind-Singh-21/post/Insulin_plant_Costus_igneus_Does_it_really_work_against_diabetes_How_it_works/attachment/5d24b4ac3843b0b9825a0193/AS%3A778778351509504%401562686636104/download/1.pdf
- Reddy Peasari J, sri Motamarry S, Varma KS, et al. Chromatographic analysis of phytochemicals in Costus igneus and computational studies of flavonoids. Inform Med Unlocked. 2018;13:34–40. doi:10.1016/j.imu.2018.10.004
- Arul B, Kothai R, Josephine A, et al. Hypoglycemic activity of Casearia esculenta roxb. in normal and diabetic albino rats. Orig Artic Iran J Pharm Res. 2006;1:47–51. https://www.sid.ir/en/Journal/ViewPaper.aspx?ID=79506 [accessed June 2, 2022].
- Chattopadhyay RR. A comparative evaluation of some blood sugar lowering agents of plant origin. J Ethnopharmacol. 1999;67:367–372. doi:..Rao, M.M. V, Hariprasad, T.P.N., 2021. In silico analysis of a potential antidiabetic phytochemical erythrin against therapeutic targets of diabetes. Silico Pharmacol. 9, 5. doi:10.1007/S40203-020-00065-8
- Bisht S. S.S.-J. of P.S. and Research, undefined 2010. Anti-hyperglycemic and antidyslipidemic potential of Azadirachta indica leaf extract in STZ-induced diabetes mellitus, Citeseer. (n.d.). http://citeseerx.ist.psu.edu/viewdoc/download?doi=10.1.1.193.7268&rep=rep1&type=pdf (accessed June 2, 2022).
- Ajiboye BO, Oloyede HOB, Salawu MO. Antihyperglycemic and antidyslipidemic activity of Musa paradisiaca-based diet in alloxan-induced diabetic rats. Food Sci Nutr. 2018;6:137. doi:10.1002/FSN3.538
- Arif R, Ahmad S, Mustafa G, et al. Molecular docking and simulation studies of antidiabetic agents devised from hypoglycemic polypeptide-P of Momordica charantia. Biomed Res Int. 2021;2021:1–15. doi: 10.1155/2021/5561129
- Chevalier S, Burgess SC, Malloy CR, et al. The greater contribution of gluconeogenesis to glucose production in obesity is related to increased whole-body protein catabolism. Diabetes. 2006;55:675–681. doi:10.2337/DIABETES.55.03.06.DB05-1117
- Perry RJ, Camporez JPG, Kursawe R, et al. Hepatic acetyl CoA links adipose tissue inflammation to hepatic insulin resistance and type 2 diabetes. Cell. 2015;160:745–758. doi:10.1016/J.CELL.2015.01.012
- Chen C, Zhou S, Meng Q. A molecular docking study of Rhizoma Atractylodis and Rhizoma Atractylodis Macrocephalae herbal pair with respect to type 2 diabetes mellitus. J Tradit Chinese Med Sci. 2018;5:185–198. doi:10.1016/J.JTCMS.2018.05.004
- Kasina SVSK, Baradhi KM. Dipeptidyl Peptidase IV (DPP IV) Inhibitors, (2022) 1–5. https://www.ncbi.nlm.nih.gov/books/NBK542331/ [accessed June 2, 2022].
- E.D. Dixon, A.D. Nardo, T. Claudel, M. Trauner, H. Popper, The role of lipid sensing nuclear receptors (PPARs and LXR) and metabolic lipases in obesity, diabetes and NAFLD, Mdpi.Com. (2021). doi:10.3390/genes12050645
- Hardikar MR, Varma ME, Kulkarni AA, et al. Elucidation of hypoglycemic action and toxicity studies of insulin-like protein from Costus igneus. Phytochemistry. 2016;124:99–107. doi:10.1016/J.PHYTOCHEM.2016.02.001
- Suchitra MR, Srinithya N, Sivakumar R, et al. Inhibitory effect of Costus igneus Linn (Insulin Leaf) on alpha amylase, alpha glucosidase activity to facilitate antihyperglycemic effect in Type 2 diabetes mellitus. J Glob Trends Pharm Sci. 2021;12:9782–9786. https://www.jgtps.com/.
- Kim S, Chen J, Cheng T, et al. PubChem in 2021: new data content and improved web interfaces. Nucleic Acids Res. 2021;49:D1388–D1395. doi:10.1093/NAR/GKAA971
- Lipinski CA, Discovery M, Lombardo F, et al. Experimental and computational approaches to estimate solubility and permeability in drug discovery and development settings. Elsevier. 1997;23:3–25. https://www.sciencedirect.com/science/article/pii/S0169409X96004231.
- Whirl-Carrillo M, McDonagh EM, Hebert JM, et al. Pharmacogenomics knowledge for personalized medicine. Clin Pharmacol Ther. 2012;92:414–417. doi:10.1038/CLPT.2012.96
- Wishart DS, Feunang YD, Guo AC, et al. DrugBank 5.0: a major update to the DrugBank database for 2018. Nucleic Acids Res. 2018;46:D1074–D1082. doi:10.1093/NAR/GKX1037
- Banerjee P, Eckert AO, Schrey AK, et al. ProTox-II: a webserver for the prediction of toxicity of chemicals. Nucleic Acids Res. 2018;46:W257–W263. doi:10.1093/NAR/GKY318
- Berman HM, Westbrook J, Feng Z, et al. The Protein Data Bank. Nucleic Acids Res. 2000;28:235–242. doi:10.1093/NAR/28.1.235
- Guex N, Peitsch MC. SWISS-MODEL and the Swiss-Pdb Viewer: an environment for comparative protein modeling. Electrophoresis. 1997;18:2714–2723. doi:10.1002/ELPS.1150181505
- Hanwell MD, Curtis DE, Lonie DC, et al. Avogadro: an advanced semantic chemical editor, visualization, and analysis platform. J. Cheminform. 2012;4:1–17. doi:10.1186/1758-2946-4-17/FIGURES/14
- Kozlovskii I, Popov P. Spatiotemporal identification of druggable binding sites using deep learning. Commun Biol. 2020;31:1–12. doi:10.1038/s42003-020-01350-0
- Wallace AC, Laskowski RA, Thornton JM. LIGPLOT: a program to generate schematic diagrams of protein-ligand interactions. Protein Eng Des Sel. 1995;8:127–134. doi:10.1093/PROTEIN/8.2.127
- Pettersen EF, Goddard TD, Huang CC, et al. UCSF Chimera—a visualization system for exploratory research and analysis. J Comput Chem. 2004;25:1605–1612. doi:10.1002/JCC.20084
- Bowers KJ, Chow E, Xu H., R.O.D.-P. of the, undefined 2006. n.d Scalable algorithms for molecular dynamics simulations on commodity clusters, Dl.Acm.Org. https://dl.acm.org/doi/abs/10.11451188455.1188544.
- Martyna GJ, Tobias DJ. M.L.K.-T.J. of chemical physics, undefined 1994, constant pressure molecular dynamics algorithms. Aip Scitation Org. 1994;101:44130. doi:10.1063/1.467468
- Garg S, Anand A, Lamba Y, et al. Molecular docking analysis of selected phytochemicals against SARS-CoV-2 Mpro receptor. Vegetos. 2020;33:766–781. doi:10.1007/S42535-020-00162-1/TABLES/3
- Imran M, Iqbal S, Hussain A, et al. In silico screening, SAR and kinetic studies of naturally occurring flavonoids against SARS CoV-2 main protease. Arab J Chem. 2022;15:103473. doi:10.1016/J.ARABJC.2021.103473
- Kumar Vishwas D. A remedial anti-diabetic insulin plant. Int Res J Mod Eng Technol Sci. 1475;05(05):2582–5208.
- Shinde S, Surwade S, Sharma R. Costus ignus: insulin plant and it’s preparations as remedial approach for diabetes mellitus. Artic Int J Pharm Sci Res. 2022;13:1551. doi:10.13040/IJPSR.0975-8232.13(4).1551-58
- reddy Peasari J, sri Motamarry S, Varma KS, et al. Chromatographic analysis of phytochemicals in Costus igneus and computational studies of flavonoids. Informatics Med Unlocked. 2018;13:34–40. doi:10.1016/J.IMU.2018.10.004
- Khan M, Patujo J, Mushtaq I, et al. Anti-diabetic potential, crystal structure, molecular docking, DFT, and optical-electrochemical studies of new dimethyl and diethyl carbamoyl-N, N′-disubstituted based thioureas. J Mol Struct. 2022;1253:132207. doi:10.1016/J.MOLSTRUC.2021.132207
- Jaradat N, Khasati A, Hawi M, et al. Antidiabetic, antioxidant, and anti-obesity effects of phenylthio-ethyl benzoate derivatives, and molecular docking study regarding α-amylase enzyme. Sci Reports. 2022;121. 12(2022):1–9. doi:10.1038/s41598-022-07188-2
- Daina A, Michielin O, Zoete V. SwissADME: a free web tool to evaluate pharmacokinetics, drug-likeness and medicinal chemistry friendliness of small molecules. Sci Rep. 2017;7:17. doi: 10.1038/SREP42717
- Lipinski CA, Lombardo F, Dominy BW, et al. Experimental and computational approaches to estimate solubility and permeability in drug discovery and development settings. Adv Drug Deliv Rev. 1997;23:3–25. doi:10.1016/S0169-409X(96)00423-1
- Lipinski C. Drug discovery today. Technol. 2004a;1(4):337–341.
- Lipinski CA. Lead-and drug-like compounds: the rule-of-five revolution. Drug Discov Today: Technol. 2004b;1:337–341. doi:10.1016/j.ddtec.2004.11.007
- Marondedze E, Govender K. Computational investigation of the binding characteristics of β-amyloid fibrils. Biophysical Chemistry. 2019;256:106281. doi: 10.1016/j.bpc.2019.106281
- Obermeier B, Verma A, Ransohoff RM. The blood–brain barrier. Handbook of clinical neurology. 1st ed. Vol. 133, Elsevier; 2016. Chapter 3.
- Kim MT, Sedykh A, Chakravarti SK, et al. Critical evaluation of human oral bioavailability for pharmaceutical drugs by using various cheminformatics approaches. Pharm Res. 2014;31:1002–1014. doi:10.1007/s11095-013-1222-1
- Pettersen EF, Goddard TD, Huang CC, et al. UCSF Chimera—a visualization system for exploratory research and analysis. J Comput Chem. 2004;25:1605–1612. doi:10.1002/jcc.20084
- Klaholz BP, Moras D. C-H-O Hydrogen bonds in the nuclear receptor RARc—a potential tool for drug selectivity. Structure. 2002;10:1197–1204. doi:10.1016/S0969-2126(02)00828-6
- Mishra A, Dey S. Molecular docking studies of a cyclic octapeptide-cyclosaplin from sandalwood. Biomolecules. 2019;9(11):740. doi:10.3390/biom9110740
- Imran M, Iqbal S, Hussain A. In silico screening, SAR and kinetic studies of naturally occurring flavonoids against SARS CoV-2 main protease. Arab J Chem. 2022;15(1):103473. doi:10.1016/j.arabjc.2021.103473
- Lakhera S, Devlal K, Ghosh A, et al. Modelling the DFT structural and reactivity study of feverfew and evaluation of its potential antiviral activity against COVID-19 using molecular docking and MD simulations. Chem Pap. 2022;76:2759–2776. doi:10.1007/S11696-022-02067-6
- Kattaru S, Manne Mudhu S, Echambadi Loganathan S, et al. Increased insulin and GLUT2 gene expression and elevated glucokinase activity in β-like cells of islets of langerhans differentiated from human haematopoietic stem cells on treatment with Costus igneus leaf extract. Mol Biol Rep. 2021;48:4477–4485. doi:10.1007/S11033-021-06467-X/FIGURES/5