Abstract
Explorative experiments were done to investigate the possibility that tomato plants infected by Botrytis cinerea have a different emission of volatile organic compounds (VOC) than healthy plants. This was done by headspace analysis of volatiles emitted by detached leaves of infected and healthy plants. Principal component analysis (PCA) of GC-FID chromatograms revealed clearly separated clusters between infected and control leaves and identification of an interesting compound. In further analysis by GC-MS, the significantly distinctive component (p≤0.05) was identified as the sesquiterpene α-copaene. In previous work on herbivore damage, α-copaene was not distinctive, which may suggest that α-copaene may be specifically associated to fungal infections in tomato.
Introduction
Botrytis cinerea is a major fungal pathogen which is known to cause grey mould in at least 235 host plant species, including greenhouse crops like tomato (Jarvis Citation1977). Therefore, about $20m / year is spent worldwide on chemical control of B. cinerea in greenhouses (Elad & Stewart Citation2004). This control depends upon first identifying the presence of a pathogen, a procedure which is currently dependent upon skilled personnel and is not automated. Current trends in glasshouse technology and management are founded on increasing automation and improved sensors, and this should also apply to disease or pathogen detection within the crop. These developments, and a drive to uncover how B. cinerea colonizes tomato tissue, are the motivation for this work.
Plants produce a wide range of volatile and non-volatile secondary metabolites (Croteau et al. Citation2000). In the past these compounds received little attention from ecologists and evolutionary biologists as it was thought that they were waste products of primary metabolism. Today, secondary metabolites are widely studied (Dixon Citation2001, Theis & Lerdau Citation2003) and, because they can play a role in protecting plants against a wide variety of microorganisms (viruses, bacteria, and fungi) (Wink Citation1988) they are increasingly the focus of breeding programmes including metabolic engineering (Aharoni et al. Citation2005). The monoterpenes (C10) and sesquiterpenes (C15) are secondary metabolite terpenoids that are volatile at temperatures commonly encountered under field and glasshouse conditions, thus contributing to the volatile organic compounds (VOCs) produced by plants. Monoterpenes are known as components of the volatile essences of flowers and of the essential oils of herbs and spices. Sesquiterpenes are also found in essential oils and, in addition, numerous sesquiterpenes act as phytoalexins, antibiotic compounds produced by plants in response to microbial challenge, and as antifeedants that discourage herbivory (Croteau et al. Citation2000, for review see Stoessl et al. Citation1976). Volatile terpenoids that are induced by insect herbivory have also been shown to be implicated in the attraction of natural enemies of these insects (Kappers et al. Citation2005). Given their role in pest and disease response and their volatile nature it has been suggested that measuring the type and amounts of terpenoids in the air of a glasshouse might be a means of monitoring the health of glasshouse crops (Baratto et al. Citation2005). This idea is particularly relevant to future closed greenhouse designs which will have no free ventilation and within which VOCs could accumulate, thus making detection more feasible. An implication of this is, of course, that these concentrated VOCs will have an increased effect on crops when they act as messengers of some kind (Wheeler et al. Citation2004). In research on other stresses, like herbivore damage (Ament et al. Citation2004) and mechanical damage (Maes Citation2002), the stressed tomato plants produced a different spectrum of VOCs than control plants. The hypothesis tested here is that B. cinerea-infected tomato plants produce a different spectrum of VOCs than healthy tomato plants. Measuring changes in the VOC fingerprint would therefore allow discrimination between a healthy tomato plant and an infected tomato plant, which could eventually lead to a disease detection system. The main research question to be answered is whether we can discriminate between a control plant and a plant infected with B. cinerea based on the VOC fingerprint. The second research question is to identify the VOCs that allow for this discrimination to be made, because these VOCs would be candidates for designing a disease detection system.
Material and methods
Plant material
Tomato seedlings (Lycopersicon esculentum Mill. cv. Moneymaker) were grown in 17 cm pots in potting compost (Lentse Potgrond, Lent, The Netherlands) in a greenhouse (natural light) with day and night temperatures of 20°C and 18°C, respectively. The relative humidity was always 70%. Prior to the experiments tomato plants were transferred to a climate room at 25±1°C and 65±10% relative humidity. Irradiance was supplied by fluorescent tubes (TLD 58W / 84, Philips, Eindhoven, The Netherlands) for 15 h / 9 h light / dark photoperiod with a photosynthetically active radiation of 120 µmolm−2s−1at leaf height. The light intensity was measured with a Li-250 photosynthetically active quantum flux meter (LiCor, Lincoln, Nebraska, USA).
Inoculation procedures
Botrytis cinerea strain (B0510) was cultured on malt extract agar (CM0059, Oxoid, Basingstoke, UK) at 25±1°C in 9-cm Petri dishes in the dark. Plates were inoculated in a laminar flow hood with a small droplet of spore suspension. After four days, cultures were exposed for 24 h to near-UV light to stimulate sporulation. One to two weeks after near-UV stimulation, conidia were harvested from the sporulating mycelium by washing with sterile water, containing 0.05% Tween 20 (Merck-Schuchardt, Hohenbrunn, Germany), and rubbing the mycelium. Conidia were filtered through glass wool, washed three times by centrifuging (8 min, 800 rpm, 20°C) and resuspended in sterile water. The spores were finally resuspended in an inoculum buffer prepared as described by Benito et al. (Citation1998) at a density of 109L−1. The inoculum buffer consisted of filter-sterilized water (0.2 µm, FP30 / 0,2 CA-S, Schleicher & Schuell, Dassel, Germany), supplemented with Gamborg's B5 medium (Duchefa Biochemie bv, Haarlem, The Netherlands), 10 mM glucose and 10 mM potassium phosphate (pH 6). The conidia concentration was counted under a light microscope with the use of a haemocytometer. The suspension was pre-incubated for 2–3 h, with occasional shaking by hand. Leaves of 4- and 7-week-old tomato plants were inoculated on the upper surface with eight 2 µl droplets on each of 8 leaves per plant. After inoculation, the plants were placed inside a transparent plastic tent for 16 h at 95% RH to stimulate infection. Relative humidity was checked with a humidity sensor (HMP 233, Vaisala, Helsinki, Finland) and the air was humidified periodically with an ultrasonic humidifier (572011, Conrad Electronic GmbH, Hirschau, Germany) attached to a time switch. After a 16-h infection period, the relative humidity was reduced to 85%; this humidity level accelerated the spreading of infection. Control plants were treated in exactly the same way, except that the inoculation droplets contained no spores. The infected and control plants were separated from each other during the infection process to prevent contamination of the control plants.
Sampling of volatiles
Six hours before headspace sampling, inoculated plants were taken out of the humidifier to allow droplets on the leaf surfaces to evaporate. Plants were placed in a separate part of the climate room to prevent cross-contamination by other plants. Leaves (1±0.5 g fresh weight) were carefully removed from the plant with a scalpel and placed in a glass Petri dish (300 ml). Care was taken not to damage the trichomes on the leaf. The petiole of the detached leaf was placed in purified water (Milli Q, Millipore, Bedford, MA, USA) to prevent dehydration, which itself could have induced VOC release. The Petri dish was immediately closed by a glass lid containing rubber septum (7824, Alltech, Deerfield, IL, USA). For quantification, volatiles were statically collected on polydimethylsiloxane (PDMS) fibres, which were housed in a solid phase microextraction (SPME) portable field sampler (1 cm coating length, 100 µm film thickness, Supelco, Bellefonte, PA, USA). Prior to use, fibres were conditioned in a gas chromatograph (GC) injection port at 250°C and subjected to two 30-min GC runs to purge any contaminating volatiles. Sampling was carried out by inserting the SPME piercing needle through the septum and exposing the fibre to the headspace for 2 h. There were 5 replicate samples of both the infected and control leaves. For identification of VOCs, dynamic sampling had to be performed to increase absolute amounts of the volatiles absorbed. In the dynamic sampling procedure the volatiles were collected on cartridges containing 90 mg Tenax TA (20 / 30 mesh, Chrompack, Walnut Creek, CA, USA) and head-space air was drawn through the tubes using a portable battery-operated air sampler with constant flow of 100 ml / min±5% (Ametek / du Pont de Nemours & Co., type Alpha-2, DEHA International, Huizen, The Netherlands). The sample collection times were 30 or 60 min and the flow-rate was checked with a soap bubble meter. Both infected and control leaves were sampled in three replicates. All glassware used in the experimental set-up was thoroughly cleaned with detergent and water and afterwards heated overnight in an oven (Binder, South Korea) at 250°C to remove any contaminating volatiles.
Analysis of volatiles
Volatiles were thermally desorbed for 5 min in a Varian 3400 CX gas chromatograph equipped with a flame ionization detector (Varian, Walnut Creek, CA, USA). The injections were made in splitless mode with a 0.75-mm inner diameter glass inlet liner (Supelco, Bellefonte, PA, USA). The GC-FID was operated with a injector temperature of 250°C and a detector temperature of 300°C. Compounds were separated on a capillary DB-5 column (30 m×0.25 mm ID, film thickness 0.25 µm; J&W Scientific, Folsom, California) at 40°C for 5 min after which the temperature was increased at 8°C min−1 to 225°C; this temperature was then maintained for 5 min. Nitrogen was used as the carrier gas. After 5 min the split was opened at 1:50. Data were collected by a personal computer running the Peaknet software package (Dionex Corporation, Sunnyvale, CA, USA). An n-alkane mix (C10–C17) was run through the column to provide a scale for the calculation of the retention index (RI). For most VOCs the RI is known (Adams Citation2001). Therefore we could identify the region in the chromatogram that was interesting for further analysis. For the VOCs from tomato plants the RI was between 1000 and 1700. For GC-MS analysis, volatiles were transferred to a capillary DB-5 column (60 m×0.25 mm ID, film thickness 0.25 µm) mounted in a Varian 3400 GC and connected to a Finnigan MAT 95 mass spectrometer. The oven temperature was set at 60°C for 4 min and then programmed to 220°C at a rate of 6°C min−1. The mass spectrometer was operated in the 70 eV electron impact ionization mode and scanning was from mass 24–300 at 0.7 sD−1. Compounds were identified by comparing the mass spectra and RI with those in the Adams collection (Adams Citation2001) and in the Wageningen Mass Spectral Library.
Statistical analysis
A fundamental problem in GC-FID data analysis is finding a suitable representation of this high-dimensionality data. Linear transformation is often chosen for dimension reduction in multivariate data (Hyvarinen Citation1999). Principal component analysis (PCA) (Jolliffe Citation1986) is a well known linear transformation method for reducing the dimensionality of metabolomics datasets (Jansen et al. Citation2004, Hendriks et al. Citation2005, Norwich Research Park, Citation2006). PCA defines a model of multivariate data. This model is a lower dimensional subspace that explains the direction of maximum variance in the original data matrix. Our multivariate data is a matrix with 18000 columns with retention variables measured on a set of 10 rows of samples (called objects). These samples are the chromatograms from the headspace of control and infected leaves. The first few principal components (PCs) explain most of the variance in the original data. Between chromatograms the retention time of identical compounds can differ; this is a problem inherent to GC analysis (Pate et al. Citation1998, Rohrback & Ramos Citation2003, Tomasi et al. Citation2004, Hendriks et al. Citation2005). Therefore, prior to PCA, the reduced chromatograms were pre-processed with the correlation optimized wrapping (COW) alignment algorithm (Tomasi et al. Citation2004).
COW is a piecewise or segmented data pre-processing method aimed at aligning a sample data vector to a reference vector by allowing limited changes in segment lengths on the sample vector (Tomasi et al. Citation2004). The chromatogram with the highest number of compounds was chosen as reference, as suggested by Tomasi et al. (Citation2004). The COW algorithm was used as implemented in Cowtool (v 1.1, BioCentrumDTU, Technical University of Denmark). The parameters were: segment length (m) was 25, so the number of segments (N) was therefore 720, and slack (t) was 2.
After alignment the data was loaded into a multivariate statistics software package (Unscrambler v 9.5, Camo A / S, Trondheim, Norway) for PCA analysis. First, the date was mean-normalized, which is a standard procedure when performing PCA on chromatography data, then the PCA was conducted for nine principal components. Mean normalization consists of dividing each chromatogram by its average peak height. Peak areas for the GC-FID data were calculated using the Gaussian function in PeakFit v4.12 (Seasolve Software Inc, Framingham, MA, USA) for peak fitting and calculation of peak area. The data was baseline corrected with the function Best 2D. GC-FID and GC-MS peak areas were first normalized by the area percent method. Thus the total area of the identified peaks in each chromatogram was 100%. Then an independent t-test (SPSS Inc, Chicago, Il, USA) was performed to test whether the mean peak area of the identified peaks of healthy control leaves and infected leaves are statistically different from each other.
Results
The penetration of the host tissue by B. cinerea is a process that can be divided into three sequential phases (Benito et al. Citation1998): Killing of the host tissue, formation of primary lesion, and formation of secondary lesion. After five days infected leaves had severe secondary lesions, whereas the control leaves had no visual lesions. These leaves were used for determination and identification of volatiles. In and , raw GC-FID chromatograms obtained from head-space samples of a healthy, control leaf and an infected leaf are shown. Clear differences between the control and infected leaf chromatograms are evident. An infected leaf emits a greater amount of VOCs, and the large peak at 20 min in infected leaves () is nearly absent in control leaves (). The region between C10 and C17 contains the VOCs known to be emitted by tomato plants; therefore, for further analyses the data was reduced to the region between 10 and 25 min, which is equivalent to 18,000 data points.
Figure 1. Raw GC-FID chromatogram of the headspace of a healthy detached leaf (A), a C7-C17 n-alkane mix (B) is shown below as a reference.
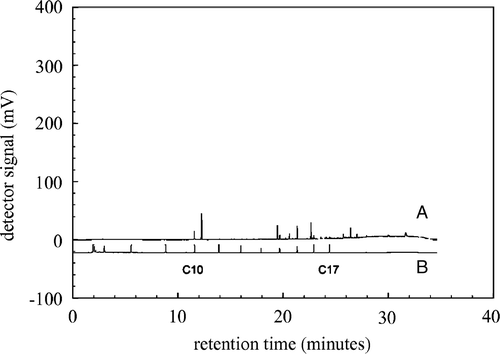
Figure 2. Raw GC-FID chromatogram of the headspace of an infected detached leaf (A), a C7-C17 n-alkane mix (B) is shown below as a reference.
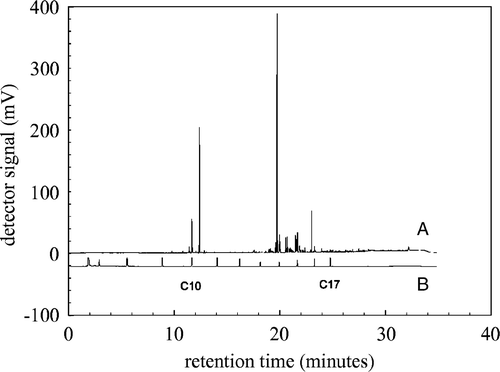
Principal component analysis
Principal components build a link between samples (chromatograms) and variables (detector signal at the different retention times) by means of scores and loadings. The scatter plot of the scores on the first two principal components shows clustering of the five control (1–5) and five infected samples (6–10) (). The first two principal components explained 96% of the variance present in the data set. The loading plot is used to identify which compounds are most responsible for separating of the 10 chromatograms into two groups. In , it is shown that infected leaves have a positive first principal component and healthy control leaves have a negative first principal component. The loading plot of the first principal component is shown in . If a variable has a high positive loading, it means that all chromatograms with a positive first principal component have higher than average values for that value. Thus the high positive loading in of the variable at data point 11,500 explains why all infected leaf chromatograms have a positive first principal component. The data point at 11,500 is equivalent to a retention time of 20 min.
Identification of the volatile fingerprint
The VOCs detected by GC-MS were mostly mono- and sesquiterpenes. In , the VOCs present in control and infected samples are shown (excluding all Tenax-derived impurities). Six out of the 27 different VOCs that were detected occurred in each chromatogram. The dominant peak at 20 min in the GC-FID chromatograms of the headspace of infected leaves could now be identified as α-copaene. Injection of an authentic α-copaene standard (27814, Fluka, Buchs, Switzerland) into the GC-FID showed an identical retention time to the major peak located at 20 min in the GC-FID chromatograms of headspace air from infected leaves. Cyclosativene and β-cubebene have a retention index close to α-copaene, as shown in , but different mass spectra. To be sure, the mass spectrum of the α-copaene peak was carefully checked for any deviations in intensity or extra peaks which could point to a co-eluting other compound.
Table I All detected volatiles emitted by tomato leaves by GC-MS analysis. Compounds are ordered according to their calculated retention index (RI). Samples of type I are peak areas from chromatograms of the headspace of infected leaves, samples of type C are peak areas from chromatograms of the headspace of control leaves. The compounds that are marked with an A occurred in each sample. Peak areas marked with B also contain impurities and peak areas marked with C also contain other terpenes. Trace levels are marked ‘tr’., ‘n.d.’ means not detected in the mass spectra and ‘ − ’ means not in this chromatogram.
Statistical analyses of GC-FID and GC-MS data
For proper statistical tests of the possible significance of differences in volatile production, normalization of the peak area is needed. In dynamic sampling this is done by injection of a standard with a known concentration into the sampling tube. In static sampling with SPME, which was done for acquiring the GC-FID data, this is not practical (Zabaras & Wyllie Citation2001). In SPME analysis headspace concentrations are calculated by injection of a monoterpene and sesquiterpene solution of which the concentration is known. The GC-FID should be equipped with a special 100% PDMS column (Maes Citation2002). This column was however not available in our laboratory. Normalization by the area percent method is then the most convenient method for normalization.
Peak areas of identified peaks in the GC-FID and GC-MS chromatograms were calculated and a t-test was done on these peak areas to discriminate between healthy control leaves and infected leaves. The GC-FID peak area of two major tomato volatile compounds (Buttery et al. Citation1987, Maes Citation2002, Ament et al. Citation2004) (11.70 and 12.40 min) previously identified by GC-MS as 2-carene and β-phellandrene, and the peak (described here) at 20 min, which was identified as α-copaene, were used. These three peaks were also identified by calculating their retention index. In , the result of that test is shown. All three compounds were found to be significantly different between control and infected samples (p<0.05).
Table II Volatiles emitted by tomato leaves, t-test on the GC-FID data. Compounds are ordered according to their calculated Retention index (RI). The values indicate the means of the normalized peak areas in percentages and standard deviation of each treatment group. The p-values have been calculated with an independent t-test (n=5). Independent samples, normality, and equal variances were assumed. The compounds that are marked with an asterisk (*) were found to be significantly different (p≤0.05) for infested leaves versus control leaves.
The peak areas of the five peaks that were present in all GC-MS chromatograms were tested for statistical significance. In , the results of that test are shown. In this case, 2-carene and α-copaene were found to be significantly different between control and infected samples (p<0.05).
Table III Volatiles emitted by tomato leaves, t-test on the GC-MS data. Compounds are ordered according to their calculated Retention index (RI). The values indicate the means of the normalized peak areas in percentages and standard deviation of each treatment group. The p-values have been calculated with an independent t-test (n=3). Independent samples, normality, and equal variances were assumed. The compounds that are marked with an asterisk (*) were found to be significantly different (p≤0.05) for infested leaves versus control leaves.
Discussion
This study was undertaken to identify volatile compounds associated to Botrytis infection of tomato plants. The production of volatiles may be used as a non-destructive measurement of plant health. Here we have shown that infected tomato leaves produce increased amounts of VOCs and that α-copaene is the most prominent volatile induced by the tomato leaf-Botrytis cinerea interaction. This conclusion is based upon the principal components analysis of the GC-FID data which identified a peak with an RI of 20 min as the major contributor to the dominant axis separating healthy and infected leaves, and the identification of this 20-min peak as α-copaene using GC-MS. The limonene peak (RI 1029), one of the other major compounds observed in the GC-MS chromatograms (), could not be identified in the GC-FID chromatograms. This is possibly because this compound is masked in the β-phellandrene peak (RI 1030), which has an almost identical RI. VOCs marked with an ‘A’ in were also found in studies on herbivore stressed tomato plants by (Dicke et al Citation1998, Farag & Paré Citation2002, Thaler et al Citation2002, Maes Citation2002, Kant et al Citation2004). Notably, the production of α-copaene was not found to increase significantly in any of these studies. This suggests that α-copaene production may be associated with fungal infections in tomato. So, α-copaene may be at the least specific for infections and possibly specific for fungal infections or even those of Botrytis.
It is known that other compounds are formed during the interaction between tomato leaves and B. cinerea, especially NO, H2O2 (Mahalingam & Fedoroff Citation2003) and ethylene (Diaz et al. Citation2002). These are of low molecular weight and, in the case of NO and H2O2, they are highly reactive with fast degradation times. The release of NO and H2O2 is related to programmed cell death, which occurs in response to pathogens and various abiotic stresses (Levine Citation2004). It is possible to detect ethylene, and (in spite of their reactive nature) NO and H2O2 online (Cristescu et al. Citation2002, Conrath et al. Citation2004). These volatiles are, however, not suitable for monitoring fungal infection because they are emitted after most biotic and abiotic stresses. Botrydial (C17H26O5) is a byciclic sesquiterpene produced in plant tissues infected by B. cinerea (Deighton et al. Citation2001). The vapor pressure of this compound is, however, too low for it to make a significant contribution to the VOCs produced by the infected leaves at ambient temperatures, and it could, therefore, not be detected
Ideally the identification of plant disease marker compounds should be correlated with measurements of the enzymes responsible for the synthesis of the compounds and the genes which encoded the enzymes. By this more complete analysis of volatile production, and its control, can the association of a specific volatile with a particular stimulus be better understood (Dicke et al. Citation2003). However the reactions catalyzed by many of these enzymes are complex and diverse, often generating one to upwards 30 reaction products (Steele et al. Citation1998). In the case of volatile production by Arabidopsis flowers, only two enzymes are responsible for the complex mixture of sesquiterpenes (Tholl et al. Citation2005). To our knowledge, no α-copaene synthase has been so far identified (Cane P, Tholl D, Croteau R, Fraga D, personal commication), and until this enzyme (or its gene) has been identified a more complete analysis of the control of α-copaene synthesis will not be possible. Nonetheless the physiological data presented here do strongly suggest that α-copaene is good candidate molecule for the detection of Botrytis (and possibly other fungal) infections in crops
We thank the Plant Pathology group of Jan van Kan (Wageningen University) for assistance and discussions about the colonization of the tomato leaves. We also thank Arjen van de Peppel and Joke Oosterkamp of the Horticultural Production Chains group (Wageningen University) for their assistance with the inoculation and the GC-FID analysis.
References
- Adams RP. 2001 . Identification of essential oil components by gas chromatography/quadrupole mass spectrometry . Allured, Carol Stream , Illinois, , USA .
- Aharoni , A , Jongsma , MA and Bouwmeester , HJ . 2005 . Volatile science? Metabolic engineering of terpenoids in plants . Trends Plant Sci , 10 : 594 – 602 .
- Ament , K , Kant , MR , Sabelis , MW , Haring , MA and Schuurink , RC . 2004 . Jasmonic acid is a key regulator of spider mite-induced volatile terpenoid and methyl salicylate emission in tomato . Plant Physiol , 135 : 2025 – 2037 .
- Baratto , C , Faglia , G , Pardo , M , Vezzoli , M , Boarino , L , Maffei , M , Bossi , S and Sberveglieri , G . 2005 . Monitoring plants health in greenhouse for space missions . Sensors Actuators B , 108 : 278 – 284 .
- Benito , EP , ten Have , A , van 't Klooster , JW and van Kan , J. 1998 . Fungal and plant gene expression during synchronized infection of tomato leaves by Botrytis cinerea . Eur J Plant Pathol , 104 : 207 – 220 .
- Buttery , R , Ling , L and Light , D . 1987 . Tomato leaf volatile aroma components . J Agric Food Chem , 35 : 1039 – 1042 .
- Conrath , U , Amoroso , G , Kohle , H and Sultemeyer , F . 2004 . Noninvasive online detection of nitric oxide from plants and some other organisms by mass spectrometry . Plant J , 38 : 1015 – 1022 .
- Cristescu , SM , Martinis , DD , te Lintel Hekkert , S , Parker , DH and Harren , FJM . 2002 . Ethylene production by Botrytis cinerea in vitro and in tomatoes . Appl Environ Microbiol , 68 : 5342 – 5350 .
- Croteau , R , Kutchan , T and Lewis , N . 2000 . “ Natural products (secondary metabolites) ” . In Biochemistry and molecular biology of plants , Edited by: Buchanan , B , Gruissem , W and Jones , R . 1250 – 1318 . Rockville : American Society of Plant Physiologists .
- Deighton , N , Muckenschnabel , I , Colmares , AJ , Collado , IG and Williamson , B . 2001 . Botrydial is produced in plant tissues infected by Botrytis cinerea . Phytochemistry , 57 : 689 – 692 .
- de Kraker , J-W , Franssen , MCR , de Groot , A , König , WA and Bouwmeester , HJ . 1998 . (+)-Germacrene A biosynthesis, the committed step in the biosynthesis of bitter sesquiterpene lactones in chicory . Plant Physiol , 117 : 1381 – 1392 .
- Diaz , J , ten Have , A and van Kan , J . 2002 . The role of ethylene and wound signaling in resistance of tomato to Botrytis cinerea . Plant Physiol , 129 : 1341 – 1351 .
- Dicke , M , Takabayashi , J , Posthumus , MA , Schutte , C and Krips , OE . 1998 . Plant phytoseiid interactions mediated by herbivore induced plant volatiles: Variation in production of cues and in responses of predatory mites . Experim Appl Acarol , 22 : 311 – 333 .
- Dicke , M , van Poecke , R and de Boer , J . 2003 . Inducible indirect defence of plants: From mechanisms to ecological functions . Basic Appl Ecol , 4 : 27 – 42 .
- Dixon , RA . 2001 . Natural products and plant disease resistance . Nature , 411 : 843 – 847 .
- Elad , Y and Stewart , A . 2004 . “ Microbial control of Botrytis spp ” . In Botrytis: “Biology, pathology and control , Edited by: Elad , Y , Williamson , B , Tudzynsky , B and Delen . 223 – 241 . Dordrecht, , The Netherlands : Kluwer Academic Publishers .
- Farag , MA and Paré , PW . 2002 . C6 green leaf volatiles trigger local and systemic VOC emissions in tomato . Phytochemistry , 61 : 545 – 554 .
- Hendriks , MMWB , Cruz-Juarez , L , Bont , DD and Hall , RD . 2005 . Preprocessing and exploratory analysis of chromatographic profiles of plant extracts . Analyt Chim Acta , 545 : 53 – 64 .
- Hyvarinen , A . 1999 . Survey on independent component analysis . Neural Computing Surveys , 2 : 94 – 128 .
- Jansen , J , Hoefsloot , H , Boelens , H , van der Greef , J and Smilde , A . 2004 . Analysis of longitudinal metabolomics data . Bioinformatics , 20 : 2438 – 2446 .
- Jarvis , WR . ( 1977 ). Botryotinia and Botrytis species . Taxonomy and pathogenicity . Canadian Department of Agriculture . Monograph 15 , Harrow , Ontario , Canada .
- Jolliffe , IT . 1986 . Principal component analysis , New York, , USA : Springer Verlag .
- Kant , MR , Ament , K , Sabelis , MW , Haring , MA and Schuurink , RC . 2004 . Differential timing of spider mite-induced direct and indirect defenses in tomato plants . Plant Physiol , 135 : 483 – 495 .
- Kappers , IF , Aharoni , A , van Herpen , TWJM , Luckerhoff , LLP , Dicke , M and Bouwmeester , HJ . 2005 . Genetic engineering of terpenoid metabolism attracts bodyguards to arabidopsis . Science , 309 : 2070 – 2072 .
- Levine , A . 2004 . “ Programmed cell death in plant response to biotic stress (pathogen attack) ” . In Programmed cell death in plants , Edited by: Gray , J . 213 – 250 . Oxford : Blackwell Publishing .
- Maes , K . 2002 . Volatiles emitted by plants: An in vitro approach [dissertation] , Gent, , Belgium : Gent University .
- Mahalingam , R and Fedoroff , N . 2003 . Stress response, cell death and signalling: The many faces of reactive oxygen species . Physiologia Plantarum , 119 : 56 – 68 .
- Norwich Research Park [internet] . Norwich, UK. [cited 11 July 2006 ]; Available from: http://www.metabolomics-nrp.org.uk/techniques.html#Multivariate%20Analysis
- Pate , ME , Thornhill , NF , Chandwani , R , Hoare , M and Titchener-Hooker , NJ . 1998 . Preprocessing of chromatographic data for principal component analysis . Bioprocess Engineering , 19 : 297 – 305 .
- Rohrback BG , Ramos LS. 2003 . Aligning chromatograms . Gulf Coast Conference, Galveston Island , Texas .
- Steele , CL , Crock , J , Bohlman , J and Croteau , R . 1998 . Sesquiterpene synthases from grand fir (Abies grandis) . J Biological Chem , 273 : 2027 – 2089 .
- Stoessl , A , Stotherst , J and Ward , E . 1976 . Sesquiterpenoid stress compounds of the Solanaceae . Phytochemistry , 15 : 855 – 872 .
- Thaler , JS , Farag , MA , Paré , PW and Dicke , M . 2002 . Jasmonate-deficient plants have reduced direct and indirect defences against herbivores . Ecol Lett , 5 : 764 – 774 .
- Theis , N and Lerdau , M . 2003 . The evolution of function in plant secondary metabolites . Int J Plant Sciences , 164 : 93 – 102 .
- Tholl , D , Chen , F , Petri , J , Gershenzon , J and Pichersky , E . 2005 . Two sesquiterpene synthases are responsible for the complex mixture of sesquiterpenes emitted from arabidopsis ?owers . Plant J , 42 : 757 – 771 .
- Tomasi , G , van den Berg , F and Andersson , C . 2004 . Correlation optimized warping and dynamic time warping as preprocessing methods for chromatographic data . J Chemometrics , 18 : 231 – 241 .
- Wheeler , RM , Peterson , BV and Stutte , GV . 2004 . Ethylene production throughout growth and development of plants . HortScience , 39 : 1541 – 1545 .
- Wink , M . 1988 . Plant breeding: importance of plant secondary metabolites for protection against pathogens and herbivores . Theoretical Appl Genet , 75 : 225 – 233 .
- Zabaras , D and Wyllie , SG . 2001 . Quantitative analysis of terpenoids in the gas phase using headspace solidphase microextraction (hsspme) . Flavour Fragrance J , 16 : 411 – 416 .