ABSTRACT
Introduction
Implanted neurostimulation devices are gaining traction as therapeutic options for people with certain forms of drug-resistant focal epilepsy. Some of these devices enable chronic electroencephalography (cEEG), which offers views of the dynamics of brain activity in epilepsy over unprecedented time horizons.
Areas covered
This review focuses on clinical insights and basic neuroscience discoveries enabled by analyses of cEEG from an exemplar device, the NeuroPace RNS® System. Applications of RNS cEEG covered here include counting and lateralizing seizures, quantifying medication response, characterizing spells, forecasting seizures, and exploring mechanisms of cognition. Limitations of the RNS System are discussed in the context of next-generation devices in development.
Expert opinion
The wide temporal lens of cEEG helps capture the dynamism of epilepsy, revealing phenomena that cannot be appreciated with short duration recordings. The RNS System is a vanguard device whose diagnostic utility rivals its therapeutic benefits, but emerging minimally invasive devices, including those with subscalp recording electrodes, promise to be more applicable within a broad population of people with epilepsy. Epileptology is on the precipice of a paradigm shift in which cEEG is a standard part of diagnostic evaluations and clinical management is predicated on quantitative observations integrated over long timescales.
1. Introduction
Epilepsy is the enduring tendency for recurrent seizures [Citation1] and the fourth most common neurological disorder [Citation2]. With a lifetime prevalence of 1 in 26, epilepsy affects over 50 million people worldwide [Citation2,Citation3]. Accentuating the global burden of epilepsy, one third of affected individuals have seizures that are incompletely controlled by anti-seizure medications (ASMs), termed drug-resistant epilepsy (DRE) [Citation4]. Many people with DRE are candidates for resection or ablation of seizure-producing brain tissue [Citation5], provided that the involved region(s) can be precisely delineated. Although highly effective, these destructive treatments are irreversible, potentially morbid, and not always feasible, underscoring the need for additional therapies. Recently, several forms of brain electrical stimulation—including implanted devices for vagus nerve stimulation (VNS), responsive neurostimulation (RNS), and deep brain stimulation (DBS)—have emerged as palliative treatment options for certain forms of DRE [Citation6,Citation7]. Despite operating through conceptually distinct strategies, these devices all deliver electrical energy to the brain to reduce seizures over time [Citation6]. Beyond this essential therapeutic function, all these devices also collect information that can be useful diagnostically. For example, newer models of VNS detect changes in the heart rate and body position that may relate to seizures [Citation8] and a recently approved device for thalamic DBS senses local field potential (LFP) power within specific frequency bands [Citation9]. Among approved neurostimulation devices for epilepsy, however, the RNS® System (NeuroPace, Inc.) is the only one that stores multichannel, direct recordings of cortical activity (electrocorticograms, ECoGs) and counts of detections of interictal epileptiform activity (IEA) and electrographic seizures, data that will form the basis of this review.
The RNS System comprises a cranially implanted neurostimulator, which houses the electronics and a battery, connected to two four-electrode cortical strips and/or depth leads [Citation10] placed intracranially at the seizure focus/foci [Citation11]. These leads mediate continuous sensing of neural activity, and in response to detections of abnormal (i.e. epileptiform) activity, they enable delivery of electrical pulses intended to terminate incipient seizures [Citation12–15]. A canonical use case involves bilateral mesial temporal seizure onsets, for which one depth lead is placed in each hippocampus, typically via a trans-occipital approach [Citation11], and the neurostimulator is programmed to detect and stimulate epileptiform activity on both sides; with average use in a configuration like this, the neurostimulator battery life is approximately 8.4 years [Citation12]. Safety of device implantation, including risk of infection (3.7% per procedure), compares favorably with other neurostimulation devices [Citation16,Citation17]. Stimulation is typically imperceptible to patients, even in eloquent cortical regions [Citation18], although stimulation-triggered symptoms occur rarely [Citation19–21]. The therapeutic benefits of this ‘closed-loop’ approach are now well-established by long-term clinical trials demonstrating 75% median reduction in seizure frequency over several years of treatment [Citation22–29], with 18.4% of patients achieving a period of seizure freedom lasting at least 1 year [Citation25]. A diverse, ever-expanding array of patients are candidates for RNS System therapy [Citation30–39], including those undergoing concurrent resection [Citation40,Citation41], and ~3,000 patients have been implanted to date in the US. Clinical success and cost-effectiveness [Citation42] of the RNS System in adult populations have motivated off-label use in pediatric populations, with promising early results [Citation43–49], and a dedicated pediatric clinical trial is being planned. Since its approval by the Food and Drug Administration in 2013, the use of the RNS System has also expanded due to growing awareness of its potential as a diagnostic tool. Indeed, epilepsy is among the most dynamic disorders in neurology, and the chronicity of RNS cEEG helps address the pitfalls of temporal undersampling in epilepsy [Citation50]. RNS cEEG is stable over years of recording [Citation51–54], and patients periodically download and transmit data stored on the device—including recordings triggered by prolonged detections of epileptiform activity (‘Long Episodes,’ LE’s) or by patients swiping a small magnet over the neurostimulator—to a secure online data repository (Patient Data Management System, PDMS; ). In PDMS, clinicians can view raw and graphical representations of patients’ neural data, enabling remote monitoring of epilepsy that is particularly advantageous in the age of telemedicine [Citation55] (). RNS cEEG is not without limitations: spatial coverage is sparse, sampling rate is low (250 Hz), and owing to memory constraints on the device, ECoGs are not continuous, which introduces temporal bias into recorded data [Citation56]. Still, the opportunity to access direct recordings of brain activity from ambulatory patients in naturalistic settings over many years is unparalleled and circumvents several limitations of intracranial EEG monitoring in acute inpatient environments [Citation57,Citation58].
Here, we review a diverse array of applications of RNS cEEG which collectively highlight its diagnostic potential and utility for clinical and research purposes (). We consider advantages and limitations of current technology and the implications for development of next-generation devices. We conclude by discussing advances in brain activity sensing with DBS devices and emerging devices for long-term recordings using subscalp electrodes which will expand availability of cEEG to a broader patient population. Overall, this review argues that, just as implantable long-term heart rhythm monitors catalyzed a revolution in cardiology [Citation180], devices for cEEG promise to bring about a sea change in clinical epileptology, including advances in activities as fundamental as counting seizures.
Figure 1. RNS System chronic intracranial electroencephalography (RNS cEEG).(center) RNS System neurostimulator is shown connected to two four-contact depth leads placed in bilateral hippocampi (green), though cortical strip leads and many other lead locations are also possible. (A) Four-channel bipolar-montaged RNS electrocorticogram (ECoG) showing interictal epileptiform activity (IEA; spikes, in this example) arising independently from the bilateral hippocampi. Hourly counts of detections of IEA are stored on the neurostimulator. Tick marks on x-axis indicate time in seconds, as in panel (B), which shows ECoGs that capture electrographic seizures of left or right hippocampal onset. (C) Magnet-triggered ECoGs. Magnet swipe over the RNS neurostimulator triggers ECoG storage, typically 60-sec before the swipe and 30-sec after the swipe. (top) Patient magnet swipe (red arrowhead and blue flag ‘M’) just after the offset of a seizure indicates that the event was perceptible to the patient. (bottom) Data from one patient showing that, over months, magnet swipes (gray flag ‘M’) to mark clinical seizures tend to occur on the rising phases of multidien cycles of daily IEA (blue bars). (D) Temporal trends in epileptiform activity. (top) Each row is a histogram representing normalized weekly average detections of IEA binned by hour of the day, revealing consistent circadian cycles with most detections occurring during the night (day/night represented by sun/moon icons). (middle) Long Episodes (proxy for electrographic seizures) demonstrate approximate two-week periodicity and a strong tendency for seizures to cluster over 1–3 days. (bottom) Daily IEA counts sharply decline after initiation of a new anti-seizure medication (blue arrowhead), possibly indicating efficacy of this medication. Blue lines in middle and bottom panels represent 28-day rolling average of the data. Data stored on the neurostimulator is synchronized with a secure online data bank (Patient Data Management System, PDMS) for review by clinicians
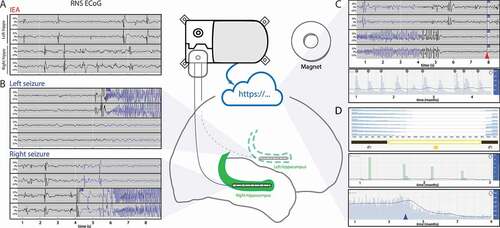
Figure 2. Clinical and research applications of RNS cEEG. (A) cEEG can reveal that subclinical (electrographic) seizures outnumber clinical seizures reported by patients. (B) In patients with bitemporal epilepsy, seizure lateralization determined by short-duration studies, like video-EEG in the Epilepsy Monitoring Unit (EMU), is prone to error due to undersampling. For example, one admission (EMU 1) might capture 3 left and 2 right seizures, while an admission of the same duration performed at a later time (EMU 2) could, by chance, capture 3 left seizures only, potentially leading to different conclusions about the patient’s surgical candidacy. The longer recording duration of cEEG (light green shading) can yield more accurate estimates of seizure lateralization. Analysis of epileptiform activity in RNS cEEG can help determine effective treatments (C; treatment 2 suppresses IEA to a greater extent than treatment 1) and can help characterize clinical spells based on the presence or absence of epileptiform activity in corresponding ECoGs (D). (E) Cycles of IEA (blue line) help determine periods of time (often the rising phases of these cycles) when seizures (red dots) are more likely to occur, though seizures may not occur during every high-risk period (dark red shading). (F) As a neuroscience research tool, RNS cEEG can reveal neurophysiological correlates of task performance in ambulatory subjects serially over long periods of time
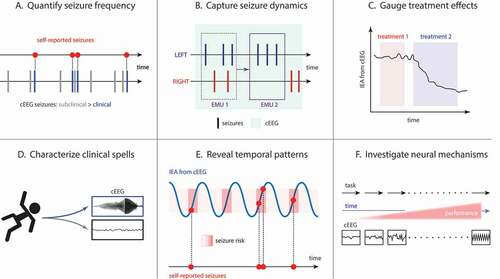
2. Tracking Seizures
Monitoring disease activity is critical for prognostication and treatment optimization in epilepsy, yet there is a paucity of disease biomarkers, aside from rates of seizures reported by patients themselves [Citation59]. Seizure diaries kept by people with epilepsy or their caregivers are the gold standard for monitoring epilepsy activity in both clinical practice and clinical trials [Citation60]. With the advent of the internet, online diaries and mobile applications have been developed to improve compliance with seizure charting. However, self-reported seizure diaries are known to be highly unreliable, with limited correlation with actual seizure frequency [Citation61–63]. Using counts of LEs, a reliable proxy for electrographic seizures in many patients [Citation64], recent studies have shown that electrographic seizures often outnumber seizures that patients report clinically, but LEs correlate strongly with diary-reported seizures and have high negative predictive value, such that patients are likely to be seizure-free on days without LEs [Citation65,Citation66]. In patients with mesial temporal lobe epilepsy, the interictal spike rate and frequency-specific spectral features in RNS cEEG strongly differentiate clinical outcomes and can serve as biomarkers of prognosis [Citation67]. Preliminary analyses suggest that ictal frequency modulation is also a biomarker of favorable response to RNS [Citation68]. Thus, RNS cEEG can potentially supplement seizure diaries by providing an objective biomarker for relative seizure burden and response to therapy [Citation69]. Quantification of electrographic seizure frequency can help corroborate clinical phenomena involving seizure patterns, such as seizure clustering () [Citation70]; the ‘running down’ effect, a progressive diminution of epileptiform activity after resective surgery [Citation71]; and the ‘capacitative’ property of seizures reported by some individuals, whereby longer seizure-free intervals tend to be followed by more severe seizures [Citation72]. However, caveats to relying on seizure data from RNS cEEG involve storage limitations on the device, which create temporal bias toward the most recent events, and the need for periodic downloads of information stored on the device, which can result in incomplete data if patients are noncompliant [Citation56]. Furthermore, like other intracranial hardware, implantation of the RNS System can temporarily reduce seizure frequency for 3–5 months (the ‘implant effect’ [Citation73]), creating a Heisenbergian scenario in which the recording device perturbs the very quantity it measures. Still, the chronicity of RNS cEEG is a major advantage when estimating intrinsically dynamic quantities, such as the proportion of seizures arising from each hemisphere in patients with bilateral independent seizure onsets [Citation181].
3. Seizure Lateralization and Localization
Beyond estimating seizure frequency, RNS cEEG can help determine seizure onset location(s). The most well-studied applications of RNS cEEG in this regard involve patients with bitemporal epilepsy, a specific phenotype within the spectrum of temporal lobe epilepsies [Citation74]. In the RNS System clinical trials, 43% of patients had bitemporal epilepsy [Citation25], and the median seizure frequency reduction in this group was 70% [Citation28]. Seizure laterality in bitemporal epilepsy is dynamic and can evolve over long timescales [Citation75,Citation76,Citation181]. In an analysis of 82 patients from the RNS System clinical trials who had bilateral mesial temporal leads, the average time to record bilateral electrographic seizures was 41.6 days [Citation77], considerably longer than the duration of most inpatient video-EEG (vEEG) evaluations, likely because these ambulatory patients were on ASMs during the recording period. With RNS cEEG, seven of 11 patients originally presumed to have unilateral seizures were found to have bilateral seizures, and, conversely, nine of 71 patients thought to have bilateral seizures had only unilateral seizures captured in long-term recordings [Citation77]. The latter group of ‘functionally unilateral’ patients, in whom ASMs presumably control seizures from one hemisphere only, is critical to identify, as they may be candidates for unilateral resective surgery [Citation78,Citation79]. Indeed, in one series, unilateral resection resulted in seizure freedom in 71% of patients for whom RNS cEEG revealed highly asymmetric seizure lateralization; this outcome is particularly remarkable because, by definition, these patients were not initially deemed candidates for resective surgery [Citation80,Citation81]. Seizure localization with RNS cEEG is more challenging than lateralization, owing to severely limited spatial sampling with only two four-electrode sensing leads, but, in highly select cases involving a narrow pre-test localization hypothesis, long-term recordings can yield information that contributes to identification of the seizure onset zone [Citation78,Citation82]. Information on seizure lateralization and localization can stratify candidacy for future surgical interventions, but, even in patients who do not go on to resective or ablative surgery, RNS cEEG can help characterize the effects of medications and other factors that influence seizure control.
4. Effects of Medications and Metabolites
4.1 Anti-seizure medications
The sporadic nature of seizures necessitates long periods of observation to ascertain the impact of exogenous or endogenous variables on seizure frequency [Citation83]. RNS cEEG can help unravel cause-and-effect relationships, as counts of IEA detections can be correlated with medication changes or fluctuations in physiological variables. For example, decreases in the rate of detections of IEA and LEs in the first 1–2 weeks after starting a new ASM predict long-term efficacy of that ASM [Citation84,Citation85]. Conversely, lack of an early decrease in IEA and LEs predicts inefficacy of a newly started ASM [Citation84]. Thus, RNS cEEG provides an early, objective metric of ASM efficacy that may help clinicians optimize treatment, facilitating identification of effective ASMs and minimizing duration of treatment with ASMs that are not beneficial [Citation86].
4.2 Other drugs and glucose
Therapy optimization with RNS cEEG can extend beyond ASMs to other pharmacological agents. In a recent report of a patient with bitemporal epilepsy, RNS detection counts revealed reversible exacerbation of hippocampal excitability by an antidepressant, mirtazapine [Citation87]. A report of another patient with bitemporal epilepsy described how increased RNS detections were observed in relation to excessive caffeine consumption on weekends, enabling specific dietary modification that improved seizure control [Citation88]. Similarly, RNS cEEG has been used to quantitatively assess the increase in epileptiform activity associated with e-cigarette use (vaping) [Citation89], which could potentially motivate behavior modification for therapeutic purposes. In a patient with type 1 diabetes, glucose levels correlated with seizure laterality [Citation90], the level of IEA [Citation91], and the circadian distribution of seizures [Citation92–95], illustrating how fluctuations in endogenous metabolites can be related to neurophysiological activity and suggesting that glycemic control in diabetic patients could be a target for therapeutic interventions to improve seizure control. Anecdotally, a common clinical observation is that quantitative, graphical representations of neural activity () as it relates to lifestyle choices and salient psychosocial variables can make cause-and-effect relationships concrete and can powerfully motivate behavioral changes in some patients [Citation55].
4.3 Sleep physiology
Few human behaviors have been studied in relation to epilepsy more than sleep [Citation96], but potential effects of neurostimulation devices on sleep are incompletely understood. The largest literature exists for VNS, and it is known that VNS is associated with sleep-disordered breathing, including obstructive sleep apnea [Citation97,Citation98], possibly owing to effects on laryngeal motility [Citation99]. Stimulation voltage-dependent sleep disruption can also occur in association with thalamic DBS [Citation100]. In a cohort of five subjects, RNS cEEG with concurrent polysomnography revealed that epileptiform activity, but not RNS System stimulations, triggered arousals, providing preliminary evidence that RNS is not associated with significant sleep disruption [Citation101]. In the future, the opportunity to access intracranial EEG during sleep serially over multiple nights—well beyond recording durations that are possible during acute inpatient monitoring—could prove to be advantageous, particularly in light of the fact that some sleep-related neurophysiological biomarkers in epilepsy are dynamic over timescales of days to weeks [Citation102]. With an older model of the RNS System (RNS-300M), cEEG during sleep was limited by an onboard analog 4 Hz high-pass filter that removes much delta activity, but the maximal bandwidth of recording from a newer model (RNS-320) is 1–90 Hz [Citation103]. Seizures occurring during sleep are the most likely to be underreported by patients [Citation62] but can be captured by RNS cEEG. In addition to quantifying unrecognized nocturnal seizures, RNS cEEG can facilitate characterization of spells whose etiology is unclear.
5. Spell Characterization
Inpatient vEEG is the gold standard for characterizing clinical spells based on the presence or absence of associated epileptiform activity [Citation104], but its limitations include high cost, dependence on bed availability, inconvenience for patients, brief recording duration (days), and the artificial nature of the inpatient environment and the seizure-provoking maneuvers that are often employed for practical purposes [Citation105]. As a costly, invasive modality with highly limited spatial sampling, RNS cEEG cannot supplant vEEG. Yet, assuming that RNS leads are accurately placed at the site(s) from which seizures arise in the brain, RNS cEEG can facilitate spell characterization in naturalistic ambulatory settings by providing intracranial recordings that may support or refute an epileptic etiology for a patient’s clinical symptoms. As outlined above, electrographic seizures are commonly under-reported by patients implanted with the RNS System [Citation65], but having intracranial electrodes with this device also provides a sensitive means of distinguishing focal aware seizures from nonepileptic events in patients who over-report seizures [Citation66]. Indeed, it is possible to leverage magnet-triggered ECoGs () and other RNS cEEG data to distinguish epileptic seizures from panic attacks, psychosis, and other psychiatric conditions that may have similar clinical manifestations [Citation106]. These diagnostic opportunities, involving objective records of illness that could potentially supersede patient report as ground truth, introduce unprecedented ethical dilemmas for practicing neurologists [Citation107,Citation108] and new experiences of illness for patients [Citation109]. Yet, increased accuracy in epileptic seizure detection using devices that provide cEEG [Citation110] holds much promise, such as improving statistical power and reducing cost of clinical trials, thereby accelerating discovery of new therapeutics [Citation111]. Reliable seizure detection over long timescales made possible by cEEG devices has also enabled quantitative analyses, revealing that seizure timing is less random than once thought.
6. Seizure Cycles and Forecasting
The seemingly random, unpredictable nature of seizure occurrence is a core feature of epilepsy and one of the most debilitating aspects of the disorder [Citation112]. Yet, a cyclical temporal structure for seizures in epilepsy has been purported since antiquity [Citation113], and, with the advent of cEEG and modern statistical techniques, historical explanations for seizure cycles—based on celestial, hormonal, and environmental factors—became testable [Citation83,Citation114,Citation115]. Recent studies using cEEG in humans and in animals have yielded converging evidence (reviewed in [Citation116]) for the existence of cycles of epileptic brain activity operating over diverse timescales: daily (circadian) [Citation93–95,Citation110,Citation117–121], multi-day (multidien) [Citation117,Citation119–121], and yearly (circannual) [Citation83,Citation120]. Identification of these cycles indicated that seizures are not entirely random events and propelled the decades-old field of seizure prediction to new heights where seizure forecasting—which aims to quantify the uncertainty of seizure occurrence over future horizons—has become the favored approach [Citation122,Citation123].
The landmark NeuroVista trial demonstrated feasibility of a seizure advisory system, based on cEEG, that warned of seizures minutes in advance [Citation110]. Subsequent analyses [Citation118,Citation124–129] of data from this trial yielded numerous transformative insights and advanced the concept of probabilistic forecasting of seizure likelihood [Citation130] although mostly over short time horizons (minutes). The NeuroVista device is no longer available, but studies of RNS cEEG have revealed that (a) IEA cycles occur in discrete chronotypes at the circadian and multidien timescales [Citation120], (b) electrographic and clinical seizures preferentially occur at certain phases of circadian and multidien cycles of IEA [Citation117,Citation120], and (c) knowledge of seizure phase preference can be leveraged to estimate accurately the probability of seizure occurrence up to several days in advance in some individuals [Citation131]. To date, the NeuroVista trial remains the only truly prospective trial of seizure prediction, but, with the high prevalence of IEA cycles [Citation120] and with the RNS System now in widespread clinical use in the U.S., a large-scale prospective trial of probabilistic seizure forecasting is imminently feasible. The ability to anticipate periods of heightened seizure risk opens the door to new therapeutic strategies, including chronotherapy, the time-varying adjustment of medications and other interventions to reduce seizures [Citation132]. Other applications include scheduling diagnostic tests, such as hospital admission for vEEG, based on times of greatest seizure likelihood to maximize diagnostic yield [Citation133]. While these emerging applications will require further study, RNS cEEG has already proven to be a valuable tool for research in neuroscience.
7. Neuroscience Research Tool
7.1 Studying cortical physiology
Research on patients undergoing intracranial EEG for pre-surgical seizure localization has yielded fundamental discoveries about vision, speech, decision-making, memory, and sensorimotor processing in the human brain [Citation134]. Yet, intrinsic limitations of acute inpatient monitoring, including limited patient mobility, perioperative variables, rapid medication changes, and the unavoidable distractions and interruptions related to hospital care, are prohibitive for certain types of testing. Furthermore, intracranial EEG is typically limited to ~3 weeks recording duration due to patient tolerance and risk of infection [Citation57], which precludes studies of chronic neurophysiology. RNS cEEG circumvents some of these limitations, given its chronicity, high signal-to-noise, and wireless nature. Although the RNS System was designed to detect pathological brain activity, serial recordings of speech-evoked neural responses in cortical language centers are stable over 1.5 years with RNS cEEG [Citation51], indicating that the device is also capable of measuring physiological activity related to essential human behavior. Indeed, hippocampal activity associated with cognitive tasks probing associative memory [Citation135] and calculation ability [Citation136] has also been recorded with RNS cEEG. Leveraging the opportunity to access intracranial recordings in untethered ambulatory subjects, researchers have recently incorporated the RNS System into a wireless mobile platform [Citation103] that enables monitoring of neural responses in freely moving humans engaged in spatial navigation tasks [Citation136,Citation137]. Among other insights, RNS cEEG from this platform has revealed that the human hippocampus encodes the spatial location of oneself and others in shared environments and that these neural representations are modulated by cognitive state [Citation138].
7.2 Brain stimulation for other disorders
The ability to detect behaviorally relevant, state-dependent neural signatures in RNS cEEG enables novel therapeutic strategies for disorders resulting from neural circuit dysfunction. Indeed, closed-loop brain stimulation, in which stimulation is delivered in response to detection of neurophysiological biomarkers that track specifically with clinical symptoms, is a promising approach to refractory neuropsychiatric disorders [Citation139]. Using this approach, the RNS System is being investigated as a novel therapeutic strategy for treatment-resistant depression (TRD) [Citation140,Citation141], Tourette’s syndrome [Citation142], and loss-of-control eating [Citation143]. Given evidence of modest but positive effects of RNS therapy on mood and cognition [Citation144–147], RNS cEEG provides a unique opportunity to study the influence of direct electrical stimulation on these higher-order brain functions over long periods of time.
7.3 Mechanism of RNS
Despite the ever-expanding applications of neurostimulation devices, the fundamental mechanisms underlying the effects of direct brain electrical stimulation remain opaque [Citation148]. Building on early work by Penfield and Jasper [Citation149], RNS was conceived by analogy to cardiac defibrillators as a strategy for terminating epileptiform activity with electrical counterstimulation [Citation150,Citation151]. Yet, decades later, the precise mechanism(s) by which RNS reduces seizures remain incompletely understood. Ironically, recent analyses of RNS cEEG have shed light on the effects of RNS therapy itself and an emerging view involves multiple effects that operate over divergent timescales. In the seconds following stimulation, RNS cEEG demonstrates decreased spectral power [Citation152] and suppression of high-frequency phase-locking [Citation153], effects which may desynchronize neural activity and contribute to acute seizure termination. In contrast to these acute (also termed ‘direct’) effects, several ‘indirect’ (i.e. not time-locked to stimulation) effects of RNS have been described—including spontaneous seizure termination, ictal frequency modulation, and fragmentation of electrographic seizure patterns—and, in a small study cohort, these effects were associated with better clinical outcomes than direct effects [Citation154]. The existence of indirect effects of RNS suggests a role for neuroplasticity and network-level mechanisms, which unfold over longer timescales than those required for acute seizure termination [Citation155], consistent with the gradual reduction in seizure frequency observed over years of RNS therapy in clinical trials [Citation25]. Indeed, recent evidence suggests that chronic, stimulation-dependent reorganization of interictal functional network connectivity underlies the therapeutic response to RNS [Citation156]. If corroborated by future studies, this observation has major implications for clinical device programming—typically aimed at stimulating early in the evolution of seizures while minimizing interictal stimulation—by suggesting that ‘nonspecific’ interictal stimulation may actually be critical for therapeutic efficacy. Furthermore, in light of the cyclical fluctuation of seizure risk [Citation130], the effects of RNS stimulation may be state-dependent, such that stimulation parameters that are effective at reducing seizures in one seizure risk state might be ineffective or even counterproductive in another seizure risk state [Citation157]. By analogy, early evidence indicates that efficacy of closed-loop stimulation for TRD may also depend on the mood state [Citation140,Citation141]. These insights, enabled by RNS cEEG, have resulted in a modern view of the therapeutic mechanism of RNS that diverges sharply from the original conception of acute seizure termination resulting from ictal stimulation and that may instead involve chronic, state-dependent network plasticity driven by interictal stimulation although these mechanisms are not mutually exclusive.
7.4 Mechanisms of other neurostimulation devices
The availability of three approved neurostimulation devices for DRE (VNS, RNS, and DBS) has led to increasing use of more than one neurostimulator in a single patient [Citation158,Citation159]. Despite scant objective evidence for treatment synergy between devices, some such effects are suspected. At a minimum, it is clear that failure to respond to one device does not necessarily predict failure to respond to others [Citation158,Citation160,], perhaps owing to the fact that each device likely works through mechanisms that are at least partly distinct [Citation6]. For patients implanted with the RNS System, RNS cEEG provides a means by which to elucidate the effects of other neurostimulation devices on neural activity. For example, RNS cEEG revealed that VNS therapy produces acute, frequency-specific desynchronization of neural activity [Citation161]. In another patient implanted with a thalamic DBS device and the RNS System, RNS cEEG revealed that anterior thalamic nuclei stimulation causes acute effects—phasic, frequency-dependent responses in hippocampus and temporal neocortex—and also chronic effects—progressive modulation of functional network connectivity and suppression of hippocampal epileptiform activity [Citation160]. These findings directly inform development of next-generation devices [Citation162,Citation163], which will be equipped with enhanced diagnostic capabilities [Citation56], advanced algorithms for seizure detection and stimulation parameter optimization [Citation164–167], adaptive stimulation design [Citation168], and patient-facing interfaces [Citation114]. In addition to such features, next-generation devices should ideally be minimally invasive and applicable to all people with epilepsy.
8. Subscalp EEG
Only about 50% of seizures are reported by people with epilepsy, mostly due to lack of awareness that a seizure has occurred [Citation63], and the inaccuracy of seizure counting limits optimal epilepsy management. As such, virtually all people with epilepsy could benefit from reliable, objective measurement of seizures, yet, despite the insights gained from modalities like RNS cEEG, risks intrinsic to implantation of intracranial devices [Citation16,Citation169,Citation170] hinder their widespread use. Ambulatory EEG and home video telemetry systems have advantages over routine ~30-min EEGs and inpatient vEEG [Citation171,Citation172], but their dependence on scalp electrodes, which require regular maintenance to retain high signal quality, and the limited recording durations (~1–2 weeks at most) remain significant disadvantages [Citation50,Citation173]. Subscalp EEG recording devices, involving electrodes implanted between the scalp and the cranium, overcome these limitations and promise transformative advances in clinical epilepsy [Citation173]. Several subscalp EEG systems are in development by groups around the world, and they differ with respect to the number of channels, degree of invasiveness, and primary intended purpose (e.g. seizure counting, seizure localization, cloud platform integration, etc.) [Citation173]. These devices are capable of recording EEG for up to eight months [Citation174] with recording quality comparable with other techniques [Citation175,Citation176], and they may be able to provide many of the same insights as RNS cEEG, including quantifying seizure frequency change in response to ASMs [Citation177], revealing circadian and multidien seizure cycles [Citation174,Citation178], and facilitating seizure forecasting [Citation179]. Patient tolerance and safety data are limited thus far, but the lone prospective trial of a subscalp EEG device did not yield major concerns [Citation178]. With additional study, subscalp EEG may become a routine component of diagnostic evaluations in epilepsy, analogous to the role of subcutaneous cardiac rhythm monitors in cardiology [Citation180].
9. Thalamic DBS with Brain Sensing
Recent clinical trials demonstrating effective seizure reduction by stimulation of the thalamus [Citation182–184] have rekindled interest in neural recordings from this deep structure. Although the thalamus is not typically sampled during intracranial seizure monitoring, recent investigations using RNS System [Citation185–191], DBS devices [Citation192], or stereo-EEG [Citation193,Citation194] with thalamic depth leads have demonstrated that ictal activity can be recorded in the thalamus, suggesting that thalamic sensing could facilitate therapeutic interventions [Citation193]. In 2020, the Medtronic PerceptTM PC platform was approved for DBS treatment of movement disorders, obsessive-compulsive disorder, and epilepsy. Whereas other approved DBS devices deliver electrical stimulation without recording, Percept PC is the first commercially available DBS platform with in vivo brain sensing capability [Citation9]. It provides a limited form of cEEG involving manually triggered 30-second LFP snapshots and power, averaged over 10 min, within a user-specified 5 Hz-wide frequency range of the LFP [Citation9]. At present, therapeutic strategies leveraging these types of cEEG data are better defined for movement disorders, like Parkinson’s disease [Citation195], than for epilepsy, but this will likely change over time with greater understanding of corticothalamic physiology during seizures [Citation192,Citation196,Citation197].
10. Conclusion
Epilepsy is among the most dynamic disorders in neurology, and the wide temporal lens of cEEG helps capture the spatiotemporal features of seizures in ways that are not possible with short timescale recordings. Although the RNS System is primarily a therapeutic device, RNS cEEG has found diverse and powerful applications in clinical and research domains, such as by revealing cyclical patterns of brain activity that manifest over long timescales and determine momentary seizure risk. Yet, significant limitations of current devices have motivated development of next-generation technology, including subscalp EEG devices and DBS systems that sense LFPs, with the promise of even greater diagnostic insights and potentially broader applicability. Compared to the latest iteration of the RNS System, next-generation devices will likely accept more than two leads and enable continuous EEG recording for longer durations and with higher sampling rates. Future devices may also employ new signal processing strategies based on machine learning or convolutional neural networks that facilitate early seizure detection [Citation198] and tailored delivery of responsive therapy that adapts to momentary brain state [Citation157,Citation199]. Finally, the ability of implanted devices to communicate with wearable sensors will be critical for seizure forecasting and other longitudinal applications [Citation200,Citation201,Citation202]. The field of epilepsy has already experienced a revolution in Big Data, but these recent advances usher in the age of Long Data, during which computational strategies suitable for processing EEG of unprecedented chronicity will present new opportunities for personalized treatments.
11. Expert Opinion
Sporadic seizures are the hallmark of epilepsy, one of the most dynamic disorders in neurology. Detection of paroxysmal physiological abnormalities and faithful characterization of their spatiotemporal features, long-term patterns, and association with endogenous and exogenous variables pose major challenges for the clinical epileptologist. Cardiologists treating heart arrhythmias have long faced similar problems, which were solved through development of technology enabling long-duration recordings of cardiac activity in ambulatory patients. In epilepsy, the advent of days-long inpatient vEEG and ambulatory EEG recording techniques were transformative methods for studying seizures, and the field is now on the precipice of another paradigm shift related to implanted devices that provide cEEG. Currently, the only approved device that provides direct cortical recordings over long periods of time (years) is the RNS System, a cranially implanted, closed-loop neurostimulation device that stores intermittent samples of four-channel, bipolar-montaged ECoG and hourly counts of detections of epileptiform activity recorded with two intracranial leads. Although used primarily as a therapeutic device to reduce seizures, the RNS System is also a vanguard for a new generation of devices that provide recordings of brain activity with unprecedented chronicity. As such, epileptologists’ early experience with this device foreshadows clinical and research possibilities that are on the horizon. For example, RNS cEEG has already demonstrated utility for counting seizures unrecognized by patients, lateralizing seizures in bitemporal epilepsy, quantifying response to new ASMs, determining the etiology of clinical spells, forecasting the likelihood of seizures hours to days in advance, and exploring fundamental mechanisms of human cognition.
The RNS System has limitations, many stemming from the fact that it was not designed as a diagnostic device. Implantation of this intracranial device is invasive, spatial sampling is severely limited, onboard memory capacity is small, detection algorithms are simple and nonadaptive, and data transmission is cumbersome. Currently implanted in ~3000 patients in the U.S., the RNS System is well-established as a safe, effective therapy for certain forms of drug-resistant focal epilepsy, but these intrinsic limitations may preclude scaling to all people with epilepsy who could benefit from cEEG. Indeed, widespread use of cEEG hinges on the development of less invasive devices designed specifically for diagnostic applications. Several sub-scalp EEG devices currently in development are particularly promising in this regard, and early reports suggest that they may be able to provide some of the same types of diagnostic insights as RNS cEEG, although further validation of this emerging technology is necessary. For new thalamic DBS devices that are capable of LFP sensing, more research will be needed to determine how these signals from deep nuclei should be incorporated into the care of people with epilepsy.
As cEEG becomes increasingly woven into the fabric of modern epileptology, clinical practice is expected to evolve in several ways. Devices for cEEG will likely be employed earlier in the patient journey from initial diagnosis to definitive treatment, perhaps even obviating conventional inpatient monitoring in select cases, which could have a favorable impact on health care economics. Clinical management will be predicated on objective seizure counts from cEEG and not solely on error-prone patient self-reports. Novel treatment paradigms, such as chronotherapy based on forecasted fluctuations in seizure risk, will be possible, and patient-facing interfaces may allow sharing of seizure risk forecasts with patients who would benefit from this quantified uncertainty. Integration of cEEG devices with other implanted and wearable devices and with multimodal streams of physiological data will help create an ‘Internet of Things’ in epilepsy. Since RNS cEEG has demonstrated such immense potential as a research tool, advances in sampling rate, spatial coverage, and data streaming capabilities will facilitate longitudinal studies that shed light on aging, mechanisms of cognition, and chronic disease processes. Creative applications of cEEG may eventually surpass the originally envisioned scope and intent of this technology, raising new ethical dilemmas. What happens when conclusions based on cEEG conflict with patient self-reports? How closely and frequently should voluminous cEEG data be reviewed, and who should own these data and derivatives thereof? What are the ramifications of providing patients with seizure risk estimates that may unduly influence their behavior? If these questions defy easy answers, it is because they arise at the leading edge of a rapidly evolving field that is as dynamic as epilepsy itself.
Article highlights
The RNS System is a cranially implanted neurostimulation device that is approved for treatment of drug-resistant focal epilepsy and that provides a limited form of chronic electroencephalography (cEEG).
RNS cEEG can help quantify seizure frequency and lateralization, the efficacy of anti-seizure medications, and the extent to which clinical spells correlate with epileptiform brain activity.
RNS cEEG has also proven to be a valuable tool for neuroscience research, such as by revealing cyclical patterns of brain activity that determine periods of heightened seizure risk and that can be leveraged to forecast seizure likelihood days in advance.
Long-term recordings of brain activity are advantageous in dynamic disorders like epilepsy, and minimally invasive sub-scalp devices for cEEG that are currently in development will find increasing applications in clinical and research realms.
Additional information
Funding
References
- Rao VR, Lowenstein DH. Epilepsy. Curr Biol. 2015;25(17):R742–746.
- England MJ, Liverman CT, Schultz AM, et al. Epilepsy across the spectrum: promoting health and understanding. A summary of the Institute of Medicine report. Epilepsy Behav. 2012;25(2):266–276.
- Epilepsy: a public health imperative. Geneva: World Health Organization; 2019.
- Kwan P, Schachter SC, Brodie MJ. Drug-resistant epilepsy. N Engl J Med. 2011;365(10):919–926.
- Wiebe S, Jette N. Pharmacoresistance and the role of surgery in difficult to treat epilepsy. Nat Rev Neurol. 2012;8(12):669–677.
- Markert MS, Fisher RS. Neuromodulation - science and practice in epilepsy: vagus nerve stimulation, thalamic deep brain stimulation, and responsive neurostimulation. Expert Rev Neurother. 2019;19(1):17–29.
- Fisher RS, Velasco AL. Electrical brain stimulation for epilepsy. Nat Rev Neurol. 2014;10(5):261–270.
- Fisher B, DesMarteau JA, Koontz EH, et al. Responsive vagus nerve stimulation for drug resistant epilepsy: a review of new features and practical guidance for advanced practice providers. Front Neurol. 2020;11:610379.
- Jimenez-Shahed J. Device profile of the percept PC deep brain stimulation system for the treatment of Parkinson’s disease and related disorders. Expert Rev Med Devices. 2021;18(4):319–332.
- Drees C, McDermott D, Sillau S, et al. Seizure outcome with responsive neurostimulation (RNS) comparing strip versus depth leads. Epilepsy Behav. 2020;112:107402.
- Krucoff MO, Wozny TA, Lee AT, et al. Operative technique and lessons learned from surgical implantation of the NeuroPace Responsive Neurostimulation® System in 57 consecutive patients. Oper Neurosurg (Hagerstown). 2021;20(2):E98–e109.
- Jarosiewicz B, Morrell MM. The RNS System: brain-responsive neurostimulation for the treatment of epilepsy. Expert Rev Med Devices. 2021;18(2):129–138.
- Skarpaas TL, Jarosiewicz B, Morrell MJ. Brain-responsive neurostimulation for epilepsy (RNS(®) System). Epilepsy Res. 2019;153:68–70.
- Morrell MJ, Halpern C. Responsive direct brain stimulation for epilepsy. Neurosurg Clin N Am. 2016;27(1):111–121.
- Matias CM, Sharan A, Wu C. Responsive neurostimulation for the treatment of epilepsy. Neurosurg Clin N Am. 2019;30(2):231–242.
- Weber PB, Kapur R, Gwinn RP, et al. Infection and erosion rates in trials of a cranially implanted neurostimulator do not increase with subsequent neurostimulator placements. Stereot Funct Neuros. 2017;95(5):325–329
- Lee B, Zubair MN, Marquez YD, et al. A single-center experience with the NeuroPace RNS System: a review of techniques and potential problems. World Neurosurg. 2015;84(3):719–726.
- Jobst BC, Skarpaas TL, Morrell MJ. Response: therapeutic brain-responsive neurostimulation in eloquent cortex can be delivered without symptoms. Epilepsia. 2017;58(8):1488.
- Hixon AM, Brown MG, McDermott D, et al. RNS modifications to eliminate stimulation-triggered signs or symptoms (STS): case series and practical guide. Epilepsy Behav. 2020;112:107327.
- Niketeghad S, Muralidharan A, Patel U, et al. Phosphene perceptions and safety of chronic visual cortex stimulation in a blind subject. J Neurosurg. 2019;132(6):2000–2007.
- Quraishi IH, Hirsch LJ. Patient-detectable responsive neurostimulation as a seizure warning system. Epilepsia. 2021;62(7):e110–e116.
- Bergey GK, Morrell MJ, Mizrahi EM, et al. Long-term treatment with responsive brain stimulation in adults with refractory partial seizures. Neurology. 2015;84(8):810–817.
- Heck CN, King-Stephens D, Massey AD, et al. Two-year seizure reduction in adults with medically intractable partial onset epilepsy treated with responsive neurostimulation: final results of the RNS System Pivotal trial. Epilepsia. 2014;55(3):432–441.
- Morrell MJ. Responsive cortical stimulation for the treatment of medically intractable partial epilepsy. Neurology. 2011;77(13):1295–1304.
- Nair DR, Laxer KD, Weber PB, et al., Nine-year prospective efficacy and safety of brain-responsive neurostimulation for focal epilepsy. Neurology. 2020;95(9):e1244–e1256.
- Geller EB. Responsive neurostimulation: review of clinical trials and insights into focal epilepsy. Epilepsy Behav. 2018;88:11–20.
- Jobst BC, Kapur R, Barkley GL, et al. Brain-responsive neurostimulation in patients with medically intractable seizures arising from eloquent and other neocortical areas. Epilepsia. 2017;58(6):1005–1014.
- Geller EB, Skarpaas TL, Gross RE, et al. Brain-responsive neurostimulation in patients with medically intractable mesial temporal lobe epilepsy. Epilepsia. 2017;58(6):994–1004.
- Razavi B, Rao VR, Lin C, et al. Real-world experience with direct brain-responsive neurostimulation for focal onset seizures. Epilepsia. 2020;61(8):1749–1757.
- Ma BB, Rao VR. Responsive neurostimulation: candidates and considerations. Epilepsy Behav. 2018;88:388–395.
- Benbadis SR, Geller E, Ryvlin P, et al. Putting it all together: options for intractable epilepsy: an updated algorithm on the use of epilepsy surgery and neurostimulation. Epilepsy Behav. 2018;88:33–38.
- Feyissa AM, Mohamed AS, Tatum WO, et al., Brain-responsive neurostimulation in adult-onset rasmussen’s encephalitis. 2021;Epilepsy Behav Rep. 15:100445.
- Chen H, Dugan P, Chong DJ, et al. Application of RNS in refractory epilepsy: targeting insula. Epilepsia Open. 2017;2(3):345–349.
- Nune G, Arcot Desai S, Razavi B, et al. Treatment of drug-resistant epilepsy in patients with periventricular nodular heterotopia using RNS® System: efficacy and description of chronic electrophysiological recordings. Clin Neurophysiol. 2019;130(8):1196–1207.
- Ernst LD, Krause KL, Kellogg MA, et al. Novel Use of Responsive Neurostimulation (RNS System) in the treatment of super refractory status epilepticus. J Clin Neurophysiol. 2019;36(3):242–245.
- Ma BB, Fields MC, Knowlton RC, et al. Responsive neurostimulation for regional neocortical epilepsy. Epilepsia. 2020;61(1):96–106.
- Yang JC, Harid NM, Nascimento FA, et al. Responsive neurostimulation for focal motor status epilepticus. Ann Clin Transl Neurol. 2021;8(6):1353–1361.
- McDermott DS, Mirro EA, Fetrow K, et al. Brain-Responsive Neurostimulation for the treatment of adults with epilepsy in tuberous sclerosis complex: a case series. Epilepsia Open. 2021;6(2):419–424.
- Zawar I, Krishnan B, Mackow M, et al. The efficacy, safety, and outcomes of brain-responsive neurostimulation (RNS® System) therapy in older adults. Epilepsia Open. 2021. https://doi.org/10.1002/epi4.12541
- Enatsu R, Alexopoulos A, Bingaman W, et al. Complementary effect of surgical resection and responsive brain stimulation in the treatment of bitemporal lobe epilepsy: a case report. Epilepsy Behav. 2012;24(4):513–516.
- Tran DK, Tran DC, Mnatsakayan L, et al. Treatment of multi-focal epilepsy with resective surgery plus responsive neurostimulation (RNS): one institution’s experience. Front Neurol. 2020;11:545074.
- Youngerman BE, Mahajan UV, Dyster TG, et al. Cost-effectiveness analysis of responsive neurostimulation for drug-resistant focal onset epilepsy. Epilepsia. 2021. https://doi.org/10.1111/epi.17049.
- Bercu MM, Friedman D, Silverberg A, et al. Responsive neurostimulation for refractory epilepsy in the pediatric population: a single-center experience. Epilepsy Behav. 2020;112:107389.
- Singhal NS, Numis AL, Lee MB, et al. Responsive neurostimulation for treatment of pediatric drug-resistant epilepsy. Epilepsy Behav Case Rep. 2018;10:21–24.
- Kokoszka MA, Panov F, La Vega-Talbott M, et al. Treatment of medically refractory seizures with responsive neurostimulation: 2 pediatric cases. J Neurosurg Pediatr. 2018;21(4):421–427.
- Theroux L, Shah Y, Cukier Y, et al. Improved seizure burden and cognitive performance in a child treated with responsive neurostimulation (RNS) following febrile infection related epilepsy syndrome (FIRES). Epileptic Disord. 2020;22(6):811–816.
- Mortazavi A, Elliott RS, Phan TN, et al. Responsive neurostimulation for the treatment of medically refractory epilepsy in pediatric patients: strategies, outcomes, and technical considerations. J Neurosurg Pediatr. 2021;1–8.
- Panov F, Ganaha S, Haskell J, et al. Safety of responsive neurostimulation in pediatric patients with medically refractory epilepsy. J Neurosurg Pediatr. 2020;26(5):525–532.
- Nagahama Y, Zervos TM, Murata KK, et al. Real-world preliminary experience with responsive neurostimulation in pediatric epilepsy: a multicenter retrospective observational study. Neurosurgery. 2021. https://doi.org/10.1093/neuros/nyab343.
- Baud MO, Schindler K, Rao VR. Under-sampling in epilepsy: limitations of conventional EEG. Clin Neurophysiol Pract. 2021;6:41–49.
- Rao VR, Leonard MK, Kleen JK, et al. Chronic ambulatory electrocorticography from human speech cortex. Neuroimage. 2017;153:273–282.
- Wu C, Evans JJ, Skidmore C, et al. Impedance variations over time for a closed-loop neurostimulation device: early experience with chronically implanted electrodes. Neuromodulation. 2013;16(1):46–50.
- Sillay KA, Rutecki P, Cicora K, et al. Long-term measurement of impedance in chronically implanted depth and subdural electrodes during responsive neurostimulation in humans. Brain Stimul. 2013;6(5):718–726.
- Sillay KA, Ondoma S, Wingeier B, et al. Long-term surface electrode impedance recordings associated with gliosis for a closed-loop neurostimulation device. Ann Neurosci. 2018;25(4):289–298.
- Mirro EA, Halpern CH. Letter: using continuous intracranial electroencephalography monitoring to manage epilepsy patients during COVID-19. Neurosurgery. 2020;87(3):E409–e410.
- Sisterson ND, Wozny TA, Kokkinos V, et al. A rational approach to understanding and evaluating responsive neurostimulation. Neuroinformatics. 2020;18(3):365–375.
- Shah AK, Mittal S. Invasive electroencephalography monitoring: indications and presurgical planning. Ann Indian Acad Neurol. 2014;17(Suppl 5):S89–94.
- Parvizi J, Kastner S. Promises and limitations of human intracranial electroencephalography. Nat Neurosci. 2018;21(4):474–483.
- Hegde M, Lowenstein DH. The search for circulating epilepsy biomarkers. Biomark Med. 2014;8(3):413–427.
- Fisher RS, Blum DE, DiVentura B, et al. Seizure diaries for clinical research and practice: limitations and future prospects. Epilepsy Behav. 2012;24(3):304–310.
- Blum DE, Eskola J, Bortz JJ, et al. Patient awareness of seizures. Neurology. 1996;47(1):260–264.
- Hoppe C, Poepel A, Elger CE. Epilepsy: accuracy of patient seizure counts. Arch Neurol. 2007;64(11):1595–1599.
- Elger CE, Hoppe C. Diagnostic challenges in epilepsy: seizure under-reporting and seizure detection. Lancet Neurol. 2018;17(3):279–288.
- Quigg M, Sun F, Fountain NB, et al. Interrater reliability in interpretation of electrocorticographic seizure detections of the responsive neurostimulator. Epilepsia. 2015;56(6):968–971.
- Quigg M, Skarpaas TL, Spencer DC, et al. Electrocorticographic events from long-term ambulatory brain recordings can potentially supplement seizure diaries. Epilepsy Res. 2020;161:106302.
- Chen H, Koubeissi M. Seizure occurrences: patient report, scalp EEG, and RNS electrocorticography findings. J Clin Neurophysiol. 2020;37(4):306–309.
- Arcot Desai S, Tcheng TK, Morrell MJ. Quantitative electrocorticographic biomarkers of clinical outcomes in mesial temporal lobe epileptic patients treated with the RNS(R) system. Clin Neurophysiol. 2019;130(8):1364–1374.
- Venkatesh P, Sneider D, Danish M, et al. Quantifying a frequency modulation response biomarker in responsive neurostimulation. J Neural Eng. 2021;18(4):046017.
- Young MG, Vadera S, Lin JJ, et al. Using electrocorticogram baseline seizure frequency to assess the efficacy of responsive neurostimulation. Epilepsy Behav. 2018;85:7–9.
- Chiang S, Haut SR, Ferastraoaru V, et al. Individualizing the definition of seizure clusters based on temporal clustering analysis. Epilepsy Res. 2020;163:106330.
- Geller AS, Friedman D, Fang M, et al. Running-down phenomenon captured with chronic electrocorticography. Epilepsia Open. 2018;3(4):528–534.
- Fan JM, Chiang S, Rao VR. Evidence for long memory in focal seizure duration. Epilepsia Open. 2021;6(1):140–148.
- Sun FT, Arcot Desai S, Tcheng TK, et al. Changes in the electrocorticogram after implantation of intracranial electrodes in humans: the implant effect. Clin Neurophysiol. 2018;129(3):676–686.
- Didato G, Chiesa V, Villani F, et al. Bitemporal epilepsy: a specific anatomo-electro-clinical phenotype in the temporal lobe epilepsy spectrum. Seizure. 2015;31:112–119.
- Smart O, Rolston JD, Epstein CM, et al. Hippocampal seizure-onset laterality can change over long timescales: a same-patient observation over 500 days. Epilepsy Behav Case Rep. 2013;1:56–61.
- Spencer D, Gwinn R, Salinsky M, et al. Laterality and temporal distribution of seizures in patients with bitemporal independent seizures during a trial of responsive neurostimulation. Epilepsy Res. 2011;93(2–3):221–225.
- King-Stephens D, Mirro E, Weber PB, et al., Lateralization of mesial temporal lobe epilepsy with chronic ambulatory electrocorticography. Epilepsia. 2015;56(6):959–967.
- DiLorenzo DJ, Mangubat EZ, Rossi MA, et al. Chronic unlimited recording electrocorticography-guided resective epilepsy surgery: technology-enabled enhanced fidelity in seizure focus localization with improved surgical efficacy. J Neurosurg. 2014;120(6):1402–1414.
- Feyissa AM, Mirro EA, Wabulya A, et al. Brain-responsive neurostimulation treatment in patients with GAD65 antibody-associated autoimmune mesial temporal lobe epilepsy. Epilepsia Open. 2020;5(2):307–313.
- Hirsch LJ, Mirro EA, Salanova V, et al., Mesial temporal resection following long-term ambulatory intracranial EEG monitoring with a direct brain-responsive neurostimulation system. Epilepsia. 2020;61(3):408–420.
- Englot DJ. Responsive neurostimulation in epilepsy: wall to block seizures or bridge to resection? Epilepsy Curr. 2020;20(5):265–266.
- Chan AY, Knowlton RC, Chang EF, et al. Seizure localization by chronic ambulatory electrocorticography. Clin Neurophysiol Pract. 2018;3:174–176.
- Rao VR, Leguia MG, Tcheng TK. Cues for seizure timing. Epilepsia. 2021;62(Suppl S1):S15–s31.
- Quraishi IH, Mercier MR, Skarpaas TL, et al. Early detection rate changes from a brain-responsive neurostimulation system predict efficacy of newly added antiseizure drugs. Epilepsia. 2020;61(1):138–148.
- Skarpaas TL, Tcheng TK, Morrell MJ. Clinical and electrocorticographic response to antiepileptic drugs in patients treated with responsive stimulation. Epilepsy Behav. 2018;83:192–200.
- Tjong E, Peng YY. Antiepileptic drug optimization using ambulatory electrocorticographic data from an implanted brain-responsive neurostimulator. Clin Case Rep. 2020;8(3):441–448.
- Warner NM, Gwinn RP, Doherty MJ. Individualizing therapies with responsive epilepsy neurostimulation - A mirtazapine case study of hippocampal excitability. Epilepsy Behav Case Rep. 2016;6:70–72.
- Mackow MJ, Krishnan B, Bingaman WE, et al. Increased caffeine intake leads to worsening of electrocorticographic epileptiform discharges as recorded with a responsive neurostimulation device. Clin Neurophysiol. 2016;127(6):2341–2342.
- Oster JM, Tatum P, Monigan C, et al. Seizures noted by responsive neurostimulation from e-cigarette use (vaping). J Clin Neurophysiol. 2021;Publish Ahead of Print. https://doi.org/10.1097/WNP.0000000000000866. online ahead of print.
- Kinnear KM, Warner NM, Gersappe A, et al. Pilot data on responsive epilepsy neurostimulation, measures of sleep apnea and continuous glucose measurements. Epilepsy Behav Case Rep. 2018;9:33–36.
- Pappas A, Kubsad S, Baud MO, et al. Does glucose influence multidien cycles of interictal and/or ictal activities? Seizure. 2021;85:145–150.
- Wright KE, Kollmyer DM, Warner NM, et al., How might tissue glucose influence responsive neurostimulation detection? 2019;Epilepsy Behav Rep. 12:100331.
- Spencer DC, Sun FT, Brown SN, et al. Circadian and ultradian patterns of epileptiform discharges differ by seizure-onset location during long-term ambulatory intracranial monitoring. Epilepsia. 2016;57(9):1495–1502.
- Anderson CT, Tcheng TK, Sun FT, et al. Day-night patterns of epileptiform activity in 65 patients with long-term ambulatory electrocorticography. J Clin Neurophysiol. 2015;32(5):406–412.
- Duckrow RB, Tcheng TK. Daily variation in an intracranial EEG feature in humans detected by a responsive neurostimulator system. Epilepsia. 2007;48(8):1614–1620.
- Frauscher B, Gotman J. Sleep, oscillations, interictal discharges, and seizures in human focal epilepsy. Neurobiol Dis. 2019;127:545–553.
- Parhizgar F, Nugent K, Raj R. Obstructive sleep apnea and respiratory complications associated with vagus nerve stimulators. J Clin Sleep Med. 2011;7(4):401–407.
- Marzec M, Edwards J, Sagher O, et al. Effects of vagus nerve stimulation on sleep-related breathing in epilepsy patients. Epilepsia. 2003;44(7):930–935.
- Zambrelli E, Saibene AM, Furia F, et al. Laryngeal motility alteration: a missing link between sleep apnea and vagus nerve stimulation for epilepsy. Epilepsia. 2016;57(1):e24–27.
- Voges BR, Schmitt FC, Hamel W, et al. Deep brain stimulation of anterior nucleus thalami disrupts sleep in epilepsy patients. Epilepsia. 2015;56(8):e99–e103.
- Ruoff L, Jarosiewicz B, Zak R, et al. Sleep disruption is not observed with brain-responsive neurostimulation for epilepsy. Epilepsia Open. 2020;5(2):155–165.
- Gliske SV, Irwin ZT, Chestek C, et al. Variability in the location of high frequency oscillations during prolonged intracranial EEG recordings. Nat Commun. 2018;9(1):2155.
- Topalovic U, Aghajan ZM, Villaroman D, et al., Wireless programmable recording and stimulation of deep brain activity in freely moving humans. Neuron. 2020; 108(2): 322–334.e329.
- Eddy CM, Cavanna AE. Video-electroencephalography investigation of ictal alterations of consciousness in epilepsy and nonepileptic attack disorder: practical considerations. Epilepsy Behav. 2014;30:24–27.
- Benbadis SR, Beniczky S, Bertram E, et al. The role of EEG in patients with suspected epilepsy. Epileptic Disord. 2020;22(2):143–155.
- Issa Roach AT, Chaitanya G, Riley KO, et al. Optimizing therapies for neurobehavioral comorbidities of epilepsy using chronic ambulatory electrocorticography. Epilepsy Behav. 2020;102:106814.
- Hegde M, Chiong W,Rao VR. New Ethical and Clinical Challenges in “Closed-Loop” Neuromodulation. Neurology. 2021;96(17):799–804.
- Yan H, Ibrahim GM. Resective epilepsy surgery involving eloquent cortex in the age of responsive neurostimulation: a value-based decision-making framework. Epilepsy Behav. 2019;99:106479.
- Haeusermann T, Lechner CR, Fong KC, et al. Closed-loop neuromodulation and self-perception in clinical treatment of refractory epilepsy. AJOB Neurosci 2021:1–13. https://doi.org/10.1080/21507740.2021.1958100
- Cook MJ, O’Brien TJ, Berkovic SF, et al. Prediction of seizure likelihood with a long-term, implanted seizure advisory system in patients with drug-resistant epilepsy: a first-in-man study. Lancet Neurol. 2013;12(6):563–571.
- Goldenholz DM, Tharayil JJ, Kuzniecky R, et al. Simulating clinical trials with and without intracranial EEG data. Epilepsia Open. 2017;2(2):156–161.
- Dumanis SB, French JA, Bernard C, et al. Seizure forecasting from idea to reality. Outcomes of the My Seizure Gauge Epilepsy Innovation Institute workshop. eNeuro. 2017;4(6). https://doi.org/10.1523/ENEURO.0349-17.2017
- Wilson JV, Reynolds EH. Texts and documents. Translation and analysis of a cuneiform text forming part of a Babylonian treatise on epilepsy. Med Hist. 1990;34(2):185–198.
- Baud MO, Rao VR. Gauging seizure risk. Neurology. 2018;91(21):967–973.
- Leguia MG, Rao VR, Kleen JK, et al. Measuring synchrony in bio-medical timeseries. Chaos: An Interdisciplinary Journal of Nonlinear Science. 2021;31(1):013138.
- Karoly PJ, Rao VR, Gregg NM, et al., Cycles in epilepsy. Nat Rev Neurol. 2021;17(5):267–284.
- Baud MO, Kleen JK, Mirro EA, et al., Multi-day rhythms modulate seizure risk in epilepsy. Nat Commun. 2018;9(1): 88.
- Karoly PJ, Ung H, Grayden DB, et al. The circadian profile of epilepsy improves seizure forecasting. Brain. 2017;140(8):2169–2182.
- Karoly PJ, Goldenholz DM, Freestone DR, et al. Circadian and circaseptan rhythms in human epilepsy: a retrospective cohort study. Lancet Neurol. 2018;17(11):977–985.
- Leguia MG, Andrzejak RG, Rummel C, et al., Seizure Cycles in Focal Epilepsy. JAMA Neurol. 78(4): 454–463. 2021.
- Gregg NM, Nasseri M, Kremen V, et al. Circadian and multiday seizure periodicities, and seizure clusters in canine epilepsy. Brain Commun. 2020;2(1):fcaa008.
- Mormann F, Andrzejak RG, Elger CE, et al. Seizure prediction: the long and winding road. Brain. 2007;130(2):314–333.
- Kuhlmann L, Lehnertz K, Richardson MP, et al. Seizure prediction - ready for a new era. Nat Rev Neurol. 2018;14(10):618–630.
- Karoly PJ, Cook MJ, Maturana M, et al. Forecasting cycles of seizure likelihood. Epilepsia. 2020;61(4):776–786.
- Kuhlmann L, Karoly P, Freestone DR, et al. Epilepsyecosystem.org: crowd-sourcing reproducible seizure prediction with long-term human intracranial EEG. Brain. 2018;141(9):2619–2630.
- Brinkmann BH, Wagenaar J, Abbot D, et al. Crowdsourcing reproducible seizure forecasting in human and canine epilepsy. Brain. 2016;139(6):1713–1722.
- Cook MJ, Varsavsky A, Himes D, et al. The dynamics of the epileptic brain reveal long-memory processes. Front Neurol. 2014;5:217.
- Karoly PJ, Nurse ES, Freestone DR, et al. Bursts of seizures in long-term recordings of human focal epilepsy. Epilepsia. 2017;58(3):363–372.
- Maturana MI, Meisel C, Dell K, et al. Critical slowing down as a biomarker for seizure susceptibility. Nat Commun. 2020;11(1):2172.
- Baud MO, Proix T, Rao VR, et al. Chance and risk in epilepsy. Curr Opin Neurol. 2020;33(2):163–172.
- Proix T, Truccolo W, Leguia MG, et al., Forecasting seizure risk in adults with focal epilepsy: a development and validation study. Lancet Neurol. 2021;20(2):127–135.
- Sanchez Fernandez I, Loddenkemper T. Chronotherapeutic implications of cyclic seizure patterns. Nat Rev Neurol. 2018;14(12):696–697.
- Karoly PJ, Eden D, Nurse ES, et al. Cycles of self-reported seizure likelihood correspond to yield of diagnostic epilepsy monitoring. Epilepsia. 2021;62(2):416–425.
- Chiong W, Leonard MK, Chang EF. Neurosurgical patients as human research subjects: ethical considerations in intracranial electrophysiology research. Neurosurgery. 2018;83(1):29–37.
- Henin S, Shankar A, Hasulak N, et al. Hippocampal gamma predicts associative memory performance as measured by acute and chronic intracranial EEG. Sci Rep. 2019;9(1):593.
- Meisenhelter S, Testorf ME, Gorenstein MA, et al. Cognitive tasks and human ambulatory electrocorticography using the RNS System. J Neurosci Methods. 2019;311:408–417.
- Aghajan ZM, Schuette P, Fields TA, et al. Theta oscillations in the human medial temporal lobe during real-world ambulatory movement. Curr Biol. 2017;27(24):3743–3751.e3743.
- Stangl M, Topalovic U, Inman CS, et al. Boundary-anchored neural mechanisms of location-encoding for self and others. Nature. 2021;589(7842):420–425.
- Provenza NR, Matteson ER, Allawala AB, et al. The case for adaptive neuromodulation to treat severe intractable mental disorders. Front Neurosci. 2019;13:152.
- Scangos KW, Khambhati AN, Daly PM, et al. Closed-loop neuromodulation for treatment-resistant depression. Nat Med, (2021). 27 10 1696–1700 https://doi.org/10.1038/s41591-021-01480-w
- Scangos KW, Makhoul GS, Sugrue LP, et al. State-dependent responses to intracranial brain stimulation in a patient with depression. Nat Med. 2021;27(2):229–231.
- Molina R, Okun MS, Shute JB, et al. Report of a patient undergoing chronic responsive deep brain stimulation for Tourette syndrome: proof of concept. J Neurosurg. 2018;129(2):308–314.
- Wu H, Adler S, Azagury DE, et al. Brain-responsive neurostimulation for loss of control eating: early feasibility study. Neurosurgery. 2020. https://doi.org/10.1093/neuros/nyaa300.
- Loring DW, Jarosiewicz B, Meador KJ, et al. Mood and quality of life in patients treated with brain-responsive neurostimulation: the value of earlier intervention. Epilepsy Behav. 2021;117:107868.
- Loring DW, Kapur R, Meador KJ, et al. Differential neuropsychological outcomes following targeted responsive neurostimulation for partial-onset epilepsy. Epilepsia. 2015;56(11):1836–1844.
- Meador KJ, Kapur R, Loring DW, et al. Quality of life and mood in patients with medically intractable epilepsy treated with targeted responsive neurostimulation. Epilepsy Behav. 2015;45:242–247.
- Chan AY, Rolston JD, Rao VR, et al. Effect of neurostimulation on cognition and mood in refractory epilepsy. Epilepsia Open. 2018;3(1):18–29.
- Borchers S, Himmelbach M, Logothetis N, et al. Direct electrical stimulation of human cortex - the gold standard for mapping brain functions? Nat Rev Neurosci. 2012;13(1):63–70.
- Penfield W, Jasper H. Epilepsy and the functional anatomy of the human brain. Boston: Little, Brown and Company; 1954.
- Lesser RP, Kim SH, Beyderman L, et al. Brief bursts of pulse stimulation terminate afterdischarges caused by cortical stimulation. Neurology. 1999;53(9):2073–2081.
- Kossoff EH, Ritzl EK, Politsky JM, et al. Effect of an external responsive neurostimulator on seizures and electrographic discharges during subdural electrode monitoring. Epilepsia. 2004;45(12):1560–1567.
- Rønborg SN, Esteller R, Tcheng TK, et al. Acute effects of brain-responsive neurostimulation in drug-resistant partial onset epilepsy. Clin Neurophysiol. 2021;132(6):1209–1220.
- Sohal VS, Sun FT. Responsive neurostimulation suppresses synchronized cortical rhythms in patients with epilepsy. Neurosurg Clin N Am. 2011;22(4):481–488.
- Kokkinos V, Sisterson ND, Wozny TA, et al. Association of closed-loop brain stimulation neurophysiological features with seizure control among patients with focal epilepsy. JAMA Neurol. 2019;76(7):800–808.
- Bruzzone MJ, Issa N, Rose S, et al. Insights into the therapeutic effect of responsive neurostimulation assessed with scalp EEG recording: a case report. J Clin Neurophysiol. 2018;35(5):438–441.
- Khambhati AN, Shafi A, Rao VR, et al. Long-term brain network reorganization predicts responsive neurostimulation outcomes for focal epilepsy. Sci Transl Med. 2021;13(608):eabf6588. 13 608 https://doi.org/10.1126/scitranslmed.abf6588
- Chiang S, Khambhati AN, Wang ET, et al. Evidence of state-dependence in the effectiveness of responsive neurostimulation for seizure modulation. Brain Stimul. 2021;14(2):366–375.
- Brown M, Ernst L, Spencer D. Brain-responsive stimulation (RNS) and vagal nerve stimulation (VNS) in combination for the treatment of drug-resistant focal epilepsy. American Epilepsy Society Annual Meeting, Abst. 3.16 (2019).
- Barbaro MF, Chesney K, Kramer DR, et al. Dual responsive neurostimulation implants for epilepsy. J Neurosurg. 2019;1–7.
- Silva AB, Khambhati AN, Speidel BA, et al. Effects of anterior thalamic nuclei stimulation on hippocampal activity: chronic recording in a patient with drug-resistant focal epilepsy Epilepsy & Behavior Reports .2021;16:100467.
- Ernst LD, Steffan PJ, Srikanth P, et al. Electrocorticography analysis in patients with dual neurostimulators supports desynchronization as a mechanism of action for acute vagal nerve stimulator stimulation J Clin Neurophysiol. 2021, https://doi.org/10.1097/WNP.0000000000000847.
- Kremen V, Brinkmann BH, Kim I, et al. Integrating brain implants with local and distributed computing devices: a next generation epilepsy management system. IEEE J Transl Eng Health Med. 2018;6:2500112.
- Kwon CS, Jetté N, Ghatan S. Perspectives on the current developments with neuromodulation for the treatment of epilepsy. Expert Rev Neurother. 2020;20(2):189–194.
- Sun Y, Friedman D, Dugan P, et al. machine learning to classify relative seizure frequency from chronic electrocorticography. J Clin Neurophysiol. 2021;https://doi.org/10.1097/WNP.0000000000000858.
- Constantino AC, Sisterson ND, Zaher N, et al. Expert-level intracranial electroencephalogram ictal pattern detection by a deep learning neural network. Front Neurol. 2021;12:603868.
- Sisterson ND, Wozny TA, Kokkinos V, et al. Closed-loop brain stimulation for drug-resistant epilepsy: towards an evidence-based approach to personalized medicine. Neurotherapeutics. 2019;16(1):119–127.
- Xu K, Zheng Y, Zhang F, et al. An energy efficient AdaBoost cascade method for long-term seizure detection in portable neurostimulators. IEEE Trans Neural Syst Rehabil Eng. 2019;27(11):2274–2283.
- De Ridder D, Maciaczyk J, Vanneste S. The future of neuromodulation: smart neuromodulation. Expert Rev Med Devices. 2021;18(4):307–317.
- Giles TX, Bennett J, Stone CE, et al. Characterizing complications of intracranial responsive neurostimulation devices for epilepsy through a retrospective analysis of the federal MAUDE database. Neuromodulation. 2020; https://doi.org/10.1111/ner.13259
- Wei Z, Gordon CR, Bergey GK, et al. Implant site infection and bone flap osteomyelitis associated with the NeuroPace Responsive Neurostimulation System. World Neurosurg. 2016;88:687.e681–687.e686.
- Kandler R, Ponnusamy A, Wragg C. Video ambulatory EEG: a good alternative to inpatient video telemetry? Seizure. 2017;47:66–70.
- Kaundal A, Hegde V, Khan H, et al. Home video EEG telemetry. Pract Neurol. 2021;21(3):212–215.
- Duun-Henriksen J, Baud M, Richardson MP, et al. A new era in electroencephalographic monitoring? Subscalp devices for ultra-long-term recordings. Epilepsia. 2020;61(9):1805–1817.
- Viana PF, Duun-Henriksen J, Glasstëter M, et al. 230 days of ultra long-term subcutaneous EEG: seizure cycle analysis and comparison to patient diary. Ann Clin Transl Neurol. 2021;8(1):288–293.
- Bacher D, Amini A, Friedman D, et al. Validation of an EEG seizure detection paradigm optimized for clinical use in a chronically implanted subcutaneous device. J Neurosci Methods. 2021;358:109220.
- Weisdorf S, Gangstad SW, Duun-Henriksen J, et al. High similarity between EEG from subcutaneous and proximate scalp electrodes in patients with temporal lobe epilepsy. J Neurophysiol. 2018;120(3):1451–1460.
- Weisdorf S, Zibrandtsen IC, Kjaer TW. Subcutaneous EEG monitoring reveals AED response and breakthrough seizures. Case Rep Neurol Med.2020 Jan 28;2020:8756917.
- Weisdorf S, Duun-Henriksen J, Kjeldsen MJ, et al. Ultra-long-term subcutaneous home monitoring of epilepsy-490 days of EEG from nine patients. Epilepsia. 2019;60(11):2204–2214.
- Stirling RE, Maturana MI, Karoly PJ, et al. Seizure forecasting using a novel sub-scalp ultra-long term EEG monitoring system. Front Neurol. 2021;12:713794.
- Nadkarni A, Devgun J, Jamal SM, et al. Subcutaneous cardiac rhythm monitors: state of the art review. Expert Rev Med Devices. 2021;18(7):587-596.
- Chiang S, Fan JM, Rao VR. Bilateral temporal lobe epilepsy: How many seizures are required in chronic ambulatory electrocorticography to estimate the laterality ratio? Epilepsia. 2021 (in press) doi:https://doi.org/10.1111/epi.17113
- Salanova V, Sperling MR, Gross RE, et al. The SANTÉ study at 10 years of follow-up: effectiveness, safety, and sudden unexpected death in epilepsy. Epilepsia. 2021;62(6):1306–1317.
- Salanova V, Witt T, Worth R, et al. Long-term efficacy and safety of thalamic stimulation for drug-resistant partial epilepsy. Neurology. 2015;84(10):1017–1025.
- Fisher R, Salanova V, Witt T, et al. Electrical stimulation of the anterior nucleus of thalamus for treatment of refractory epilepsy. Epilepsia. 2010;51(5):899–908.
- Elder C, Friedman D, Devinsky O, et al. Responsive neurostimulation targeting the anterior nucleus of the thalamus in 3 patients with treatment-resistant multifocal epilepsy. Epilepsia Open. 2019;4(1):187–192.
- Burdette DE, Haykal MA, Jarosiewicz B, et al. Brain-responsive corticothalamic stimulation in the centromedian nucleus for the treatment of regional neocortical epilepsy. Epilepsy Behav. 2020;112:107354.
- Kokkinos V, Urban A, Sisterson ND, et al. Responsive neurostimulation of the thalamus improves seizure control in idiopathic generalized epilepsy: a case report. Neurosurgery. 2020;87(5):E578–E583.
- Kwon CS, Schupper AJ, Fields MC, et al. Centromedian thalamic responsive neurostimulation for Lennox-Gastaut epilepsy and autism. Ann Clin Transl Neurol. 2020;7(10):2035–2040.
- Herlopian A, Cash SS, Eskandar EM, et al. Responsive neurostimulation targeting anterior thalamic nucleus in generalized epilepsy. Ann Clin Transl Neurol. 2019;6(10):2104–2109.
- Gummadavelli A, Zaveri HP, Spencer DD, et al. Expanding brain-computer interfaces for controlling epilepsy networks: novel thalamic responsive neurostimulation in refractory epilepsy. Front Neurosci. 2018;12:474.
- Burdette D, Mirro EA, Lawrence M, et al. Brain-responsive corticothalamic stimulation in the pulvinar nucleus for the treatment of regional neocortical epilepsy: a case series. Epilepsia Open. 2021;6(3):611–617.
- Gregg NM, Marks VS, Sladky V, et al. Anterior nucleus of the thalamus seizure detection in ambulatory humans. Epilepsia. 2021;62(10):e158–e164.
- Toth E, Kumar S, Ganne C, et al. Machine learning approach to detect focal-onset seizures in the human anterior nucleus of the thalamus. J Neural Eng. 2020;17(6):066004.
- Pizzo F, Roehri N, Giusiano B, et al. The ictal signature of thalamus and basal ganglia in focal epilepsy: a SEEG study. Neurology. 2021;96(2):e280–e293.
- Gilron R, Little S, Perrone R, et al. Long-term wireless streaming of neural recordings for circuit discovery and adaptive stimulation in individuals with Parkinson’s disease. Nat Biotechnol. 2021;39(9):1078–1085.
- Paz JT, Davidson TJ, Frechette ES, et al. Closed-loop optogenetic control of thalamus as a tool for interrupting seizures after cortical injury. Nat Neurosci. 2013;16(1):64–70.
- Gregg NM, Sladky V, Nejedly P, et al. Ambulatory IEEG biomarkers and epilepsy rhythms during anterior thalamic nuclei stimulation. American Epilepsy Society Annual Meeting, Abst. 456 (2020).
- Dümpelmann M. Early seizure detection for closed loop direct neurostimulation devices in epilepsy. J Neural Eng. 2019;16(4):041001.
- Chiang S, Goldenholz DM, Moss R, et al. Prospective validation study of an epilepsy seizure risk system for outpatient evaluation. Epilepsia. 2020;61(1):29–38.
- Stirling RE, Grayden DB, D’Souza W, et al. Forecasting seizure likelihood with wearable technology. Front Neurol. 2021;12:704060.
- Brinkmann BH, Karoly PJ, Nurse ES, et al. Seizure diaries and forecasting with wearables: epilepsy monitoring outside the clinic. Front Neurol. 2021;12:690404.
- Lhatoo SD, Bernasconi N, Blumcke I, et al. Big data in epilepsy: clinical and research considerations. Report from the Epilepsy Big Data Task Force of the International League Against Epilepsy. Epilepsia. 2020;61(9):1869–1883.