ABSTRACT
Prior research indicates that digital smoking cessation interventions can be effective, but little is known about their active ingredients. Therefore, this review aimed to examine the associations of content (behaviour change techniques [BCTs]), delivery features (delivery mode, readability, ease-of-use), and socioeconomic position with effectiveness. Systematic searches and hand searches were conducted from February to June 2023 to identify experimental evaluations of digital smoking cessation interventions published since 2004. Random-effects meta-analyses were used to explore intervention effectiveness. Meta-CART were used to explore whether content, delivery features, or socioeconomic position moderate effectiveness and assessed interactions between potential moderators. Meta-regressions were performed as sensitivity checks. For k = 29 studies (n = 42,662), the authors provided sufficient data and materials for inclusion in the primary analyses. Participants in the intervention groups had greater odds of successfully quitting smoking (OR = 1.29, 95% CI: 1.10–1.51, p = .002) with similar effect sizes across socioeconomic groups (ORlow SEP = 1.25, 95% CI: 1.00–1.57, p = .048; ORhigh SEP = 1.36, 95% CI: 1.06–1.76, p = .017). No delivery features were significantly associated with effectiveness. The BCT ‘commitment’ was associated with larger effects in populations with high, but not low, socioeconomic positions. There were no significant interactions between potential moderators. Digital smoking cessation interventions are effective across socioeconomic groups. Uncertainty around active ingredients remains.
Introduction
Smoking is still a key contributor to worldwide morbidity and mortality (Department of Health and Social Care, Citation2017; Lewer et al., Citation2020; Marteau et al., Citation2021; NHS Digital, Citation2020; Office for National Statistics, Citation2022). Socioeconomic differences in the success rates of quit attempts play a crucial role in maintaining the social gradient in smoking (Hiscock et al., Citation2012; Kotz & West, Citation2009; Marteau et al., Citation2021), which, in turn, contributes to stark inequalities in health outcomes and life expectancy (Lewer et al., Citation2020). Individual-level, behavioural interventions with and without pharmacological support have historically been unable to compensate for these inequalities in quit success (T. Brown et al., Citation2014; Hill et al., Citation2014). However, novel and improved smoking cessation interventions, especially for disadvantaged groups, are still needed to achieve the UK government target of ‘Smokefree 2030’ (i.e., a smoking prevalence of under 5% by the year 2030) for all groups (Hopkinson, Citation2020), even if all of the government’s recently announced planned policies are implemented (Department of Health and Social Care, Citation2023).
Digital behaviour change interventions (DBCIs) are services or products aimed at changing behaviours, such as smoking, delivered through computer or mobile technologies, such as mobile phones, smartphone applications, websites, or wearable devices (West & Michie, Citation2016). They have the potential to fill treatment gaps (Abernethy et al., Citation2022) as they do not present the same barriers as other treatment modalities, such as face-to-face behavioural support (Kale et al., Citation2019; Kwah et al., Citation2019). Barriers to face-to-face support include a lack of time, monetary, and childcare resources to attend inflexible appointments (Kale et al., Citation2019; Kwah et al., Citation2019). Since DBCIs can be used remotely and asynchronously, they do not present these same barriers. DBCIs can also be more discrete than other intervention modalities, potentially preventing barriers related to shame, lack of social support, and privacy concerns (Kale et al., Citation2019; Kwah et al., Citation2019). Additionally, there is considerable interest in DBCIs by healthcare providers and the National Health Service (NHS) due to their potential reach and scalability (Abernethy et al., Citation2022; NHS, Citation2019). While there is a plentiful supply of DBCIs for smoking cessation, most are not evidence-informed and few have been formally evaluated, and may therefore be inadequate to address the complexity of smoking behaviour (Abernethy et al., Citation2022; Tofighi et al., Citation2019; Ubhi, Kotz, et al., Citation2016). Reviews of different types of DBCIs for smoking cessation developed in a research context have found that these DBCIs are more effective than non-active controls (Amiri & Khan, Citation2023; Graham et al., Citation2016; Griffiths et al., Citation2018; Kingkaew, Citation2018; Liu et al., Citation2023; McCrabb et al., Citation2019; Taylor et al., Citation2017; Whittaker et al., Citation2019). However, their effectiveness compared to active controls is unclear (Graham et al., Citation2016; Griffiths et al., Citation2018; Liu et al., Citation2023; Taylor et al., Citation2017; Whittaker et al., Citation2019).
Ascertaining what differentiates more from less effective interventions can offer insight into their active ingredients, which can then aid in the designing of more effective interventions in the future. However, previous systematic reviews that have tried to identify which content and delivery features of interventions are the active ingredients have reached divergent conclusions (Bartlett et al., Citation2014; Black et al., Citation2020; de Ruijter et al., Citation2022; Griffiths et al., Citation2018; Kingkaew, Citation2018; McCrabb et al., Citation2019). The reasons for these different findings are not entirely clear. However, it may be partly attributable to different inclusion criteria and interactions between different ‘entities’ of each behaviour change intervention ‘scenario’ (Michie et al., Citation2020). That is, different attributes of each intervention and study are included in the review, such as intervention content, delivery mode, and the target population. Specifically, there are indications that the effectiveness of DBCIs for smoking cessation may vary according to the socioeconomic characteristics of the population using them (J. Brown et al., Citation2014; Coleman et al., Citation2022). Interventions relying on or targeting specific mechanisms may be more or less effective for people in different socioeconomic positions due to differences in material and social circumstances, resources, and barriers, including general and (digital) health literacy as well as smoking-related and quitting-related cognitions (Estrela et al., Citation2023; Hiscock et al., Citation2012; Twyman et al., Citation2014). This may contribute to socioeconomic disparities in quit success (Hiscock et al., Citation2012). However, the mechanisms underlying this potential effect moderation by socioeconomic position are still unclear (Kock et al., Citation2019; Siddiqi & Dogar, Citation2014; Smith et al., Citation2021). Another possibility is that there are synergistic or antagonistic effects when certain intervention contents are combined with each other or certain delivery features. For example, a certain intervention content (e.g., strengthening of the non-smoker identity) may be effective in the presence of certain other content (e.g., focus on past success) or when delivered by text messaging where it is readily accessible in the environment where a person usually smokes, compared with delivery on a website, where accessing it might be more difficult in the context where a person usually smokes. The past decade has seen substantial advances in behaviour change theory (e.g., Michie et al., Citation2013, Citation2020) and statistical techniques (e.g., Li et al., Citation2017). These innovations allow us to explore divergent findings and heterogeneity in study results in new ways to further our understanding of what works for whom under which circumstances.
There are a few taxonomies that can be used to reliably code different attributes of DBCIs to compare interventions, identify potential active ingredients, and guide the development of new interventions. One of the most comprehensive, reliable, and well-validated taxonomies of potential active ingredients is the Behaviour Change Technique Taxonomy v1 (BCTTv1) (Abraham et al., Citation2015; Michie et al., Citation2013; Ogden, Citation2016). Behaviour Change Techniques (BCTs) are generalisable content components of a behaviour change intervention that can be observed and replicated (Michie et al., Citation2013). The BCTTv1 has been used in several reviews aiming to identify active ingredients of smoking cessation interventions (Bartlett et al., Citation2014; Black et al., Citation2020; de Ruijter et al., Citation2022; Griffiths et al., Citation2018; Kingkaew, Citation2018; McCrabb et al., Citation2019). However, reviews of the relationship between intervention attributes besides BCTs, including delivery features (such as delivery mode, language, and usability), and intervention effectiveness are scarce (Bartlett et al., Citation2014; Black et al., Citation2020; Griffiths et al., Citation2018). This is partly due to a lack of coherent, widely used, well-validated taxonomies to conceptualise the intervention attributes (Lehto & Oinas-Kukkonen, Citation2011; Oinas-Kukkonen & Harjumaa, Citation2009; Sama et al., Citation2014; Yang, Citation2021). However, a taxonomy of ease-of-use features for DBCIs has been developed and found to have good to acceptable reliability (Ubhi, Kotz, et al., Citation2016; Ubhi, Michie, et al., Citation2016). Additionally, readability can be reliably assessed using the Gunning-Fog-Index (Gunning, Citation1969), a standardised and widely used measure of text complexity (Bothun et al., Citation2021; Grossman et al., Citation1994; Kue et al., Citation2021; Szmuda et al., Citation2020). The delivery mode can be coded based on a recently developed and validated ontology (Marques et al., Citation2020).
In summary, a better understanding of which features of DBCIs make them effective for whom is necessary to develop improved interventions to decrease smoking rates and improve population health and reduce health inequalities.
Aims and research questions
The aim of this review is to identify which intervention content and delivery features of DBCIs for smoking cessation are associated with intervention effectiveness and to explore whether and how socioeconomic position (SEP) moderates this relationship.
Specifically, this systematic review seeks to answer the following questions:
What are the associations between the effectiveness of DBCIs for smoking cessation and (i) readability (ii) ease-of-use, (iii) behaviour change techniques, and (iv) mode of delivery?
To what extent are these associations moderated by SEP?
Methods
This systematic review follows the Preferred Reporting Items for Systematic Reviews and Meta-Analysis (PRISMA) and Cochrane Collaboration guidelines (Hartmann-Boyce & Lindson, Citation2023; Higgins et al., Citation2022; Page et al., Citation2021). Certainty of evidence as classified using the GRADE approach (Guyatt et al., Citation2008).
Search strategy and selection criteria
The search strategy was developed in collaboration with a subject librarian. MEDLINE, PsycINFO, EMBASE, CINAHL, ASSIA, Web of Science Core Collection, the ACM Digital Library, the IEEE Digital Library, the Cochrane Central Register of Controlled Trials (CENTRAL), and ProQuest Dissertations were searched from 2004 to 21 February 2023, using keywords and database-specific subject headings related to the concepts of ‘digital interventions’ (e.g., cell phone* or cellular phone* or mobile phone* or mobile device* or smart device* or phone-based or phonebased or smartphone* or app or apps or telecommunication or telehealth or telemedicine or ehealth or e health or internet or online or on line or digital* or computer* or laptop* or iPad* or (tablet adj5 (internet or computer or digital or mobile or internet or electronic or cellular or web or online or on line or smart* or mobile*)) or m health or mhealth or u health or uhealth or email or e mail or electronic mail or text messag* or SMS or chat-based or text-based or multimedia messag* or MMS or social media), ‘smoking cessation’, (e.g., (quit* or stop* or give* or ceas* or cess*) adj3 (smok* or tobacco* or cigar* or pipe* or shisha* or hookah* or nargile* or narghile* or waterpipe*)) and ‘experimental evaluation’ (e.g., compare or compared or comparison or randomised controlled trial or controlled clinical trial or placebo or drug therapy or random* or groups or trial or experiment* or quasiexperiment* or (pilot adj2 stud*) or (feasib* adj2 stud*)). Equivalent terms were connected with the Boolean operator ‘or’ while those referring to different concepts were connected using the Boolean operator ‘and’. In databases where they were available, medical subject headings were used. Additionally, the dblp computer science library, the British Library e-theses online service (eThOS), and the National Institute for Health Research UK Be Part of Research (formerly Clinical Trials Gateway) were hand-searched in May and June 2023. Reference list searches of reviews on similar topics were undertaken in May and June 2023. The full search strategy used for each database is provided in section A of the supplementary files. The search strategy was limited to studies published since 2004; this is often selected as the year in which digital technology, and specifically the internet, shifted towards primarily interoperable, user-centred applications and services to promote sharing of media and information, collaboration, and social connectedness (Roser et al., Citation2015; Wilson et al., Citation2011). DBCIs from before this period are likely to be incommensurable with later ones and of limited relevance to the development of future DBCIs.
To be included in the review, studies needed to report experimental or quasi-experimental evaluations of individual-level smoking cessation interventions delivered primarily through digital technologies (West & Michie, Citation2016). Our definition of digital technologies included, but was not limited to, email, websites or web-based games, mobile or tablet applications, SMS text messages, MMS multimedia messages, social media, and wearables. Interventions that consisted of a real-time talk-based intervention delivered over the phone were not included. Only studies using abstinence from smoking combustible tobacco (such as cigarettes, cigars, pipes, waterpipes, and cigarillos) as an outcome were included. This means that studies measuring only smoking reduction or measuring abstinence from electronic nicotine delivery systems, non-combustible tobacco, or nicotine replacement therapy were not included. We did not apply any geographical limits on where the study could be conducted, but the intervention needed to be conducted in the English language. This was a pragmatic choice to allow us to code readability in a reliable and valid manner. No restrictions were placed on study populations; studies conducted with adults or adolescents, in a community or clinical context, and studies looking exclusively at specific subpopulations, such as pregnant people, people with chronic illnesses, and people in low SEPs, were all eligible for inclusion. No restrictions were applied on the control condition.
Only studies reporting a measure of SEP were included to allow us to analyse effectiveness and moderators of effectiveness by SEP. If studies did not report differential effects by SEP, authors were contacted up to three times to request the relevant data and materials at either the participant or summary level.
Measures
Intervention effectiveness, measured in terms of the odds that a participant in the intervention group would be abstinent from smoking compared with a participant in the control group, was the primary outcome. The odds ratios (ORs) and 95% confidence intervals (CIs) of abstinence outcomes were calculated using the data extracted from each study and any additional data provided by the authors. The most rigorous measure of abstinence (Piper et al., Citation2020; West et al., Citation2005) reported or made available by the study authors was extracted. This means that if a study, for example, reported both 30- and 7-day point-prevalence abstinence at 6 months, the 30-day measure was used.
Readability, ease-of-use, modes of delivery, and BCTs were used to characterise intervention content and delivery features. Study authors were contacted to request intervention materials in order to characterise these intervention features.
The readability of the intervention text was assessed using the Gunning-Fog-Index (GFI; Gunning, Citation1969), which is a reliable, standardised, and widely used measure of text complexity (Bothun et al., Citation2021; Grossman et al., Citation1994; Kue et al., Citation2021; Szmuda et al., Citation2020). Based on classifications of reading difficulty by the National Work Group on Literacy and Health and NHS guidelines for the readability of patient information, the scores were split into three categories (NHS Health Education England, Citationn.d.; Report of the National Work Group on Literacy and Health, Citation1998): ‘Easy’ (GFI of 6 or below); ‘Average’ (GFI above 6 and below or equal to 9); ‘Difficult’ (GFI of above 9); and not text-based/written. For the moderator analysis, the categories ‘Easy’ and ‘Average’ were collapsed into one because only one intervention was categorised as ‘Easy’.
Ease-of-use features were coded based on the ease-of-use taxonomy (Ubhi, Michie, et al., Citation2016). This ease-of-use taxonomy has been specifically developed for DBCIs and has been previously found to have a good to acceptable reliability (Ubhi, Kotz, et al., Citation2016; Ubhi, Michie, et al., Citation2016). We modified this taxonomy to exclude the readability item as it was assessed separately with the GFI. Therefore, overall ease-of-use was scored from 0 (none of the features are present) to 8 (all the features are present).
The mode of delivery was coded based on a recently developed and validated ontology that outlines various modes of delivery, including ones using digital technologies (Marques et al., Citation2020).
Intervention content was coded using BCTs from the BCTTv1 (Michie et al., Citation2013). The BCTTv1 contains 93 BCTs across 16 BCT domains that can be mapped on the COM-B (Capability, Opportunity, Motivation, Behaviour) and Theoretical Domains Framework (TDF) models of behaviour as well as the Behaviour Change Wheel (BCW) guide for intervention development (Atkins et al., Citation2017).
If a study included multiple control conditions, the most minimal control was included in the quantitative synthesis, in line with recommendations by the Cochrane Collaboration (Higgins et al., Citation2022). To determine the most minimal control condition, we, hierarchically, either picked the inactive control condition if one existed, followed the authors’ description in the original paper, or used our own judgment and knowledge of the research literature to determine which control condition was the least active (e.g., if a study compared a more active study app to both a less active app and print materials, we chose the print materials as the most minimal control).
Study quality and risk of bias were assessed using the current version of the Cochrane Risk of Bias Tool (Sterne et al., Citation2019).
Data extraction
The literature search results, deduplication, abstract screening, and full-text sifting of records were managed using Rayyan (Ouzzani et al., Citation2016; Rayyan – Intelligent Systematic Review – Rayyan). Abstract screening and full-text sifting were done by comparing study characteristics to tabulated inclusion and exclusion criteria. Interrater agreement was assessed using kappa coefficients as appropriate. The strength of agreement as indicated by kappa coefficients can be interpreted as follows: poor (<0.00), slight (0.00–0.20), fair (0.21–0.40), moderate (0.41–0.60), substantial (0.61–0.80), almost perfect (0.81–1.00) (Landis & Koch, Citation1977).
CL did the literature search, screened all abstracts and full texts, extracted the data, and assessed all studies for risk of bias. TO screened 20% of abstracts to assess the reliability of inclusion (PABAK: 0.783, 95% CI: 0.745–0.818). To assess reliability in the coding of intervention content and delivery features, TO also double-coded 20% of included studies for BCTs (PABAK: 0.778; 95% CI: 0.738–0.813), ease-of-use (PABAK: 0.432; 95% CI: 0.220–0.614), and mode of delivery (100% agreement). TO also assessed 20% of included studies for risk of bias (overall risk of bias Fleiss’ κ = 0.40, p = .003; risk of bias for individual questions Fleiss’ κ = 0.63, p < .001). All disagreements were resolved through discussion to reach a consensus.
Analyses
All studies were included in the analyses for which authors provided sufficient data and intervention materials to estimate differential effect sizes by SEP and code the relevant intervention features. Random-effects models were used because there was likely residual heterogeneity between intervention effect sizes that was not accounted for by the moderators investigated and the ‘true’ effect sizes of the populations were likely to be different (Borenstein et al., Citation2010). All analyses were performed in RStudio version 4.3.1. Conventional meta-analyses were performed using the ‘metafor’ package (Viechtbauer, Citation2010) and meta-CART analyses were conducted using the ‘metacart’ package (Li et al., Citation2017, Citation2019).
Meta-analysis
A conventional random-effects meta-analysis was performed on the overall effect sizes of all included studies to estimate the overall effectiveness of DBCIs for smoking cessation (K = 29). Additionally, subgroup random-effects meta-analyses using the separate effect sizes for high (K = 27) and low (K = 28) SEP were conducted. Subgroup analyses stratified by whether the control condition was active (K = 14) or inactive (K = 15) were performed. The meta-analyses were also repeated on an extended dataset including studies for which authors provided sufficient data to estimate differential effect size by SEP, but not sufficient intervention materials to allow for coding of relevant intervention features (K = 48).
Meta-CART
To explore moderators of effect size, meta-CART analyses were conducted. Meta-CART is a data-driven meta-analytic method to identify moderators of effect size (Li et al., Citation2017, Citation2019). As such, it has a higher power to detect main effects and interactions between multiple moderators of effect size than traditional methods such as multivariate meta-regression and subgroup meta-analysis (Li et al., Citation2017, Citation2019). In line with the pre-registered review protocol, the following moderators were added to meta-CART model: Mode of delivery (dichotomised into mobile-based and not-mobile-based); ease-of-use (dichotomised using a median split); readability (three categories: ‘not text-based/written’, ‘easy or average’ [GFI of 9 or below], and ‘difficult’ [GFI over 9]); SEP (dichotomised as high or low based on the measure used by the authors of the original study); BCTs (numerical variable coding whether each BCT was present in the intervention, but not control condition [1], present in both control and intervention or neither [0], or present in the control, but not intervention condition [−1]); individual BCTs were included as potential moderators if they were present in the intervention, but not control condition for at least four effect sizes (63/93 BCTs, see section B of the supplementary files). Two types of sensitivity analyses were performed in which BCTs were entered as binary categorical variables: one in which all observations which had a BCT that was present in the control, but not the intervention condition were dropped, and one where all −1 cases (present in control but not intervention) were recoded to 0 (absence in intervention). The meta-CART analysis used random-effects analysis, with the pruning parameter set to c = 1 (Li et al., Citation2019). The other parameters were kept at their default values (maximum number of splits = 5; minimum number of studies in parent node before splitting = 6; stopping rule for the decrease of between subgroups Q = 0.00001; minimum number of studies in a terminal node = 3, 10-fold cross-validation).
Sensitivity analyses
Exploratory random-effects meta-regressions were used to examine the robustness of the findings from the meta-CART analysis. For these exploratory meta-regressions, moderators were first entered into their own univariate meta-regressions that controlled for false discovery rate using the Benjamini–Hochberg correction (Benjamini & Hochberg, Citation1995). If multiple moderators were found to be significant in these corrected univariate meta-regressions, they were added to a multivariate meta-regression.
Sensitivity analyses excluding studies at high risk of bias, using self-reported abstinence as the outcome, with high (>50% (Hartmann-Boyce & Lindson, Citation2023)) dropout, and representing outliers in effect size were performed for both the conventional meta-analyses and the meta-CART analysis. To explore the robustness of the meta-CART algorithm, analyses were repeated with the pruning parameter set to c = 0.5 and c = 0.0 to allow for greater power (while controlling the Type 1 error less tightly) (Li et al., Citation2017, Citation2019). In further sensitivity analyses of the meta-CART analyses, BCT domains were used instead of BCTs and mode of delivery was left as a categorical non-dichotomised variable.
Pre-registration
The study protocol was pre-registered on OSF (https://osf.io/4cgdb/) and Prospero (ID: CRD42023398393) after conducting preliminary searches but before starting systematic literature searches.
Changes to the pre-registration
There was an error in the pre-registered protocol, which stated that readability would be categorised in the following way: Easy (Gunning-Fog-Index of 6 or below); Average (Gunning-Fog-Index of 9 or below); Difficult (Gunning-Fog-Index of 10 or above). This left a gap between the categorisations for average and difficult, and categorisation was corrected so that the category of difficult started from a Gunning-Fog-Index of above 9. A planned sensitivity analysis excluding studies that were quasi-randomised was not performed since there were no quasi-randomised studies. BCTs were added as numerical, rather than categorical, variables to also account for occasions where the BCTs were present in the control, but not in the intervention condition. However, since entering BCTs as quasi-continuous variables has the – likely not warranted – assumption that the presence of a BCT in the intervention and control is equivalent. It also does not test specifically whether using a certain BCT as part of an intervention is linked to the effectiveness of that intervention. Therefore, two types of sensitivity analyses in which BCTs were entered as binary variables were added as well: one in which all observations which had a BCT that was present in the control, but not the intervention condition were dropped, and one where all −1 cases were recoded to 0 s.
The following unplanned sensitivity analyses were added: Because the pre-planned meta-CART analysis did not find evidence of effect moderation, exploratory meta-regressions were used to examine the robustness of these findings. Conventional meta-analyses were also repeated on an extended dataset including studies for which authors provided sufficient data to estimate differential effect size by SEP, but not sufficient intervention materials to allow for coding of relevant intervention features.
Results
Search and sift
A total of 11,966 studies (5857 after exclusion of duplicates: 5927 duplicates automatically removed using Systematic Review Accelerator (Clark et al., Citation2020); 182 additional duplicates removed manually) were identified by the systematic searches of the above-mentioned databases, 751 full-text reports were retrieved and screened for eligibility (). Of these, 180 met the inclusion criteria. Additionally, 125 records were identified through hand-search, retrieved, and screened for eligibility. Of these, 17 met the inclusion criteria. This yielded an overall sample of 197 studies. For 29 of these studies, authors provided sufficient data and intervention materials to estimate differential effect size by SEP and allow for the coding of relevant intervention features. For an additional 19 studies, authors provided sufficient data to estimate differential effect size by SEP, but not sufficient intervention materials to allow for coding of relevant intervention features. A list of all studies eligible for inclusion can be found in section C of the supplementary files.
Descriptives
Details on study and intervention characteristics can be found in and , respectively. Details on the extended set of studies used in some of the sensitivity analyses can be found in section D of the supplementary files.
Table 1. Study characteristics of the 29 studies included in the main analysis.
Table 2. Intervention characteristics of the 29 studies included in the main analysis.
Study characteristics
A total of 29 studies providing K = 55 effect sizes split by SEP (27 for high SEP and 28 for low SEP) were included in the analyses. The studies were published between 2009 and 2023, with the majority (k = 19) being published in the last five years (since 2019).
All included studies were randomised trials. Sample sizes of included studies ranged from 28 to 28,112, with a mean of 1471 (SD = 5217) and a median of 209 participants. Across studies, 63.4% (SD = 21.6) of participants were female, with a minimum of 18.5% and a maximum of 100% female participants. The grand mean age of participants across the 27 studies that reported mean age was 36.2 (SD = 9.0). Across the 25 studies that reported mean cigarettes per day at baseline, the grand mean was 15.3 (SD = 4.9). Of the 29 included studies, 17 (58.6%) required participants to have some level of interest in quitting smoking as an inclusion criterion, while 2 (6.9%) required participants to be willing to receive cessation advice, and 5 (17.2%) recruited participants from a pool of users of an existing smoking cessation intervention; the remaining 5 studies (17.2%) did not have a motivation criterion. Follow-up durations raged from 4 to 40 weeks, with a mean of 17.6 (SD = 9.3) and a median of 21 weeks. Overall, 14 studies (48.3%) had a follow-up duration of at least 26 weeks (6 months). The outcome measure was self-reported abstinence for 13 studies (44.8%), while 14 studies (48.3%) used biochemically verified abstinence in line with the Russell Standard (West et al., Citation2005). Additionally, one study (3.5%) used biochemically verified abstinence but did not report the cut-offs used, and one (3.5%) used biochemically verified abstinence, but with substantially more lenient cut-offs than recommended in the Russell Standard. Point-prevalence abstinence was used in 15 studies (51.7%), while sustained abstinence 14 studies (48.3%). The control condition was active in 14 studies (48.3%) and inactive in the remaining 15 studies (51.7%). Active controls included reduced versions of the intervention, alternative interventions, and interventions offered through non-digital means.
Socioeconomic position
Measures of SEP were varied. The majority of studies (17; 58.6%) used education as an indicator. Income (personal or household) was used as an indicator of SEP in six studies (20.7%), while occupational social grade was used in four studies (13.8%). Homelessness and subjective financial status were used in one study (3.5%) each. Exact cut-offs for different categorisations also differed between studies, in part reflecting the different times and places in which the studies were conducted. The percentage of participants classified as low SEP in each study ranged from 1.4% to 100%, with a mean of 46.1% (SD = 23.8) and a median of 43.9%.
Study quality
A total of 13 studies (44.8%) were judged to be at low risk of bias, with 10 studies (34.5%) raising some concerns in terms of risk of bias, and 6 studies (20.7%) being judged to be at high risk of bias (). A table showing the risk of bias for individual studies and domains can be found in section E of the supplementary files. An overview of the risk of bias for all 48 studies included in the extended analysis can be found in section F of the supplementary files.
Figure 2. Risk of bias for the studies included in the main analyses. The domain ‘Timing of identification or recruitment of participants’ is only applicable to cluster randomised trials and therefore was relevant for only two of the included studies (as indicated by the grey colour for the remaining studies).
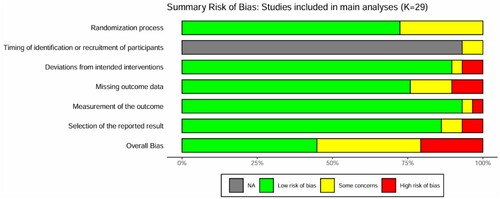
Intervention characteristics
Delivery features
Regarding readability, seven interventions (24.1%) were not in writing (i.e., they used videos or other visual or spoken modalities), so readability was not an applicable measure for them. For the remaining 22 interventions, the mean GFI was 8.06 (SD = 1.41; median = 8.02). In categorical terms, 17 interventions (58.6%) were classified as being of average readability, 4 (13.8%) as being difficult to read, and only one (3.5%) as being easy to read.
Ease-of-use was generally high with a mean score of 6.5 (SD = 1.2; median = 7; range: 4–8) out of 8. Seven interventions (24.1%) met the ease-of-use criteria across all ease-of-use dimensions. All interventions met the ease-of-use criteria in the dimension of Pattern Recognition. Across the remaining seven dimensions, most, but not all studies, met the criteria: Page Names (27; 93.1%), Text Formatting (26; 89.7%), Font Size (25; 86.2%), Clear and Consistent Language (22; 75.9%), Layout (21; 72.4%), Aesthetics (20; 69.0%), and Minimum Text (18; 62.1%).
Modes of delivery were varied. Overall, 14 (48.3%) interventions used more than one mode of delivery. The three most common modes of delivery were exclusively by mobile application (6; 20.7%), exclusively by text messaging (5; 17.2%), and by a combination of website and email (5; 17.2%). A total of 17 interventions (58.6%) were at least partly mobile-phone-based.
Intervention content
A list of the individual BCTs used in the intervention and control conditions of each study can be found in section G of the supplementary files. Interventions used a range of BCTs, although some were present in most interventions. The most common BCTs used in the intervention conditions were 1.1 Goal Setting (Behaviour) and 3.1 Social Support (Unspecified) (25; 86.2% each); 5.1 Information about Health Consequences and 11.2 Reduce Negative Emotions (22; 75.8% each); and 4.2 Information about Antecedents (21; 72.4%). The mean number of BCTs in intervention conditions was 26.0 (SD = 14.9, Median = 26, Range = 4–48). The most common BCTs used in the control conditions were similar: 1.1 Goal Setting (Behaviour) (18; 62.1%); 3.1 Social Support (Unspecified) (17; 58.6% each); 11.1 Pharmacological Support (15; 51.7%); 5.1 Information about Health Consequences (13; 44.8%) and 11.2 Reduce Negative Emotions (11; 37.9% each). The mean number of BCTs in control conditions was 10.2 (SD = 10.5, Median = 5, Range = 0–34). The most common BCTs appearing in interventions, but not controls were: 1.2 Problem Solving (15; 51.7%); 5.2 Salience of Consequences (14; 48.3%); 5.6 Information about Emotional Consequences, 6.2 Social Comparison, 9.1 Credible Source, and 13.2 Framing/Reframing (13; 44.8% each). The mean number of BCTs in the intervention, but not control condition was 16.4 (SD = 15.4, Median = 8, Range = −7 to 48). The negative integer indicates that there were more BCTs in the control than in the intervention condition. Histograms showing the distribution of number of BCTs across intervention and control conditions can be found in section H of the supplementary files.
Meta-analyses
A summary of the findings can be found in .
Table 3. Summary of findings table.
Main analyses
The main meta-analysis using only the 29 studies for which we have sufficient information to characterise the intervention in terms of content and delivery features indicates that overall, DCBIs significantly improved abstinence rates (OR = 1.29, 95% CI: 1.10–1.51, p = .002; I2 = 32.7%, Q(df = 28) = 45.54, p = .019; (A)). The main effect of the intervention remained consistent in sensitivity analyses excluding studies deemed at high risk of bias, excluding studies using self-reported abstinence as outcome measure, excluding studies representing outliers in effect size, and excluding studies with low follow-up rates.
When running separate subgroup meta-analyses by SEP, the effect sizes were similar for low (OR = 1.25, 95% CI: 1.00–1.57, p = .048; I2 = 22.3%, Q(df = 27) = 30.16, p = .307; (B)) and high (OR = 1.36, 95% CI: 1.06–1.76, p = .017; I2 = 52.5%, Q(df = 26) = 57.74, p < .001; (C)) SEP. The effect sizes were also similar for studies using an active control (OR = 1.30, 95% CI: 1.06–1.59, p = .013; I2 = 42.7%, Q(df = 13) = 23.91, p = .032) and inactive control (OR = 1.29, 95% CI: 0.98–1.69, p = .069; I2 = 21.1%, Q(df = 14) = 20.69, p = .110). Neither SEP (p = .659) nor activeness of control (p = .958) were significant moderators of effect size in meta-regressions, which is in line with the similarity in effect sizes seen in the subgroup meta-analyses.
Visual inspection of the funnel plot (section I in the supplementary files) and an Egger’s test (z = 0.903, p = .367) gave no indication of publication bias.
Extended set of studies
When repeating the meta-analyses on the extended set of studies that also includes the 19 studies for which we do not have sufficient materials to characterise the interventions in terms of content and delivery features, the results mirror those of the main analysis. Overall, DCBIs significantly improve quit rates (OR = 1.48, 95% CI: 1.30–1.68, p < .001; I2 = 46.4%, Q(df = 47) = 89.95, p < .001; (A)). This remained consistent in sensitivity analyses excluding studies deemed at high risk of bias, excluding studies using self-reported abstinence as outcome measure, excluding studies representing outliers in effect size, and excluding studies with low follow-up rates.
Figure 4. Forest plot showing the individual and pooled effect sizes of the k = 48 studies for which authors provided sufficient materials for inclusion in the meta-CART and meta-regressions: overall (A), among populations in low SEPs (B), among populations in high SEPs (C).
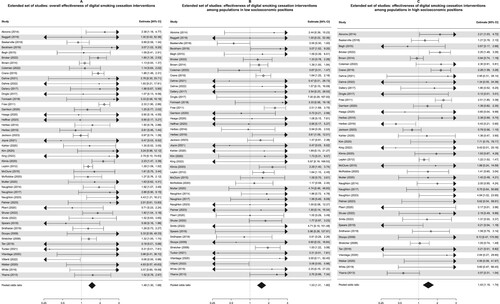
When running separate subgroup meta-analyses by SEP, the effect sizes were similar for low (OR = 1.53, 95% CI: 1.31–1.80, p < .001; I2 = 23.5%, Q(df = 45) = 48.58, p = .331; (B)) and high (OR = 1.43, 95% CI: 1.18–1.74, p < .001; I2 = 61.5%, Q(df = 40) = 100.00, p < .001; (C)) SEP. The effect sizes were somewhat greater for studies using an inactive (OR = 1.67, 95% CI: 1.34–2.09, p < .001; I2 = 39.8%, Q(df = 26) = 49.56, p = .004) compared to an active (OR = 1.36, 95% CI: 1.18–1.57, p < .001; I2 = 39.8%, Q(df = 20) = 30.89, p = .057). However, neither SEP (p = .614) nor activeness of control (p = .119) were significant moderators of effect size in meta-regressions, which is in line with the findings on the smaller set of studies and the overlapping confidence intervals in the subgroup meta-analyses.
Visual inspection of the funnel plot (section I in the supplementary files) and an Egger’s test (z = 0.321, p = .748) gave no indication of a publication bias.
Moderators of effectiveness using meta-CART
Despite substantial heterogeneity in effect sizes (Q(df = 54) = 88.81, p = .002, τ2 = 0.102), the main meta-CART analysis did not detect any moderation by SEP, intervention content, or delivery features. This remained consistent across the following sensitivity analyses: entering BCTs as binary categorical variables, using BCT domains instead of individual BCTs as moderators, leaving mode of delivery as a categorical non-dichotomised variable, excluding studies deemed to be at high risk of bias, excluding studies using self-reported abstinence as outcome measure, excluding studies representing outliers in effect size, and excluding studies with high attrition rates. The findings remained consistent when setting the pruning parameter to c = 0.5 and c = 0.0 to allow for greater power (while controlling the Type 1 error less tightly) and when running the tree separately by SEP. Details of the initial unpruned three and the cross-validation tables of the main meta-CART analysis and the separate trees by SEP can be found in section J of the supplementary files.
Exploratory meta-regressions
To explore the nature of the null effect found in the main meta-CART analysis, we ran a series of meta-regressions on the overall effect size, and the effect sizes stratified by SEP.
In univariate meta-regressions using a false discovery rate controlled significance level of p = .05, only two BCTs, ‘Commitment’ (b = 0.49, 95% CI: 0.27–0.71, p < .001) and ‘Credible Source’ (b = −0.38, 95% CI: −0.61 to −0.15, p = .001) were significantly associated with effectiveness. When entering both moderators into a multivariate meta-regression, the effect of ‘Commitment’ remained (b = 0.39, 95% CI: 0.09–0.69, p = .012), while the effect of ‘Credible Source’ was attenuated (b = −0.14, 95% CI: −0.43 to 0.15, p = .329).
Exploratory meta-regressions stratified by SEP revealed somewhat divergent results. When running univariate meta-regressions on low SEP effect sizes, no moderators were significant. The association between the BCT ‘Commitment’ and effectiveness is non-significant, but the point estimate is in the same direction (b = 0.11, 95% CI: −0.38 to 0.61, p = .656). Among high SEP groups, only the BCT ‘Commitment’ (b = 0.79, 95% CI: 0.35–1.24, p = .001) was associated with effectiveness. Multivariate meta-regressions were therefore not warranted in these stratified meta-regressions. Results from sensitivity analyses entering BCTs into the meta-regressions as binary variables largely mirrored those from the main analyses (see section K of the supplementary files).
Discussion
DBCIs for smoking cessation improve the odds of smoking abstinence and are equally effective across high and low SEP groups. Interventions tended to be easy to use and used various modes of delivery. However, text-based interventions were largely not easy to read. The DBCIs included in this review used a variety of BCTs, but certain BCTs, such as ‘Goal Setting (Behaviour)’ and ‘Social Support (Unspecified)’, were present in the vast majority of interventions. Delivery mode, ease-of-use, and readability were not significantly associated with intervention effectiveness. Meta-CART analyses did not detect any interactions between potential moderators of effectiveness. Exploratory meta-regression indicated that one BCT, ‘Commitment’ (i.e., asking the participant to (re)affirm pledges to quit smoking), was associated with larger effect sizes, both in general and among populations with high SEP, but not among populations with low SEP.
This review used broader inclusion criteria compared with previous reviews (but see Amiri & Khan, Citation2023) which have often focussed on particular types of digital interventions such as apps/text messaging (Kingkaew, Citation2018; Whittaker et al., Citation2019) or internet-based interventions alone (Graham et al., Citation2016; McCrabb et al., Citation2019; Taylor et al., Citation2017), or specific subpopulations such as pregnant people (Griffiths et al., Citation2018).
Our finding that, overall and in comparison to inactive controls, DBCIs are effective for increasing smoking cessation rates is in line with previous research (Amiri & Khan, Citation2023; Graham et al., Citation2016; Griffiths et al., Citation2018; Kingkaew, Citation2018; Liu et al., Citation2023; McCrabb et al., Citation2019; Whittaker et al., Citation2019). However, unlike some previous reviews, we also found evidence that DBCIs are effective when compared to active controls (Graham et al., Citation2016; Griffiths et al., Citation2018; Taylor et al., Citation2017; Whittaker et al., Citation2019).
Previous research on the equity impact of DBCIs for smoking cessation has been equivocal. Some previous research has indicated that quit success is lower among smokers in lower SEP (Kotz & West, Citation2009; Reid et al., Citation2010) and that smoking cessation interventions may be less effective for smokers in low SEPs (T. Brown et al., Citation2014; Hiscock et al., Citation2015). However, a previous observational population study has indicated that websites in particular may potentially be (more) effective for smoking cessation for people in low SEPs (Jackson et al., Citation2019). We found that DBCIs are effective for populations in both high and low SEPs and no indication for differences in effectiveness by SEP. Our review also extends the findings from two previous reviews. One previous review of individual-level behavioural smoking cessation interventions delivered through various means found that overall, these interventions are about equally effective for participants with high and low SEP (Kock et al., Citation2019). Another review found targeted technology-mediated interventions are effective for disadvantaged groups (Boland et al., Citation2018). Our review extends these findings to non-targeted DBCIs and by exploring whether SEP, intervention, or delivery features moderate the effectiveness of DBCIs. While our review finds no evidence of that DBCIs are less effective in helping smokers in low SEPs quit, they might still contribute to health inequalities if there are disparities in access to them. Although rates of smartphone ownership and home internet access are increasing, a digital divide still persists, with those who are younger, in higher SEPs, and residents in higher income countries having higher rates of internet access and smartphone ownership (Schumacher & Kent, Citation2020).
In moderator analyses, positive associations between BCT ‘Commitment’ and effectiveness were observed in the overall population and when restricting the analyses to high SEP populations. This is not in line with previous research, which has not shown a link between this BCT and effectiveness in DBCIs for smoking cessation but has found associations between other BCTs and effectiveness (Griffiths et al., Citation2018; Kingkaew, Citation2018; McCrabb et al., Citation2019). However, previous studies of this type have also been inconsistent with each other. A reason for this may be that the reviews have used different inclusion criteria for studies (in terms of intervention type, study type, population), potential moderators of effect size, and data extraction and meta-analytic methods. Interpretation of the finding that the BCT ‘Commitment’ significantly moderates effectiveness in the high SEP group only is complicated by the fact that the meta-CART analyses did not find evidence that SEP moderates the effectiveness of the BCT ‘Commitment’. Additionally, the confidence intervals of the moderation effect estimates of ‘Commitment’ in the meta-regressions using high and low SEP samples overlap, suggesting that while the effect of ‘Commitment’ is only statistically significant in the high SEP group, the difference between the effects in the high and low SEP groups may not in itself be significant (Gelman & Stern, Citation2006). However, the differential findings of its impact on effectiveness when running SEP-separated meta-regressions are nevertheless interesting. It suggests that it is possible that different intervention aspects may be differentially effective for different populations. It also provides further support for the idea that SEP shapes the kind of support people need or would benefit from most when it comes to smoking cessation. A potential reason why high SEP populations may particularly benefit from interventions utilising the BCT ‘Commitment’ is the socioeconomic pattering of smoking-related social norms and beliefs. The mechanism of action through which ‘Commitment’ is believed to aid behaviour change is by highlighting and linking the behaviour to certain principles and values that outline what is good, desirable, and important for someone and their relevant others (Carey et al., Citation2019). Previous research has highlighted that anti-smoking norms and high smoking risk perceptions are more prevalent in more socioeconomically advantaged communities (Hiscock et al., Citation2012; Huisman et al., Citation2012; van Wijk et al., Citation2019). Therefore, reaffirming one’s commitment not to smoke may be of greater relevance for people in high SEPs.
The finding that the ease-of-use of DBCIs for smoking cessation is high is in line with previous research on the ease-of-use features of apps (Ubhi, Kotz, et al., Citation2016). Furthermore, there is some overlap with the most common BCTs identified in this study and those identified in previous studies, such as the prevalence of the BCTs ‘Goal Setting (Behaviour)’, ‘Social Support (Unspecified)’, ‘Information about Antecedents’, and ‘Information about Health Consequences’ (Griffiths et al., Citation2018; Kingkaew, Citation2018; McCrabb et al., Citation2019). The finding that DBCIs for smoking cessation are overwhelmingly not easy to read is in line with previous research indicating low readability of smoking cessation apps and websites (Bock et al., Citation2004; Ferron et al., Citation2017). We are not aware of any previous research characterising DBCIs for smoking cessation in terms of how they map onto the mode of delivery ontology (Marques et al., Citation2020), which means that we cannot gauge to what extent the modes of delivery used in the interventions included in this review are reflective of any trends or changes over time.
The null finding of the current meta-CART analysis and the limited associations between intervention features and effectiveness might represent a genuine lack of a relationship, but it might also be the result of insufficient statistical power. While meta-CART analyses have greater statistical power than conventional multivariate meta-analyses, it is possible that k = 55 effect sizes are insufficient to find a genuine effect in our sample. A previous simulation study has shown that the recovery rate/power improves with more studies, if more/all moderators are binary, and that although a larger number of moderators do not drastically reduce the power, it is still somewhat reduced (Li et al., Citation2019). Another potential reason for the null finding might be that our measures of intervention features were insufficiently detailed. A major limitation of the BCTTv1 is that there is no way to control for the ‘dose’ of BCTs delivered. However, the intensity of a BCT is likely to differ within and between studies. Our coding of BCTs as −1 if present in control, but not intervention, 0 if present in both control and intervention or neither, and 1 if present in intervention, but not control and entering that into the analysis as a continuous/numeric variable implicitly assumes that BCTs are equivalent regardless of whether they occur in the control or intervention condition. However, this is not always the case. For many studies, even when there was a large overlap in the BCTs delivered in the intervention and control groups, the intervention group tended to receive a more intensive version of the BCT. For example, one study compared a personalised and tailored interactive website to a static control website (J. Brown et al., Citation2014). Both the intervention and the control website contained the BCT ‘Pharmacological Support’ (i.e., supplying or promoting use of nicotine replacement therapy or other smoking cessation medication). However, the intervention website contained much more extensive descriptions outlining the pros and cons of different forms of pharmacotherapy and more detailed descriptions of how to use them. In another study, which compared two apps, both the intervention and the control app contained the BCT ‘Distraction’ (i.e., recommend or provide distraction from potential behavioural cues) (Baskerville et al., Citation2018). However, the intervention app had an integration with social media and integrated interactive social distractions, while the control app merely advised using an alternative focus for attention.
Moreover, our approach to coding intervention features was unable to account for the way that participants actually engaged with the intervention. Previous studies investigating usage of DBCIs found that users of may pick and choose to use only specific features of interventions (Morrison et al., Citation2018). Participants in the reviewed studies might have been able to pick out content that they needed or was useful for them from the intervention even when the interventions were not highly useable or easy to read, or contained content that was inaccessible or irrelevant to them. This might also explain why the interventions appeared to be equally effective across socioeconomic groups despite their low readability. A third potential reason for the null findings could be a failure to account for confounding factors. There are likely to be remaining unmeasured confounds and intervention features that could not be fully accounted for by using random-effects models.
In addition to the above-mentioned issues, there are other limitations of this review. The measures used to operationalise readability and ease-of-use are limited. The ease-of-use taxonomy may apply unequally across intervention platforms and has so far only been validated for apps (Ubhi, Kotz, et al., Citation2016; Ubhi, Michie, et al., Citation2016). Additionally, judgements for dimensions such as aesthetics are likely influenced by the current aesthetic norms of digital media and platforms. Although the coders tried to account for this, they were probably unable to fully equivalise this measure. Additionally, some intervention features which might influence effectiveness such as personalisation were not measured. Furthermore, the commensurability between different measures of SEP or even the nominally same measure of SEP across different countries and times is likely to be limited. A further limitation of this review is its limited generalisability. Since, for pragmatic reasons, only studies evaluating English-language interventions were included, most studies included in this review were conducted in Western, predominantly English-speaking countries, and particularly the UK and the US. Therefore, these findings might not generalise to other populations. Additionally, only authors of 29 out of 197 studies (14.7%) provided sufficient data and intervention materials to estimate differential effect size by SEP and allow for the coding of relevant intervention features. While for 48 out of 197 studies (24.4%), authors provided sufficient data to estimate differential effect size by SEP. While this response rate was in line with our expectations, it is still fairly low. It likely also contributes to a selection bias because authors of older or lower quality studies may have been less likely to provide data and materials.
In terms of implications, this review provides further evidence that DBCIs are a promising modality for smoking cessation for people from across socioeconomic strata. The similarity in effectiveness by SEP means DBCIs for smoking cessation may be able to reduce health inequalities if they can be rolled out in an equitable manner. However, insight into the active ingredients of these interventions remains limited. Our evidence suggests that high SEP populations may particularly benefit from the inclusion of the BCT ‘Commitment’. This review does highlight that future research on DBCIs for smoking cessations might benefit from factorial designs to identify and establish causal links between intervention features and effectiveness more clearly. This may aid in our understanding of how and why DBCIs for smoking cessation work.
Conclusions
In conclusion, this review suggests that DBCIs for smoking cessation are effective across socioeconomic groups, although there is some indication based on exploratory analyses that specific BCTs may be more or less effective depending on the target population’s SEP. It also highlights that the currently available research literature offers only limited insights into the active ingredients of DBCIs for smoking cessation, how they interact with each other, and how they are moderated by the target population.
Supplemental Material
Download PDF (2.1 MB)Acknowledgements
We acknowledge the subject librarian Debora Marletta, for her support in developing the search strategy. CL, JB, CG, and OP conceptualised and designed the study, CL conducted the systematic literature search with the help of a subject librarian, and CL and TO performed the title and abstract screening, quality appraisal and data extraction. CL conducted data management, data analysis and synthesis of data. CL prepared the manuscript and all authors edited the manuscript, read, and approved the final version of the article.
Data availability statement
Data and syntax used for analysis are available on OSF (https://osf.io/4cgdb/) once the results are published.
Additional information
Funding
References
- Abernethy, A., Adams, L., Barrett, M., Bechtel, C., Brennan, P., Butte, A., Faulkner, J., Fontaine, E., Friedhoff, S., & Halamka, J. (2022). The promise of digital health: Then, now, and the future. NAM Perspectives.
- Abraham, C., Wood, C. E., Johnston, M., Francis, J., Hardeman, W., Richardson, M., & Michie, S. (2015). Reliability of identification of behavior change techniques in intervention descriptions. Annals of Behavioral Medicine, 49(6), 885–900. https://doi.org/10.1007/s12160-015-9727-y
- Amiri, S., & Khan, M. A. B. (2023). Digital interventions for smoking abstinence: A systematic review and meta-analysis of randomized control trials. Journal of Addictive Diseases, 41(1), 4–29. https://doi.org/10.1080/10550887.2022.2058300
- Atkins, L., Francis, J., Islam, R., O’Connor, D., Patey, A., Ivers, N., Foy, R., Duncan, E. M., Colquhoun, H., & Grimshaw, J. M. (2017). A guide to using the theoretical domains framework of behaviour change to investigate implementation problems. Implementation Science, 12(1), 1–18. https://doi.org/10.1186/s13012-016-0533-0
- Bartlett, Y. K., Sheeran, P., & Hawley, M. S. (2014). Effective behaviour change techniques in smoking cessation interventions for people with chronic obstructive pulmonary disease: A meta-analysis. British Journal of Health Psychology, 19(1), 181–203. https://doi.org/10.1111/bjhp.12071
- Baskerville, N. B., Struik, L. L., Guindon, G. E., Norman, C. D., Whittaker, R., Burns, C., Hammond, D., Dash, D., & Brown, K. S. (2018). Effect of a mobile phone intervention on quitting smoking in a young adult population of smokers: Randomized controlled trial. JMIR mHealth and uHealth, 6(10), e10893. https://doi.org/10.2196/10893
- Benjamini, Y., & Hochberg, Y. (1995). Controlling the false discovery rate: A practical and powerful approach to multiple testing. Journal of the Royal Statistical Society Series B: Statistical Methodology, 57(1), 289–300. https://doi.org/10.1111/j.2517-6161.1995.tb02031.x
- Black, N., Johnston, M., Michie, S., Hartmann-Boyce, J., West, R., Viechtbauer, W., Eisma, M. C., Scott, C., & de Bruin, M. (2020). Behaviour change techniques associated with smoking cessation in intervention and comparator groups of randomized controlled trials: A systematic review and meta-regression. Addiction, 115(11), 2008–2020. https://doi.org/10.1111/add.15056
- Bock, B. C., Graham, A. L., Sciamanna, C. N., Krishnamoorthy, J., Whiteley, J., Carmona-Barros, R., Niaura, R. S., & Abrams, D. B. (2004). Smoking cessation treatment on the Internet: Content, quality, and usability. Nicotine & Tobacco Research, 6(2), 207–219. https://doi.org/10.1080/14622200410001676332
- Boland, V. C., Stockings, E. A., Mattick, R. P., McRobbie, H., Brown, J., & Courtney, R. J. (2018). The methodological quality and effectiveness of technology-based smoking cessation interventions for disadvantaged groups: A systematic review and meta-analysis. Nicotine & Tobacco Research, 20(3), 276–285. https://doi.org/10.1093/ntr/ntw391
- Borenstein, M., Hedges, L. V., Higgins, J. P. T., & Rothstein, H. R. (2010). A basic introduction to fixed-effect and random-effects models for meta-analysis. Research Synthesis Methods, 1(2), 97–111. https://doi.org/10.1002/jrsm.12
- Bothun, L. S., Feeder, S. E., & Poland, G. A. (2021). Poor readability of COVID-19 vaccine information for the general public: A lost opportunity. medRxiv, 2021.06.11.21258778. https://doi.org/10.1101/2021.06.11.21258778
- Brown, J., Michie, S., Geraghty, A. W., Yardley, L., Gardner, B., Shahab, L., Stapleton, J. A., & West, R. (2014). Internet-based intervention for smoking cessation (StopAdvisor) in people with low and high socioeconomic status: A randomised controlled trial. The Lancet Respiratory Medicine, 2(12), 997–1006. https://doi.org/10.1016/S2213-2600(14)70195-X
- Brown, T., Platt, S., & Amos, A. (2014). Equity impact of European individual-level smoking cessation interventions to reduce smoking in adults: A systematic review. European Journal of Public Health, 24(4), 551–556. https://doi.org/10.1093/eurpub/cku065
- Carey, R. N., Connell, L. E., Johnston, M., Rothman, A. J., De Bruin, M., Kelly, M. P., & Michie, S. (2019). Behavior change techniques and their mechanisms of action: A synthesis of links described in published intervention literature. Annals of Behavioral Medicine, 53(8), 693–707.
- Clark, J., Glasziou, P., Del Mar, C., Bannach-Brown, A., Stehlik, P., & Scott, A. M. (2020). A full systematic review was completed in 2 weeks using automation tools: A case study. Journal of Clinical Epidemiology, 121, 81–90. https://doi.org/10.1016/j.jclinepi.2020.01.008
- Coleman, T., Clark, M., Welch, C., Whitemore, R., Leonardi-Bee, J., Cooper, S., Hewitt, C., Jones, M., Sutton, S., Watson, J., Daykin, K., Ussher, M., Parrott, S., & Naughton, F. (2022). Effectiveness of offering tailored text message, self-help smoking cessation support to pregnant women who want information on stopping smoking: MiQuit3 randomised controlled trial and meta-analysis. Addiction, 117(4), 1079–1094. https://doi.org/10.1111/add.15715
- Department of Health and Social Care. (2017). Towards a smokefree generation: A tobacco control plan for England. HM Government. https://www.gov.uk/government/publications/towards-a-smoke-free-generation-tobacco-control-plan-for-england
- Department of Health and Social Care. (2023). Stopping the start: Our new plan to create a smokefree generation. HM Government. https://www.gov.uk/government/publications/stopping-the-start-our-new-plan-to-create-a-smokefree-generation/stopping-the-start-our-new-plan-to-create-a-smokefree-generation
- de Ruijter, D., Mergelsberg, E., Crone, M., Smit, E., & Hoving, C. (2022). Identifying active ingredients, working mechanisms, and fidelity characteristics reported in smoking cessation interventions in Dutch primary care: A systematic review. Nicotine & Tobacco Research, 24(5), 654–662. https://doi.org/10.1093/ntr/ntab236
- Estrela, M., Semedo, G., Roque, F., Ferreira, P. L., & Herdeiro, M. T. (2023). Sociodemographic determinants of digital health literacy: A systematic review and meta-analysis. International Journal of Medical Informatics, 177, 105124. https://doi.org/10.1016/j.ijmedinf.2023.105124
- Ferron, J. C., Brunette, M. F., Geiger, P., Marsch, L. A., Adachi-Mejia, A. M., & Bartels, S. J. (2017). Mobile phone apps for smoking cessation: Quality and usability among smokers with psychosis. JMIR Human Factors, 4(1), e7. https://doi.org/10.2196/humanfactors.5933
- Gelman, A., & Stern, H. (2006). The difference between “significant” and “not significant” is not itself statistically significant. The American Statistician, 60(4), 328–331. https://doi.org/10.1198/000313006X152649
- Graham, A. L., Carpenter, K. M., Cha, S., Cole, S., Jacobs, M. A., Raskob, M., & Cole-Lewis, H. (2016). Systematic review and meta-analysis of Internet interventions for smoking cessation among adults. Substance Abuse and Rehabilitation, 7, 55–69. https://doi.org/10.2147/SAR.S101660
- Griffiths, S. E., Parsons, J., Naughton, F., Fulton, E. A., Tombor, I., & Brown, K. E. (2018). Are digital interventions for smoking cessation in pregnancy effective? A systematic review and meta-analysis. Health Psychology Review, 12(4), 333–356. https://doi.org/10.1080/17437199.2018.1488602
- Grossman, S. A., Piantadosi, S., & Covahey, C. (1994). Are informed consent forms that describe clinical oncology research protocols readable by most patients and their families? Journal of Clinical Oncology, 12(10), 2211–2215. https://doi.org/10.1200/JCO.1994.12.10.2211
- Gunning, R. (1969). The fog index after twenty years. Journal of Business Communication, 6(2), 3–13. https://doi.org/10.1177/002194366900600202
- Guyatt, G. H., Oxman, A. D., Vist, G. E., Kunz, R., Falck-Ytter, Y., Alonso-Coello, P., & Schünemann, H. J. (2008). GRADE: An emerging consensus on rating quality of evidence and strength of recommendations. Bmj, 336(7650), 924–926. https://doi.org/10.1136/bmj.39489.470347.AD
- Hartmann-Boyce, J., & Lindson, N. (2023). Assessing and minimizing risk of bias in randomized controlled trials of tobacco cessation interventions: Guidance from the Cochrane Tobacco Addiction Group. Addiction, 118(9), 1811–1816. https://doi.org/10.1111/add.16220
- Higgins, J. P. T., Thomas, J., Chandler, J., Cumpston, M., Li, T., Page, M. J., & Welch, V. A. (2022). Cochrane handbook for systematic reviews of interventions (6.3). Cochrane. www.training.cochrane.org/handbook
- Hill, S., Amos, A., Clifford, D., & Platt, S. (2014). Impact of tobacco control interventions on socioeconomic inequalities in smoking: Review of the evidence. Tobacco Control, 23(e2), e89. https://doi.org/10.1136/tobaccocontrol-2013-051110
- Hiscock, R., Bauld, L., Amos, A., Fidler, J. A., & Munafò, M. (2012). Socioeconomic status and smoking: A review. Annals of the New York Academy of Sciences, 1248(1), 107–123. https://doi.org/10.1111/j.1749-6632.2011.06202.x
- Hiscock, R., Dobbie, F., & Bauld, L. (2015). Smoking cessation and socioeconomic status: An update of existing evidence from a national evaluation of English stop smoking services. BioMed Research International, 2015(1), 274056. https://doi.org/10.1155/2015/274056
- Hopkinson, N. S. (2020). The path to a smoke-free England by 2030. BMJ, 368, m518. https://doi.org/10.1136/bmj.m518
- Huisman, M., Van Lenthe, F. J., Giskes, K., Kamphuis, C. B., Brug, J., & Mackenbach, J. P. (2012). Explaining socio-economic inequalities in daily smoking: A social–ecological approach. European Journal of Public Health, 22(2), 238–243. https://doi.org/10.1093/eurpub/ckr039
- Jackson, S. E., Kotz, D., West, R., & Brown, J. (2019). Moderators of real-world effectiveness of smoking cessation aids: A population study. Addiction, 114(9), 1627–1638. https://doi.org/10.1111/add.14656
- Kale, D., Gilbert, H., & Sutton, S. (2019). An exploration of the barriers to attendance at the English stop smoking services. Addictive Behaviors Reports, 9, 100141. https://doi.org/10.1016/j.abrep.2018.10.005
- Kingkaew, P. (2018). Optimising the development of effective mobile health behaviour change interventions: Text messages to support smoking cessation in Thailand.
- Kock, L., Brown, J., Hiscock, R., Tartan-Birch, H., Smith, C., & Shahab, L. (2019). Individual-level behavioural smoking cessation interventions tailored for disadvantaged socioeconomic position: A systematic review and meta-regression. The Lancet Public Health, 4(12), E628–E644. https://doi.org/10.1016/S2468-2667(19)30220-8
- Kotz, D., & West, R. (2009). Explaining the social gradient in smoking cessation: It’s not in the trying, but in the succeeding. Tobacco Control, 18(1), 43–46. https://doi.org/10.1136/tc.2008.025981
- Kue, J., Klemanski, D. L., & Browning, K. K. (2021). Evaluating readability scores of treatment summaries and cancer survivorship care plans. JCO Oncology Practice, 17(10), 615–621. https://doi.org/10.1200/OP.20.00789
- Kwah, K. L., Fulton, E. A., & Brown, K. E. (2019). Accessing National Health Service stop smoking services in the UK: A COM-B analysis of barriers and facilitators perceived by smokers, ex-smokers and stop smoking advisors. Public Health, 171, 123–130. https://doi.org/10.1016/j.puhe.2019.03.012
- Landis, J. R., & Koch, G. G. (1977). The measurement of observer agreement for categorical data. Biometrics, 33(1), 159–174. https://doi.org/10.2307/2529310
- Lehto, T., & Oinas-Kukkonen, H. (2011). Persuasive features in web-based alcohol and smoking interventions: A systematic review of the literature. Journal of Medical Internet Research, 13(3), e46. https://doi.org/10.2196/jmir.1559
- Lewer, D., Jayatunga, W., Aldridge, R. W., Edge, C., Marmot, M., Story, A., & Hayward, A. (2020). Premature mortality attributable to socioeconomic inequality in England between 2003 and 2018: An observational study. The Lancet Public Health, 5(1), e33–e41. https://doi.org/10.1016/S2468-2667(19)30219-1
- Li, X., Dusseldorp, E., & Meulman, J. J. (2017). Meta-CART: A tool to identify interactions between moderators in meta-analysis. British Journal of Mathematical and Statistical Psychology, 70(1), 118–136. https://doi.org/10.1111/bmsp.12088
- Li, X., Dusseldorp, E., & Meulman, J. J. (2019). A flexible approach to identify interaction effects between moderators in meta-analysis. Research Synthesis Methods, 10(1), 134–152. https://doi.org/10.1002/jrsm.1334
- Liu, L., Zhao, Y., Li, J., Zhang, N., Lan, Z., & Liu, X. (2023). Efficacy of digital therapeutics in smoking cessation: A systematic review and meta-analysis. Medicine in Novel Technology and Devices, 17, 100209. https://doi.org/10.1016/j.medntd.2023.100209
- Marques, M. M., Carey, R. N., Norris, E., Evans, F., Finnerty, A. N., Hastings, J., Jenkins, E., Johnston, M., West, R., & Michie, S. (2020). Delivering behaviour change interventions: Development of a mode of delivery ontology. Wellcome Open Research, 5(125), 1–27. https://doi.org/10.12688/wellcomeopenres.15906.1
- Marteau, T. M., Rutter, H., & Marmot, M. (2021). Changing behaviour: An essential component of tackling health inequalities. Bmj, 372(8282).n332. https://doi.org/10.1136/bmj.n332
- McCrabb, S., Baker, A. L., Attia, J., Skelton, E., Twyman, L., Palazzi, K., McCarter, K., Ku, D., & Bonevski, B. (2019). Internet-based programs incorporating behavior change techniques are associated with increased smoking cessation in the general population: A systematic review and meta-analysis. Annals of Behavioral Medicine, 53(2), 180–195. https://doi.org/10.1093/abm/kay026
- Michie, S., Richardson, M., Johnston, M., Abraham, C., Francis, J., Hardeman, W., Eccles, M. P., Cane, J., & Wood, C. E. (2013). The behavior change technique taxonomy (v1) of 93 hierarchically clustered techniques: Building an international consensus for the reporting of behavior change interventions. Annals of Behavioral Medicine, 46(1), 81–95. https://doi.org/10.1007/s12160-013-9486-6
- Michie, S., West, R., Finnerty, A. N., Norris, E., Wright, A. J., Marques, M. M., Johnston, M., Kelly, M. P., Thomas, J., & Hastings, J. (2020). Representation of behaviour change interventions and their evaluation: Development of the upper level of the behaviour change intervention ontology. Wellcome Open Research, 5(123), 1–30.
- Morrison, L. G., Geraghty, A. W., Lloyd, S., Goodman, N., Michaelides, D. T., Hargood, C., Weal, M., & Yardley, L. (2018). Comparing usage of a web and app stress management intervention: An observational study. Internet Interventions, 12, 74–82. https://doi.org/10.1016/j.invent.2018.03.006
- NHS. (2019). The NHS long term plan. https://www.longtermplan.nhs.uk/
- NHS Digital. (2020). Statistics on smoking, England 2020. https://digital.nhs.uk/data-and-information/publications/statistical/statistics-on-smoking/statistics-on-smoking-england-2020
- NHS Health Education England. (n.d.). Training and educational resources. Educating and Training the Workforce. Retrieved January 30, 2023, from https://www.hee.nhs.uk/our-work/population-health/training-educational-resources
- Office for National Statistics. (2022). Adult smoking habits in the UK: 2021. https://www.ons.gov.uk/peoplepopulationandcommunity/healthandsocialcare/healthandlifeexpectancies/bulletins/adultsmokinghabitsingreatbritain/2021#smoking-prevalence-based-on-data-from-the-annual-population-survey-aps
- Ogden, J. (2016). Celebrating variability and a call to limit systematisation: The example of the behaviour change technique taxonomy and the behaviour change wheel. Health Psychology Review, 10(3), 245–250. https://doi.org/10.1080/17437199.2016.1190291
- Oinas-Kukkonen, H., & Harjumaa, M. (2009). Persuasive systems design: Key issues, process model, and system features. Communications of the Association for Information Systems, 24(28), 485–500.
- Ouzzani, M., Hammady, H., Fedorowicz, Z., & Elmagarmid, A. (2016). Rayyan—A web and mobile app for systematic reviews. Systematic Reviews, 5(1), 1–10. https://doi.org/10.1186/s13643-016-0384-4
- Page, M. J., McKenzie, J. E., Bossuyt, P. M., Boutron, I., Hoffmann, T. C., Mulrow, C. D., Shamseer, L., Tetzlaff, J. M., Akl, E. A., Brennan, S. E., Chou, R., Glanville, J., Grimshaw, J. M., Hróbjartsson, A., Lalu, M. M., Li, T., Loder, E. W., Mayo-Wilson, E., McDonald, S., … Moher, D. (2021). The PRISMA 2020 statement: An updated guideline for reporting systematic reviews. International Journal of Surgery, 88, 105906. https://doi.org/10.1016/j.ijsu.2021.105906
- Piper, M. E., Bullen, C., Krishnan-Sarin, S., Rigotti, N. A., Steinberg, M. L., Streck, J. M., & Joseph, A. M. (2020). Defining and measuring abstinence in clinical trials of smoking cessation interventions: An updated review. Nicotine & Tobacco Research, 22(7), 1098–1106. https://doi.org/10.1093/ntr/ntz110
- Reid, J. L., Hammond, D., Boudreau, C., Fong, G. T., Siahpush, M., & on behalf of the ITC Collaboration. (2010). Socioeconomic disparities in quit intentions, quit attempts, and smoking abstinence among smokers in four western countries: Findings from the international tobacco control four country survey. Nicotine & Tobacco Research, 12(suppl_1), S20–S33. https://doi.org/10.1093/ntr/ntq051
- Report of the National Work Group on Literacy and Health. (1998). Communicating with patients who have limited literacy skills. The Journal of Family Practice, 46(2), 168–176.
- Roser, M., Ritchie, H., & Ortiz-Ospina, E. (2015). Internet. Our World in Data. https://ourworldindata.org/internet
- Sama, P. R., Eapen, Z. J., Weinfurt, K. P., Shah, B. R., & Schulman, K. A. (2014). An evaluation of mobile health application tools. JMIR mHealth and uHealth, 2(2), e19. https://doi.org/10.2196/mhealth.3088
- Schumacher, S., & Kent, N. (2020). 8 charts on internet use around the world as countries grapple with COVID-19. Pew Research Center. https://www.pewresearch.org/short-reads/2020/04/02/8-charts-on-internet-use-around-the-world-as-countries-grapple-with-covid-19/
- Siddiqi, K., & Dogar, O. (2014). Can the internet help economically disadvantaged smokers? The Lancet Respiratory Medicine, 2(12), 947–948. https://doi.org/10.1016/S2213-2600(14)70214-0
- Smith, C. E., Hill, S. E., & Amos, A. (2021). Impact of population tobacco control interventions on socioeconomic inequalities in smoking: A systematic review and appraisal of future research directions. Tobacco Control, 30(e2), e87. https://doi.org/10.1136/tobaccocontrol-2020-055874
- Sterne, J. A. C., Savović, J., Page, M. J., Elbers, R. G., Blencowe, N. S., Boutron, I., Cates, C. J., Cheng, H.-Y., Corbett, M. S., Eldridge, S. M., Emberson, J. R., Hernán, M. A., Hopewell, S., Hróbjartsson, A., Junqueira, D. R., Jüni, P., Kirkham, J. J., Lasserson, T., Li, T., … Higgins, J. P. T. (2019). Rob 2: A revised tool for assessing risk of bias in randomised trials. BMJ, 366, l4898. https://doi.org/10.1136/bmj.l4898
- Szmuda, T., Özdemir, C., Ali, S., Singh, A., Syed, M. T., & Słoniewski, P. (2020). Readability of online patient education material for the novel coronavirus disease (COVID-19): A cross-sectional health literacy study. Public Health, 185, 21–25. https://doi.org/10.1016/j.puhe.2020.05.041
- Taylor, G., Dalili, M., Semwal, M., Civljak, M., Sheikh, A., & Car, J. (2017). Internet-based interventions for smoking cessation. Cochrane Database of Systematic Reviews, 2017(9), 1465–1858. https://doi.org/10.1002/14651858.CD007078.pub5
- Tofighi, B., Chemi, C., Ruiz-Valcarcel, J., Hein, P., & Hu, L. (2019). Smartphone apps targeting alcohol and illicit substance use: Systematic search in in commercial app stores and critical content analysis. JMIR mHealth and uHealth, 7(4), e11831. https://doi.org/10.2196/11831
- Twyman, L., Bonevski, B., Paul, C., & Bryant, J. (2014). Perceived barriers to smoking cessation in selected vulnerable groups: A systematic review of the qualitative and quantitative literature. BMJ Open, 4(12), e006414. https://doi.org/10.1136/bmjopen-2014-006414
- Ubhi, H. K., Kotz, D., Michie, S., van Schayck, O. C. P., Sheard, D., Selladurai, A., & West, R. (2016). Comparative analysis of smoking cessation smartphone applications available in 2012 versus 2014. Addictive Behaviors, 58, 175–181. https://doi.org/10.1016/j.addbeh.2016.02.026
- Ubhi, H. K., Michie, S., Kotz, D., van Schayck, O. C. P., Selladurai, A., & West, R. (2016). Characterising smoking cessation smartphone applications in terms of behaviour change techniques, engagement and ease-of-use features. Translational Behavioral Medicine, 6(3), 410–417. https://doi.org/10.1007/s13142-015-0352-x
- van Wijk, E. C., Landais, L. L., & Harting, J. (2019). Understanding the multitude of barriers that prevent smokers in lower socioeconomic groups from accessing smoking cessation support: A literature review. Preventive Medicine, 123, 143–151. https://doi.org/10.1016/j.ypmed.2019.03.029
- Viechtbauer, W. (2010). Conducting meta-analyses in R with the metafor package. Journal of Statistical Software, 36(3), 1–48. https://doi.org/10.18637/jss.v036.i03
- West, R., Hajek, P., Stead, L., & Stapleton, J. (2005). Outcome criteria in smoking cessation trials: Proposal for a common standard. Addiction, 100(3), 299–303. https://doi.org/10.1111/j.1360-0443.2004.00995.x
- West, R., & Michie, S. (2016). Guide to development and evaluation of digital behaviour change interventions in healthcare. Silverback.
- Whittaker, R., McRobbie, H., Bullen, C., Rodgers, A., Gu, Y., & Dobson, R. (2019). Mobile phone text messaging and app-based interventions for smoking cessation. Cochrane Database of Systematic Reviews, 2019(10), 1465–1858. https://doi.org/10.1002/14651858.CD006611.pub5
- Wilson, D. W., Lin, X., Longstreet, P., & Sarker, S. (2011). Web 2.0: A definition, literature review, and directions for future research.
- Yang, Q. (2021). Theory-based social and non-social engagement features in smoking cessation mobile apps: A content analysis. International Journal of Environmental Research and Public Health, 18(17). 9106. https://doi.org/10.3390/ijerph18179106