ABSTRACT
3D printing has become highly applicable in modern life recently. The industry has brought a facelift to most others. However, this technology still exists some shortcomings, and it therefore has not been generalised to bring the best benefits to users. In this paper, based on multilayer perceptron and convolution neural network models, we propose a new data-driven machine learning platform for predicting optimised parameters of the 3D printing process from a model design to a complete product. This finding can open up great advances in the current 3D printing technology. Accordingly, the results obtained allow us to predict quickly and accurately some decisive parameters of the traditional 3D printing process such as time, weight and length while the input was fuzzy with a part of the initial information missing. The proposed approach does not need to account for the shape, size and material of the printed object, but it can perform the process automatically without other extra factors. After completing the model, a configurator is proposed to set the parameters for the respective printer types, which makes the 3D printing process simple and fast.
Disclosure statement
No potential conflict of interest was reported by the author(s).
Additional information
Funding
Notes on contributors
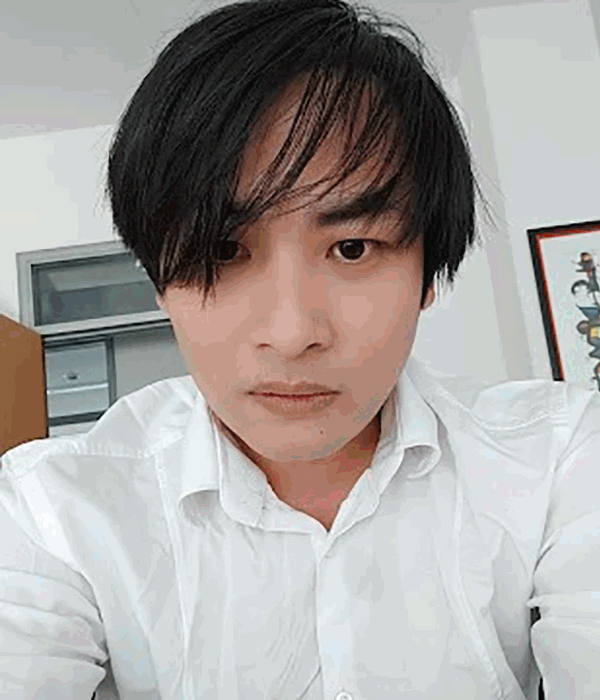
Phuong Dong Nguyen
Phuong Dong Nguyen was born in 1987 in Nha Trang, Khanh Hoa, Vietnam. Graduated Master of Computer Science in 2016 from Ho Chi Minh City University of Technology, Vietnam. The main research direction is machine learning, deep learning, optimization algorithms, processing special data types.
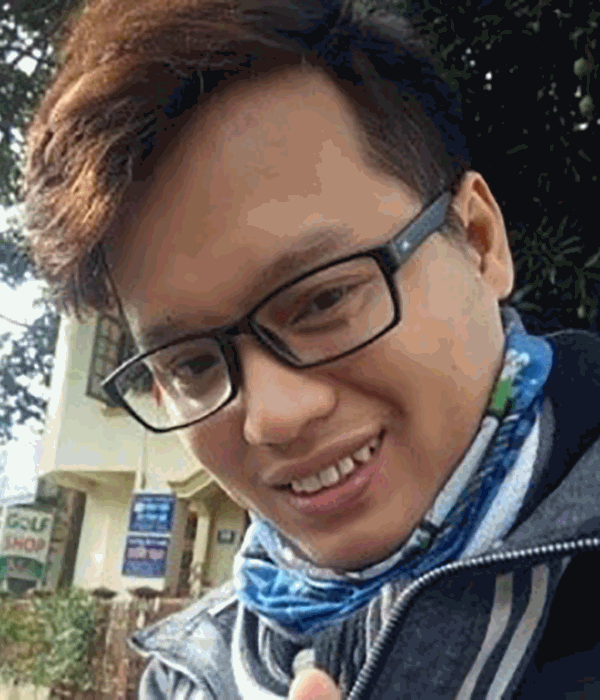

Thanh Q. Nguyen
Thanh Q. Nguyen was born in 1988. He received a master degree in Engineering Mechanics in 2011 from the Ho Chi Minh University of Technology, Ho Chi Minh City, Vietnam. The main research direction is defect recognition through the identification and processing of random measurement signals to serve the assessment of bridge condition, dynamics and control.
Dr. Q. B. Tao has been working as a lecturer at the Faculty of Mechanical Engineering, University of Science and Technology, The University of Danang, Vietnam since 2006. He received his Ph.D in Automation from Paris-Saclay University (France) in 2016. He has published more than 20 articles indexed in WoS-ISI. His research interests focus on computational methods, mechanics of materials, fracture mechanics, advanced materials, computational fracture & damage mechanics.
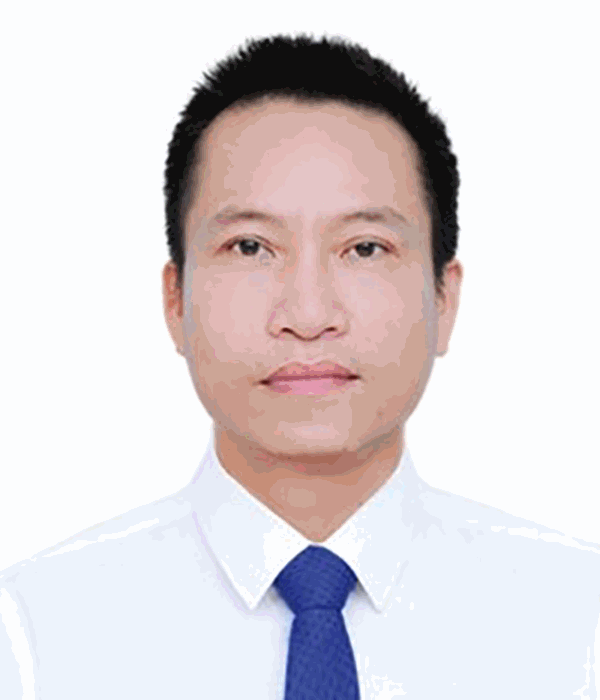
Frank Vogel
Frank Vogel is founder and General Manager of inuTech GmbH. Mr Vogel received his Diploma in Mathematics from University of Bayreuth (Germany) in 1993 followed by a R&D position at ANSYS, Inc. in USA before forming his self-employment in 1995 and inuTech GmbH (together with CADFEM International AG and Prof. Klaus Schittkowski) in 2000. During the years of his self-employment and at inuTech he has initiated, led, and executed R&D and software development projects for major enterprises (i.e. ANSYS, SIEMENS, Tadano, Fischerwerke, Voith Hydro, DANA, AIRBUS, AREVA NP, LMS, Daimler, Bosch, Volkswagen, CADFEM, Meyer Werft, BMW, ThyssenKrupp Stahl, Schaeffler, etc.) in areas such as Finite Element Analysis, mathematical modelling and numerical solution of PDEs, nonlinear optimization and control and professional software development project. inuTech currently has 25 innovative and highly qualified employees, possessing many years of research and development experience in the area of mathematical and numerical modelling with partial differential equations (PDEs), optimization, optimal control, etc. …, serving more than 300 customers worldwide.
Prof. H. Nguyen-Xuan is the Director of CIRTech Institute, HUTECH University, Vietnam. He is currently an adjunct professor at China Medical University (Taiwan), and a visiting professor at Sejong University (South Korea). Dr. Nguyen-Xuan received his Ph.D. in Computational Mechanics from the University of Liège (Belgium) in 2008. His researches focus on computational mathematics and engineering (CSE) for advanced development of robust computational methods with high performance computing in combination with machine learning, material and structural modeling and 3D printing. He has published more than 300 peer-reviewed papers (more than 250 articles indexed in WoS-ISI). These papers remarkable made him well-known as a Highly Cited Researcher (rank in the top 1% by citations) in seven continuous years from 2014 to 2021 in Computer Science. Dr. Nguyen-Xuan was the winner of Georg Forster Research – Alexander von Humboldt award (2016), Outstanding Humboldtian Award (2019), Alexander von Humboldt Renewed Research Stay Award (2019), the Alexander von Humboldt Foundation Digital Cooperation Fellowship (2021), excellent Vietnam National University HCMC researchers award in six continuous years from 2008 to 2013, and Nguyen Van Dao's award from association of Mechanics of Vietnam in 2011.