ABSTRACT
Introduction
Drug-induced liver injury (DILI) is a potentially lethal condition that heavily impacts the pharmaceutical industry, causing approximately 21% of drug withdrawals and 13% of clinical trial failures. Recent evidence suggests that the use of Liver-Chip technology in preclinical safety testing may significantly reduce DILI-related clinical trial failures and withdrawals. However, drug developers and regulators would benefit from guidance on the integration of Liver-Chip data into decision-making processes to facilitate the technology’s adoption.
Areas covered
This perspective builds on the findings of the performance assessment of the Emulate Liver-Chip in the context of DILI prediction and introduces two new decision-support frameworks: the first uses the Liver-Chip’s quantitative output to elucidate DILI severity and enable more nuanced risk analysis; the second integrates Liver-Chip data with standard animal testing results to help assess whether to progress a candidate drug into clinical trials.
Expert opinion
There is now strong evidence that Liver-Chip technology could significantly reduce the incidence of DILI in drug development. As this is a patient safety issue, it is imperative that developers and regulators explore the incorporation of the technology. The frameworks presented enable the integration of the Liver-Chip into various stages of preclinical development in support of safety assessment.
1. Introduction
Despite rigorous and often multifaceted preclinical safety testing, drug-induced liver injury (DILI) remains a significant concern during clinical trials and post-marketing surveillance [Citation1–3]. As the primary organ responsible for concentrating and metabolizing xenobiotics, the liver is particularly vulnerable to injury from compounds across nearly every class of drug [Citation4]. Symptoms of DILI may range from subtle elevations in liver enzymes to more acute liver failure and death, with clinical manifestations including cholestasis, steatosis, immune cell recruitment, or fibrosis. DILI can be sufficient to halt clinical trials, necessitate black box warning labels, or prompt drug withdrawal [Citation5].
In serving their responsibility to protect patients, drug developers and regulators require robust and high-quality data demonstrating the safety of new drugs prior to approving their use in clinical trials. This necessarily means evaluating the candidate’s potential to cause harm, including DILI. As such, pharmaceutical developers invest considerable effort in preclinical assessments of DILI risk among candidate drugs.
Up until December 2022 [Citation6] regulators in the United States and much of the world have predominantly demanded the use of two non-human animal species in preclinical safety testing [Citation7,Citation8]. As a result, evidence from animal models such as rodents, dogs, and primates are often heavily weighted when determining a candidate drug’s safety. However, animal-to-human species differences [Citation9] can diminish the predictive validity – a quantitative measure of a decision tool’s performance [Citation10]—of these models and enable prohibitively toxic drugs to progress into clinical trials [Citation11]. This is most evident in clinical trial failure rates, wherein 30% of failed trials are attributed to toxicity, approximately 13% of which cite DILI as a primary driving factor [Citation12]. The high clinical trial failure rate, along with several other lines of evidence, indicates that animal models alone are insufficient to adequately assess the DILI risk of new drug candidates [Citation13–17].
Over the past decade, researchers have shown that Organ-on-a-Chip technology provides human-relevant data and recapitulates human biology [Citation18–22]. Organ-Chips enable in vitro modeling of human organ biology by replicating key attributes of cellular microenvironments, such as three-dimensional (3D) cytoarchitectures, tissue-tissue interfaces, vascular perfusion and fluid flow, immune environment, and other relevant features. Researchers have accurately replicated complex functions of several organ types in Organ-Chips, supporting the use of this technology as a decision-making tool in preclinical drug development [Citation23,Citation24].
Ewart et al. recently evaluated the ability of the Emulate Liver-Chip – an Organ-Chip modeled after the human liver sinusoid – to predict DILI risk [Citation25]. Following guidelines [Citation26,Citation27] formulated by the Innovation and Quality (IQ) Consortium – a collaboration of biopharmaceutical companies – and supplementing these with a quantitative assessment of predictive accuracy, they showed that the Liver-Chip could faithfully flag the liver’s pathophysiological response to known hepatotoxic drugs. Specifically, the researchers demonstrated that the Liver-Chip was able to detect hepatotoxic drugs that slipped through animal testing with 87% sensitivity. These results suggest that broad use of the Liver-Chip could lead to a nearly eightfold reduction in hepatotoxic drugs entering the clinic. Moreover, the study strongly supports the Liver-Chip’s use in preclinical toxicology assessments due to its potential impact on patient safety: 11 of the drugs used in the study led to 242 deaths due to DILI, and the Liver-Chip correctly flagged each of them.
Following the history of prior pharmaceutical decision-support technologies, it is evident that broad adoption of Liver-Chip technology in drug development calls for the establishment of frameworks for data interpretation and integration. Such frameworks were developed after the introduction of the hERG assay [Citation28], for example, to guide users on suitable contexts of use, understanding of quantitative outputs, and inclusion of the new assay in decision-making processes. In the case of the hERG assay, this integrated approach has significantly reduced the prevalence of QT prolongation during clinical trials, and there have been no cases of new drugs being removed from the market post-launch due to this cause [Citation28].
The objective of this article is to present two frameworks for integrating Liver-Chip data into DILI risk assessment workflows that will assist drug developers in interpreting data from Liver-Chips and increase their confidence when they progress a drug candidate into a clinical trial. The first framework uses the quantitative nature of the Liver-Chip’s output to categorize the tested compounds into the standard Garside DILI severity categories, enabling researchers to gauge the severity of predicted DILI [Citation29]. The second framework describes how Liver-Chip data can be integrated with data from two animal species to guide decision-making. Together, these two frameworks allow the Liver-Chip to be incorporated into hazard identification and preclinical risk assessment to guide management or mitigation strategies.
2. Liver-Chip as a DILI prediction tool
The Liver-Chip recapitulates cell and tissue structures within the microenvironment of the human liver sinusoid using two parallel microfluidic channels separated by a porous membrane (). Primary human hepatocytes are cultured between two layers of extracellular matrix (ECM) in the upper ‘parenchymal’ channel. The lower ‘vascular’ channel contains primary human liver sinusoidal endothelial cells (LSECs), Kupffer cells, and stellate cells in ratios that approximate those observed in vivo [Citation25].
Figure 1. Structure of the Emulate Liver-Chip. The Liver-Chip employs two parallel microfluidic channels (A and H) that are separated by a porous polymer membrane (D) and lined with the major cell types present within the human liver sinusoid. Primary human hepatocytes (C) are situated in the upper channel (A) and sandwiched between two layers of extracellular matrix (B). Liver Sinusoidal Endothelial cells (G), Kupffer cells (F) and stellate cells (E) are cultured on the opposite side of the membrane in the lower channel (H). Figure 1 is original artwork of Emulate Inc. The figure is reproduced from [Citation30] with permission from Emulate Inc.
![Figure 1. Structure of the Emulate Liver-Chip. The Liver-Chip employs two parallel microfluidic channels (A and H) that are separated by a porous polymer membrane (D) and lined with the major cell types present within the human liver sinusoid. Primary human hepatocytes (C) are situated in the upper channel (A) and sandwiched between two layers of extracellular matrix (B). Liver Sinusoidal Endothelial cells (G), Kupffer cells (F) and stellate cells (E) are cultured on the opposite side of the membrane in the lower channel (H). Figure 1 is original artwork of Emulate Inc. The figure is reproduced from [Citation30] with permission from Emulate Inc.](/cms/asset/c87ff192-0fc9-47f8-ae1c-a90ebb0c0e5e/iedc_a_2255127_f0001_oc.jpg)
Characterization of the Liver-Chip has shown it to be a reproducible and functional model of human liver tissue [Citation18,Citation25,Citation31], and prior studies have specifically demonstrated its ability to capture the toxicity of drugs that have led to cholestasis, steatosis, and fibrosis [Citation18]. In particular, the Liver-Chip displayed robust transcriptional, morphological, and biomarker profiles that are consistent with industry guidelines for characterizing liver microphysiological systems for DILI prediction [Citation26]. The predictive validity of the Liver-Chip was evaluated by testing 27 small molecule drugs in Liver-Chips using cells from three different donors. 22 of the tested drugs are known to be hepatotoxic in humans and have demonstrated varying degrees of DILI in clinical settings. As toxicity depends on drug concentration, the drugs were evaluated at up to eight different concentrations that bracket the human plasma unbound Cmax. Liver-Chip readouts included the inhibition of albumin production (quantified as an IC50), rise in secreted alanine aminotransferase (ALT) levels (a clinical biomarker of liver damage), and morphological scoring of hepatocyte injury. A score was calculated using these readouts by determining the lowest drug concentration with observed toxicity and comparing that concentration to the published unbound human plasma Cmax required for efficacy. We refer to this computed number as the ‘Liver-Chip DILI score,’ with lower scores indicating greater DILI risk.
The sensitivity and specificity of the Liver-Chip were determined by considering drugs with Liver-Chip DILI scores below 375 as predicted toxic. Comparing these predictions to clinical data, the study demonstrated that the Liver-Chip produced a high degree of sensitivity (87%) and specificity (100%) [Citation25]. Notably, all hepatotoxic drugs used in the study had previously been deemed safe enough for use in humans based on preclinical toxicology evaluation involving two different animal models, only to later be revealed as hepatotoxic in the clinic, resulting in withdrawal from the market or a black box label warning. Animal models (together with the decision systems used to evaluate their findings) can therefore be viewed as the baseline for the Liver-Chip’s 87% sensitivity result, indicating the Liver-Chip’s ability to capture nearly 7 out of every 8 toxic drugs that would otherwise evade traditional safety testing workflows and be administered to human subjects. In turn, 100% specificity is important for ensuring that nontoxic drugs are not rejected erroneously; this is a crucial consideration when incorporating new assays into safety evaluation workflows, as safe and effective drugs are too valuable to incorrectly reject, and lower specificity would create confusion when reporting a compound as DILI-positive as to the veracity of that signal. Accordingly, the findings suggest that the integration of Liver-Chips into preclinical workflows could substantially reduce the number of hepatotoxic drug candidates that enter the clinic, with low risk of blocking any ‘good’ drugs.
3. Predicting DILI severity
In the context of use of DILI prediction, models that only support binary readouts of ‘toxic’ and ‘nontoxic’ are of limited value when deciding whether a drug candidate is worth continued development: Risk tolerance to DILI and other adverse events varies by disease area and patient population, and a risk of low-grade DILI may be acceptable in certain situations (e.g. oncology). Therefore, researchers look to models that provide quantitative outputs to help support nuanced decision-making during preclinical toxicity assessments.
Although the analysis in Ewart et al. [Citation25] focused on determining if drugs were toxic versus nontoxic, the Liver-Chip DILI score can provide deeper insight into DILI severity by mapping a compound’s Liver-Chip DILI score () to the likelihood of falling into DILI severity categories based on clinical outcome as described by Garside et al. [Citation29].
Figure 2. Workflow for assessing potential DILI severity. when a compound of interest is run through the Liver-Chip, its effects on albumin production, alanine transaminase (ALT) release, and hepatocyte morphology are measured. These readouts are combined with the compound’s human plasma Cmax for efficacy to compute a Liver-Chip DILI score. These scores can then be mapped to industry-standard DILI risk categories to provide the drug developer with information on the potential severity of injury that may be caused by the compound if it progresses into the clinic. Used with permission of Emulate Inc.
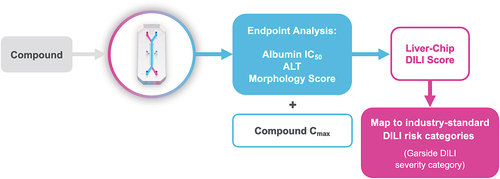
The Garside DILI scale, based on data from 144 drugs, divides compounds into five severity categories: Garside’s category 1, the most serious of the set, represents compounds that caused severe DILI and have been withdrawn from clinical markets or given black box warning labels due to hepatotoxicity. Categories 2 and 3 are defined by drugs that have led to symptomatic liver injury and have either caused acute liver failure or elicited liver injury without inducing organ failure (respectively). Category 4 speaks to drugs for which there is evidence of elevated liver enzymes, but symptomatic liver injury was absent. Lastly, category 5, the least severe, represents drugs that have not been reported to elicit any DILI signals.
To relate the Liver-Chip DILI score to clinical DILI severity, Liver-Chip DILI scores were banded into five ranges that align with the Garside DILI severity categories. Within each band, the positive predictive value (PPV) was calculated for each Garside category to define the probability that a compound would fall into that Garside category. In this analysis, Garside categories 4 and 5 were grouped, as both correspond to the absence of symptomatic liver injury (). The analysis demonstrated that any compounds with Liver-Chip DILI score values lower than 31 should be excluded from further development, as these compounds are almost certain to cause acute liver injury (correspond to Garside category 1). In contrast, compounds with Liver-Chip DILI scores greater than 375 are predicted nontoxic (contingent on 87% sensitivity). However, Liver-Chip DILI scores between 31 and 375 should be evaluated using the framework outlined in and within the broader context of the project and therapeutic area.
Figure 3. Mapping of Liver-Chip DILI score to DILI severity categories. Liver-Chip DILI scores can be divided into five bands, and these can be mapped to a prediction of DILI severity. Indicated along the columns are Garside severity categories, a standard hepatotoxicity ranking system that is based on clinical observation. As the Liver-Chip DILI score increases, the positive predictive value (PPV) shifts toward the less severe risk categories. Used with permission of Emulate Inc.
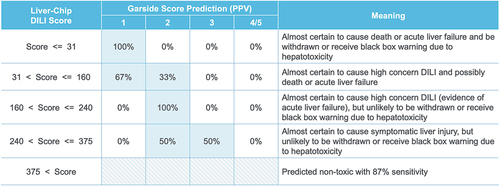
This framework enhances the value pharmaceutical developers can derive from the Liver-Chip by enabling them to consider potential DILI severity for drug candidates. This should help guide researchers to make informed decisions based on the context of the individual drug development program and its specific risk tolerance.
As with any decision-making tool, there are limitations. For example, was developed using the presently available Liver-Chip data, which leaves some combinations of Garside Score and Liver-Chip DILI score bands sparsely covered. This will improve over time as adoption of the Liver-Chip increases and more data are made available, leading to the probability assignments and band thresholds in being updated.
4. Integrating Liver-Chip and animal data
Following the Elixir Sulfanilamide tragedy in 1937 that was responsible for the deaths of at least 100 patients, drug developers in the United States are required by the Food and Drug Administration (FDA) to test the safety of promising drug candidates in at least two different animal species before advancing them into clinical trials, with similar regulatory policies in place around the world [Citation32]. Even with the passage of the FDA Modernization Act in December 2022 [Citation6,Citation33]—which allows for researchers to use non-animal models in preclinical testing if scientifically appropriate – it is likely that animals will continue to play an influential role in preclinical toxicity testing for the time being [Citation32]. This is because animal models benefit from extensive historical data to inform their use, offer researchers a way to investigate toxicity in multiple organs within a single study, and capture whole-organism response that may include complex interactions between interconnected organ systems [Citation34].
However, ample evidence suggests that animal models frequently fail to predict toxic outcomes, leading to staggeringly high clinical trial failure rates and patient harm [Citation13–17]. Fortunately, recent findings indicate that the Liver-Chip may be highly capable in detecting hepatotoxic drugs that animal models permit into the clinic [Citation25], suggesting that the Liver-Chip may be a valuable addition to drug development workflows to be used alongside animal models. We therefore present here a second framework, which demonstrates how Liver-Chip data can be integrated with animal testing results to guide preclinical decision-making ().
Figure 4. Positioning the Liver-Chip into the drug development workflow to support regulatory submission. Use of the human Liver-Chip after or concurrently with animal good Laboratory Practice (GLP) toxicology studies can provide drug developers with further confidence in the safety profile of a candidate drug prior to entry into clinical trials. Liver-Chip DILI scores obtained in this manner can be integrated with the animal study results using the decision matrix in Figure 5. Alternatively, the Liver-Chip may be used prior to animal testing to help researchers divest from prohibitively toxic compounds before animals are sacrificed for safety testing or to limit the range of doses used in animal experiments. This alternative workflow is consistent with 3 R goals as described in Ewart et al., 2022 [Citation25]. Used with permission of Emulate Inc.
![Figure 4. Positioning the Liver-Chip into the drug development workflow to support regulatory submission. Use of the human Liver-Chip after or concurrently with animal good Laboratory Practice (GLP) toxicology studies can provide drug developers with further confidence in the safety profile of a candidate drug prior to entry into clinical trials. Liver-Chip DILI scores obtained in this manner can be integrated with the animal study results using the decision matrix in Figure 5. Alternatively, the Liver-Chip may be used prior to animal testing to help researchers divest from prohibitively toxic compounds before animals are sacrificed for safety testing or to limit the range of doses used in animal experiments. This alternative workflow is consistent with 3 R goals as described in Ewart et al., 2022 [Citation25]. Used with permission of Emulate Inc.](/cms/asset/ab640b68-ca46-4963-824f-7eea41ce7cea/iedc_a_2255127_f0004_oc.jpg)
Figure 5. Decision matrix for combining animal model and Liver-Chip data to improve preclinical decision making. This chart maps out the possible scenarios faced by a project toxicologist after performing studies in two animal species and cross-referencing these with possible findings from a human Liver-Chip study. This analysis can furthermore leverage quantitative output from the Liver-Chip as captured by the Liver-Chip DILI score to provide a sense of potential DILI severity, affording greater confidence in the decision to progress a candidate drug into the clinic. Used with permission of Emulate Inc.
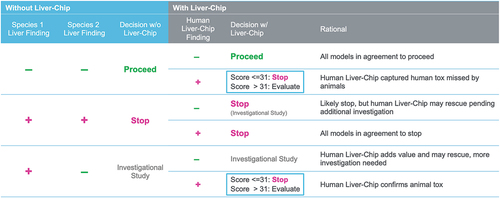
illustrates a workflow in which the human Liver-Chip may be used after or concurrently with animal GLP toxicology studies to provide users with further confidence in the safety profile of a candidate drug prior to entry into clinical trials. Alternatively, the Liver-Chip may be used prior to animal testing to help researchers divest from prohibitively toxic compounds before animals are sacrificed for safety testing or to limit the range of doses used in animal experiments. This alternative workflow is consistent with 3 R goals to reduce, refine and replace animal testing [Citation34].
Liver-Chip data can be integrated with animal study results using the decision matrix in : The matrix maps out the possible scenarios faced by a project toxicologist after performing studies in two animal species and cross-referencing these with possible findings from a human Liver-Chip study. The first three columns of the decision matrix illustrate how animal data would guide drug development decisions in the absence of Liver-Chip data. The remaining columns show how Liver-Chip data would integrate with and augment the decisions made based on animal data alone. The decision matrix reveals that Liver-Chip data could particularly impact decision-making in two scenarios: in the case where both animal species clear the drug candidate but the Liver-Chip flags toxicity – this is the scenario explored by Ewart et al. [Citation25]—and in the case where the two animal species conflict in their findings and the toxicity is confirmed by the Liver-Chip. In this second scenario, the Liver-Chip may be able to provide additional insight into DILI risk to help guide the decision and form part of the weight of evidence package. In both scenarios, the analysis can leverage quantitative output from the Liver-Chip as captured by the Liver-Chip DILI Score to indicate potential DILI severity, affording greater confidence in the decision to progress a candidate drug into the clinic.
The advantage of integrating animal and Liver-Chip data as enabled by this framework is that it combines the respective advantages of both systems: Drug developers and regulators can continue to benefit from the wealth of historical understanding available for animal models as well as their ability to capture whole organism response; in turn, they can also leverage the improved capacity to detect human DILI and the ability to assess its severity that the Liver-Chip affords. Downsides to this approach are limited. Of note, Ewart et al. constructed an economic model that illustrates that the extra cost of Liver-Chip experiments should be vastly offset by the reduction in clinical trial failures [Citation25]. Similarly, the presented framework benefits significantly from the Liver-Chip’s reported 100% specificity, which indicates that the likelihood of generating false-positive signals is low; an assay with lesser specificity would require significant additions to the workflow to avoid erroneously rejecting ‘good’ drugs, which are too valuable to discard.
The framework in can also be used to address a key question raised at an FDA-IQ MPS workshop [Citation35] that asked whether animal versions of the Liver-Chip could aid in making preclinical safety decisions. According to the workshop proposal, comparison of results obtained using Liver-Chips lined with cells isolated from relevant animal species used in preclinical DILI testing (e.g. rodents, dogs) with those resulting from studies with human Liver-Chips could help to clarify which observed differences are due to species-specific biology (human vs. animal) as opposed to technological differences (chip vs. in vivo). enables addressing the utility of the animal Liver-Chips in combination with human Liver-Chips by evaluating them in each of the six scenarios listed. Specifically, in the scenario where both animal experiments are negative for DILI, the analysis benefits from the human Liver-Chip’s high specificity (100%), which was shown to be many times more accurate than animal models in a study analyzing 27 different drugs with known safety profiles in dogs, rats, and humans [Citation25]. Moreover, given past results that also showed rat and dog Liver-Chips replicate DILI for multiple drugs in a species-specific manner [Citation18], there is a high likelihood that animal Liver-Chips would similarly come back negative and not provide helpful information. Similarly, in the scenario where both in vivo animal experiments are positive for DILI, human Liver-Chip data are unlikely to overrule the two species at the present time, and so supplementing with animal Liver-Chips is moot. When two different preclinical animal models produce conflicting results and the human Liver-Chip indicates a high likelihood for DILI, drug developers would benefit from the Liver-Chip’s 100% specificity. Thus, the human Liver-Chip’s indication of toxicity deserves notice and provides great value regardless of animal Liver-Chip experiments. However, animal Liver-Chips could have value when results from two in vivo experiments conflict and the human Liver-Chip is negative for DILI. Here, an animal Liver-Chip corresponding to the species that indicated toxicity in vivo could help in the investigation of the mechanism of toxicity that could rescue the drug candidate. However, this usage falls under the umbrella of investigational toxicology, which is a separate context of use from DILI prediction and is typically engaged as a separate follow-on process only when demanded. Accordingly, animal Liver-Chips may indeed be helpful for mechanistic and investigational toxicology, but they are likely of limited utility in the context of DILI prediction.
Another consideration for integrating the Liver-Chip into safety workflows is the model’s experimental throughput – the number and size of the experiments that can be reasonably performed. Throughput needs vary greatly depending on the context in which a technology is used within the drug development process and needs to be balanced with the desired biological complexity. The workflow in corresponds specifically to the lead optimization phase, where drug-development projects typically profile 3 to 5 candidate drugs. Fortunately, assessing this number of candidate drugs is well within the throughput of Liver-Chip technology, as illustrated by the Ewart et al. study [Citation25].
5. Conclusion
In this paper, two frameworks have been presented that guide the interpretation of Liver-Chip data and its subsequent use in decision-making during preclinical DILI risk assessments. The first framework introduces the Liver-Chip DILI Score and how it can be used to consolidate quantitative data from the Liver-Chip to predict compounds’ clinical DILI severity according to Garside categories. By taking advantage of the quantitative nature of the Liver-Chip, this framework provides a more nuanced assessment of DILI risk and can inform pharmaceutical decision-making with greater accuracy.
The second framework describes how Liver-Chips can be integrated into typical preclinical safety workflows involving two animal species. The proposed methodology for combining Liver-Chip and animal data permits drug developers and regulators to benefit from the advantages of both model types to establish a more holistic assessment of DILI risk. With minimal downsides, this approach can provide pharmaceutical decision-makers with greater confidence in determining the safety profile of prospective therapeutics.
Overall, the two frameworks have significant potential to improve DILI risk assessment and, ultimately, drug development workflows. By incorporating the Liver-Chip as a complementary tool to existing biological models, pharmaceutical companies can make more informed decisions about which drug candidates to advance further in their pipeline. Furthermore, by reducing the likelihood of DILI-related failures in clinical trials and withdrawals of drugs from market, these frameworks have the potential to improve patient safety and drug development productivity.
While the guidance provided here is specific to the prediction of DILI risk, these frameworks can serve as templates for the future development of additional Organ-Chip workflows that address the remaining top causes of safety failures. The pharmaceutical industry and regulatory communities are encouraged to adopt these frameworks and to continue to explore the potential of Organ-on-a-Chip technology to improve drug development processes and enhance patient safety. By doing so, the community can move toward a more efficient, effective, and safe approach to drug development.
6. Expert Opinion
History books point to tragic events which have shaped the testing of new pharmaceuticals prior to human testing. Following the deaths of more than 100 patients who took the cough medicine Elixir Sulfanilamide in 1937, a legislative act was passed in 1938 mandating animal toxicity testing prior to human clinical trial. Further clinical monitoring was favored with the Kefauver-Harris Amendment in 1962 following the discovery that Thalidomide treatment was linked to an increase in newborn birth abnormalities. Although animal testing has enabled many valuable therapeutics to reach patients, safety concerns still plague the pharmaceutical industry. DILI can lead to fatalities, liver transplantation or the pausing of a clinical trial, increasing the already lengthy drug development times. Indeed, since January 2022, there have been seven reported cases of DILI during clinical trials and at least two patient fatalities from a drug that is on the market. The time is now to update preclinical safety testing by supplementing the animal models with human-relevant data obtained from organ-on-a-chip technology. With published data showing that the Liver-Chip has an 87% sensitivity and 100% specificity, and its use can deliver a $3 billion productivity gain to the pharmaceutical industry [Citation25]—together with the passage of the FDA Modernization Act in December 2022—there has never been a better time to protect patient safety using 21st-century technology.
The article highlights a framework that drug developers and regulators can employ to improve confidence in the critical decision to move a drug candidate into human testing. Characterized in line with pharmaceutical industry guidelines, Liver-Chip’s quantitative output can be used to predict the DILI severity category, especially when combined with animal model data. It is conceivable that the Liver-Chip can co-exist with animal models and that with time, prevailing data could rationally demonstrate improved patient-safety and the potential for a further reduction in animal use. If such a framework is deployed to other characterized Organ-Chips, further strides can be made in assuring patient safety as well as greater productivity gains within the pharmaceutical industry.
Looking to the future, disease models created using Organ-on-a-Chip technology can be employed to extend efficacy determination prior to first-time in human clinical trial. Such models that capture the complexity of the innate and adaptive human immune system will offer tremendous benefit for humans especially given the species differences.
Article highlights
Liver toxicity remains a leading cause of drug attrition during clinical development and post-market approval, and human-relevant models such as the Liver-Chip have demonstrated the ability to catch toxicity missed by current safety testing.
The Liver-Chip’s performance in predicting drug-induced liver injury (DILI) was assessed in accordance with third-party expert guidelines, demonstrating 87% sensitivity and 100% specificity. This could enable nearly an eightfold reduction in hepatotoxic compounds that enter the clinic.
The technology’s adoption would benefit from the development of guidelines for the integration of Liver-Chip data into decision-making processes to aid drug developers and regulators. Accordingly, two decision-support frameworks are presented in this article.
The first framework maps the quantitative output of the Liver-Chip to clinical DILI severity categories to enable more nuanced risk assessment.
The second framework details how human Liver-Chip data can be used together with animal model findings to enhance preclinical drug safety decision making ahead of clinical trials.
Author contributions
D Levner and L Ewart contributed equally to the conception and evaluation of the frameworks presented in this paper, and both also contributed equally to the manuscript preparation, writing, and review.
Declaration of interest
D Levner is a co-founder, employee, board member, and equity holder in Emulate Inc. L Ewart is an employee of Emulate Inc. and an equity holder. The authors have no other relevant affiliations or financial involvement with any organization or entity with a financial interest in or financial conflict with the subject matter or materials discussed in the manuscript apart from those disclosed.
Reviewer Disclosures
Peer reviewers on this manuscript have no relevant financial or other relationships to disclose.
Acknowledgments
The authors would like to thank Jim Corbett and Jenn Perkins for their valuable discussion and support during the development of the concepts presented within this paper.
Additional information
Funding
References
- Center for Drug Evaluation and Research & Center for Biologics Evaluation and Research. Guidance for industry drug-induced Liver injury: premarketing clinical evaluation. Drug-induced Liver injury: premarketing clinical evaluation (2009). Available from: https://www.fda.gov/media/116737/download
- Siramshetty VB, Nickel J, Omieczynski C. Withdrawn—a resource for withdrawn and discontinued drugs. Nucleic Acids Res. 2015;44:D1080–D1086. doi: 10.1093/nar/gkv1192
- Onakpoya IJ, Heneghan CJ, Aronson JK. Post-marketing withdrawal of 462 medicinal products because of adverse drug reactions: a systematic review of the world literature. BMC Med. 2016;14(1):10. doi: 10.1186/s12916-016-0553-2
- David S, Hamilton JP. Drug-induced Liver injury. US Gastroenterol Hepatol Rev. 2010;6:73–80.
- Thakkar S, Chen M, Fang H, et al. The liver toxicity knowledge base (LKTB) and drug-induced liver injury (DILI) classification for assessment of human liver injury. Exp Rev Gastroenterol Hepatol. 2017;12:31–38. doi: 10.1080/17474124.2018.1383154
- Wadman M. FDA no longer needs to require animal tests before human drug trials. Science. 2003;379:127–128. doi: 10.1126/science.adg6276
- Junod SW FDA and clinical drug trials: a short history. FDA and clinical drug trials: a short history (2009). Available at: https://www.fda.gov/media/110437/download. (Accessed: 15th Jun 2023)
- Swaters D, van Veen A, Ritskes-Hoitinga M, et al. A history of regulatory animal testing: what can we learn? Altern Lab Anim. 2022;50:322–329. doi: 10.1177/02611929221118001
- DeKeyser JG. Shou M species differences of drug-metabolizing enzymes. Encyclopedia Of Drug Metabolism And Interactions. 2012. doi: 10.1002/9780470921920.edm005
- Scannell JW, Bosley J, Hickman JA, et al. Predictive validity in drug discovery: what it is, why it matters and how to improve it. Nat Rev Drug Discov. 2022;21(12):915–931. doi: 10.1038/s41573-022-00552-x
- Sun D, Gao W, Hu H, et al. Why 90% of clinical drug development fails and how to improve it? Acta Pharm Sin B. 2022;12:3049–3062. doi: 10.1016/j.apsb.2022.02.002
- Valentin JP, Redfern WS. Prevalence, frequency, and impact of safety related issues throughout the pharmaceutical life cycle. The Toxicologist. 2017. Abstract Number 1722. https://www.toxicology.org/pubs/docs/tox/2017tox.pdf
- Akhtar A. A the flaws and human harms of animal experimentation. Camb Q Healthc Ethics. 2015;24:407–419. doi: 10.1017/S0963180115000079
- Pound P, Ritskes-Hoitinga M. Ritskes-Hoitinga M is it possible to overcome issues of external validity in preclinical animal research? Why most animal models are bound to fail. J Transl Med. 2018;16: doi: 10.1186/s12967-018-1678-1
- Ingber DE. Is it time for reviewer 3 to request human organ chip experiments instead of animal validation studies? Adv Sci. 2020;7(22):2002030. doi: 10.1002/advs.202002030
- Matthews RA. Medical progress depends on animal models - doesn’t it? J R Soc Med. 2008;101:95–98. doi: 10.1258/jrsm.2007.070164
- Bailey J, Thew M, Balls M. An analysis of the use of animal models in predicting human toxicology and drug safety. Altern Lab Anim. 2014;42:181–199.
- Jang KJ, Otieno MA, Ronxhi J, et al. Reproducing human and cross-species drug toxicities using a liver-chip. Sci, trans med. 2019;11(517):eaax5516. doi: 10.1126/scitranslmed.aax5516
- Jang KJ, Mehr AP, Hamilton GA, et al. Human kidney proximal tubule-on-a-chip for drug transport and nephrotoxicity assessment. Integr Biol. 2013;5(9):1119–1129. doi: 10.1039/c3ib40049b
- Apostolou A, Panchakshari RA, Banerjee A, et al. A novel microphysiological colon platform to decipher mechanisms driving human intestinal permeability. Cell Mol Gastroenterol Hepatol. 2021;12(5):1719–1741. doi: 10.1016/j.jcmgh.2021.07.004
- Kerns SJ, Belgur C, Petropolis D, et al. Human immunocompetent Organ-on-Chip platforms allow safety profiling of tumor-targeted T-cell bispecific antibodies. Elife. 2021;10:e67106. doi: 10.7554/eLife.67106
- Pediaditakis I, Kodella KR, Manatakis DV, et al. A microengineered Brain-Chip to model neuroinflammation in humans. iScience. 2022;25:104813. doi: 10.1016/j.isci.2022.104813
- Ingber DE. Human organs-on-chips for disease modelling, drug development and personalized medicine. Nat Rev Genet. 2022;23(8):467–491. doi: 10.1038/s41576-022-00466-9
- Low L, Mummery C, Berridge BR, et al. Organs-on-chips: into the next decade. Nat Rev Drug Discov. 2021;20(5):345–361. doi: 10.1038/s41573-020-0079-3
- Ewart L, Apostolou A, Briggs SA, et al. Performance assessment and economic analysis of a human liver-chip for predictive toxicology. Commun Med. 2022;2(1):154. doi: 10.1038/s43856-022-00209-1
- Baudy AR, Otieno MA, Hewitt P, et al. Liver microphysiological systems development guidelines for safety risk assessment in the pharmaceutical industry. Lab Chip. 2019;20:215–225.
- Fabre K, Berridge B, Proctor WR, et al. Introduction to a manuscript series on the characterization and use of microphysiological systems (MPS) in pharmaceutical safety and ADME applications. Lab Chip. 2020;20(6):1049–1057. doi: 10.1039/C9LC01168D
- Vargas HM, Rolf MG, Wisialowski TA, et al. Time for a fully integrated nonclinical–clinical risk assessment to streamline QT prolongation liability determinations: a pharma industry perspective. Clin Pharmacol Ther. 2020;109:310–318. doi: 10.1002/cpt.2029
- Garside H, Marcoe KF, Chestnut-Speelman J, et al. Evaluation of the use of imaging parameters for the detection of compound-induced hepatotoxicity in 384-well cultures of HEPG2 cells and cryopreserved primary human hepatocytes. Toxicol In Vitro. 2014;28:171–181.
- Organ-Chip Model – Liver-Chip. Emulate Inc. https://emulatebio.com/liver-chip/ [cited 31 Aug, 2023]
- Shi Q, Arefin A, Ren L, et al. Co‐culture of human primary hepatocytes and nonparenchymal liver cells in the Emulate® Liver‐Chip for the study of drug‐induced liver injury. Curr Protoc. 2022;2(7):2;e478. doi: 10.1002/cpz1.478
- Sántha M. Biologia futura: animal testing in drug development—the past, the present and the future. Biologia Futura. 2020;71(4):443–452. doi: 10.1007/s42977-020-00050-4
- S.5002 - 117th congress (2021-2022): FDA Modernization act 2.0. S.5002 - FDA Modernization act 2.0 (2022). Available at: https://www.congress.gov/bill/117th-congress/senate-bill/5002. (cited 15th Jun 2023)
- Sewell F, Edwards J, Prior H, et al. Opportunities to apply the 3Rs in safety assessment programs. ILAR J. 2016;57(2):234–245. doi: 10.1093/ilar/ilw024
- Baran S, Brown PC, Baudy AR, et al. Perspectives on the evaluation and adoption of complex in vitro models in drug development: workshop with the FDA and the pharmaceutical industry (IQ MPS Affiliate). ALTEX. 2022;39(2):297–314. doi: 10.14573/altex.2112203