Abstract
The land surface broadband emissivity (LSBE) is a key parameter for estimating surface radiation, and there have been many studies of the LSBE at global or local scales. However, few studies have validated the surface emissivity database with multi-point field measurement data using infrared radiometry, especially in China. In this study, we focus on the validation of the emissivity product of the global land surface satellite (GLASS) LSBE database for northern China for the period from 2006 to 2011. Specifically, we have employed an eight-day averaged, gridded emissivity product in the 8–13.5 µm spectral range produced at a spatial resolution of 1000 m from the Moderate Resolution Imaging Spectrometer albedo product using a new algorithm. The GLASS LSBE database was validated over bare surfaces with field measurement data from sand samples collected at many pseudo-invariant sand dune sites located in western and northwestern China. By comparing measured emissivity for different land surface types at different sites and different times, it was shown that the results were consistent and that the accuracy of the field measurements was reliable. The results of the validation of GLASS LSBE with these field emissivity data showed very good agreement.
1. Introduction
The inversion and measurement of land surface emissivity (LSE) is an important issue in thermal infrared remote sensing. Surface emissivity of an isothermal homogeneous emitter is defined as the ratio of the actual emitted radiance to the radiance emitted from a black body at the same thermodynamic temperature (Norman and Becker Citation1995). Surface emissivity and temperature indicate the inherent efficiency of the surface in converting heat energy into radiant energy, as well as determining the emitted thermal infrared spectral radiance (Caselles et al. Citation1995) and other fundamental parameters measured in thermal infrared remote sensing (Gillespie et al. Citation2011). Knowledge of the land surface temperature and emissivity (LST/E) is of prime interest when studying the energy and water balance of the Earth, biosphere, and atmosphere (Jacob et al. Citation2004). Errors in the surface emissivity influence the inversion of LSTs, and some studies have shown that for a material at 300 K, an emissivity error of 0.5% at 11 µm corresponds to a surface temperature error of 0.3 K (Galve et al. Citation2008), and an error of 1.5% at 8.6 µm will result in a surface temperature error of approximately 1 K (Hulley and Hook Citation2009a).
Land surface broadband emissivity (LSBE) is a key variable in the calculation of the Earth's radiation budget (Ogawa et al. Citation2003; Zhou et al. Citation2003). It is an important measure of energy and matter exchange between the atmosphere and surface, of global ocean circulation (Valor and Caselles Citation1996), in numerical weather prediction and hydrological models, and in radiation assessments for climate simulations and predictions (Cheng et al. Citation2010b; Jin and Liang Citation2006; Liang Citation2004; Liang et al. Citation2010; Pequignot, Chedin, and Scott Citation2008; Sellers, Dickinson, and Randall Citation1997). In recent years, many emissivity products have been generated by space-borne sensors, such as the Moderate Resolution Imaging Spectrometer (MODIS) (Wan and Li Citation1997; Wan Citation2008), the Atmospheric Infrared Sounder (AIRS) (Susskind, Barnet, and Blaisdell Citation2003), and Advanced Spaceborne Thermal Emission And Reflection (ASTER) (Gillespie et al. Citation1998). Earlier studies (Gillespie et al. Citation1998; Sabol et al. Citation2009) found the accuracy of the ASTER surface emissivity standard product to be within the predicted level of ±0.015, but under some circumstances, such as humid atmospheres, unaccounted-for boundary layer conditions, or electronic striping, the performance appears to deteriorate. Gillespie et al. (Citation2011) found systematic warping and the presence of equivalent spectra at about the level of the predicted error for individual bands. In contrast, Li and Becker (Citation1993) found that the accuracy of the mean and difference emissivities from MODIS in the wavelength ranges 10.3–11.3 µm and 11.5–12.5 µm was 0.004. However, the MODIS emissivity is an LST byproduct, and the day/night LST method has some inherent theoretical and implementational complications (Momeni and Saradjian Citation2007). Moreover, MODIS has a higher temporal resolution (twice daily), but its emissivity product is much coarser (5 km) (Cheng and Liang Citation2012). Furthermore, due to the varying spatial, spectral, and temporal resolutions, and the different algorithms used to retrieve the surface emissivity, there are often discrepancies between these products.
For spatially distributed LSBE, thermal infrared remote sensing (TIR) is a unique technology. Ogawa et al. (Citation2002, Citation2003) pointed out that LSBE can be estimated as a linear combination of the channel estimates. However, surface heterogeneities will induce nonlinear effects. Many algorithms to estimate LSE from satellite passive sensor data have already been investigated, and some of the widely accepted approaches include the Temperature Emissivity Separation (TES) algorithm (Gillespie et al. Citation1996, Citation1998; Schmugge, Hook, and Coll Citation1998; Schmugge et al. Citation2002), the Temperature Independent Spectral Indices of Emissivity (TISIE) approach (Becker and Li Citation1990; Li and Becker Citation1993; Nerry, Petitcolin, and Stoll Citation1998; Petitcolin, Nerry, and Stoll Citation2002a, Citation2002b; Petitcolin and Vermote Citation2002), day/night method (Becker and Li Citation1990; Wan and Li Citation1997; Watson Citation1992), gray-body emissivity method (Barducci and Pippi Citation1996), Normalized Difference Vegetation Index (NDVI)-based emissivity calculation method (Sobrino and Raissouni Citation2000; Van De Griend and Owe Citation1993; Valor and Caselles Citation1996), and classification-based estimation (Snyder et al. Citation1998), all of which take a numerical approach to estimating the absolute emissivity. Li et al. (Citation2013) presented an overview of the LSE retrieval from satellite data. There are few studies on the direct inversion of LSBE from thermal infrared data. The usual practice is to invert the narrow-band emissivity first and then convert it to LSBE.
A few have focused on the LSBE at global or local scales. Ogawa and Schmugge (Citation2004) and Ogawa, Schmugge, and Rokugawa (Citation2008) mapped the global LSBE using the MODIS emissivity product, whose spatial resolution is 5 km and the North African LSBE using the ASTER emissivity product, whose spatial resolution is 90 m. Wilber, Kratz, and Gupta (Citation1999) generated a global LSBE (5–100 µm) map with a 10°×10°spatial resolution. Tang et al. (Citation2011) proposed to estimate the 3–14 µm and 3–∞ µm broadband emissivity (BBE) by combining MODIS narrow-band emissivity of channels 29, 31, and 32. At present, moderate spatial resolution LSBE on the order of 1 km is simply not available (Cheng and Liang Citation2012). Cheng and Liang (Citation2013) investigated the accuracy of remote sensing BBE at different spectral ranges in estimating net surface long-wave radiation and found that BBE can achieve optimal accuracy at a spectral range of 8.0–13.5 µm. Based on soil spectra from the Johns Hopkins University Spectral Library, BBE at 8.0–13.5 µm and the seven corresponding MODIS narrow-band black-sky albedos were calculated. A significant linear relationship between BBE and the seven narrow-band black-sky albedos was explored. This relationship was initially verified for bare soil (Cheng and Liang Citation2012) and then for homogeneous vegetated areas (Ren et al. Citation2012).
A few authors have previously attempted to validate surface emissivity products (Schmugge and Ogawa Citation2006; Schmugge et al. Citation2003). Hulley and Hook (Citation2009a) validated the North American ASTER LandSurface Database (NAALSED) v2.0 emissivity product over arid and semi-arid regions using nine pseudo-invariant sand dune sites with laboratory measurements in western and southwestern USA. Gillespie et al. (Citation2011) studied the residual errors of ASTER temperature and emissivity standard products (AST08 and AST05). Hulley and Hook (Citation2009b) analyzed the temporal and spatial variations of the MOD11B1 LST/E product for versions 4, 4.1, and 5 and validated each product version with laboratory emissivity measurements of sand samples collected in the Namib Desert in Namibia. Momeni and Saradjian (Citation2007) evaluated the NDVI-based emissivity of MODIS bands 31 and 32 using emissivity data derived by the day/night LST algorithm. Jacob et al. (Citation2004) compared the LSE and radiometric temperatures derived from MODIS and ASTER sensors. The MODIS estimates were computed using the TISIE method and the ASTER estimates were derived using the TES algorithm. Sobrino, Raissouni, and Li (Citation2001) compared LSE computed using four algorithms from National Oceanic and Atmospheric Administration (NOAA) data. We can see that there have been few validation exercises using multi-point field measurement data from infrared radiometry, especially in China. The focus of this work is the precision of the global land surface satellite (GLASS) LSBE standard product in northern China, rather than its scaling. In this study, the prime goal is to validate the accuracy of GLASS LSBE with field measurements and to understand uncertainties in the LSBE products.
2. GLASS LSBE database
GLASS emissivity is a BBE (8–13.5 µm) product derived from AVHRR VNIR data and MODIS albedos using newly developed algorithms (Cheng et al. Citation2010a, Citation2011, Citation2013; Cheng and Liang Citation2012, Citation2013; Ren et al. Citation2012). GLASS emissivity has two parts: (1) the global eight-day 1-km land surface BBE retrieved from MODIS albedos from 2000 to 2010 and (2) the global eight-day 5-km land surface BBE retrieved from AVHRR VNIR reflectance from 1981 to 1999.
Since 2011, based on making comprehensive use of domestic and overseas remote sensing data sources, a new inversion algorithm and production system for the LSBE standard product on a global scale has been developed. The MODIS albedo products and quality control data, the MODIS NDVI product, and the ASTER emissivity product were used to develop the LSBE product in the 8–13.5 µm spectral range (Cheng and Liang Citation2012). The temporal and spatial resolutions of the product are eight days and 1 km, respectively. The objective of the new emissivity algorithm was to establish the relationship between the MODIS albedo and ASTER LSBE for bare soil on a global scale to help generate a global LSBE product (Cheng and Liang Citation2012). In the algorithm used to generate GLASS BBE from MODIS albedos, land surface was classified into five types according to NDVI threshold values: water, snow, or ice; bare soil (0 < NDVI ≤ 0.156); vegetated area (NDVI > 0.156); and transition zone (0.1 < NDVI < 0.2). Areas of overlapping bare soil and transition zone as well as of transition zone and vegetated area were noted. BBEs of water and snow or ice were set as 0.985 based on a combination of BBE calculated from the emissivity spectrum in the ASTER spectral library (http://spclib.jpl.nasa.gov) and the MODIS University of California Santa Barbara Emissivity Library (http://www.icess.ucsb.edu/modis/EMIS/html/em.html) and BBE simulated through radiative transfer models. BBEs of bare soil, vegetated areas, and transition zones were formulated as the linear function of seven MODIS narrow-band black-sky albedos. When NDVI was lower than 0.1 or higher than 0.2, the formula for bare soil or vegetated areas was used to calculate individual BBE values. In areas of overlapping bare soil and transition zone (0.1 < NDVI ≤ 0.156), BBE was taken as the average of values calculated using formulas for bare soil and transition zones. By contrast, BBE for areas of overlapping transition zone and vegetated area (0.156 < NDVI < 0.2) was taken as the average of values calculated using formulas for transition zones and vegetated areas. The algorithm for retrieving global land surface BBE from AVHRR VNIR data was similar to that for retrieving global land surface BBE from MODIS albedos. A bare soil pixel was identified as 0 < NDVI ≤ 0.2, a transition zone pixel as 0.145 ≤ NDVI ≤ 0.243, and a vegetated area pixel as NDVI ≥ 0.2. The nonlinear formulas for bare soil, vegetated areas, and transition zones were established using nonlinear regression with extracted reflectance-BBE pairs. The vegetation algorithm for the LSBE product has been discussed in a separate study (Cheng and Liang Citation2013; Ren et al. Citation2012).
Due to the difficulty of direct validation of emissivity derived from remote sensing data, in this study, we will evaluate the GLASS LSBE database using pseudo-invariant sand dune sites in northern China.
3. Field measurements of emissivity at pseudo-invariant sites in northern China
The following sections describe the location of the validation sample sites and the methodology for measuring the emissivity at the field sample points, and then, a comparison of GLASS LSBE with the measured results is discussed.
3.1. Sample points in northern China
Commonly, field measurement data are collected from multi-pixel regions and summarized statistically (Gillespie et al. Citation2011). The sample areas should be large enough to reduce the effects of measurement imprecision but small enough so that homogeneous areas can be studied (e.g. Sabol et al. Citation2009). Based on this, we selected three pseudo-invariant sand dune sites in China: the Taklimakan Desert in Xinjiang, the Dunhuang Gobi radiometric calibration site in Gansu, and the western desert or sandy region in Inner Mongolia. Many surface emissivity field data have been measured for a variety of surface types. highlights the locations of the separate field campaign sites used in the study and the field observation images in the field sites using three thermal infrared spectrometers.
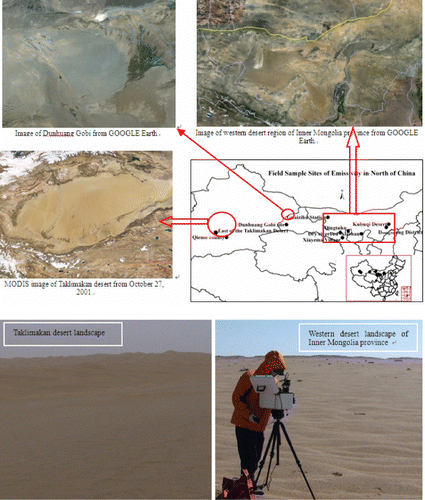
The Taklimakan Desert is the largest active desert in China and the second largest in the world and lies in a depression between two high, rugged mountain ranges (see http://earthobservatory.nasa.gov/IOTD/view.php?id=1925). Located in 72°14′–90°37′E, 36°23′–42°6′N, the middle of the desert has a typical continental climate, with high winds and temperatures and low annual rainfall. In the sand dune areas of the Taklimakan, dune height is generally 100–200 m, but can reach 300 m. The types of sand dunes are compound, sand hills, sand ridges, and tower-type dunes with honeycomb-like, feathery, scaly, and unpredictable surface types. During the day in the Taklimakan, the scorching sun can sometimes raise sand surface temperatures to 70–80°C, so there is strong evaporation.
In the western region of Inner Mongolia, there are two areas of sandy land (Hunshandak sandy land, Mu Us sandy land), three deserts (Kubuqi Desert, Tengger Desert, and BadainJaran Desert), and a large area of grassland undergoing desertification. It is located in 98°23′–111°20′E, 37°17′–42°9′N. From 6–24 April 2010, there was a joint mission of field campaigns lasting 17 days. In this period, the emissivity data of large homogeneous surfaces with different surface types at different sample sites were obtained and processed. These emissivity data were used to validate the LSBE products with the new algorithm.
The Dunhuang satellite radiometric calibration site is located in a suburb of Dunhuang City, Gansu Province, in northwestern China, with the geographic coordinates 40°05′–40°25′N, 94°20′–94°40′E. It covers an area of approximately 400 km2 and rises from 1105 to 1250 m above sea level. The surface is a mixture of sand and gray gravel, and some mixed, product-less vegetation. From 1999 to 2006, reflectance data were obtained continuously at different times and locations at the Dunhuang radiometric calibration site, and it was shown that the changes were relatively stable. The Gobi surface emissivity spectrum of the Dunhuang radiometric calibration site is one of the key pieces of land surface data used to calibrate thermal infrared remote sensors. Hence, field measurement of the emissivity spectrum was conducted every year from 1999 to 2010. Some of these data were used to validate the LSBE products. shows field sample site scope, measured time span, observation conditions, instruments used, and GLASS product time for validation.
Table 1. Lists of field sample sites, time spans, observation conditions, instruments used, and GLASS product times.
3.2. Method of field measurements and calculation
In the field campaigns, flat, relatively uniform areas were selected. A portable Fourier transform thermal infrared spectrometer (102F, D&P Instruments, USA, formerly known as Designs & Prototypes, Ltd.) and a Labsphere gold plate were used to measure spectral radiance emitted by the target and the environment. The spectral range of the spectrometer was 2–16 µm and the spectral resolution was 4, 8, or 16 cm−1, and optionally 2 cm−1. The instrument operates in the temperature range from 15 °C to 35 °C and has a standard aperture lens (field of view angle 4.8°) with an optional 2″ (2.4°), 4″ (1.2°), or 6″ (0.8°) lens with a corresponding hot and cold calibration blackbody. It was mainly used in the Taklimakan Desert and western region of Inner Mongolia from 2010 to 2011. At the Dunhuang radiometric calibration site, the BOMEM MR154 Fourier transform spectroradiometer and CE312 field measurement radiometers were used in different years and Gobi emissivity spectrum data were obtained for different times and locations. CE312 field measurement radiometers and an Infragold board with a known reflectance in the thermal infrared range were used to measure the channel emissivity of the Gobi surface at the Dunhuang calibration site in August 2006 and July 2007. Duplicate measurements were carried out in the afternoon between 14:00 and 15:00 in these months.
In the field, each of the three spectral radiometers needed to be preheated and placed at a height of about 1 m above the ground. After initial calibration with a thermal infrared blackbody at five known temperatures, the measurements of the target radiation and infrared golden board were performed alternately. Before the target was measured, it was important to select a flat area for the sample site where there was no near-surface wind, no clouds, and a relatively stable atmosphere. In order to ensure the measured emissivity was reliable, the target data collection was repeated three times for every observation and three measurements were made at each point. For each observation, measurements of the land surface and the golden board were performed alternately. Emissivity spectral data were obtained at various times and locations. The emissivity spectrum was derived from the radiometric measurements by the iterative spectrally smooth temperature-emissivity separation (ISSTES) algorithm (Xiao et al. Citation2003; Zhang et al. Citation2009). Through a large number of measurements, if the difference of each channel emissivity among different measured times was within 0.02 at each site, those measured emissivities were judged to be reliable and selected for validation. Of course, there was some measured uncertainty. For example, since the change in surface temperature was large and fast in each measurement, especially at noon, it was difficult to measure very accurately. In order to avoid the problem, we conducted the measurement in a time period in which surface temperature change was minimal.
In the central Taklimakan Desert, measurements were conducted at two relatively homogeneous sites on 20–24 April 2011. At each site, we conducted three measurements and then we randomly chose two points within a distance of approximately 500 m from the site and conducted three measurements at each of these points. The nine derived emissivity spectra were then averaged and taken as the emissivity spectrum for the site. A similar process was used in the western region of Inner Mongolia.
In addition, a variety of valuable field observation data were gathered at every sample site, including atmospheric parameters (aerosol, atmospheric optical thickness, etc.), land surface parameters (surface emissivity, ground reflected spectrum, soil texture, surface temperature, soil moisture, and vegetation cover types), and conventional meteorological variables (temperature, humidity, pressure, and wind), among others.
3.3. Results of field measurements
3.3.1. Emissivity of the same land surface type at different sites
At different sites in the central Taklimakan Desert and western region of Inner Mongolia, the surface feature of the experimental measurements was desert or sandy land. shows the changes in the emissivity spectrogram at 8–13.5 µm located in the southwest and east of the Taklimakan Desert. We found that, both in terms of the general trend and the wavelength emissivity values, the differences between the measured emissivity at the two sites were small. The similarity in the sand emissivity in different regions of the central Taklimakan was due to the relatively uniform surface, showing that sand emissivity in the surrounding desert was similar and the measured results are reliable.
shows changes in the emissivity spectra for the same land surface type at different sites in the western region of Inner Mongolia. These sand sites include the Kubuqi Desert, Xiayema Village in Minqin County of Gansu Province, a dry riverbed in Inner Mongolia, and Qingtuhu and Guaizihu stations (). The same trend was observed for each curve in the 8–13 µm band. The largest differences between the measured emissivity spectra were mainly in the 8.3–9.3 µm range. The changes in the emissivity spectra in the 9.3–13.5 µm range were rather consistent. This also shows that the sand emissivity at different sand sites in western Inner Mongolia was similar and the measured results are reliable.
Some studies have found that the emissivity of most natural surfaces on Earth at wavelengths between 8 and 12 µm ranges from 0.65 to close to 1.0. Narrowband emissivity less than 0.85 is typical for most desert and semi-arid areas due to the strong quartz absorption feature in the 8–9.5 µm range (Snyder et al. Citation1998). From the above figure, most of the measured emissivity spectra between 8 and 9.5 µm are lower than 0.85. This phenomenon may be due to some sites being located in the semi-arid areas of the Hexi Corridor in Gansu Province. This further demonstrates that our measurements are very reliable and that these measured results can also be used to verify the GLASS LSBE product.
3.3.2. Emissivity of different land surface types
During the field measurements, many surface types, such as sand, saline-alkali soil, bare soil, and dry grassland in spring were included. shows the measured emissivity spectra between 8 and 13.5 µm for sand in the Taklimakan Desert, saline-alkali soil in Qiemo County of Xinjiang, bare soil in the Alashan regions, and dry grassland in the Dongsheng District of Ordos City, Inner Mongolia.
In , it can be seen that different surface types have different emissivity spectral features, especially in the 8–11 µm range. There is a significant trough in the 8.3–9.3 µm range, and different surface types have different trough shapes and depths, with the emissivity of sand in most deserts being significantly lower than that of the other surface types and the emissivity of dry grassland in spring being the highest. From the changes in the emissivity spectra, the changes in the grassland emissivity are seen to be most stable and those of the sand emissivity change fastest. It has been found that the emissivities of some surface types, such as vegetation, snow and ice, and water, are relatively stable (Salisbury and D'Aria Citation1992; Salisbury, D'Aria, Wald Citation1994). This was also reflected in our observations of the dry grassland emissivity. The LSBE of soil (in the 8–12 µm spectral range) has been shown to change dramatically, ranging from 0.81 to 0.99 (Ogawa et al. Citation2003). Our measured bare soil emissivity varied in the range 0.90 to 0.96 in the 8–13.5 µm spectral range. This may be because of the existence of some grass and sand in bare soil regions.
3.3.3. Comparison of measured emissivity with the spectral library data
In order to better verify the accuracy of the measurement results, the sandy surface and water emissivity spectra were compared with the ASTER JHU (Johns Hopkins University) spectral library (). The three solid lines represent emissivity spectral curves for three samples of the soil (aridisol) from the spectral library. The four dotted lines are the field-measured emissivity spectral curves at four sandy sites (left figure).
Comparing the two types of curves, the trends of the spectral library emissivity curve were consistent with those measured in the field. The emissivity in the 8–9.5 µm range was low and increased dramatically in the 9.5–10.5 µm range and from 10.5 to 13.5 µm it was stable. However, there were still differences between the measured spectral data and the curve from the spectral library, especially in the 8–9.5 µm range. It should be noted that local differences in the measured data and spectral library data show the uniqueness of China's western surface.
We also chose the water emissivity spectrum measured in Qinghai Lake to compare with the ASTER JHU spectral library (right figure). It shows good agreement and demonstrates that our field measurement technology and the accuracy of the field measurement data are reliable.
3.3.4. Emissivity at different times in Dunhuang Gobi
The Gobi surface emissivity spectrum at the Dunhuang radiometric calibration site is important for the validation of the GLASS LSBE product. Based on the ISSTES algorithm, the Dunhuang Gobi surface emissivity spectrum was measured using the BOMEM MR154 Fourier transform spectroradiometer in July and October 2007. Emissivity spectral data were obtained at different times and locations (Zhang et al. Citation2009). These spectral data were convolved with the channel response function of the CE312 radiometer, and the measured LSBE in the 8–13.5 µm range was 0.927 in July 2007 and 0.923 in October 2007. On 18 August 2006, the Dunhuang Gobi surface emissivity was also measured using the CE312 radiometer and the result at 8–13.5 µm was 0.945. The differences between these three-channel emissivities at different times were within 0.018. It shows good consistency and can be used for the LSBE validation with the Dunhuang Gobi surface.
4. Validation and discussion of GLASS LSBE
Sabol et al. (Citation2009) indicated the difficulties of validating emissivity over rock and soil sites for the ASTER emissivity product. As these issues also exist in the GLASS LSBE, we decided to examine the emissivity over homogeneous water and sand in different regions and at different temperatures. The pseudo-invariant sand dune sites in northern China chosen for the validation study include a wide range of materials such as hornblende, pure quartz, calcite, and feldspar and cover a wide range of emissivity in the 8–13.5 µm range. Using the GPS sampling coordinates, emissivity data from the GLASS LSBE products were extracted for each sample site.
For vegetated surfaces, because emissivity can vary significantly with plant species, area density, and growth stage (Snyder et al. Citation1998), it is difficult to measure the vegetation temperature and emissivity in the field, and thus, they have not been used to validate the LSBE products.
For each sand dune site in the western region of Inner Mongolia, the GLASS LSBE false-color visible images are shown in . The visible images give an indication of the size, color, and surrounding environment of the sand dunes, and sampling sites are indicated by a red cross and triangle surrounded by rectangles.
From , we can see that the emissivity of the GLASS LSBE product and measured data in the 8–13.5 µm range are very consistent for Qinghai Lake. For the sandy land in the western region, the inversion results of the GLASS LSBE product were greater than the measured emissivity in the field, and for dry grassland during the winter, inversion results were less than the measured emissivity. On the whole, however, the inversion results and measured emissivity were consistent. The mean and standard deviation (spatial) in emissivity for all sand samples collected were 0.0059 and 0.0114, respectively. The validation results show that GLASS LSBE captures the measured emissivity of all of the dune sands very well. From the right-hand figure, the difference between the GLASS inversion emissivity and the measured data is ±0.02. This indicates that the GLASS inversion emissivity product is sufficiently accurate in the sand dune sites in northern China.
For fully vegetated surfaces, the determination is relatively simple since the emissivity is almost spatially uniform. However, for arid and semi-arid land with sparse vegetation, the determination of emissivity is more difficult and the emissivity of the exposed soil and rock is highly variable (Snyder et al. Citation1998). Hence, we can understand the discrepancies between the GLASS LSBE product and the measured emissivity in the dry or desertified grassland in the western region of Inner Mongolia.
In the center of the Taklimakan Desert, the average emissivity spectra were converted into LSBEs at 8–13.5 µm. The measured LSBEs were 0.915 and 0.913, and the LSBEs computed using the new algorithm were 0.928 and 0.929. The average difference in the GLASS inversion emissivity and the data measured with the 102F instrument was 0.015.
For the Gobi surface at the Dunhuang calibration site, the measured average LSBE using CE312 was 0.945, and the BBE calculated with the new algorithm was 0.946. The difference between the GLASS inversion emissivity and the data measured using the CE312 was 0.001. The measured average LSBEs using the BOMEM MR154 instrument were 0.927 and 0.923. The retrieved LSBE were 0.947 and 0.945. The average difference between the GLASS inversion emissivity and the data measured using the MR154 was 0.021.
Previous studies have found that the surface emissivity depends on various factors, such as water content, chemical composition, structure, roughness, and the observation conditions (Snyder et al. Citation1998). The selected sand dune sites in northern China were mostly distributed in desert regions, such as the Taklimakan Desert, Kubuqi Desert, Dunhuang Gobi, Tengger Desert, BadainJaran Desert, and Hunshandak sandy land. These areas are characterized by almost zero soil moisture, flat surfaces on a 1-km scale, and changes in stability. Therefore, these sites are ideal locations for verification and the results are reliable. In summary, the new LSBE is of high quality if the established inversion algorithm using the relationship between the MODIS albedo and ASTER emissivity is significant. The reason may be that the MODIS albedo is one of the most robust products available and ASTER emissivity performs well for bare soil.
5. Conclusions
The GLASS LSBE has been validated using a set of measured surface emissivity data for bare surfaces at different sand dune sites in northern China. The validation data consist of eight-day averaged LSE data measured using the 102F and CE312 radiometers during the spring (March–April) and autumn (July–October) from 2006 to 2011.
Validation over arid and semi-arid regions and some desert was performed by collecting sand samples from medium to larger sand dune sites in northwestern China (Huo et al. Citation2011). Sand dune sites in the western region of Inner Mongolia, the central Taklimakan Desert, and Dunhuang Gobi have been found to be suitable targets for the validation of LSBE data due to their consistent and homogeneous mineralogy over long time periods.
The field-measured emissivity spectra showed very good agreement in amplitude and spectral shape at these dune sites with each other and with the ASTER JHU spectral library. Comparison of the measured emissivity for the same land surface type at different sites, for different land surface types at the same sites, and for different times in the same regions showed good consistency and demonstrated that our field measurement technology is rather reliable. Hence, the measured emissivity data can be used for LSBE validation in northwestern China.
GLASS LSBE and field emissivity data at different sites showed very good agreement. The mean and standard deviation (spatial) in emissivity in the 8–13.5 µm range for all sand samples collected in the western region of Inner Mongolia were 0.0059 and 0.0114, respectively. The difference between the GLASS inversion emissivity and measured data was ±0.02.
In the center of the Taklimakan Desert, the measured emissivities converted from the average emissivity spectra in the 8–13.5 µm range were 0.915 and 0.913, respectively. The average difference between the GLASS inversion emissivity and data measured with the 102F instrument were within 0.015.
For the Gobi surface at the Dunhuang calibration site, the measured average BBE using the CE312 was 0.945. The difference between the GLASS inversion emissivity and data measured using the CE312 was 0.001. The measured average BBEs using the BOMEM MR154 instrument were 0.927 and 0.923. The average difference between the GLASS inversion emissivity and measured data was within 0.021.
The combined mean emissivity difference between the GLASS LSBE and the field-measured results for all validation sites was about 1.1%. This emissivity difference is equivalent to approximately a 1 K error in the LST for a material at 300 K in the TIR.
In summary, these results indicate that the new algorithm for the GLASS LSBE product works well over arid and semi-arid regions and over most deserts such as the Taklimakan. It opens up the potential for using GLASS LSBE to compare and validate LSBE products from other sensors with much coarser spatial resolutions, such as MODIS (5 km), and to estimate the surface radiation.
Acknowledgements
The research was carried out with the support of China's Research and Development Special Fund for Public Welfare Industry [Meteorology GYHY201206002, GYHY200906022-1, and GYHY201306077]; a key project in the national science & technology pillar program during the Eleventh Five-Year Plan of China titled “Improved integrated observations of experiments to support quantitative sandstorm remote sensing and model prediction (2008BAC40B05)”; and a key project of the National High Technology Research and Development Program (863 Program) titled “Generation and applications of global products of essential land variables [2009AA122103-01].” We kindly thank our experiment team including Dr Zhang Y. et al. for assisting us with fieldwork in the western region of Inner Mongolia and also Dr Cheng J. from Beijing Normal University for providing the GLASS LSBE database from 2006 to 2011.
References
- Barducci, A., and I. Pippi. 1996. “Temperature and Emissivity Retrieval from Remotely Sensed Images Using the “Grey Body Emissivity” Method.” IEEE Transactions on Geoscience and Remote Sensing 34 (3): 681–695. doi:10.1109/36.499748.
- Becker, F., and Z.-L. Li. 1990. “Temperature Independent Spectral Indices in Thermal Infrared Bands.” Remote Sensing of Environment 32 (1): 17–33. doi:10.1016/0034-4257(90)90095-4.
- Caselles, V., C. Coll, E. Valor, and E. Rubio. 1995. “Mapping Land Surface Emissivity Using AVHRR Data: Application to La Mancha, Spain.” Remote Sensing Reviews 12 (3–4): 311–333. doi:10.1080/02757259509532289.
- Cheng, J., and S. Liang. 2012. “A New Algorithm for Estimating Global Bare Soil Broadband Emissivity Using MODIS Albedo.” IEEE Transactions on Geoscience and Remote Sensing. doi:10.1175/1520-0442(2002)015<3123:TLSCOT>2.0.CO;2.
- Cheng, J., and S. Liang, 2013. “Estimating Global Land Surface Broadband Thermal-Infrared Emissivity from the Advanced Very High Resolution Radiometer Optical Data.” International Journal of Digital Earth. doi:10.1080/17538947.2013.783129.
- Cheng, J., S. Liang, Q. Liu, and X. Li. 2011. “Temperature and Emissivity Separation from Ground-Based MIR Hyperspectral Data.” IEEE Transactions on Geoscience and Remote Sensing 49 (4): 1473–1484. doi:10.1109/TGRS.2010.2076818.
- Cheng, J., S. Liang, J. Wang, and X. Li. 2010a. “A Stepwise Refining Algorithm of Temperature and Emissivity Separation for Hyperspectral Thermal Infrared Data.” IEEE Transactions on Geoscience and Remote Sensing 48 (3): 1588–1597. doi:10.1109/TGRS.2009.2029852.
- Cheng, J., S. Liang, F. Weng, J. Wang, and X. Li. 2010b. “Comparison of Radiative Transfer Models for Simulating Snow Surface Thermal Infrared Emissivity.” IEEE Journal of Selected Topics in Applied Earth Observations and Remote Sensing 3 (3): 323–336. doi:10.1109/JSTARS.2010.2050300.
- Cheng, J., S. Liang, Y. Yao, and X. Zhang. 2013. “Estimating the Optimal Broadband Emissivity Spectral Range for Calculating Surface Longwave Net Radiation.” IEEE Geoscience and Remote Sensing Letters 10 (2): 401–405. doi:10.1109/LGRS.2012.2206367.
- Galve, J. M., C. Coll, V. Caselles, and E. Valor. 2008. “An Atmospheric Radiosounding Database for Generating Land Surface Temperature Algorithms.” IEEE Transactions on Geoscience and Remote Sensing 46 (5): 1547–1557. doi:10.1109/TGRS.2008.916084.
- Gillespie, A., S. Rokugawa, S. Hook, T. Matsunaga, and A. Kahle. 1996. Temperature/Emissivity Separation Algorithm. Theoretical Basis Document, Version 2.1. Greenbelt, MD, USA: NASA/GSFC.
- Gillespie, A., S. Rokugawa, T. Matsunaga, J. S. Cothern, S. Hook, and A. B. Kahle. 1998. “A Temperature and Emissivity Separation Algorithm for Advanced Spaceborne Thermal Emission and Reflection Radiometer (ASTER) Images.” IEEE Transactions on Geoscience and Remote Sensing 36 (4): 1113–1126. doi:10.1109/36.700995.
- Gillespie, A. R., E. A. Abbott, L. Gilson, G. Hulley, J. C. Jiménez-Muñoz, J. A. Sobrino. 2011. “Residual Errors in ASTER Temperature and Emissivity Standard Products AST08 and AST05.” Remote Sensing of Environment 115 (12): 3681–3694. doi:10.1016/j.rse.2011.09.007.
- Hulley, G. C., and S. J. Hook. 2009a. “The North American ASTER Land Surface Emissivity Database (NAALSED) Version 2.0.” Remote Sensing of Environment 113 (9): 1967–1975. doi:10.1016/j.rse.2009.05.005.
- Hulley, G. C., and S. J. Hook. 2009b. “Intercomparison of Versions 4, 4.1 and 5 of the MODIS Land Surface Temperature and Emissivity Products and Validation with Laboratory Measurements of Sand Samples from the Namib Desert, Namibia.” Remote Sensing of Environment 113 (2009): 1313–1318.
- Huo, W., Q. He, X. H. Yang, X. C. Liu, G. F. Ding, and Y. J. Cheng. 2011. “The Research on Grain Size Characteristic of Desert in North of China.” Research of Soil and Water Conservation 18 (6): 6–11. ( In Chinese).
- Jacob, F., F. Petitcolin, T. Schmugge, E. Vermote, K. Ogawa, and A. French. 2004. “Comparison of Land Surface Emissivity and Radiometric Temperature from MODIS and ASTER Sensors.” Remote Sensing of Environment 83: 1–18.
- Jin, M., and S. Liang. 2006. “An Improved Land Surface Emissivity Parameter for Land Surface Models Using Global Remote Sensing Observations.” Journal of Climate 19 (12): 2867–2881. doi:10.1175/JCLI3720.1.
- Li, Z.-L., and F. Becker. 1993. “Feasibility of Land Surface Temperature and Emissivity Determination from AVHRR Data.” Remote Sensing of Environment 43 (1): 67–85. doi:10.1016/0034-4257(93)90065-6.
- Li, Z.-L., H. Wu, N. Wang, S. Qiu, J. A. Sobrino, Z. Wan, B.-H. Tang, and G. Yan. 2013. “Land Surface Emissivity Retrieval From Satellite Data.” International Journal of Remote Sensing 34 (9–10): 3084–3127. doi:10.1080/01431161.2012.716540.
- Liang, S. 2004. Quantitative Remote Sensing of Land Surface. New Jersey: John Wiley and Sons.
- Liang, S., W. Kustas, G. Schaepman-Strub, and X. Li. 2010. “Impacts of Climate Change and Land Use Change on Land Surface Radiation and Energy Budgets.” IEEE Journal of Selected Topics in Applied Earth Observations and Remote Sensing 3 (3): 219–224. doi:10.1109/JSTARS.2010.2053570.
- Momeni, M., M. R. Saradjian. 2007. “Evaluating NDVI-Based Emissivities of MODIS Bands 31 and 32 Using Emissivities Derived By Day/Night LST Algorithm. Remote Sensing of Environment 106 (2): 190–198. doi:10.1016/j.rse.2006.08.005.
- Nerry, F., F. Petitcolin, and M. P. Stoll. 1998. “Bidirectional Reflectivity in AVHRR Channel 3: Application to a Region in Northern Africa.” Remote Sensing of Environment 66 (3): 298–316. doi:10.1016/S0034-4257(98)00066-2.
- Norman, J. M., and F. Becker. 1995. “Terminology in Thermal Infrared Remote-Sensing of Natural Surfaces.” Agricultural and Forest Meteorology 77 (3–4): 153–166. doi:10.1016/0168-1923(95)02259-Z.
- Ogawa, K., and T. Schmugge. 2004. “Mapping Surface Broadband Emissivity of the Sahara Desert Using ASTER and MODIS Data.” Earth Interactions 8 (7): 1–14. doi:10.1175/1087-3562(2004)008<0001:MSBEOT>2.0.CO;2.
- Ogawa, K., T. Schmugge, F. Jacob, and A. French. 2002. “Estimation of Broadband Land Surface Emissivity from Multispectral Thermal Infrared Remote Sensing.” Agronomie: Agriculture and Environment 22: 695–696. doi:10.1051/agro:2002055.
- Ogawa, K., T. Schmugge, F. Jacob, and A. French. 2003. “Estimation of Land Surface Window (8–12 m) Emissivity from Multi-Spectral Thermal Infrared Remote Sensing—A Case Study in a Part of Sahara Desert.” Geophysical Research Letters 30 (2): 1067–1071. doi:10.1029/2002GL016354.
- Ogawa, K., T. Schmugge, and S. Rokugawa. 2008. “Estimating Broadband Emissivity of Arid Regions and its Seasonal Variations Using Thermal Infrared Remote Sensing.” IEEE Transactions on Geoscience and Remote Sensing 46 (2): 334–343. doi:10.1109/TGRS.2007.913213.
- Pequignot, E., A. Chedin, and N. A. Scott. 2008. “Infrared Continental Surface Emissivity Spectra Retrieved From AIRS Hyperspectral Sensor.” Journal of Applied Meteorology and Climatology 47: 1619–1633. doi:10.1175/2007JAMC1773.1.
- Petitcolin, F., F. Nerry, and M.-P. Stoll. 2002a. “Mapping Directional Emissivity at 3.7 Using a Simple Model of Bi-Directional Reflectivity.” International Journal of Remote Sensing 23 (17): 3443–3472. doi:10.1080/01431160110075569.
- Petitcolin, F., F. Nerry, and M.-P. Stoll. 2002b. “Mapping Temperature Independent Spectral Indices of Emissivity and Directional Emissivity in AVHRR Channels 4 and 5.” International Journal of Remote Sensing 23 (17): 3473–3491. doi:10.1080/01431160110075578.
- Petitcolin, F., and E. Vermote. 2002. “Land Surface Reflectance, Emissivity and Temperature from MODIS Middle and Thermal Infrared Data.” Remote Sensing of Environment 83 (1–2): 112–134. doi:10.1016/S0034-4257(02)00094-9.
- Ren, H. Z., S. L. Liang, G. J. Yan, and J. Cheng. 2012. “Empirical Algorithms to Map Global Broadband Emissivities Over Vegetated Surfaces.” IEEE Transactions on Geoscience and Remote Sensing 1–13. doi:10.1109/TGRS.2012.2216887.
- Sabol, D. E., Jr., A. R. Gillespie, E. Abbott, and G. Yamada. 2009. “Field Validation of the ASTER Temperature-Emissivity Separation Algorithm.” Remote Sensing of Environment 113 (11): 2328–2344. doi:10.1016/j.rse.2009.06.008.
- Salisbury, J. W., and D. M. D'Aria. 1992. “Emissivity of Terrestrial Materials in the 8–14 µm Atmospheric Window.” Remote Sensing of Environment 42 (2): 83–106. doi:10.1016/0034-4257(92)90092-X.
- Salisbury, J. W., D. M. D'Aria, and A. Wald. 1994. “Measurements of Thermal Infrared Spectral Reflectance of Frost, Snow, and Ice.” Journal of Geophysical Research 99 (B12): 24235–24240. doi:10.1029/94JB00579.
- Schmugge, T., A. French, J. C. Ritchie, A. Rango, and H. Pelgrum. 2002. “Temperature and Emissivity Separation from Multispectral Thermal Infrared Observations.” Remote Sensing of Environment 79 (2–3): 189–198. doi:10.1016/S0034-4257(01)00272-3.
- Schmugge, T., S. J. Hook, and C. Coll. 1998. “Recovering Surface Temperature and Emissivity from Thermal Infrared Multispectral Data.” Remote Sensing of Environment 65 (2): 121–131. doi:10.1016/S0034-4257(98)00023-6.
- Schmugge, T., and K. Ogawa. 2006. “Validation of Emissivity Estimates from ASTER and MODIS Data.” In Proceeding of: Geoscience and Remote Sensing Symposium, 260–262. Las Cruces, NM: New Mexico State University. doi:10.1109/IGARSS.2006.71.
- Schmugge, T., K. Ogawa, F. Jacob, A. French, A. Hsu, and J. C. Ritchie. 2003. “Validation of Emissivity Estimates from ASTER Data.” Proceeding of: Geoscience and Remote Sensing Symposium, 1873–1875. USDA Hydrology & Remote Sensing Lab, August. doi:10.1109/IGARSS.2003.1294278 ISBN: 0-7803-7929-2.
- Sellers, P. J., R. E. Dickinson, and D. A. Randall. 1997. “Modeling the Exchange of Energy, Water and Carbon between the Continents and the Atmosphere.” Science 275 (5299): 502–509. doi:10.1126/science.275.5299.502.
- Snyder, W. C., Z. Wan, Y. Zhang, and Y.-Z. Feng. 1998. “Classification Based Emissivity For Land Surface Temperature Measurement From Space.” International Journal of Remote Sensing 19 (14): 2753 2774. doi:10.1080/014311698214497.
- Sobrino, J. A., and N. Raissouni. 2000. “Toward Remote Sensing Methods for Land Cover Dynamic Monitoring: Application to Morocco.” International Journal of Remote Sensing 21 (2): 353–366. doi:10.1080/014311600210876.
- Sobrino, J. A., N. Raissouni, and Z. L. Li. 2001. “A Comparative Study of Land Surface Emissivity Retrieval from NOAA Data.” Remote Sensing of Environment 75 (2): 256–266. doi:10.1016/S0034-4257(00)00171-1.
- Susskind, J., C. D. Barnet, and J. M. Blaisdell. 2003. “Retrieval of Atmospheric and Surface Parameters from AIRS/AMSU/HSB Data in the Presence of Clouds.” IEEE Transactions on Geoscience and Remote Sensing 41 (2): 390–409. doi:10.1109/TGRS.2002.808236.
- Tang, B.-H., H. Wu, C. Li, and Z.-L. Li. 2011. “Estimation of Broadband Surface Emissivity from Narrowband Emissivities.” Optics Express 19 (1): 185–192. doi:10.1364/OE.19.000185.
- Valor, E., and V. Caselles. 1996. “Mapping Land Surface Emissivity from NDVI: Application to European, African, and South American Areas.” Remote Sensing of Environment 57 (3): 167–184. doi:10.1016/0034-4257(96)00039-9.
- Van De Griend, A. A., and M. Owe. 1993. “On the Relationship Between Thermal Emissivity and the Normalized Difference Vegetation Index For Natural Surfaces.” International Journal of Remote Sensing 14 (6): 1119–1131. doi:10.1080/01431169308904400.
- Wan, Z. 2008. “New Refinements and Validation of the MODIS Land-Surface Temperature/Emissivity Products.” Remote Sensing of Environment 112 (1): 59–74. doi:10.1016/j.rse.2006.06.026.
- Wan, Z., and Z.-L. Li. 1997. “A Physics-Based Algorithm for Retrieving Land-Surface Emissivity and Temperature from EOS/MODIS Data.” IEEE Transactions on Geoscience and Remote Sensing 35 (4): 980–996. doi:10.1109/36.602541.
- Watson, K. 1992. “Two-Temperature Method for Measuring Emissivity.” Remote Sensing of Environment 42 (2): 117–121. doi:10.1016/0034-4257(92)90095-2.
- Wilber, A. C., D. P. Kratz, and S. K. Gupta. 1999. Surface Emissivity Maps for use in Satellite Retrievals of Longwave Radiation. Hampton, Virginia: NASA Tech Publ NASA/TP-1999-209362. Accessed March 23, 2013. http://www.cs.odu.edu/~mln/ltrs-pdfs/NASA-99-tp209362.pdf.
- Xiao, Q., Q. H. Liu, X. W. Li, L. F. Chen, Q. Liu, X. Z. Xin. 2003. “A Field Measurement Method of Spectral Emissivity and Research on the Feature of Soil.” Journal of Infrared Millimeter Waves 22 (5): 373–378. (In Chinese).
- Zhang, Y., Y. Li, Z. G. Rong, X. Q. Hu, L. J. Zhang, J. J. Liu. 2009. “Field Measurement of Gobi Surface Emissivity Spectrum at Dunhuang Calibration Site of China.” Spectroscopy and Spectral Analysis 29 (5): 1213–1217. (In Chinese).
- Zhou, L., R. Dickinson, K. Ogawa, Y. Tian, M. Jin, and T. Schmugge. 2003. “Relations between Albedos and Emissivities from MODIS and ASTER Data Over North African Desert.” Geophysical Research Letters 30 (20): 2026–2029. doi:10.1029/2003GL018069.