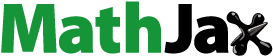
ABSTRACT
Arctic sea ice and its snow cover are important components of the cryosphere and the climate system. A series of in situ snow measurements were conducted during the seventh Chinese Arctic expedition in summer 2016 in the western Arctic Ocean. In this study, we made an analysis of snow features on Arctic sea ice based on in situ observations and the satellite-derived parameter of snow grain size from MODIS spectral reflectance data. Results indicate that snow depth on Arctic sea ice varied between 19 and 241 mm, with a mean value of 100 mm. The mean density of the snow was 340.4 kg/m3 during the expedition, which was higher than that reported in previous literature. The measurements revealed that a depth hoar layer was widely developed in the snow, accounting for 30%∼50% of the total snow depth. The equivalent snow grain size was small on the surface and large at the bottom in snow pits. The average relative error between MODIS-derived snow grain size and in situ measured surface snow grain size is 14.6%, indicating that remote sensing is a promising method to obtain large-scale information of snow grain size on Arctic sea ice.
1. Introduction
Arctic sea ice has undergone rapid changes in recent decades, as substantiated by the reduction in its extent and thickness, loss of multi-year ice coverage, and intensification of snow melting on sea ice (Comiso Citation2011; Laxon et al. Citation2013; Wang et al. Citation2019). Arctic sea ice and its snow cover are important components of the cryosphere and the climate system (Webster et al. Citation2018; Meredith et al. Citation2019). Snow on Arctic sea ice is the most direct response to climate change and has a significant impact on the process of Earth’s radiation and energy transmission, which influences the growth-melting process of sea ice and the heat exchange process of the atmosphere-ocean (Webster et al. Citation2014; Rösel et al. Citation2018). Snow is the primary atmospheric source input to Arctic sea ice, with characteristics of high albedo and low thermal conductivity, which has a significant impact on the growth and melting process of Arctic sea ice (Eicken Citation2004; Kern et al. Citation2015; Sturm and Massom Citation2016).
Field studies and direct observations of snow on Arctic sea ice are of great significance to characterize the mechanisms of Arctic sea ice and climate change. Snow cover on sea ice significantly modifies the physical and radiative properties of the variable sea ice environment, which modulates the sensitivity and response of sea ice to anthropogenic warming (Ledley Citation1991; Webster et al. Citation2018). However, in situ data on the snow on Arctic sea ice are very sparse (Cheng et al. Citation2008; Meredith et al. Citation2019). From 1937 to 1991, the Soviet Union operated the series of ‘North Pole’ drifting stations on Arctic sea ice. By using these data, Warren et al. (Citation1999) gave a climatic distribution of monthly snow depth and snow density on Arctic sea ice, which provided early snow observational dataset for climatic studies. In 2008, the third Chinese Arctic expedition was carried out in the summer. Dou and Xiao (Citation2013) reported the physical characteristics of snow cover on western Arctic sea ice during the third Chinese Arctic expedition. It supplemented multiple snow information on Arctic sea ice in the summer season, however, snow measurements with multiple parameters did not carry out during subsequent Chinese expeditions until the seventh Chinese Arctic expedition. Rösel et al. (Citation2018) presented sea ice and snow thickness measurements during the N-ICE2015 expedition from January to June of 2015 in the Atlantic sector of the Arctic Ocean north of Svalbard. They focused on the snow parameter of depth on sea ice and compared it with that measured by snow buoys. Information on snow parameters is not only crucial for the development of detailed snow numerical models but also important for developing remote sensing retrieval algorithms (Shokr and Sinha Citation2015). However, there is still a lack of knowledge of snow information (not only snow depth but also snow parameters of density, stratigraphy, grain size, etc.) on Arctic sea ice, especially during the summer of the snow melting period (Stocker et al. Citation2013; Meredith et al. Citation2019).
Snow grain size is a fundamental parameter of snow, which has a great impact on snow albedo that plays a key role in the earth’s radiation balance (Domine et al. Citation2008). The thermodynamic state of snow is related to snow grain size because of the metamorphism determined by gradients of temperature and vapor (Lyapustin et al. Citation2009). Snow grain size affects the radiation balance of snow and ice, which is an important parameter for the climatological model (Zege et al. Citation2008). Satellite remote sensing data could obtain large-scale information on snow grain size on Arctic sea ice. The core idea of the retrieval method for snow grain size based on remote sensing data is to establish the relationship between snow grain size and the optical spectrum. A commonly used retrieval model is the Analytical Asymptotic Radiative Transfer (AART) model (Kokhanovsky and Zege Citation2004; Lyapustin et al. Citation2009; Carlsen et al. Citation2017). Scambos et al. (Citation2007) retrieved snow grain size for the Antarctic continent from Moderate Resolution Imaging Spectroradiometer (MODIS) data by applying the AART model. Lyapustin et al. (Citation2009) conducted a similar study on retrieving snow grain size over Greenland. A comparison of MODIS-derived snow grain size in central Antarctica with in situ measurements was carried by Carlsen et al. (Citation2017). These snow grain size retrieval studies focused on the snow on the polar ice sheet. Compared to the snow on the Antarctic continent and Greenland, snow on Arctic sea ice is thin and fast-changing, which put forward a question of whether the remote sensing retrieval method original used for land snow grain size could be applied to the retrieval of snow grain size on the Arctic sea ice.
The first objective of this paper is to present an analysis of snow features on Arctic sea ice, consisting of in situ measurements of snow depth, snow density, snow stratigraphy, and snow grain size during the seventh Chinese Arctic expedition in summer 2016. These precious measurement data are all potentially useful for the validation of model simulation and satellite remote sensing retrieval. Another objective is the validation of AART model for the Arctic application of snow grain size on sea ice. The retrieved large-scale snow grain size on Arctic sea ice using satellite data would be assessed against the in situ measurements during the seventh Chinese Arctic expedition.
2. Data and methods
2.1. In situ snow observations during the seventh Chinese Arctic expedition
The seventh Chinese Arctic expedition was based on vessel R/V Xuelong from 11 July 2016 to 26 September 2016. During the expedition, six short-term ice stations (3∼4 hours each) and one long-term ice station (8 days) had been implemented (). They distributed in the Pacific Arctic sector (including the Chukchi Sea and the Central Arctic), spanning 6 latitudes and 19 longitudes.
Figure 1. Cruise tracks of the seventh Chinese Arctic expedition with ice station (IS) location and identification (numbers and date (MMDD)); LIS denotes the 8-day long-term ice station from 7 August to 15 August.
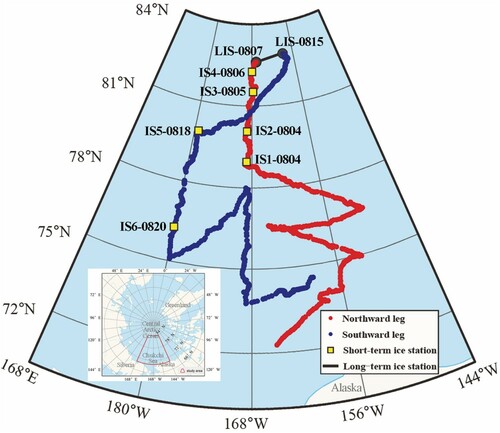
Based on these ice stations, we measured snow parameters of snow depth, snow density, snow stratification, and snow grain size. The instruments used to measure these snow parameters are shown in . A total of 12 snow lines and 171 sample points of snow depth were performed using a steel ruler with an accuracy of 1 mm (). Snow density was measured using a dielectric-sensor instrument (LK-1604 Snow Fork produced by Toikka Company in Finland). The Snow Fork is normally used in a sampling configuration where the prongs are inserted horizontally into the side of a snow pit. The prongs can also be placed on the snow surface for a dielectric half-space measurement or inserted into the snow surface at a 30° or 45° angle (Kinar and Pomeroy Citation2015). The Snow Fork measures the real and imaginary parts of the snow permittivity for density calculation with the accuracy of 0.02 and 0.002, respectively. We obtained 422 records of snow density during the expedition. The description of a snow profile in the snowpit usually involved the stratification and grain size within each layer. A total of 81 records of snow grain size were obtained by the portable electron microscope (Anyty-WM461 microscope produced by 3R Company in Japan). The Anyty-WM461 microscope was connected and controlled by the computer, which was used to record the micro photo of the snow in different layers placed on the checkerboard during the field measurements. The length of the long half axis () and short half axis (
) of an assumed ellipsoid (
and
is the volume and surface area of the ellipsoid, respectively) that equivalent to the snow could be measured by using 3R imaging processing soft, and then we could calculate equivalent snow grain size using the following equations (Grenfell and Warren Citation1999). The Anyty-WM461 microscope with 3R imaging processing soft could measure the axial length of an assumed ellipsoid that is equivalent to the snow with the accuracy of 1 μm.
(1)
(1)
(2)
(2)
(3)
(3)
Figure 2. Instruments used for observations of (a) snow depth, (b) snow density of surface layer (0∼3 cm), (c) snow density sub-surface layer (greater than 3 cm), and (d) snow grain size during the seventh Chinese Arctic expedition.
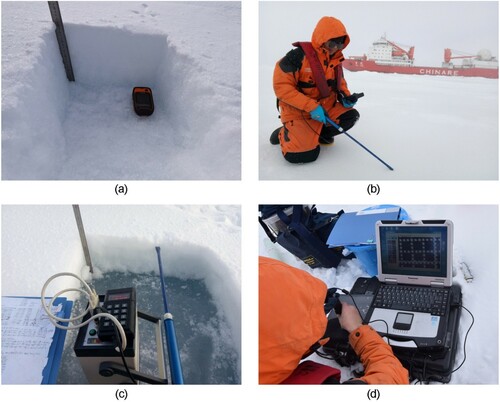
Table 1. General information for snow measurements on sea ice in ice stations during the seventh Chinese Arctic expedition.
It should be noted that the spatial variation of snow cover on sea ice is affected by the ice topography. We implemented different measurement schemes according to the ice situations at ice stations during the seventh Chinese Arctic expedition. For the situation of quasi-cyclic ice hillocks or ridges surrounding flat sea ice, the measurements of snow parameters were generally carried out near the ice ridges and away from wind erosion areas. For the situation of flat sea ice with few ice hillocks or ridges, we selected measurement sites on the snow line near and parallel to the ice fringe. It is generally accepted that the average value of observations on an ice station measured in this way could represent the local distribution of snow parameters (Dou and Xiao Citation2013).
2.2. MODIS spectral reflectance data and auxiliary data
We used spectral reflectance data to retrieve snow grain size on Arctic sea ice during the summer of 2016. Spectral reflectance data originated from MODIS Level 3 data products (MOD09A1), which are composite data of the 8-day spectral reflectance from MODIS band 1 to band 7 at a 500 m resolution. The composite data used in this study is to cover gaps caused by clouds in the study area. We downloaded the MOD09A1 data from 11 July to 11 August 2016 from NASA on the website of https://search.earthdata.nasa.gov/search?q=MOD09A1%20V006.
The microwave remote sensing data product of AMSR2 (Advanced Microwave Scanning Radiometer 2) sea ice concentration (SIC) was used as the auxiliary data to extract sea ice pixels (SIC≥15%) and calculate the snow grain size within these ice pixels. AMSR2 sea ice concentration data were acquired from the University of Bremen (https://seaice.uni-bremen.de/sea-ice-concentration/amsre-amsr2/).
2.3. AART model application for snow grain size on Arctic sea ice
The spectral reflectance of snow varies significantly with wavelength (Wiscombe and Warren Citation1980). It is high in the visible-light band (0.4∼0.7 μm) and drops dramatically in the near-infrared band (0.7∼3.0 μm). Therefore, we could use the relationship between the snow optical properties and physical properties established by the radiation transmission model to retrieve the snow grain size.
In this study, snow grain size on Arctic sea ice was explored to retrieve from MODIS data by using the AART model proposed by Kokhanovsky and Zege (Citation2004). AART applies the analytical asymptotic solution of radiative transfer in semi-infinite media with low absorption to obtain snow reflectance:
(4)
(4) where
denotes snow reflectance,
is a solution of the radiative transfer equation for the snow with zero absorption, which can be can be acquired from the lookup table given by Suttles et al. (Citation1988).
,
and
stand for cosines of solar and view zenith angles, and relative azimuth, respectively, which can be acquired from MODIS data.
is the imaginary part of the refractive index of ice,
is the wavelength, and
is an effective grain size defined by the ratio of the average volume to the average surface area of grains. The function
relates to the photon’s escape probability from the medium. Because the wavelength used for snow grain size retrieval is much smaller than
, Lyapustin et al. (Citation2009) assumed that
does not depend on the size of snow grain for simplicity, and then
can be expressed as:
(5)
(5) where
is the calibration constant depending on the type of snow. It equals 0.66 for fractal snow grains (Kokhanovsky and Zege Citation2004).
To reduce the effect of snow surface roughness, we applied the band-ratio method combined with the AART model to retrieve snow grain size from MODIS spectral reflectance data. In the band-ratio algorithm, the role of the is reduced to the second-order effect manifested through the function
(Lyapustin et al. Citation2009). We could then obtain snow grain size from Equation (4) by using the combination of a non-absorbing band with an absorbing band:
(6)
(6) where
is the reflectance of MODIS band 1 with
= 0.645 μm,
is the reflectance of MODIS band 5 with
= 1.242 μm, and the imaginary part (
) of the complex refractive ice index corresponding to band 5 is 1.20 e−5 (Warren and Brandt Citation2008). AART model was original used for the land snow grain size retrieval, there is a difference in the underlying surface when applying AART model for snow grain size on Arctic sea ice. Meanwhile, the Arctic Ocean has a lower solar elevation angle and a higher solar zenith angle (SZA). Since the AART model is limited by the condition that SZA≤78°, the pixel with SZA>78° is therefore set as a null value. To obtain accurate snow grain size values, it is necessary to remove invalid values in the study area using a snow cover mask. The snow mask can be calculated by the Normalized Difference Snow Index (NDSI), which is defined as follows:
(7)
(7) where
and
represent the reflectance of MODIS band 4 and band 6, respectively. The pixel with
and
is recognized as the snow pixel.
3. Results and discussion
3.1. Snow depth
Snow depth measured at six short-term ice stations (IS1 to IS6) and one long-term ice station (LIS) during the seventh Chinese Arctic expedition was plotted in . The measured snow depth varied between 19 and 188 mm with a mean value of 101 mm from all short-term ice stations (a). (b) shows the snow depth measured along the snow line at LIS on 8, 9, 10, 11, and 13 August. The mean snow depth increased from 105 mm on 8 August to 138 mm on 13 August. This was caused by the snowfall events observed during the period (on 10 and 13 August) at the LIS.
Figure 3. Variations of snow depth with the latitude during the seventh Chinese Arctic expedition at (a) short-term ice and (b) long-term ice stations. The vertical bars reflect the value range of the measured snow depth at each ice station. The vertical bars reflect the value range of the measured snow depth at each ice station.
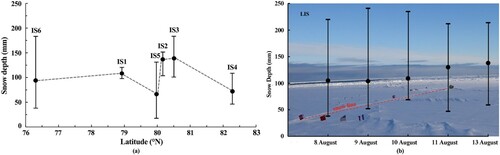
Generally, there are two possible uncertainties contained in the snow measurements, including the accuracy of the instrument and the measurement error caused by the observer. During the seventh Chinese Arctic expedition, we used a steel ruler with an accuracy of 1 mm to measure snow depth on the sea ice, but identifying the interface of snow and ice depends on the experience of the observer. Therefore, we measured snow depth more than three times to calculate the average value at each sample for reducing the in situ measurement error.
3.2. Snow density
Mean snow density from all measured samples during the seventh Chinese Arctic expedition was 340.4 kg/m3, with a value of 315.8 kg/m3 at short-term ice stations and that of 350.6 kg/m3 at long-term ice station. There was an obvious difference between the density of the surface snow layer (0∼3 cm) and the sub-surface snow layer (greater than 3 cm) in the measurement snow pits (). The observed density of the surface snow layer during the expedition was 281.4 kg/m3, and that of the sub-surface snow layer was 390.5 kg/m3, indicating that snow density in the surface layer was much lower than that of the sub-surface layer, conforming to the principle of densification of snow cover.
Figure 4. Variations of snow density at the surface layer and sub-surface layer of the snow with the latitude during the seventh Chinese Arctic expedition. The vertical bars reflect the value range of the measured snow density at each ice station.
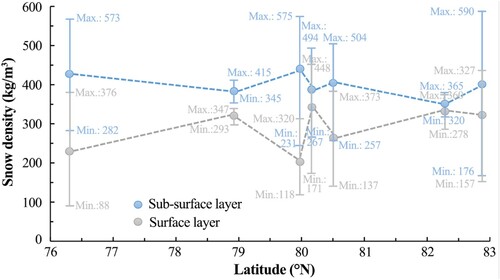
The instrument of Snow Folk was used to efficiently measure snow density on the sea ice. Uncertainty of snow density measurements using Snow Folk was reported 5 kg/m3 that related to the snow temperature and liquid water content (Moldestad Citation2005). However, we found the mean difference between the snow density measurements using the Snow Fork and using a triangular snow gravimetric sampler (Kinar and Pomeroy Citation2015) during the seventh Chinese Arctic expedition was about 15 kg/m3. The larger uncertainty of snow density may be caused by the liquid water content in the snow on summer Arctic sea ice.
Snow density is greatly affected by weather events on a daily scale. Two snowfalls and a freezing rainfall occurred during the snow measurements at the long-term station. The snowfall events led to the low surface value of snow density on 10 August (reduced by 27.1 kg/m3 compared to 9 August measured in the same locations) and 13 August (reduced by 78.0 kg/m3 compared to 12 August measured in the same locations), while the freezing rainfall event on 8 August made the high measured value of surface snow density observed on 9 August (increased by 35.9 kg/m3 compared to 8 August measured in the same locations) because of surface snow infiltration and increase in water content.
Representative work in the study of snow on Arctic sea ice was reported by Warren et al. (Citation1999). Some typical values of snow parameters given in the paper are still in use today. Warren et al. (Citation1999) reported the value of snow density was 220 ± 40 kg/m3 in August. The snow density from our measurements during the seventh Chinese Arctic expedition was 340.4 ± 42.8 kg/m3, which is much higher than that given by Warren et al. (Citation1999). This may be due to the accelerated summer melting of snow and ice in the context of Arctic warming in recent years (Meredith et al. Citation2019). Dou and Xiao (Citation2013) conducted the snow measurements during the third Chinese Arctic expedition and reported the mean density of snow was 304.00 ± 29.0 kg/m3. The value of snow density we observed (340.4 kg/m3) was very similar to the value (349 kg/m3) observed in the N-ICE2015 (Merkouriadi et al. Citation2017), and higher than that reported by Dou and Xiao (Citation2013). Global warming accelerated Arctic summer melting of snow and ice. Severe ablation would substantially increase the liquid water content in the snow on sea ice (Kwok and Cunningham Citation2008), leading to the observed density with greater value in the recent expedition.
3.3. Snow stratigraphy
shows snow stratigraphy in snow pits at different ice stations. The snow cover was composed of six snow types: new snow, wind slab, young snow, depth hoar, refreezing coarse snow, and wet snow (Colbeck et al. Citation1992; Sturm and Holmgren Citation1995; Sturm, Holmgren, and Perovich Citation2002). Snow stratigraphy showed inhomogeneity in the spatial variations. In general, it had the following basic features. First, the snow surface was usually covered with new snow or wind slab (1∼2 cm). New snow had the characteristics of soft and low density, while the wind slab was hard and smooth. Second, a depth hoar layer was widely developed in the snow, accounting for 30%∼50% of the total snow depth. The formation of depth hoar is closely linked to the vertical distribution of snow temperature, resulting in a water vapor pressure gradient in the snow layer. The sublimation of water vapor crystallizes in the upper part of the snow layer to form the depth hoar (Dou and Xiao Citation2013). Third, the bottom of the snow layer was usually refreezing coarse snow or wet snow (2∼4 cm). It could be inferred the wet snow layer was formed by the melting at the interface of snow and ice, which was probably caused by the absorption of short wave radiation there because the positive ice freeboard was observed when we removed the snow on the ice in the snow pit.
Figure 5. A schematic column of snow stratigraphy observed during the seventh Chinese Arctic expedition.
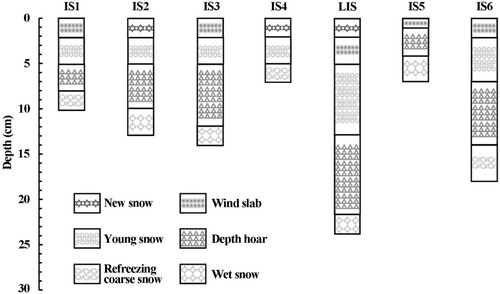
The snow stratigraphy we observed during the seventh Chinese Arctic expedition is comparable with that given by Dou and Xiao (Citation2013). We confirmed that the depth hoar layer was widely developed in the snow on Arctic sea ice, but accounting for a higher ratio to the total snow depth than that reported by Dou and Xiao (Citation2013) measured in CHIANRE-2008 (20%) and Merkouriadi et al. (Citation2017) measured in N-ICE2015 (28%). This reflects the melting-sublimation-crystallization effect in the snow has been strengthened compared to the previous observations. It should be noted that visual observations of the snow stratigraphy were related to the experience of the observer. Although we have been trained before snow stratigraphy measurements, the subjectivity on identifying different snow layers in snow pits accounts for the uncertainty of snow stratigraphy measurements.
3.4. Snow grain size
3.4.1. Snow grain size measured during the seventh Chinese Arctic expedition
Snow grain size measurements were conducted at six ice stations (IS1 to IS5 and LIS). During the measurements of snow grain size, the Anyty-WM461 microscope with 3R imaging processing soft could measure the axial length of an assumed ellipsoid that is equivalent to the snow with the accuracy of 1 μm. To reduce the measurement error, a micrometer was used to make the length calibration before each measurement. shows the statistics of equivalent snow grain size in each snow pit during the seventh Chinese Arctic expedition. The equivalent snow grain size was small on the surface and large at the bottom along with the snow stratigraphic profiles. This is mainly because the bottom of the snow layer was usually the refreezing coarse snow or wet snow caused by the melting and refreezing processes at the interface of the snow and ice.
Figure 6. Snow grain size measured during the seventh Chinese Arctic expedition. (a) The equivalent snow grain size of the surface layer (0∼3 cm) and the sub-surface layer (greater than 3 cm) in snow pits, the minimum and maximum values of measured surface and sub-surface snow grain size were labeled using blue and red numbers, respectively; (b) Micro-photos of the different snow types, the red line and marks were the in-situ measured long half axis (a) length and short half axis (b) length of an assumed ellipsoid equivalent to the snow grain which could be used to calculate snow grain size based on Equation (2) and Equation (3).
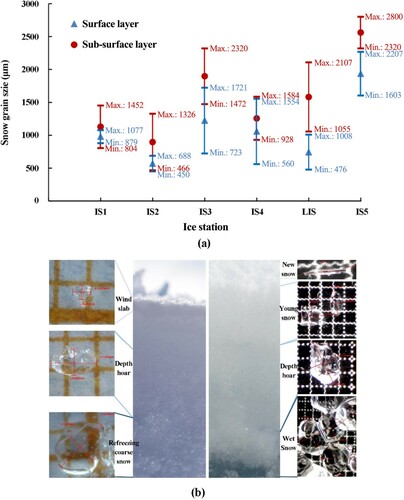
3.4.2. Snow grain size derived from MODIS
shows the spatial distribution of snow grain size on western Arctic sea ice derived from composite data of the 8-day spectral reflectance from MODIS. The range of MODIS-derived snow grain size was 0∼2000 μm, which was consistent with field-measured surface snow grain size. The average snow grain size in the study area generally decreased from mid-July to early August and then increased to mid-August. During the seventh Chinese Arctic expedition, snowfall events were observed between 29 July and 1 August, coinciding with a sudden decrease in the MODIS-derived snow grain size from 27 July to 3 August. Besides the influence of weather events, the snow grain size of the study area was also affected by the snow melting process. In mid-July, the high values of snow grain size were mainly distributed in the low-latitude, while in mid-August, snow on Arctic sea ice melt accelerated. Snow grain size presented a significant increase in the high-latitude areas (d). This can be explained by the increase of radiation energy received on the snow surface, resulting in high water content in snow, making snow grain sintered together. Snow grain size on Arctic sea ice is sensitive to the weather conditions, leading to a large and complex spatial–temporal variability, however, snow grain size derived from remote sensing data could reflect climate events to some extent and provide useful information for snow numerical models and climate models.
Figure 7. Spatial distribution of MODIS-derived snow grain size on Arctic sea ice from (a) 11 July to 18 July, (b) 19 July to 26 July, (c) 27 July to 3 August, and (d) 4 August to11 August. The locations of in-situ ice stations for snow grain size measured were presented in (d).
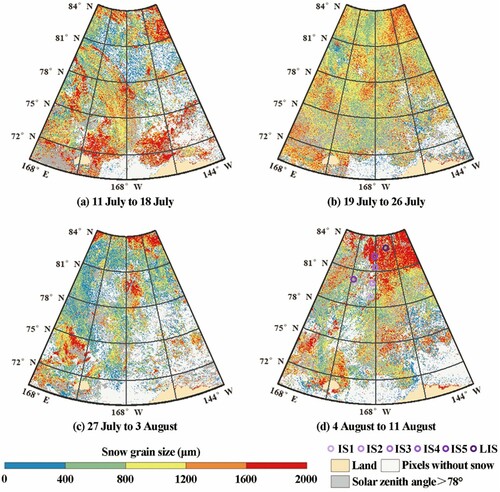
We compared the mean surface snow grain size measured at each ice station during the seventh Chinese Arctic expedition with the matching MODIS-derived snow grain size according to the in situ measurement location and time (). The relative error (RE) used in this study as the validation index was defined: , where, abs() represents the absolute value function,
is the value of MODIS-derived snow grain size, and
is the average value of the in situ measured snow grain size at each ice station. We then calculated the averaged relative error (ARE). The ARE between MODIS-derived snow grain size and in situ measured snow grain size was 14.6%, indicating that snow grain size retrieved from MOIDS is an acceptable method. The snow grain size retrieved from MODIS presented a slightly lower value than in situ measured snow grain size, mainly because MODIS could incorrectly recognize the thin cloudy area as cloudless pixels, resulting in underestimation of the value of snow grain size (Intrieri et al. Citation2002). However, AART model application for snow grain size is a promising method to obtain large-scale snow grain size information on Arctic sea ice from satellite data, because the snow grain size on Arctic sea ice does not change very rapidly and the spatial distribution is small.
4. Conclusion
This paper presents the results of station-based measurements of snow depth, snow density, snow stratigraphy, as well as snow grain size on Arctic sea ice during the seventh Chinese Arctic expedition. The combined information from all those data provides portray of snow layout on Arctic sea ice in summer. Observations show that snow depth varied between 19 and 241 mm with a mean value of 100 mm from all measurement records. Mean snow density on Arctic sea ice was 340.4 kg/m3 during the expedition, which was very similar to the value (349 kg/m3) observed in the N-ICE2015 (Merkouriadi et al. Citation2017), and higher than that reported by Warren et al. (Citation1999) and Dou and Xiao (Citation2013). This may be related to the accelerated summer melting of snow in the context of Arctic warming in recent years. Snow stratigraphy observed in snow pits was composed of six snow types: new snow, wind slab, young snow, depth hoar, refreezing coarse snow, and wet snow. We confirmed that the depth hoar layer was widely developed in the snow on Arctic sea ice, but accounting for a higher ratio to the total snow depth than that reported by Dou and Xiao (Citation2013) and Merkouriadi et al. (Citation2017).
In situ measurement of snow grain size presented the feature that snow grain size was small on the surface and large at the bottom along with the snow stratigraphic profiles. Snow grain size on Arctic sea ice at a large scale was explored to retrieve from MODIS data by using the AART model. The average relative error between MODIS-derived snow grain size and in situ measured surface snow grain size is 14.6%, indicating that the snow grain size retrieving method based on the AART model is a promising method to obtain large-scale snow grain size information on Arctic sea ice from satellite data. The average snow grain size on sea ice in the western Arctic Ocean generally decreased from mid-July to early August and then increased to mid-August. Noted that we averaged the in situ measurements of each ice station and compared them with MODIS retrieved snow grain size at collocated MODIS pixel scale. The scale differences account for the discrepancy of in situ measured snow grain size and MODIS-derived snow grain size. More snow measurement data during the Arctic expeditions need to be collected to improve the accuracy of retrieving snow grain size from remote sensing data for further work.
Geolocation information
The study area in this paper is the western Arctic Ocean, including the Chukchi Sea and the Central Arctic.
Disclosure statement
No potential conflict of interest was reported by the author(s).
Data availability statement
The data that support the findings of this study are available from the corresponding author, upon reasonable request.
Additional information
Funding
References
- Carlsen, T., G. Birnbaum, A. Ehrlich, J. Freitag, G. Heygster, L. Istomina, S. Kipfstuhl, A. Orsi, M. Schafer, and M. Wendisch. 2017. “Comparison of Different Methods to Retrieve Optical-Equivalent Snow Grain Size in Central Antarctica.” The Cryosphere 11 (6): 2727–2741. doi:https://doi.org/10.5194/tc-11-2727-2017.
- Cheng, B., Z. Zhang, T. Vihma, M. Johansson, L. Bian, Z. Li, and D. Wu. 2008. “Model Experiments on Snow and ice Thermodynamics in the Arctic Ocean with CHINARE 2003 Data.” Journal of Geophysical Research 113: C09020. doi:https://doi.org/10.1029/2007JC004654.
- Colbeck, C. S., E. Akitaya, R. Armstrong, H. Gubler, J. Lafeuille, K. Lied, D. McClung, and E. Morris. 1992. “The International Classification for Seasonal Snow on the Ground.” In The International Commission on Snow and Ice of the International Association of Scientific Hydrology-International Glaciology Society. U. S. Army CRREL, LASH Report MP 2794, Hanover, NH.
- Comiso, J. C. 2011. “Large Decadal Decline of the Arctic Multiyear Ice Cover.” Journal of Climate 25 (4): 1176–1193. doi:https://doi.org/10.1175/JCLI-D-11-00113.1.
- Domine, F., M. Albert, T. Huthwelker, H. W. Jacobi, A. A. Kokhanovsky, and M. Lehning. 2008. “Snow Physics as Relevant to Snow Photochemistry.” Atmospheric Chemistry and Physics 8: 171–208. doi:https://doi.org/10.5194/acp-8-171-2008.
- Dou, T., and C. Xiao. 2013. “Measurements of Physical Characteristics of Summer Snow Cover on Sea Ice During the Third Chinese Arctic Expedition.” Sciences in Cold and Arid Regions 5 (3): 309–315. doi:https://doi.org/10.3724/SP.J.1226.2013.00309.
- Eicken, H. 2004. “Hydraulic Controls of Summer Arctic Pack Ice Albedo.” Journal of Geophysical Research 109 (C8): C08007. doi:https://doi.org/10.1029/2003JC001989.
- Grenfell, T. C., and S. G. Warren. 1999. “Representation of a Nonspherical ice Particle by a Collection of Independent Spheres for Scattering and Absorption of Radiation.” Journal of Geophysical Research 104 (D24): 31697–31709. doi:https://doi.org/10.1029/1999JD900496.
- Intrieri, J. M., M. D. Shupe, T. Uttal, and B. J. McCarty. 2002. “An Annual Cycle of Arctic Cloud Characteristics Observed by Radar and LIDAR at SHEBA.” Journal of Geophysical Research Oceans 107 (C10): 1–5. doi:https://doi.org/10.1029/2000jc000423.
- Kern, S., K. Khvorostovsky, H. Skourup, E. Rinne, Z. Parsakhoo, V. Djepa, P. Wadhams, and S. Sandven. 2015. “The Impact of Snow Depth, Snow Density and Ice Density on Sea Ice Thickness Retrieval from Satellite Radar Altimetry: Results from the ESA-CCI Sea Ice ECV Project Round Robin Exercise.” Cryosphere 9: 37–52. doi:https://doi.org/10.5194/tc-9-37-2015.
- Kinar, N. J., and J. W. Pomeroy. 2015. “Measurement of the Physical Properties of the Snowpack.” Reviews of Geophysics 53 (2): 481–544. doi:https://doi.org/10.1002/2015RG000481.
- Kokhanovsky, A., and E. P. Zege. 2004. “Scattering Optics of Snow.” Applied Optics 43: 1589–1602. doi:https://doi.org/10.1364/AO.43.001589.
- Kwok, R., and G. F. Cunningham. 2008. “ICESat Over Arctic Sea Ice: Estimation of Snow Depth and Ice Thickness.” Journal of Geophysical Research 113: C08010. doi:https://doi.org/10.1029/2008JC004753.
- Laxon, S. W., K. A. Giles, A. L. Ridout, D. J. Wingham, R. Willatt, R. Cullen, R. Kwok, et al. 2013. “CryoSat-2 Estimates of Arctic Sea Ice Thickness and Volume.” Geophysical Research Letters 40 (4): 732–737. doi:https://doi.org/10.1002/grl.50193.
- Ledley, T. S. 1991. “Snow on Sea Ice: Competing Effects in Shaping Climate.” Journal of Geophysical Research 96 (D9): 17195–17208. doi:https://doi.org/10.1029/91JD01439.
- Lyapustin, A., M. Tedesco, Y. Wang, T. Aoki, M. Hori, and A. Kokhanovsky. 2009. “Retrieval of Snow Grain Size Over Greenland from MODIS.” Remote Sensing of Environment 113 (9): 1976–1987. doi:https://doi.org/10.1016/j.rse.2009.05.008.
- Meredith, M. P., M. Sommerkorn, S. Cassotta, C. Derksen, A. Ekaykin, A. Hollowed, G. Kofinas, et al. 2019. “Chapter 3: Polar Regions. IPCC Special Report on the Ocean and Cryosphere in a Changing Climate.” Intergovernmental Panel on Climate Change: Special Report on the Ocean and Cryosphere in a Changing Climate.
- Merkouriadi, I., J.-C. Gallet, R. M. Graham, G. E. Liston, C. Polashenski, A. Rösel, and S. Gerland. 2017. “Winter Snow Conditions on Arctic Sea Ice North of Svalbard During the Norwegian Young Sea ICE (N-ICE2015) Expedition.” Journal of Geophysical Research Atmosphere 122: 10837–10854. doi:https://doi.org/10.1002/2017JD026753.
- Moldestad, D. A. 2005. “Characteristics of Liquid Water Content and Snow Density in a Cross-Country Race Ski Track.” Bulletin of Glaciological Research 22: 39–49.
- Rösel, A., P. Itkin, J. King, D. Divine, C. Wang, M. A. Granskog, T. Krumpen, and S. Gerland. 2018. “Thin Sea Ice, Thick Snow, and Widespread Negative Freeboard Observed During N-ICE2015 North of Svalbard.” Journal of Geophysical Research Ocean 123: 1156–1176. doi:https://doi.org/10.1002/2017JC012865.
- Scambos, T. A., T. M. Haran, M. A. Fahnestock, T. H. Painter, and J. Bohlander. 2007. “MODIS-Based Mosaic of Antarctica (MOA) Data Sets: Continent-Wide Surface Morphology and Snow Grain Size.” Remote Sensing of Environment 111 (2): 242–257. doi:https://doi.org/10.1016/j.rse.2006.12.020.
- Shokr, M., and N. Sinha. 2015. Sea Ice: Physics and Remote Sensing. Washington DC: American Geophysical Union.
- Stocker, T. F., D. Qin, G. K. Plattner, M. Tignor, S. K. Allen, J. Boschung, A. Nauels, Y. Xia, B. Bex, and B. Midgley. 2013. “Climate Change 2013: The Physical Science Basis. Contribution of Working Group I to the fifth assessment report of the Intergovernmental Panel on Climate Change.” Cambridge: Cambridge University Press.
- Sturm, M., and J. Holmgren. 1995. “A Seasonal Snow Cover Classification System for Local to Global Applications.” Journal of Climate 8 (5): 1261–1283. doi:https://doi.org/10.1175/1520-0442.
- Sturm, M., J. Holmgren, and D. K. Perovich. 2002. “Winter Snow Cover on the Sea Ice of the Arctic Ocean at the Surface Heat Budget of the Arctic Ocean (SHEBA): Temporal Evolution and Spatial Variability.” Journal of Geophysical Research 107 (C10): 8047. doi:https://doi.org/10.1029/2000JC000400.
- Sturm, M., and R. A. Massom. 2016. Snow in the Sea Ice System: Friend or Foe? John Wiley & Sons, Ltd., Oxford, U. K.
- Suttles, J. T., R. N. Green, P. Minis, I. J. Walker, D. F. Yong, V. R. Taylor, and L. L. Stowe. 1988. Angular Radiation Models for Earth-Atmosphere System. Hampton, Virginia: NASA Langley Research Center.
- Wang, Q., Z. Li, P. Lu, R. Lei, and B. Cheng. 2019. “2014 Summer Arctic Sea Ice Thickness and Concentration from Shipborne Observations.” International Journal of Digital Earth 12 (8): 1814–1829. doi:https://doi.org/10.1080/17538947.2017.1421720.
- Warren, S. G., and R. E. Brandt. 2008. “Optical Constants of ice from the Ultraviolet to the Microwave: A Revised Compilation.” Journal of Geophysical Research 113: D14220. doi:https://doi.org/10.1029/2007JD0-09744.
- Warren, S. G., I. G. Rigor, N. Untersteiner, V. F. Radionov, N. N. Bryazgin, Y. I. Aleksandrov, and R. Colony. 1999. “Snow Depth on Arctic Sea Ice.” Journal of Climate 12 (6): 1814–1829. doi:https://doi.org/10.1175/1520-0442.
- Webster, M., S. Gerland, M. Holland, E. Hunke, R. Kwok, O. Lecomte, R. Massom, D. Perovich, and M. Sturm. 2018. “Snow in the Changing Sea-Ice Systems.” Nature Climate Change 8: 946–953. doi:https://doi.org/10.1038/s41558-018-0286-7.
- Webster, M. A., I. G. Rigor, S. V. Nghiem, N. T. Kurtz, S. L. Farrell, D. K. Perovich, and M. Sturm. 2014. “Interdecadal Changes in Snow Depth on Arctic Sea Ice.” Journal of Geophysical Research Oceans 119 (8): 5395–5406. doi:https://doi.org/10.1002/2014JC009985.
- Wiscombe, W. J., and S. G. Warren. 1980. “A Model for the Spectral Albedo of Snow. I Pure Snow.” Journal of the Atmospheric Sciences 37 (12): 2712–2733. doi:https://doi.org/10.1175/1520-0469(1980)037.
- Zege, E., I. Katsev, A. Malinka, A. Prikhach, and I. Polonsky. 2008. “New Algorithm to Retrieve the Effective Snow Grain Size and Pollution Amount from Satellite Data.” Annals of Glaciology 49 (1): 139–144. doi:https://doi.org/10.3189/172756408787815004.