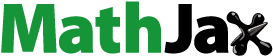
Abstract
The existing studies on persistence of ecological footprint have largely concentrated on the aggregate ecological footprint without adequately considering its components. The aim of this paper is to contribute to the existing papers on ecological footprint by examining the persistence of carbon footprint emissions for a group of 92 countries. Unlike the extant papers, this paper uses fractional integration, which allows the consideration of stationary I(0) and nonstationary I(1) cases as particular models of interest, being therefore more general and flexible than these two classical representations. The results indicate that only 25 of the 92 countries display mean reversion, with orders of integration strictly below 1 and showing transitory shocks, most of them belonging to lower middle- and low-income countries. In these 25 countries it might not be easy to change the long-run path of the carbon footprint as any policy shocks will have temporary effects. The foregoing findings can help policymakers in each nation to design efficient emission-reducing policies.
Introduction
One of the main obstacles to achieving sustainability is the biophysical confines of the planet. The Earth is essentially a closed system, and therefore it is subject to thermodynamic laws that place restrictions on the production of natural resources as well as the absorption of waste. However, over the past five decades, humanity has, to a great extent, ensured that economic activities have continued to expand, even while natural resources are gradually becoming limited [Citation1]. Therefore, there is a need to use and analyze relevant tools to determine the degree to which mankind’s demand surpasses or remains within the limits of what the Earth’s natural capital can accommodate, and to detect early warning signs and possibly project the impacts of human-driven pressures on ecosystems [Citation2]. One such tool is the carbon footprint. Generally, the carbon footprint is a component of the ecological footprint, and it denotes the hectares of land needed to sequester anthropogenic carbon dioxide emissions. It accounts for more than 60% of the total ecological footprint around the globe [Citation3].
Many aspects of the carbon footprint have not been adequately analyzed in the literature, including the stationarity of the series. There are several reasons why determining whether the carbon footprint follows a nonstationary path or a stationary trend is important. First, the existence of unit roots means that shocks to carbon footprints arising from the deployment of more fuel-efficient transportation and equipment and resulting from energy price jumps or exogenous innovation will be permanent [Citation4]. Second, the nonstationarity of the carbon footprint series has vital implications for the environmental Kuznets curve (EKC) papers that have used the carbon footprint as a representation of pollution. The assumption of trend stationarity of the pollution indicators is present in some EKC studies [Citation5]. However, an EKC study that uses a nonstationary carbon footprint series in levels as the dependent series is likely to suffer from spurious inference when the explanatory variables such as output or income are also nonstationary. Specifically, if the time series for environmental indicators contain a stochastic trend, statistical approaches including ordinary least squares (OLS) that are based on the assumption that the series are stationary could generate spurious regression inferences. Therefore, the standard diagnostic statistics which are employed to gauge OLS results will suggest a statistically meaningful relation among non-stationary time series in most cases even when there is no relationship between the data-generating processes [Citation6,Citation7]. In the conventional autoregressive distributed lag (ARDL) method of Pesaran et al. [Citation8], it is assumed that all variables included in the regression involving environmental indicators should be at most stationary at first differences. Third, the division between trend stationary and difference stationary processes is vital for evaluating the potential long-term effect of environmental policy, which depends on the projection of future emissions and appraising the precision of these forecasts. For both nonstationary and stationary series, the long-term forecasts are the extrapolated deterministic trend. Forecast uncertainty for a nonstationary series increases as the forecast horizon becomes more distant. Stationary series are not susceptible to forecast uncertainty. Therefore, the long-term effects of a policy are less certain when the series are nonstationary relative to the stationary case [Citation9]. Fourth, if the carbon footprint series at level are difference stationary, there is no prospect of convergence between them (as they are at different levels), so any conclusion of convergence on the relative carbon footprint is at best weak [Citation10].
The literature on the stationarity of pollution indicators has predominantly focussed on per capita CO2 emissions [e.g. Citation11–14]. However, per capita CO2 emissions and carbon footprint are different. Carbon footprint is expressed in terms of hectares of land, while per capita emissions are related to the total population of a country. Unlike the typical per capita emissions available in many databases, the carbon footprint incorporates goods that are imported into a country but are produced elsewhere, and generally takes into account emissions associated with international transport and shipping. Consequently, a nation’s carbon footprint can rise even if the CO2 emissions within its borders decrease.
The aim of this paper is to make two contributions to the literature on the stationarity of pollution series. It first examines the stationarity of the carbon footprint in 92 countries, which is likely to offer novel information on an indicator that has been largely ignored in the literature. This study is different from papers that have considered the stationarity of ecological footprints [Citation15–18]. Both Solarin and Bello [Citation16] and Ozcan et al. [Citation15] considered the ecological footprint but not the carbon footprint. Although Ulucak and Lin [Citation17] considered the carbon footprint, their focus was on the US and OCED. The features of carbon footprints vary across countries, and therefore policies that are fit for the US or OECD might not necessarily be suitable for other countries. Hence, the results from the current exercises are likely to guide many countries on whether their policymakers should implement environmental policies to decrease their carbon footprint or allow the internal dynamics of these countries to automatically address the increase in their carbon footprint.
The second contribution is the use of fractional integration techniques, which according to the authors’ knowledge has not been used in the past to examine stationarity of carbon footprints or even ecological footprints. The fractional integration technique is appropriate in this context first because it generalizes the standard methods based on integer degrees of differentiation, allowing for fractional values. Thus, it allows for a much greater degree of flexibility in the dynamic specification of the data; Second, it can provide us with information about the nature of the shocks, being transitory if the order of integration is smaller than 1, but permanent if that value is equal to or higher than 1.
The remainder of the paper is arranged as follows: the next section reviews the existing literature, while the data and methodology of the paper are discussed in the third section. The results are presented in the fourth section. The fifth section concludes the paper.
Literature review
There is an abundant literature on the stationarity and nonstationarity of CO2 emissions. Aldy [Citation19] and Lee and Chang [Citation20] show the convergence of the CO2 emissions of industrialized countries of the OECD, and the non-stability of the less-developed countries using different methodologies, such as the Markov chain transition matrix and the panel data stationarity testing procedure developed by Carrion-i-Silvestre et al. [Citation21], respectively. Other studies have used unit root tests to check the convergence hypothesis by testing the stationarity/nonstationarity of the CO2 emissions. Examples include the works by Ezcurra [Citation22], Panopoulou and Pantelidis [Citation23], Chang and Lee [Citation24], Romero-Ávila [Citation25], Lee et al. [Citation26], Yavuz and Yilanci [Citation27] and Ahmed et al. [Citation28], and the conclusions reached in these works are mixed.
The stationarity of CO2 emissions has been established in Christidou et al. [Citation11]. They find evidence supporting stationarity for 33 countries covering the period 1870–2006 using a nonlinear panel unit root test developed by Kapetanios, Shin, and Snell [Citation29]. Evidence of convergence on the per capita global carbon dioxide emissions is also found in Zhang et al. [Citation30]. On the other hand, there is a significant number of papers obtaining evidence in favor of the non-stationarity of CO2 emissions, some of them focussing on the convergence of CO2 emissions between countries. Thus, for example, the study of Criado and Grether [Citation31] covers the time period 1960–2002, and they investigate the long-run behavior of the per capita CO2 emissions in a panel of 162 areas of the world, finding evidence in favor of nonstationarity, flattening and right-skewed spatial distributions before the oil price shocks in the 1970s, and more stable and symmetric patterns between 1980 and 2000. Other studies showing that CO2 emissions follow unit root processes include Herrerias [Citation32], Li and Lin [Citation33] and Presno et al. [Citation34]. Along the same lines, Jaunky [Citation35] shows that carbon dioxide emissions for high-income countries are integrated of order 1 – that is, I(1). Yamazaki et al. [Citation36] also show that per capita CO2 emissions in OECD countries follow a unit root process.
Using fractional integration, Barros et al. [Citation37] study the global series of CO2 emissions and the series of each of its five components (gas, liquids, solids, cement production and gas flaring), as well as global per capita emissions for a long span of data. They empirically show that the series are nonstationary with orders of integration significantly above 1. However, Belbute and Pereira [Citation38], using a similar methodology, concluded that the aggregate world CO2 emissions and its five components are stationary and mean reverting, though exhibiting long-term memory patterns.
The ecological footprint (EF) is another environmental indicator that has aroused the interest of researchers. Ulukak and Apergis [Citation39] investigate the convergence of the per capita ecological footprint using the club clustering methodological approach for the case of the EU countries; Jia et al. [Citation40] improve the forecasting capacity of the EF indicator with the standard autoregressive integrated moving average (ARIMA) model; Solarin and Bello [Citation16] also showed the nonstationarity of EF. On the other hand, Ozcan et al. [Citation15], using unit root tests and Sequential Panel Selection Method (SPSM) procedures, found stationarity of EF for all high-income countries and for about the half of the low-income and upper middle-income countries, while nonstationarity was found for the lower middle-income economies.
Few studies have focused on the carbon footprint. Studies of the carbon footprint were only found in research on EF analyzed in its components. Ulucak and Lin [Citation17] analyzed the stochastic behavior of the EF and its components (carbon footprint, cropland, grazing land, forest products, built-up-land, and fishing grounds) for the US. Using Fourier unit root tests, they concluded that the cropland footprint and biocapacity are stationary, whereas the ecological footprint, carbon footprint, grazing land footprint, forest footprint, built-up land footprint, fishing grounds footprint and ecological deficit are nonstationary. Yilanci et al. [Citation18], using a technique developed by Bahmani-Oskooee et al. [Citation41], studied the unit root properties of the ecological footprint and its six components using panel data from 25 OECD countries for the time period 1961–2013. Their results indicate that five EF components show stationary properties, but the footprint of fishing grounds does not have mean-reverting behavior. Solarin and Bello [Citation16] provided evidence for nonstationarity of the EF, while Ozcan et al. [Citation15] concluded that the series is stationary.
From the above literature, a lack of consensus is apparent about the stationary/nonstationary nature of CO2 emissions including carbon footprint. However, all except two of these works [Citation37, Citation38] use techniques that characterize the series as stationary I(0) or nonstationary I(1), not allowing for fractional degrees of differentiation, which is the approach used in this work. , .
Data and methodology
Carbon footprint
Carbon footprint is calculated by utilizing several parameters, namely domestic electricity consumption and the combustion of fossil fuels, embodied carbon in traded items, a country’s contribution to the world’s international transport emissions and also non-fossil fuel sources of emissions [Citation42]. The total amount of CO2 emissions apportioned to a particular country is expressed in terms of global hectares by using the footprint intensity of carbon. The carbon footprint is derived from the following:
(1)
(1)
where
is the carbon footprint of a country;
is the yearly anthropogenic CO2 emissions;
is the share of carbon that is taken by the oceans;
is the yield of the productive land that is needed to capture the CO2 emissions; and
is an equivalence term for carbon as a land type.
is an adjustment term for temporal variations in yield from forests.
The annual carbon footprint dataset was generated in per capita global hectares and its components from the database of the Global Footprint Network [Citation3]. This article concentrates on 92 countries for the period 1961 to 2014, these being the only countries with a dataset available for the entire period.1
Methodology
As mentioned above, this article uses techniques based on fractional integration. For this purpose an integration of order 0 or I(0) process must first be defined. A given process {ut, t = 0, ± 1, …} is I(0) if the infinite sum of its autocovariances is finite. The white noise and the stationary and invertible AutoRegressive Moving Average (ARMA) processes can be included in this group. Having said this, a process {xt, t = 0, ± 1, …} is integrated of order d (and denoted as I(d)) if in taking its d differences the process becomes I(0). That is,
(2)
(2)
where L is the lag operator (i.e. Lxt = xt-1), d can be any real value (thus allowing for fractional values), and ut is I(0) as defined above. Then, the polynomial on the left side in EquationEquation (2)
(2)
(2) can be expressed in terms of its binomial expansion, such that, for all real d:
and thus:
In this context, d plays a crucial role, since it will be an indicator of the degree of dependence of the series. Thus, the higher the value of d is, the higher the level of association will be between the observations. These processes were introduced in the 1980s by Granger [Citation43,Citation44], Granger and Joyeux [Citation45] and Hosking [Citation46], and the first applications with aggregate data appear in the 1990s [e.g. Citation47–49]. Nowadays, they are widely applied in many different disciplines including environmental studies [e.g. Citation50,Citation51]. Note that they are very flexible in the sense that depending on the value of d, different processes can be characterized, such as anti-persistence (d < 0), short memory or I(0) behavior (d = 0); stationary long-memory processes (0 < d < 0.5), nonstationary though mean-reverting behavior (0.5 ≤ d < 1); unit roots or I(1) (d = 1); or even explosive models (d > 1). Moreover, d plays a crucial role in the determination of the nature of the shocks; if d is smaller than 1 shocks will have a transitory nature, contrary to what happens if d ≥ 1 where shocks will be permanent.
The differencing parameter d is estimated using the Whittle function in the frequency domain [Citation52]. In particular, a testing approach developed by Robinson [Citation53] is used, that is very convenient in the context of the present paper. It is a Lagrange multiplier (LM) test that is based on the following model,
(5)
(5)
where yt is the observed data, zt is a (kx1) vector of deterministic terms that might include, for instance, an intercept and time trends, and the regression errors xt are the same as in EquationEquation (1)
(1)
(1) and thus, potentially fractional. The tested null hypothesis is:
(6)
(6)
in the equations given by (5) and (1) where do in equation (6) can be any real value. As mentioned above, the test has several advantages: First, it is valid for any real value d0, thus including stationary (d0 < 0.5) but also nonstationary (d0 ≥ 0.5) hypotheses, with no need of preliminary differencing in the latter case; also, the limiting distribution is standard normal, and thus, it is not necessary to rely on numerical values obtained by Monte Carlo simulation studies as is the case with most unit root procedures; moreover, this limit behavior holds independently of the deterministic terms used in EquationEquation (3)
(5)
(5) and the way of modelling the I(0) disturbances ut in (1); finally, the test is the most efficient one in the Pitman sense against local departures from the null. The functional form of the test statistic can be found in Gil-Alana and Robinson [Citation48].
Empirical results
As a preliminary step in this section, standard unit root methods are applied to the 92 series. The tests of Dickey and Fuller [Citation54] and Elliot et al. [Citation55] were used, and the results (not reported) produced evidence in favor of unit roots in the majority of the cases. This, however, is not surprising, since these methods, along with others such as those of Kwiatkowski et al. [Citation56], Phillips and Perron [Citation57]; Ng and Perron [Citation58], focus exclusively on the I(0)/I(1) dichotomy and do not consider fractional alternatives. Moreover, it is a well-known fact that all these unit root methods have extremely low power if the alternatives are of a fractional form [e.g. Citation59–61]. Therefore, this section begins by considering the following model:
(7)
(7)
where yt is the time series being observed; α and β are the coefficients, referring respectively to the intercept and the time trend; and d is the differencing parameter.
displays the estimated values of d (and the 95% confidence bands of the non-rejection values of d using Robinson’s [Citation53] tests), under the three standard cases of no deterministic terms, an intercept, and an intercept with a linear time trend. The selected model for each series is indicated in bold in the table.
Table 1. Estimates of d for each series.
The time trend is required in a number of cases (33 countries, summarized in ), while an intercept is sufficient to describe the deterministic part in the rest of the countries. Along with the estimated values of d, the 95% confidence intervals of the non-rejection values of d, calculated using Robinson’s [Citation53] approach, are also reported. focusses on the estimated coefficients for each country. The second column displays the estimates of d, and columns 3 and 4 report the intercept and the time trend coefficients, respectively.
Table 2. List of countries with significant time trend coefficients.
Table 3. Estimated coefficients for the selected models.
A summary of the results is presented across and . displays the list of countries that show significant time trends. The highest coefficient corresponds to South Korea, followed by Malaysia, Austria and Israel. The table includes a column with a number referring to the income level: 1 for high-income, 2 for upper middle-income, 3 for lower middle-income, and 4 for low-income countries (see Appendix 2 for the classification of the countries). shows that most of the countries displays time trends referred to categories 3 and 4.
Table 4. Classification of countries according to the estimates of d.
summarizes the results in in terms of the estimated values of d. The results are grouped into three categories. The first group refers to the countries belonging to the ‘mean reversion’ case (i.e. countries with an order of integration significantly smaller than 1); the second group refers to those countries showing evidence of unit roots (i.e. with orders of integration around 1); and the third group refers to countries with ‘explosive’ behavior and orders of integration significantly above 1. In terms of the ‘mean reverting’ countries, most of them belong to category 4 (low-income countries), and just the opposite happens to the group with explosive behavior, with most countries belonging to category 1 (high-income countries). Thus, the degree of persistence seems to be highly correlated with the income level of the countries, and those with the lowest income are related to lower degrees of persistence.
Concluding comments
In this paper we have examined the stationary/nonstationary properties of the carbon footprint emissions in a group of 92 countries for the time period 1961–2014. For this purpose, we have used techniques based on the concept of fractional integration, which is more general than the standard methods based on integer degrees of differentiation (0 for stationarity and 1 for nonstationarity). Allowing the order of integration to be fractional allows us to study cases of nonstationarity though with mean reverting behavior if the differencing parameter is in the interval [0.5, 1].
The results reject the hypothesis of stationarity I(0) in all cases, but the I(1) hypothesis is also rejected in many countries; we find evidence of mean reversion (d < 1) in some countries, and evidence of explosive behavior (d > 1) in others. Thus, the use of classical methods based on the strong I(0)/I(1) dichotomy may produce erroneous conclusions regarding the properties of the series. We show that only 25 out of the 92 countries show mean reversion, most of them belonging to lower middle-income (7) or low-income (10) countries. The mean reversion in these countries implies that shocks to the carbon footprint are temporary. The carbon footprint will move back toward its initial mean or trend after experiencing a natural or economic shock. Hence, policymakers should not use excessive targets when the carbon footprint momentarily departs from the trend path, as environmental management and conservation policies aimed at decreasing the carbon footprint do not have no durable effects. The internal dynamics of these economies will tend to offer some hindsight to revert the carbon footprint to its initial trend path. Hence, excessive interference from the political authorities will be a misfit under this scenario.
At the other extreme, the highest degrees of persistence are observed in high-income countries. Economic or natural shocks hitting their carbon footprint will have long-lasting effects as the carbon footprint may not be able to move back to its original trend mean or path after such a shock.
Thus, the authorities in these economies should introduce environmental programs to decrease the footprint arising from human activities. These strategies include imposing a carbon tax to thwart carbon overuse and providing subsidies for the use of green energy sources. As the industrialized economies have developed better strategies to address the problems of carbon footprint, developing nations should imitate the steps that have been taken by the advanced nations.
One limitation of this study is that we have not considered the other elements of ecological footprints. Beyond the carbon footprint, the aggregate ecological footprint has five more components, which include fishing grounds, cropland, forest land, built-up land and grazing land footprints. Hence, future studies can take up the challenge of examining the persistence of the other subsets of the ecological footprint, which will provide more insights on ecological footprint dynamics for each country that has been covered in this paper. In addition, the possibility of structural breaks is an issue that was considered in this work. This is relevant, and wenote that various authors have shown that fractional integration and structural breaks are intimately related [e.g. Citation62, Citation63]. Although many methods have been proposed in recent years [e.g. Citation64–68], this is something that should be examined in future papers.
Acknowledgements
Luis A. Gil-Alana gratefully acknowledges financial support from the Ministerio de Ciencia y Tecnología (ECO2017-85503-R). Comments from the Editor and four anonymous reviewers are gratefully acknowledged.
Disclosure statement
No potential conflict of interest was reported by the authors.
Notes
1 See Appendix 1 for the definitions of the country abbreviations used in the tables.
References
- Mancini MS, Galli A, Niccolucci V, et al. Ecological footprint: refining the carbon footprint calculation. Ecol Indic. 2016;61:390–403. doi:10.1016/j.ecolind.2015.09.040.
- Moldan B, Janoušková S, Hák T. How to understand and measure environmental sustainability: indicators and targets. Ecol Indic. 2012;17:4–13. doi:10.1016/j.ecolind.2011.04.033.
- Global Footprint Network. (2018). Global foot print network database. Available from http://data.footprintnetwork.org/countryTrends.html
- McKitrick R. Why did US air pollution decline after 1970? Empirical Econ. 2007;33:491–513. doi:10.1007/s00181-006--4.
- Sidneva N, Zivot E. Evaluating the impact of environmental policy on the trend behavior of US emissions of nitrogen oxides and volatile organic compounds. Nat Resour Model. 2014;27:311–337. doi:10.1111/nrm.12036.
- Dergiades T, Kaufmann RK, Panagiotidis T. Long-run changes in radiative forcing and surface temperature: the effect of human activity over the last five centuries. J Environ Econ Manage. 2016;76:67–85. doi:10.1016/j.jeem.2015.11.005.
- Hendry DF, Juselius K. Explaining cointegration analysis. Energy J. 2000;21:1–42.
- Pesaran MH, Shin Y, Smith RJ. Bounds testing approaches to the analysis of level relationships. J Appl Econ. 2001;16:289–326. doi:10.1002/jae.616.
- Gil-Alana LA, Solarin SA. Have US environmental policies been effective in the reduction of US emissions? A new approach using fractional integration. Atmos Pollut Res. 2018;9:53–60. doi:10.1016/j.apr.2017.06.008.
- Nieswiadomy ML, Strazicich MC. Are political freedoms converging? Econ Inquiry. 2004;42:323–340. doi:10.1093/ei/cbh064.
- Christidou M, Panagiotidis T, Sharma A. On the stationarity of per capita carbon dioxide emissions over a century. Econ Modell. 2013;33:918–925. doi:10.1016/j.econmod.2013.05.024.
- Gil-Alana LA, Cunado J, Gupta R. Persistence, mean-reversion and non-linearities in CO2 emissions: evidence from the BRICS and G7 countries. Environ Resour Econ. 2017;67:869–883. doi:10.1007/s10640-016--3.
- Gil-Alana LA, Trani T. Time trends and persistence in the global CO2 emissions across Europe. Environ Resour Econ. 2019;73(1):213–228.
- Tiwari AK, Kyophilavong P, Albulescu CT. Testing the stationarity of CO2 emissions series in Sub-Saharan African countries by incorporating nonlinearity and smooth breaks. Res Int Bus Financ. 2016;37:527–540. doi:10.1016/j.ribaf.2016.01.005.
- Ozcan B, Ulucak R, Dogan E. Analyzing long lasting effects of environmental policies: evidence from low, middle and high income economies. Sustain Cities Soc. 2019;44:130–143. doi:10.1016/j.scs.2018.09.025.
- Solarin SA, Bello MO. Persistence of policy shocks to an environmental degradation index: the case of ecological footprint in 128 developed and developing countries. Ecol Indic. 2018;89:35–44. doi:10.1016/j.ecolind.2018.01.064.
- Ulucak R, Lin D. Persistence of policy shocks to ecological footprint of the USA. Ecol Indic. 2017;80:337–343. doi:10.1016/j.ecolind.2017.05.020.
- Yilanci V, Gorus MS, Aydin M. Are shocks to ecological footprint in OECD countries permanent or temporary? J Cleaner Prod. 2019;212:270–301. doi:10.1016/j.jclepro.2018.11.299.
- Aldy JE. Per capita carbon dioxide emissions: convergence or divergence? Environ Resour Econ. 2006;33:533–555. doi:10.1007/s10640-005--x.
- Lee CC, Chang CP. Stochastic convergence of per capita carbon dioxide emisssions and multiple structural breaks in OECD conuntries. Econ Modell. 2009;26:1375–1381. doi:10.1016/j.econmod.2009.07.003.
- Carrion-I-Silvestre JL, Barrio-Castro TD, Lopez-Bazo E. Breaking the panels: an application to the GDP per capita. Econometrics J. 2005;8:1575–1591.
- Ezcurra R. Is there cross-country convergence in carbon dioxide emissionas? Energy Policy. 2007;35:1363–1372. doi:10.1016/j.enpol.2006.04.006.
- Panopoulou E, Pantelidis T. Club convergence in carbon dioxide emissions. Environ Resour Econ. 2009;44:47–70. doi:10.1007/s10640-008--6.
- Chang CP, Lee CC. Are per capita carbon dioxide emissions converging among industrialized conuntries? New time series evidence with structural breaks. Environ Resour Econ. 2008;13:497–515.
- Romero-Ávila D. Convergence in cabon dioxide emissions among industrialised countries revisited. Energy Econ. 2008;30:2265–2282. doi:10.1016/j.eneco.2007.06.003.
- Lee CC, Chang CP, Chen PF. Do CO2 emissions levels converge among 21 OECD countries? New evidence from unit root structural break tests. Appl Econ Lett. 2008;15:551–556. doi:10.1080/13504850500426236.
- Yavuz NC, Yilanci V. Convergence in per capita carbon dioxide emissions among countries: a TAR panel unit root approach. Environ Resour Econ. 2013;54:283–291. doi:10.1007/s10640-012--x.
- Ahmed M, Khan AM, Bibi S, et al. Convergence of per capita CO2 emissions across the globe: insights via wavelet analysis. Renewable Sustainable Energy Rev. 2016;75:86–97. http://doi.org/10.1016/j.rser.2016.10.053.
- Kapetanios G, Shin Y, Snell A. Testing for a unit root in the nonlinear STAR framework. J Econometrics. 2003;112:359–379. doi:10.1016/S-4076(02)00202-6.
- Zhang Z, Zou X, Song Q, Wang T, Fu G. Analysis of the global carbon dioxide emissions from 2003 to 2015: convergence trends and regional contributions. Carbon Manage. 2018;9:45–55. doi:10.1080/17583004.2017.1418594.
- Criado CO, Grether JM. Convergence in per capita CO2 emissions: a robust distributional approach. Resour Energy Econ. 2011;33:637–665. doi:10.1016/j.reseneeco.2011.01.003.
- Herrerias MJ. The environmental convergence hypothesis: carbon dioxide emissionas according to the source of energy. Energy Policy. 2013;61:1140–1150. doi:10.1016/j.enpol.2013.06.120.
- Li X, Lin B. Global convergence in per capita CO2 emissions. Renew Sustain Energy Rev. 2013;24:357–363. doi:10.1016/j.rser.2013.03.048.
- Presno MJ, Landajo M, Fernández González P. Stochastic convergence in per capita CO2 emissions. An approach from nonlinear stationarity analysis. Energy Econ. 2018;70:563–581. doi:10.1016/j.eneco.2015.10.001.
- Jaunky V. The CO2 emissions-income nexus:evidence from rich countries. Energy Policy. 2011;39:1228–1240. doi:10.1016/j.enpol.2010.11.050.
- Yamazaki S, Tian J, Doko Tchatoka F. Are per capita CO2 emissions increasing among OECD countries? a test of trends and breaks. Appl Econ Lett. 2014;21:569–572. doi:10.1080/13504851.2013.875103.
- Barros CP, Gil-Alana LA, De Gracia FP. Stationarity and long range dependence of carbon dioxide emisssions: evidence for disaggregated data. Environ Resour Econ. 2016;63:45–56. doi:10.1007/s10640-014--3.
- Belbute JM, Pereira AM. Do global CO2 emissions from fossil-fuel consuption exhibit long memory? A fractional-integration anaysis. Appl Econ. 2017;49(40): 4055-4070. http:/doi.org/10.1080/000368446.2016.1273508.
- Ulucak R, Apergis N. Does convergence really matter for the environment? An application based on club convergence and on the ecological footprint concept for the EU countries. Environ Sci Policy. 2018;80:21–27. doi:10.1016/j.envsci.2017.11.002.
- Jia J, Zhao J, Deng H, et al. Ecological footprint simulation and prediction by ARIMA model—a case study in Henan Province of China. Ecol Indic. 2010;10:538–544. doi:10.1016/j.ecolind.2009.06.007.
- Bahmani-Oskooee M, Chang T, Wu T. Revisiting purchasing power parity in African countries: panel stationary test with sharp and smooth breaks. Appl Financ Econ. 2014;24:1429–1438. doi:10.1080/09603107.2014.925068.
- National Footprint Accounts. (2016). Working guidebook to the national footprint accounts: 2016. Available from http://www.footprintnetwork.org/content/documents/National_Footprint_Accounts_2016_Guidebook.pdf
- Granger CWJ. Long memory relationships and the aggregation of dynamic models. J Econometrics. 1980;14:227–238. doi:10.1016/0304-(80)90092-5.
- Granger CWJ. Some properties of time series data and their use in econometric model specification. J Econometrics. 1981;16:121–130. doi:10.1016/0304-(81)90079-8.
- Granger CWJ, Joyeux R. An introduction to long memory time series models and fractional differencing. J Time Ser Anal. 1980;1:15–39. doi:10.1111/j.1467-9892.1980.tb00297.x.
- Hosking JRM. Fractional differencing. Biometrika. 1981;68:165–176. doi:10.1093/biomet/68.1.165.
- Baillie RT. Long memory processes and fractional integration in econometrics. J Econometrics. 1996;73:5–59. doi:10.1016/0304-(95)01732-1.
- Gil-Alana LA, Robinson PM. Testing of unit roots and other nonstationary hypotheses in macroeconomic time series. J Econometrics. 1997;80:241–268. doi:10.1016/S-4076(97)00038-9.
- Sowell FB. Modeling long run behavior with the fractional ARIMA model. J Monetary Econ. 1992;29:277–302. doi:10.1016/0304-(92)90016-U.
- Barassi MR, Spagnolo N, Zhao Y. Fractional integration versus structural change- testing the convergence of CO2 emissions. Environ Resour Econ. 2018;71:923–968. doi:10.1007/s10640-017--z.
- Solarin SA, Gil-Alana LA, Al-Mulali U. Stochastic convergence of renewable energy consumption in OECD countries: a fractional integration approach. Environ Sci Pollut Res. 2018;25:17289–17299.
- Dahlhaus R. Efficient parameter estimation for self-similar processes. Ann Statist. 1989;17:1749–1766. doi:10.1214/aos/1176347393.
- Robinson P. Efficient tests of nonstationary hypotheses. J Am Statist Assoc. 1994;89:1420–1437. doi:10.2307/2291004.
- Dickey DA, Fuller WA. Distributions of the estimators for autoregressive time series with a unit root. J Am Statist Assoc. 1979;74:427–481. doi:10.2307/2286348.
- Elliot G, Rothenberg TJ, Stock JH. Efficient tests for an autoregressive unit root. Econometrica. 1996;64:813–836. doi:10.2307/2171846.
- Kwiatkowski D, Phillips PCD, Schmidt P, Shin Y. Testing the null hypothesis of stationarity against the alternative of a unit root: how sure are we that economic time series have a unit root? J Econometrics. 1992;54:159–178. doi:10.1016/0304-(92)90104-Y.
- Phillips PCB, Perron P. Testing for a unit root in time series regression. Biometrika. 1988;75:335–346. doi:10.2307/2336182.
- Ng S, Perron P. Lag length selection and the construction of unit root tests with good size and power. Econometrica. 2001;69:519–1554.
- Diebold FX, Rudebusch GD. On the power of Dickey-Fuller test against fractional alternatives. Econ Lett. 1991;35(2):155–160. doi:10.1016/0165-(91)90163-F.
- Hassler U, Wolters J. On the power of unit root tests against fractional alternatives. Econ Lett. 1994;45:1–5. doi:10.1016/0165-(94)90049-3.
- Lee D, Schmidt P. On the power of the KPSS test of stationarity against fractionally integrated alternatives. J Econometrics. 1996;73:285–302. doi:10.1016/0304-(95)01741-0.
- Diebold FX, Inoue A. Long memory and regime switching. J Econometrics. 2001;105:131–159. doi:10.1016/S-4076(01)00073-2.
- Granger CWJ, Hyung N. Occasional structural breaks and long memory with an application to the S&P 500 absolute stock return. J Empirical Financ. 2004;11:399–421. doi:10.1016/j.jempfin.2003.03.001.
- Gil-Alana LA. Fractional integration and structural breaks at unknown periods of time. J Time Ser Anal. 2007;29:163–185. doi:10.1111/j.1467-9892.2007.00550.x.
- Hassler U, Meller B. Detecting multiple breaks in long memory. Deutsche Bundesbank; 2011 (The case of US inflation, Discussion Paper Series 1. Economic Studies 26) Deutsche Bundesbank.
- Hyang E, Shin DW. Tests for structural breaks in memory parameters of long memory heterogeneous autoregressive models. Commun Statist Theory Models. 2018;47(21):5378–5389.
- Shao X. A simple test of changes in mean in the possible presence of long-range dependence. J Time Ser Anal. 2011;32:598–606. doi:10.1111/j.1467-9892.2010.00717.x.
- Sibbertsen P. Long memory versus structural breaks. An overview. Statist Pap. 2004;45:465–515. doi:10.1007/BF02760564.