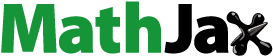
ABSTRACT
The gross calorific value (GCV), also known as the higher heating value (HHV) of coal is the amount of heat emitted upon complete combustion of coal. The GCV of coal is used to estimate various technoeconomic parameters such as boiler efficiency, combustion values, and production costs. This study seeks to examine the methods that are used to estimate the GCV of coal. These methods can be classified as either mathematical, experimental, or online methods.” It was found that linear and non-linear regression, differential scanning calorimetry (DSC), artificial intelligence (AI) methods have all been frequently used to estimate the GCV. The challenge with these approaches is that they include sophisticated machinery that demand expert operation, and the task is time consuming. Accurate and timely analysis of the GCV coal is a crucial stage in power plants, which can be achieved with online monitoring of the GCV of coal. There is not much literature on the topic of online monitoring of the coal GCV because it hasn’t been studied extensively. In power plant operation, online monitoring of the GCV of coal is a promising technology that hasn’t been studied extensively.
Introduction
Fossil fuels including fuel oil, natural gas, and coal mostly meet the world’s energy needs (Hagen and Robinson Citation2002). With regards to fossil fuels, coal is the most plentiful energy source, accounting for 40% of global energy (Mathews et al. Citation2009). Coal provides 77% of South Africa’s energy needs, 95% of this energy used by South Africa is produced by the Electricity Supply Commission (ESKOM), and coal-fired power plants produce 90% of electricity. The world’s dependence on coal as a power source is currently on call to be cut back, yet that does not take from the reality that coal is the world’s most dominant energy source.
The GCV, which is defined as the amount of heat evolved when a unit weight of coal is entirely burned, indicates the useful energy content of coal (Patel et al. Citation2007). A greater GCV indicates that less coal is needed to produce one unit of electricity (Mahapatra Citation2016). Therefore, the GCV provides an estimation of the plant’s production costs. The GCV of coal forms part of the critical parameters that can be used in calculating boiler heat balance, thermal efficiency, and boiler output (Liu and Lv Citation2020). Any industry that uses a lot of energy needs to have effective energy management (South African Bureau of Standards, SANS 17,246:2011 – Coal and Proximate Analysis Citation2011). Industries must precisely assess the energy content of their coal in order to determine and improve their energy efficiency (South African Bureau of Standards, SANS 10,320:2004 – SABS, Evaluation of coal resources and coal reserves Citation2004).
For the purposes of energy reporting and other tax incentives, such as section 12 L of the income tax law, knowing the precise energy content of coal is also crucial (Hamer, Booysen, and Mathews Citation2017). For industrial uses, it is imperative to know the precise energy content of the coal. The GCV is a parameter that plays a major role in the design and safety of power plants. As a result, numerous researchers have created models to estimate the GCV based on simpler variables like proximate and ultimate compositions (van Aarde Citation2019).
Some of these models were made for particular coal kinds from different geographical regions. It is debatable if these models can be used for coal from a new location because the characteristics of coal vary depending on the location. This study looks at the different methods used to estimate the GCV of coal classified as mathematical, experimental, and online methods.
GCV Estimation Methods
Mathematical Methods
Estimation of GCV by Regression Method
A collection of statistical techniques known as regression analysis or linear regression (LR) is used to estimate the associations between a dependent variable and one or more independent variables. It can be used to simulate the long-term link between variables and gauge how strongly the relationships between them are related (Taylor Citation2022). Researchers can use regression analysis to examine the relationship between independent and dependent variables. Typically, the dependent variable is the result (Sarstedt and Mooi Citation2014). Multiple linear regression (MLR) or multivariable regression (MR) is used to determine a mathematical relationship among several random variables. It is an extension of simple linear regression. The following section of the paper looks at a variety of methods developed by researchers to estimate the GCV of coal using regression analysis.
Mason and Gandhi (Citation1983) developed a formula for determining coal’s calorific value from its ultimate analysis by data regression analysis. A total of 775 US coal samples of all ranks were used. The equation provided zero average difference between observed and calculated values. The expression is as follows:
Q is the calculated GCV with units kJ/kg, (C), (H), (S), and (A) are the respective contents of Carbon, Hydrogen, Sulfur, and Ash in weight percent, also on a dry basis.
Given, Weldon, and Zoeller (Citation1986) developed a formula to calculate coal’s calorific value. The equation contains a term specifically relevant to the enthalpy of coal’s decomposition but ignores terms for empirical correction. The enthalpy of decomposition varies with the rank of coal and with the geological history of the coal. The equation is as follows:
Q is the GCV of coal in kJ/kg, (C), (H), (S), and (O) are the respective contents of Carbon, Hydrogen, Sulfur, and Oxygen in weight percent in the as-received coal sample.
Majumder et al. (Citation2008) developed a proximate-based analyzes correlation to determine the GCV of coal. This study aimed to focus on simplifying the already existing complex methods. A total of 250 coals were analyzed by involving major variables that influence the GCV of coal. The correlation is as follows:
GCV of coal in (MJ/kg), A is the % of ash, M is the % of moisture, is the % of volatile matter and
is the % of fixed carbon all the independent variables are in weight percentage on as-received basis.
Huda (Citation2014) developed new equations for estimating the GCV of Indonesian coals. It has been found from this study that some of the developed equations focus only on coals from certain regions. The new equations included equations with one, two, three, and four independent variables. The independent variables can be found using proximate or ultimate analysis of coal. The best equation was found to be the one with four independent variables:
M is the % of moisture, VM is the % volatile matter and FC is the % fixed carbon content in the coal.
Xu et al. (Citation2014) presented a comparative study on predicting the GCV of coal. The comparison was between regression modeling methods both linear and nonlinear for applications on online multi-spectral flame radiation features. The study found that nonlinear regression methods provided more accurate results compared to linear regression methods. the errors were found to be low, with a maximum error of 1.53%.
Seervi (Citation2015) developed a correlation on 59 coal samples from various fields in India to estimate the GCV of coal, the proximate analysis was carried out on these samples to be used as input parameters. Analysis was conducted with an emphasis on the correlation’s relationships with moisture, volatile matter, ash, and fixed carbon. The impact of moisture and ash on coal’s GCV was then discovered to be significant. The formula developed by MLR is as follows:
M is the % of moisture, A is the % ash and FC is the % fixed carbon content in the coal in are dried basis.
Gomez et al. (Citation2018) used the Fourier Transform Infrared Photoacoustic Spectroscopy (FT-IR PAS) coupled with multivariate calibration using the partial least squares (PLS) method to predict ash content, volatile matter, fixed carbon, and calorific value of coal from several mines in Colombia. The PLS is an MLR technique to correlate calibration samples. The models presented a linear character and a correlation between the reference values and the predicted values. Results provided a standard error of less than 2% for ash content, volatile matter, and fixed carbon prediction while providing an error of 0.75 for the CV of coal.
Balaeva, Miroshnichenko, and Kaftan (Citation2018) developed a method that can be used to predict the GCV of 63 test samples of coal from Ukraine, the Russian Federation, and countries outside the former Soviet Union on a moist ash-free basis. A quantitative relationship was developed to forecast the GCV of coal based on volatile matter, middle random vitrinite reflectance coefficient, and total fusainized components separately for each region. It was determined that it is reasonable to anticipate the gross calorific value of the coals independently for each region. Chelgani (Citation2019) explored relationships between the GCV of coal and valuable elements within the coal. MR was used to estimate the GCV of coal, and it was found that the ultimate analysis of coal is the best predictor.
Begum, Chakravarty, and Das (Citation2019) used proximate data and coal’s spectral reflectance data to estimate the GCV of coal. The models were created using the MLR approach. Ash, moisture, and volatile matter content are the proximate analysis of coal results. The greatest absorption band depth at five different wavelength ranges is used in the spectral reflectance data. After comparing the models, it was discovered that the spectral reflectance data had higher accuracy than the proximate data.
Go et al. (Citation2019) derived a semi-empirical model based on first principles to determine the HHV of coal. The model accounted for the technical limitations involved in coal combustion. A total of 8500 coal samples were used to generate, test, and validate the new and already existing models. The model used the proximate analysis of coal and the mass and energy balances to assist in accurately deriving the HHV models. The predicted HHV values using the models had a mean absolute error of well below 5%. The study recommended that the usefulness of a model lies in the extent of providing a theoretically sound representation of the actual phenomenon involved.
Alekhnovich (Citation2020) estimated the heating value of coal using the coal composition using well-known calculation formulas. The study found that the great difference between the measured and experimental HV values is caused by the dependence of the result on the coal characteristics unaccounted for in the calculation models. It was also found that the HV values are highly dependent on the ash content of the coal sample.
Estimation of GCV by Artificial Intelligence (AI)
AI refers to the intelligence of computers or software. It is also the area of computer science where intelligent devices are created and studied. The term “AI” can also refer to the actual devices.
Artificial Neural Network (ANN)
ANN or simulated neural networks (SNN) are a subset of machine learning. Their structure and name are modeled after the human brain, mirroring the communication between organic neurons (IBM, International Business Machines Citation2018). Artificial neurons, also known as units, are found in artificial neural networks. The entire ANN in a system is made up of these units, which are stacked in several layers. It depends on how the complicated neural networks will be used to learn the hidden patterns in the dataset whether a layer has a dozen units or millions of units. ANN’s frequently have hidden layers in addition to input and output layers. The input layer gets information that the neural network needs to interpret or learn from the outside environment. Then, after passing through one or more hidden layers, this data is transformed into useful information for the output layer. Finally, the output layer delivers an output in the form of an artificial neural network’s response to input data (Kaur Citation2023).
With most of the correlations used to determine the GCV being linear (Patel et al. Citation2007), developed seven nonlinear models to estimate the GCV of coal employing the ANN methodology. The major components of the proximate and ultimate analysis were all inputs into the main ANN model, but the other six models combined the components of the studies in different ways. The input, hidden, and output layers make up the hierarchical framework of the ANN approach.
Mesroghli, Jorjani, and Chehreh Chelgani (Citation2009) determined the GCV of coal with the help of ANN and regression. It was determined how the GCV related to the coal’s proximate and ultimate analysis. Three different input parameter groups were used to determine the most suitable estimator. The estimator that provided the best results had the following percentage inputs, Carbon, Hydrogen, Nitrogen, Oxygen, Sulfur, Moisture, and Ash. Hydrogen and Oxygen are exclusive of moisture. The expression is as follows:
Ozbayoglu, Ozbayoglu, and Ozbayoglu (Citation2012) estimated the GCV of coal using ANN, linear and nonlinear regression techniques. A total of 187 different coal samples were used, and the samples’ final and preliminary analysis served as the input parameters. The best outcomes were from estimating the GCV using the proximate and ultimate analysis results, as compared to using either proximate or ultimate analysis individually. The results from the techniques were also compared, and its was found that the models estimated the GCV value within 3% of the expected value. It was concluded that both ANN and regression techniques provided accurate results.
Kavsek et al. (Citation2013) used correlation analysis and multivariable data analysis to characterize the chemical composition of Slovenian coals, both in terms of proximate and ultimate analysis. The relationships between the chemical descriptors and coal’s HHV were established, and coal’s HHV was predicted using the proximate analysis descriptors. The discrepancy between the computed and actual HHV for both the MLR and the ANN approaches was 0.91% for MLR and 1.11% for ANN. The models were further validated using an external dataset; in MLR and ANN, the discrepancy between the computed and actual HHV was 1.39% and 1.47%, respectively.
Liu and Lv (Citation2020) examined the radial basis function (RBF) neural network and ANN structure and method characteristics to predict the GCV of coal. When predictive modeling was done, it was discovered that the RBF model performed better when small particle sizes were involved. The quality of the sample, including its size and thoroughness, was found to have a significant impact on the model’s capacity for prediction.
Co-Active Neuro-Fuzzy Inference System (CANFIS)
An CANFIS or adaptive network-based fuzzy inference system (ANFIS) is ANN that is based on the Takagi-Sugeno fuzzy inference system. The early 1990s saw the development of the method. It can combine the advantages of neural networks and fuzzy logic in a single framework because it blends both of these concepts (Jang Citation1991). CANFIS speedily solves poorly defined problems by integrating fuzzy inputs (Hemachandra and Satyanarayana Citation2013).
Erik and Yilmaz (Citation2011) used ANN and ANFIS to predict the GCV of coal. A comparison was made with regression-type methods. ANFIS models provided high performance for predicting the GCV of coal.
Behnamfard and Alaei (Citation2016) developed a mathematical and an ANFIS model to estimate two factors of proximate analysis based on the other factors. The four main factors of the proximate analysis of coal include moisture, ash, volatile matter, and fixed carbon. The GCV of coal was then estimated with the proximate analysis of coal as input parameters, using multivariable regression, the Minitab 16 software, ANFIS and MATLAB. The results indicated that the ANFIS is a more accurate tool for estimating the GCV of coal compared to the other software used. The equation determined using ANFIS is as follows:
GCV is in (kJ/kg), M is the % of moisture, A is the % ash and FC is the % fixed carbon content in the coal in are dried basis.
Verma, Singh, and Monjezi (Citation2010) used multivariate regression technique to estimate coal’s GCV. The coal’s proximate and ultimate analysis qualities were among the key input variables. In addition to the multivariate regression analysis, CANFIS supported by the genetic algorithm technique was also applied. The findings of the study offered useful and important information for predicting GCV of any type of coal in the USA.
Support Vector Machines (SVM)
SVM or support vector regression (SVR), also known as support vector networks, in machine learning, are supervised learning models with corresponding learning algorithms that evaluate data for regression and classification. SVM’s, which are based on statistical learning frameworks are among the most reliable prediction techniques (Cortes and Vapnik Citation1995).
Feng et al. (Citation2015) predicted the GCV of coal using three regression models: the SVM, the alternating conditional expectation (ACE), and the back propagation neural network (BPNN) based on proximate analysis information. The modeling outcomes demonstrated that the three techniques can typically track the variation trend of GCV with the proximate analysis parameters, SVM performs the best among the models tested in terms of generalization capabilities, and BPNN has the potential to perform better than SVM in the training stage and ACE in both the training and testing stages; however, the model’s hidden neuron number, learning rate, and initial weights have a significant impact on the prediction accuracy of BPNN. On average, ACE outperforms BPNN when it comes to generalization ability.
Tan et al. (Citation2015) used SVM to estimate coal’s HHV using coal’s proximate analysis. A total of 167 Chinese and 4549 American coal samples were used in the development and validation of the SVR-based correlations. The correlations’ average absolute errors were only 2.16% and 2.42%, respectively. Results showed that the SVR-based correlations can be more accurate when the correlation was additionally compared to published correlations. The study also considered creating a general correlation for coals from various regions, and it concluded that by using various correlations according to the region, one may estimate the HHV via proximate analysis with much greater accuracy.
Jin-Hui (Citation2016) investigated the application of statistical models for the quick and accurate online measurement of the GCV of coal. The SVM was used to predict the GCV of coal using 1400 data points and the estimating robustness is evaluated. The method was compared to existing methods and was found to have outperformed the model’s LM, nonlinear regression (NLM) and ANN.
LIBS is a form of spectroscopy that uses an extremely powerful laser pulse as the excitation source (Radziemski and Cremers Citation2006; Schetcher et al. Citation2006). Samples are atomized and excited as a result of the laser’s focus on plasma. When the focused laser reaches a specific optical breakdown threshold, which often relies on the surroundings and the target material, the plasma generation doesn’t start until then (Singh and Thakur Citation2007). LIBS is a technique used to determine the elemental composition of materials.
Lu et al. (Citation2021) estimated the CV of coal by utilizing LIBS. SVR was used to create various coal CV models. The results showed that the acoustic normalizing approach was a successful way to increase the repeatability and accuracy of measurement of coal calorific value in LIBS. It can also effectively reduce variations of shot-to-shot spectral intensities.
Project Pursuit Principle
The project pursuit principle is a statistical method presented by (Qiang and Xiaoyang Citation2006). It identifies the projections that are the most “interesting” to look at in multidimensional data. Frequently, forecasts with greater departures from a normal distribution are regarded as more intriguing. This is the “pursuit” feature that inspired the method known as matching pursuit. As each projection is identified, the data is reduced by deleting the component along that projection, and the procedure is repeated to identify new projections.
Yaun, Zhongguang, and Minfang (Citation2012) developed a model that can predict the CV of coal. The model is based on the projection pursuit principle combined with a genetic algorithm. Test results have shown that the project pursuit principle model presented high accuracy.
Random Forest (RF)
RF is a supervised Machine Learning Algorithm that is used widely in classification and regression problems. Many decision trees are built during the training phase of the random forests or random decision forests ensemble learning approach, which is used for classification, regression, and other tasks. The class that most of the trees chose is the output of the random forest for classification problems. The mean or average prediction of each individual tree is returned for regression tasks (Ho Citation1998).
Matin and Chelgani (Citation2016) used the RF method to determine the GCV of coal based on multiple investigations. An appealing contribution to the nonlinear approximation of statistical relationships between variables can be made by using RF models, a more modern technique. The model has demonstrated that the RF approach satisfactorily predicts the GCV and that the ultimate analysis parameters are the best estimators for GCV. The models for proximate and ultimate analysis “as received” are as follows:
GCV is the gross calorific value of coal in MJ/kg, M is the % of moisture, VM is the % of volatile matter, C is the % of carbon, S is the % of Sulphur, H is the % of Hydrogen and N is the % of the nitrogen in the coal as-received sample.
Buyukkanber, Haykiri-Acma, and Yaman (Citation2023) predicted the CV of coal by employing RF and ANN methodologies. The proximate and ultimate analysis of coal were used as input parameters, it was found that the methodologies that use a univariate equation of carbon can provide satisfactory predictions of the CV of coal.
Genetic Algorithm (GA)
A population of computer programs are genetically bred using the domain-independent technique known as GA to address a problem. By using analogues of naturally occurring genetic activities, genetic programming specifically iteratively creates a new generation of programs from a population of existing ones (Koza and Poli Citation2006).
Ghugare and Tambe (Citation2016) predicted the HHV of coals by employing three nonlinear correlations based on proximate and ultimate analyzes. The correlations were created using AI based on GA. The generated correlations were evaluated against the models that were already in use, and they also had to be applied to coals from various regions and rankings, as well as having a lower structural complexity than the correlations that were already based on CI. According to the study, the GA-based correlations for forecasting the HHV of coals have a great deal of potential to replace the current models.
Qi et al. (Citation2019) calculated the low calorific value (LCV) of blended coals in coal-fired power plants. Weighted sensitivity analysis and support vector regression that has been genetic algorithm-optimized (SAW-GA-SVR) were the main design considerations. Applications were completed using coal sample proximate analysis. The methodology’s capacity to model and forecast the LCV was found to be superior when compared to three other regression approaches namely, SVR, genetic algorithm optimized support vector (GA-SVR) and genetic algorithm optimized back propagation (GA-BPNN).
Wave Neural Networks (WNN)
The wavelet analysis (WA) represents signals with functions bounded in frequency and limited in time (Letelier and Weber Citation2000). WNN are a new class of models that combine neural networks (NNs) and WA (Alexandridis and Zaprants Citation2013).
Wen, Jian, and Wang (Citation2017) proposed new models that can be used to predict the GCV of coal. The models are based on WNN. The proximate and ultimate analysis of coal were used as input parameters in the model. The WNN model was also compared with already existing models, and it was revealed that the model provided outputs that were better than the existing models.
Particle Swarm Optimization (PSO)
PSO is a computer technique used in computational science that attempts to iteratively enhance a candidate solution with respect to a specified quality metric. By using a population of potential solutions, here referred to as particles, and moving them across the search space in accordance with a straightforward mathematical formula over the particle’s position and velocity, it solves problems. In addition to being led toward the best-known positions in the search space, which are updated as other particles find better positions, each particle’s movement is also impacted by its local best-known position. The swarm should move toward better answers because of this (Bonyadi and Michalewicz Citation2017).
Bui et al. (Citation2019) used a PSO – SVR model to offer a unique evolutionary-based prediction system for estimating the GCV of coal. The radial basis function, linear, and polynomial functions are among the three different kernel functions employed. The proximate components of coal were examined using 2583 samples of coal. The created classification and regression trees (CART), MLR, and principle component analysis (PCA) MR models were contrasted with the suggested PSO-SVR. The findings demonstrated that the suggested PSO-SVR model outperformed the other AI models in terms of accuracy.
S. C. Chelgani (Citation2021) calculated the GCV of coal using explainable AI and coal analysis. The AI employed is Shapley Additive exPlanations (SHAP), which ranks input variables according to their efficacy and explains the relationship between each input and its representative output. Based on the coal sample’s proximate and final “as-received” characteristics, SHAP successfully modeled the GCV.
van Aarde (Citation2019) investigated existing GCV estimation models and their application on new coals datasets. The study found errors ranging from 3.7% – 72.1%, these errors provided an insight on how far one can apply the already existing models. It was recommended that care needs to be taken in data preparation, model development, model validation and comparison of results. The study also developed a method that can be used to compare models, to assist in identifying the best model for the dataset in focus.
Experimental Methods
Estimation of GCV by Differential Scanning Calorimetry (DSC)
A technology called the DSC is used to assess temperatures and heat flows while keeping track of how materials change through time and temperature. Detailed information regarding physical and chemical changes in endothermic and exothermic processes, as well as changes in heat capacity, is provided by these measures (TA Instruments Citation2022). The DSC is equipment readily available to determine the GCV of coal by burning a coal sample in a controlled boundary. It enables the user to directly determine the heat content of coal.
Specific heats of a range of coals were measured from 300 K – 700 K using DSC. Coal samples were heated from one isothermal region to another, over a range of temperatures. The heat capacity was then determined after steady-state conditions have been attained. The specific heat increases rapidly with the content of volatile matter (Richardson Citation1993).
Kok and Keskin (Citation2001) determined the GCV of coal using complex thermal analysis techniques. Results from the method were compared with an adiabatic calorimeter. A good agreement between the methods was found, and this served as confirmation that thermal analysis is a convenient tool to determine the proximate analysis of coal samples.
A flow calorimeter-based in-situ approach for calculating the calorific value of coal was given, and a related model was created. The technology used a continuous-wave laser, a mechanical arm, a metal crucible, and a sampling cannon to sample coal powder from a coal pipe. To ensure full combustion, the coal sample was taken and ignited with the addition of oxygen at a controlled flow rate. At atmospheric pressure, the coal burnt, and the heat that was generated evaporated into the atmosphere.
The following measurements were made, the gas temperatures at the inlet and outlet, the gas flow rate, and the wall temperature. These measurements were used to calculate the total heat from combustion. The methodology was validated by comparing results with those of a bomb calorimeter with a relative error of not more than 5.37% at the worst case. The mass and energy balance (MEB) is used to determine the GCV of coal as follows.
is the input laser energy,
is the GCV of coal,
is the internal energy of the gas in the chamber,
is the internal energy of the coal particles,
is the heat absorbed by the calorimetric system,
is the heat carried by the gas flow and
is the heat transferred from the calorimeter walls to the surroundings (Wang et al. Citation2021).
Similar to differential scanning calorimetry, differential thermal analysis (DTA) is a thermos-analytical method. DTA measures any temperature differences between the sample and reference while subjecting the material under study and an inert reference to similar thermal cycles (i.e., the same chilling or heating program). The DTA curve or thermogram is then used to plot this differential temperature against time or against temperature. Relative to the inert reference, changes in the sample, whether exothermic or endothermic, can be found. A DTA curve thus offers information on the changes that have taken place, including glass transitions, crystallization, melting, and sublimation. The heat capacity of the sample has no impact on the area under a DTA peak, which represents the enthalpy change (Bhadeshia Retrieved Citation2023-09-22).
Estimation of GCV by Thermogravimetry Differential Thermal Analysis (TG/DTA)
TG/DTA monitors the change in sample weight under a controlled gas environment and temperature, as a function of temperature (and/or time). The analysis of physical or chemical processes responsible for the sample’s weight growth or loss can be done by graphing the percentage change in weight over a predetermined temperature range. Thermogravimetry (TG) has been proved to be an easy, rapid and convenient tool for screening proximate analysis of coal sample (Scientific Citation2023).
Munoz-Guillena, Linares-Solano, and de Lecea (Citation1992) examined eight samples of coal from various origins and grades using the thermogravimetry differential thermal analysis (TG/DTA) technique. In situations where an adiabatic calorimeter was not accessible, the approach was employed to calculate the calorific values of coals. This method proved to be very useful for figuring out coal’s GCV since it was validated by comparing results found by the American Society for Testing and Materials (ASTM) method and the semi-empirical method. Proximate and ultimate analysis were used as input parameters. The expression is as follows.
(C), (H), (S), (O), and (N) are the respective contents of Carbon, Hydrogen, Sulfur, Oxygen, and Nitrogen in weight percent on an as-received basis.
Online Estimation of GCV
Cheng et al. (Citation2015) found a method for calculating the GCV of coal from combustion radiation for coal-fired boilers that may be used online. The technique involved observing multiband ultraviolet, infrared, and visible combustion radiation signals. To link the important principal components that were derived with the coal’s GCV, a SVR was trained. The dynamic correlations between the multi-scale feature variables were further revealed using the Dynamic Principal Component Analysis (DPCA). The GCV values predicted by the DPCA and SVR models exhibited mean errors of 98 kcal/kg and 129.4 kcal/kg when the technique was validated using lab results.
Lu et al. (Citation2017) proposed a method to determine the online measurement of the GCV of coal. The method combined LIBS technique with artificial ANN and GA. A mean standard deviation of 0.38 MJ/kg was found for the GCV in 50 trials. These results provided high model repeatability in the GCV analysis.
Conclusions
This study has listed various methods that have been developed to estimate the GCV of coal. The methods can be summarized chronologically as follows. From as early as the 1980’s, the LR and DSC methods were used to estimate the GCV of coal. These methods showed efficiency and provided confidence for more research to be done on similar approaches. Most of the methods introduced from then after, until the early 90s looked at how to simplify the LR method by using fewer input parameters. The input parameters were mainly the ultimate and proximate analysis results of coal.
In the early 90s, the use of AI to predict the GCV were introduced and provided a more exciting path in the research area. The use of AI and LR methods to estimate the GCV of coal continued into the 2000s. Improvements were then made to investigate non-linear regression modeling methods. Nonlinear methods provided more accurate results compared to the LR method. This changed the regression modeling methods approach which induced an interest in the continuation of using regression modeling methods focusing on non-linear regression.
Methods that can be used to estimate the GCV of coal can be summarized into; the regression methods including AI and the DCS method. The regression and AI methods require the ultimate and proximate analysis of coal which causes delays in finding the GCV of coal. The DCS method includes sophisticated equipment that requires skilled operation.
With these challenges in mind, an online method to estimate the GCV of coal with the assistance of an SVR model was introduced in 2015. A few years after, another method combining the LIBS technique with artificial ANN and GA was proposed for online measurement of the GCV of coal. Online monitoring of the GCV of coal eliminates the wait and private consultation of coal analysis.
There isn’t much literature on the topic of online monitoring of the coal GCV because it hasn’t been studied extensively. It is proposed that additional research on the topic of online monitoring of the GCV of coal using easily available plant resources can be conducted.
Acknowledgement
The authors gratefully acknowledge the Tshwane University of Technology and the University of Johannesburg for providing the library resources for this study.
Disclosure statement
No potential conflict of interest was reported by the author(s).
References
- Alekhnovich, A. N. 2020. “Estimating the Heating Value of Coals Based on the Composition.” Power Technology and Engineering 10: 2–8. doi:10.1007/s10749-020-01147-5.
- Alexandridis, A. K., and A. D. Zaprants. 2013. “Wavelet Neural Networks: A Practical Guide.” Neural Networks 42: 1–27. doi:10.2139/ssrn.1923020.
- Balaeva, Y. S., D. V. Miroshnichenko, and Y. S. Kaftan. 2018. “Method for Calculating the Gross Calorific Value of Coal on a Moist Ash-Free Basis.” Solid Fuel Chemistry 52 (5): 279–288. doi:10.3103/S0361521918030023.
- Begum, N., D. Chakravarty, and B. S. Das. 2019. “Estimation of Gross Calorifc Value of Bituminous Coal Using Various Coal Properties and Reflectance Spectra.” International Journal of Coal Preparation & Utilization 42: 979–985. doi:10.1080/19392699.2019.1621301.
- Behnamfard, A., and R. Alaei. 2016. “Estimation of Coal Proximate Analysis Factors and Calorifc Value by Multivariable Regression Method and Adaptive Neuro-Fuzzy Inference System (ANFIS.” Internation Journal of Mining and Geo-Engineering 51 (1): 29–35. doi:10.22059/IJMGE.2017.62150.
- Bhadeshia, H. K. R. [2023-09-22]. Thermal analysis techniques – Differential thermal analysis. Cambridge, United Kingdom: University of Cambridge, Material Science and Metallurgy.
- Bonyadi, M. R., and Z. Michalewicz. 2017. “Particle Swarm Optimization for Single Objective Continuous Space Problems: A Review.” Evolutionary Computation 25 (1): 1–54. doi:10.1162/EVCO_r_00180.
- Bui, H. B., H. Nguyen, Y. Choi, X. N. Bui, T. Nguyen-Thoi, and Y. Zandi. 2019. “A Novel Artificial Intelligence Technique to Estimate the Gross Calorifc Value of Coal Based on Meta-Heuristic and Support Vector Regression Algorithms.” Applied Sciences 9 (22): 4868. doi:10.3390/app9224868.
- Buyukkanber, K., H. Haykiri-Acma, and S. Yaman. 2023. “Calorific Value Prediction of Coal and Its Optimization by Machine Learning Based Limited Samples in a Wide Range.” Energy 277 (6): 127666. doi:10.1016/j.energy.2023.127666.
- Chelgani, S. C. 2019. “Exploring Relationships of Gross Calorific Value and Valuable Elements with Conventional Coal Properties for North Korean Coals.” International Journal of Mining Science and Technology 29 (1): 867–871. doi:10.1016/j.ijmst.2019.09.005.
- Chelgani, S. C. 2021. “Estimation of Gross Calorific Value Based on Coal Analysis Using an Explainable Artificial Intelligence.” Machine Learning with Applications 6 (1): 100116. doi:10.1016/j.mlwa.2021.100116.
- Cheng, Y., L. Xu, X. Li, and L. Chen. 2015. “Online Estimation of Coal Calorific Value from Combustion Radiation for Coal-Fired Boilers.” Combust Science Technology 187 (10): 1487–1503. doi:10.1080/00102202.2015.1019618.
- Cortes, C., and V. Vapnik. 1995. “Support Vector Networks.” Machine Learning 20 (1): 273–297. doi:10.1007/BF00994018.
- Erik, N. Y., and I. Yilmaz. 2011. “On the Use of Conventional and Soft Computing Models for Prediction of Gross Calorific Value (GCV) of Coal.” International Journal of Coal Preparation & Utilization 31 (1): 32–59. doi:10.1080/19392699.2010.534683.
- Feng, Q., J. Zhang, X. Zhang, and S. Wen. 2015. “Proximate Analysis Based Prediction of Gross Calorific Value of Coals: A Comparision of Support Vector Machine, Alternating Conditional Expectation and Artificial Neural Network.” Fuel Processing Technology 129 (8): 120–129. doi:10.1016/j.fuproc.2014.09.001.
- Ghugare, S. B., and S. S. Tambe. 2016. “Genetic Programming Based High Performing Correlations for Prediction of Higher Heating Value of Coals of Different Ranks and from Diverse Geographies.” Journal of the Energy Institute 90 (3): 476–484. doi:10.1016/j.joei.2016.03.002.
- Given, P. H., D. Weldon, and J. H. Zoeller. 1986. “Calculation of Calorific Values of Coals from Ultimate Analysis: Theoretical Basis and Geochemical Implications.” Fuel 65 (6): 849–854. doi:10.1016/0016-2361(86)90080-3.
- Go, A. W., R. C. Agapay, Y. H. Ju, and A. T. Conag. 2019. “Unified Semi-Empirical Models for Predicting or Estimating the Heating Value of Coal and Related Properties – Theoretical Basis and Thermochemical Implications.” Combustion Science and Technology 192 (8): 1449–1474. doi:10.1080/00102202.2019.1617705.
- Gomez, Y. R., R. C. Hernandez, J. E. Guerrero, and E. Mejia-Ospino. 2018. “FTIR-PAS Coupled to Partial Least Squares for Prediction of Ash Content, Volatile Matter, Fixed Carbon and Calorific Value of Coal.” Fuel 226 (3): 536–544. doi:10.1016/j.fuel.2018.04.040.
- Hagen, H., and P. Robinson. 2002. Derivation of methodology capable of identifying suitable collectors for coal flotation by using surface dependant techniques. Thesis, CapeTown: Cape Peninsula University of Technology.
- Hamer, W., W. Booysen, and E. H. Mathews. 2017. “A Practical Approach to Managing Uncertainty in the Measurement and Verification of Energy Efficiency Savings.” South African Journal of Industrial Engineering 28 (3): 128–146. doi:10.7166/28-3-1850.
- Hemachandra, S., and R. V. Satyanarayana. 2013. “Co-Active Neuro-Fuzzy Inference System for Prediction of Electric Load.” International Journal of Electrical and Electronics 3 (2): 217–222.
- Ho, T. K. 1998. “The Random Subspace Method for Constructing Decision Forests.” IEEE Transactions on Pattern Analysis and Machine Intelligence 20 (8): 832–844. doi:10.1109/34.709601.
- Huda, M. 2014. “Development of New Equations for Estimating GCV of Indonesioan Coals.” Indonesian Mining Journal 12 (1): 10–19.
- IBM (International Business Machines). Understanding Machine Learning. 2018. Machine Learning. Limited Edition, 75. USA: John Wiley & Sons, Inc.
- Jang, J.-S. R. 1991. “Fuzzy Modeling Using Generalized Neural Networks and Kalman Filter Algorithm.” 9th National Conference on Artificial Intelligence. Anaheim, CA, USA. 762–767.
- Jin-Hui, F. U. 2016. “Application of SVM in the Estimation of GCV of Coal and a Comparison Study of the Accuracy and Robustness of SVM.” 23rd International Conference on Management Science & Engineering. Olten, Switzerland.
- Kaur H.2023. Artificial Neural Networks and its Applications. Noida, India:Geegs for Geegs.
- Kavsek, D., A. Bednarova, M. Biro, R. Kranvogl, D. B. Voncina, and E. Beinrohr. 2013. “Characterization of Slovenian Coal and Estimation of Coal Heating Value Based on Proximate Analysis Using Regression and Artificial Neural Networks.” Central European Journal of Chemistry 11 (9): 1481–1491. doi:10.2478/s11532-013-0280-x.
- Kok, M. V., and C. Keskin. 2001. “Calorific Value Determination of Coals by DTA and ASTM Methods.” Journal of Thermal Analysis and Calorimetry 64 (3): 1265–1270. doi:10.1023/A:1011569701909.
- Koza, J. R., and R. Poli. 2006. “Genetic Programming.” In Search Methodologies, edited by E K Burke and G. Kendall, 127–164. Ireland: Springer Science & Business Media.
- Letelier, J. C., and P. P. Weber. 2000. “Spike Sorting Based on Discrete Wavelet Transform Coefficients.” Journal of Neuroscience Methods 101 (2): 93–106. doi:10.1016/S0165-0270(00)00250-8.
- Liu, P., and S. Lv. 2020. “Measurement and Calculation of Calorific Value of Raw Coal Based on Artificial Neural Network Analysis Method.” Thermal Science 24 (00): 3129–3137. doi:10.2298/TSCI191106087L.
- Lu, Z., J. Mo, S. Yao, J. Zhao, and J. Lu. 2017. “Rapid Determination of the Gross Calorific Value of Coal Using Laser Induced Breakdown Spectroscopy Coupled with Artificial Networks and Genetic Algorithm.” Energy & Fuels 31 (4). doi:10.1021/acs.energyfuels.7b00025.
- Lu, P., Z. Zhuo, W. Zhang, J. Tang, T. Xing, T. Sun, and J. Lu. 2021. “Determination of Calorific Value in Coal LIBS Coupled with Acoustic Normalization.” Applied Physics B 127 (6): 82. doi:10.1007/s00340-021-07626-5.
- Mahapatra, D. 2016. A review on steam coal analysis - Calorific value. India: Trimex Industries Pvt. Ltd.
- Majumder, A. K., R. Jain, P. Banerjee, and J. P. Barnwal. 2008. “Development of a New Proximate Analysis Based Correlation to Predict Calorifc Value of Coal.” Fuel 87 (13–14): 3077–3081. doi:10.1016/j.fuel.2008.04.008.
- Mason, D. M., and K. N. Gandhi. 1983. “Formulas for Calculating the Calorific Value of Coal and Coal Chars: Development, Tests and Uses.” Fuel Processing Technology 7 (1): 11–22. doi:10.1016/0378-3820(83)90022-X.
- Mathews, E., R. W. S. Burt, C. S. Singh, R. van Liere, and World Coal Institute. 2009. Putney, London: World Coal Institute.
- Matin, S. S., and S. C. Chelgani. 2016. “Estimation of Coal Gross Calorific Value Based on Various Analyses by Random Forest Method.” Fuel 177 (2): 274–278. doi:10.1016/j.fuel.2016.03.031.
- Mesroghli, S., E. Jorjani, and S. Chehreh Chelgani. 2009. “Estimation of Gross Calorific Value Based on Coal Analysis Using Regression and Artificial Neural Networks.” International Journal of Coal Geology 79 (1): 49–54. doi:10.1016/j.coal.2009.04.002.
- Munoz-Guillena, M. J., A. Linares-Solano, and C. S. de Lecea. 1992. “Determination of Calorific Values of Coals by Differential Thermal Analysis.” Fuel 71 (5): 579–583. doi:10.1016/0016-2361(92)90157-J.
- Ozbayoglu, A. M., M. W. Ozbayoglu, and G. Ozbayoglu. 2012. Comparison of Gross Calorific Value Estimation of Turkish Coal Using Regression and Neural Networks Techniques. XXVI Internationla Mineral Processing Congress, 24-28 September 2012, New Delhi, India.
- Patel, S. U., B. J. Kumar, Y. P. Badhe, B. K. Sharma, S. Saha, S. Biswas, A. Chaudhury et al. 2007. “Estimation of Gross Calorific Value of Coals Using Artificial Neural Networks.” Fuel 86 (3): 334–344. doi:10.1016/j.fuel.2006.07.036.
- Qiang, F., and Z. Xiaoyang. 2006. “The Principle and Application of Projection Pursuit Model.” Beijing, China: Science Press.
- Qi, M., H. Luo, P. Wei, and Z. Fu. 2019. “Estimation of Low Calorific Value of Blended Coals Based on Support Vector Regression and Sensitivity in Coal-Fired Power Plants.” Fuel 236 (1): 1400–1407. doi:10.1016/j.fuel.2018.09.117.
- Radziemski, L. J., and D. A. Cremers. 2006. Handbook of laser-induced breakdown spectroscopy. New York: John Wiley.
- Richardson, M. J. 1993. “The Specific Heats of Coals, Cokes and Their Ashes.” Fuel 72 (7): 1047–1053. doi:10.1016/0016-2361(93)90307-N.
- SABS (South African Bureau of Standards). 2004. SANS 10320:2004 – Evaluation of coal resources and coal reserves. South Africa: SAMCODES - South African Mineral Codes.
- SABS (South African Bureau of Standards). 2011. SANS 17246:2011 – Coal and Proximate Analysis. South Africa: SAMCODES - South African Mineral Codes.
- Sarstedt, M., and E. Mooi. 2014. A concise guide to market research. USA: Springer. doi: 10.1007/978-3-642-12541-6_9.
- Schetcher, I., A. W. Miziolek, W. Andrzej, and P. Vincenzo. 2006. Laser-induced breakdown spectroscopy (LIBS): Fundamentals and applications. Cambridge, UK: Cambridge University Press.
- Scientific, E. Eurofins Scientific. 2023. Thermogravimetry Differential Thermal Analysis (TG/DTA). Eurofins EAG Laboratories. https://www.eag.com/techniques/phys-chem/thermogravimetry-differential-thermal-analysis-tg-dta/.
- Seervi, K. 2015. Prediction of calorific value of Indian coals by artificial neural network. Rourkela, India: National Insitute of Technology.
- Singh, J. P., and S. N. Thakur. 2007. Laser-Induced Breakdown Spectroscopy. 1st Edition, 454. Amsterdam, Netherlands: Elsevier Science.
- TA Instruments. 2022. DSc 2920 differential scanning calorimeter. USA: TA Instruments.
- Tan, P., Q. F. Ji Xia, G. Chen, and C. Zhang. 2015. “Estimation of Higher Heating Value of Coal Based on Proximate Analysis Using Support Vector Regression.” Fuel Processing Technology 138 (1): 298–304. doi:10.1016/j.fuproc.2015.06.013.
- Taylor, S. 2022. Regression Analysis - The estimation of relationships between adependent variable and one or more independent variables. Vancouver, Canada: Corporate Finance Institute. https://corporatefinanceinstitute.com/resources/data-science/regression-analysis/
- van Aarde, C. 2019. A general approach to develop and assess models estimating coal energy content. South Africa: North-West University.
- Verma, A. K., T. N. Singh, and M. Monjezi. 2010. “Intelligent Prediction of Heating Value of Coal.” Iranian Journal of Earth Sciences 2 (1): 32–38. doi:sanad.iau.ir/fa/Journal/ijes/Article/917154.
- Wang, L., X. Wu, X. Gao, Y. Wu, and K. Cen. 2021. “A Method for in-Situ Measurement of Calorific Value of Coal: A Numerical Study.” Thermochimica acta 703 (1): 179011. doi:10.1016/j.tca.2021.179011.
- Wen, X., S. Jian, and J. Wang. 2017. “Prediction Models of Calorific Value of Coal Based on Wavelet Neural Network.” Fuel 199 (1): 512–522. doi:10.1016/j.fuel.2017.03.012.
- Xu, L., Y. Cheng, R. Yin, and Q. Zhang. 2014. “Comparative Study of Regression Modeling Methods for Online Coal Calorific Value Prediction from Flame Radiation Features.” Fuel 142 (1): 164–172. doi:10.1016/j.fuel.2014.10.081.
- Yaun, J., F. U. Zhongguang, and Q. I. Minfang. 2012. “Coal Calorific Value Prediction Based on Projection Pursuit Principle.” TELKOMNIKA Indonesian Journal of Electrical Engineering 10 (6): 1287–1292. doi:10.11591/telkomnika.v10i6.1414.