ABSTRACT
Monoclonal antibodies are an important therapeutic entity, and knowledge of antibody pharmacokinetics has steadily increased over the years. Despite this effort, little is known about the extent of IgG antibody degradation in different tissues of the body. While studies have been published identifying sites of degradation with the use of residualizing and non-residualizing radiolabels, quantitative tissue clearances have not yet been derived. Here, we show that in physiologically-based pharmacokinetic (PBPK) models we can combine mouse data of Indium-111 and Iodine-125 labeled antibodies with prior physiologic knowledge to determine tissue-specific intrinsic clearances. Unspecific total tissue clearance (mL/day) in the mouse was estimated to be: liver = 4.75; brain = 0.02; gut = 0.40; heart = 0.07; kidney = 0.97; lung = 0.20; muscle = 3.02; skin = 3.89; spleen = 0.45; rest of body = 2.16. The highest catabolic activity (per g tissue) was in spleen for an FcRn wild-type antibody, but shifts to the liver for an antibody with reduced FcRn affinity. In the model developed, this shift can be explained by the liver having a greater FcRn-mediated protection capacity than the spleen. The quantification of tissue intrinsic clearances and FcRn salvage capacity increases our understanding of quantitative processes that drive the therapeutic responses of antibodies. This knowledge is critical, for instance to estimate the non-specific cellular uptake and degradation of antibodies used for targeted delivery of payloads.
Introduction
The physiologic functions and therapeutic uses of antibodies are well established. There has been rapid progress and increasing knowledge in these fields, and yet there is still very little quantitative information about antibody distribution and clearance patterns in the body. Most descriptions of antibody disposition separate clearance into “endogenous” or “non-specific” clearance and “target-mediated” clearance.Citation1,2 Non-specific degradation of antibodies occurs by pinocytosis following cellular endosomal uptake and subsequent lysosomal proteolytic degradation into amino acids or smaller peptides.Citation3 Cellular uptake of antibodies is thought to occur mostly in endothelial and haematopoietic cells.Citation4-9 Once antibodies are taken up into the endosome, they can be protected from degradation by binding to the neonatal Fc receptor (FcRn). This receptor binds antibodies in a pH-dependent manner with higher affinity at pH 6 in the endosome than at the physiologic pH of ∼7.4 in plasma.Citation10 Therefore, antibodies that are bound to FcRn in the endosome are released at neutral pH into the plasma, allowing recirculation of the antibody rather than lysosomal degradation.Citation11,12 Due to this salvage mechanism, the FcRn receptor is a main driver for the long half-life of therapeutic antibodies.Citation13-16 In FcRn-knockout (FcRn KO) mice, antibody clearance is ∼8 times higher than in wild- type mice.Citation13,17
Much effort has been invested in understanding the cellular mechanisms of uptake, recycling and catabolic degradation of antibodies but there is still little quantitative information available on the relative contribution of the individual tissues.Citation7 Only a few reports present experimental data on tissue- specific antibody degradation.Citation18-21 However, this information is important to optimize antibodies for their therapeutic objective. For example, when attaching a payload such as a toxophore to an antibody (e.g., for an anti-drug conjugate [ADC]), unspecific cellular uptake and degradation may expose non-target expressing tissues to the toxophore, inducing adverse effects. Furthermore, for many other therapeutic approaches (such as signaling inhibition and immunotherapy), it is crucial to understand how long and where antibodies remain intact in the interstitial space, where they have the potential to exert their therapeutic effect.
Yip and colleagues recently conducted a biodistribution study with measurements in plasma and several tissues using antibody constructs with different affinities to FcRn, specifically: physiologic (FcRn WT), decreased (FcRn−)and enhanced (FcRn+).Citation20 Two radiolabels that differ in their cellular residence time were used: iodine-125 (I-125), which is rapidly released from the cell and eliminated from the system, and indium-111-DOTA (In-111), which is known to reside in the cell.Citation22 Combining such data provides more information than traditional biodistribution studies using a single radiolabel. Information on the effect of FcRn on the pharmacokinetics (PK) of antibodies and the location of their degradation is contained in the data. Once a labeled antibody is degraded in the endosomal space, I-125 is quickly released and redistributed, while In-111 is trapped within the cells, leading to a radioactive signal accumulation in clearing tissues.Citation23,24
This kind of data provides information on the site of degradation of antibodies. To derive quantitative estimates for clearance, these data should ideally be put into the context of physiologically based pharmacokinetic (PBPK) modeling to account for the different localizations of the measured signal (e.g., vascular, interstitial, intracellular), while using realistic physiologic information (e.g., tissue weights, blood flows). PBPK models provide a quantitative framework for assessing unknown parameter estimates, such as intrinsic clearances of tissues, to describe continuous time-concentration profiles for each tissue that are consistent with known physiology.
In this study, we developed and applied such a PBPK model to analyze biodistribution data with a paired labeling method. Our approach allows quantitatively estimation of intrinsic tissue clearances. The FcRn WT and FcRn− data allows us to assess FcRn-mediated differences in tissue intrinsic clearance and provides information about the protection capacity of FcRn in the corresponding tissues. The FcRn+ data were not included in this analysis because no apparent PK differences to the FcRn WT data were observed. Quantification of tissue-specific contributions to total systemic clearance indicates which organs have the greatest effect on PK and which have the highest catabolic specific activity, and therefore are most prone to safety issues during antibody therapy.
Results
Whole-body PBPK model
We developed a whole-body PBPK model that accounted for radiolabels with different intracellular residence time to derive intrinsic tissue clearances and estimate FcRn salvage capacity in 9 tissues (). The known physiologic parameters used to parameterize the model are provided in Supplementary Table S1/S2. The PBPK model was simultaneously fitted to all biodistribution data. Unknown parameters were estimated (). The measured plasma PK profiles of FcRn WT and FcRn− constructs were well described by the PBPK model () in which a pronounced increase in clearance for the FcRn− construct was observed. Estimates of systemic clearance by non-compartmental analysis (NCA) were 0.011 mL/h and 0.095 mL/h for FcRn WT and FcRn−, respectively, indicating an 8.7-fold increase in clearance for the FcRn− variant. Tissue PK data, including In-111 and I-125 labeled biodistribution data for each tissue, and corresponding model predictions are presented in (FcRn WT) and (FcRn−). The model accurately captured the concentration-time profiles for both labels in all tissues. In-111 accumulation was evident and most pronounced in the liver, spleen and kidney.
Figure 1. Schematic representation of the PBPK model. The whole body representation shows the included tissues. After injection, antibodies are distributed to the tissues by the blood flows (black arrows). Extravasation into the interstitial space occurs mainly by convection. Alternatively, the antibody can be taken up by the endosome following passive uptake. From the interstitial space, the antibody is then returned to the systemic circulation by the lymphatic system (dashed arrows). In the endosome, the antibody can bind to the FcRn receptor and be recycled to the plasma or, if it does not bind, it might undergo degradation in the lysosome. Upon degradation, the radioactive label might leave the cell fairly quickly in case of a non-residualizing (I-125 / yellow star) label or be trapped in the cell for a certain time in case of a residualizing label (e.g., In-111 / red star). This difference is a good indicator of the extent of degradation.
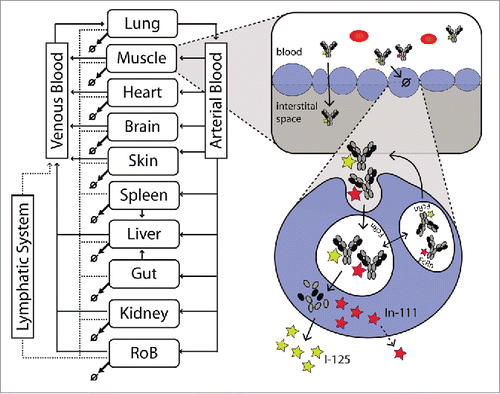
Table 1. Estimated parameters.
Figure 2. Individual plasma PK data of FcRn WT (circles) and FcRn− (triangles) anti-gD antibody are overlaid with the model predicted plasma PK.
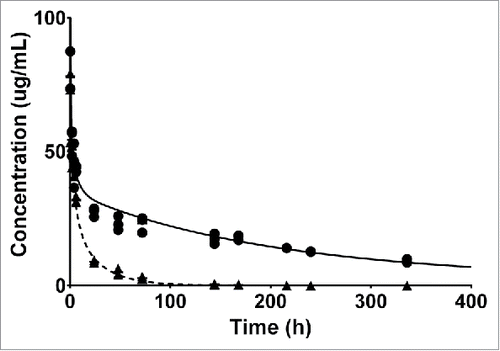
Figure 3. Represents the PBPK fit for all tissue PK data for the FcRn binding antibody. Each tissue is depicted in a subplot, including the measured data and model simulation for I-125 (white circles/gray) and In-111 (black circles/line). The gray shaded area indicates the difference between the measured PK of the I-125 and In-111 labeled antibody and is an indication of the degradation, and subsequent In-111 accumulation in the respective tissue.
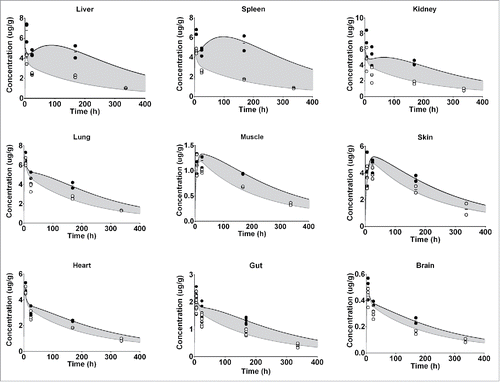
Figure 4. Represents the PBPK fit for all tissue PK data for the FcRn− antibody. Each tissue is depicted in a subplot, including the measured data and model simulation for I-125 (white circles/gray line) and In-111 (black circles/line). The gray shaded area indicates the difference between the measured PK of the I-125 and In-111 labeled antibody and is an indication of the degradation, and subsequent In-111 accumulation in the respective tissue.
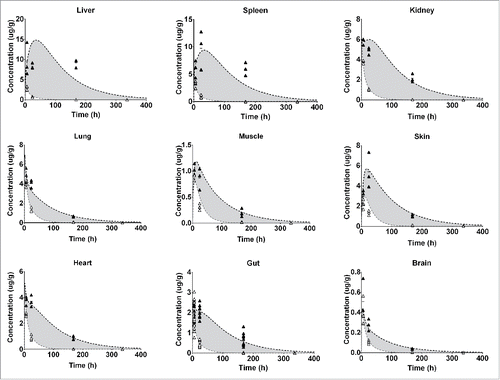
Confidence intervals for estimated model parameters were narrow. Only the convective uptake flow parameters were not very well estimated for most organs, including the brain, gut, heart, kidney, liver, lung and spleen. However, the same parameter was estimated with high confidence for skin and muscle. This may be due to insufficient sampling within the first 6 hours of the PK study. Therefore, limited information is available for the tissue uptake phase of well-perfused tissues, leading to some uncertainty in the estimation of the respective parameters. It is of note, however, that despite the broad confidence interval in this parameter the data are well captured and it does not bias the further analysis.
Based on estimated parameters, the PBPK model is capable of predicting the concentration-time profiles in different tissue subcompartments, specifically in interstitial space and residual plasma. For In-111 labeling, the retention compartment was used to derive the amount of drug degraded in each tissue. In , we outline the contributions of the different tissue sub-compartments to total tissue concentration for the gut, liver, lung and skin. These tissues are representative of the diversity of the 9 tissues included in the PBPK model. For the gut, the model predicted that interstitial space, residual plasma and retained indium label contributed significantly to the measured signal. For the liver and lung, on the other hand, a major part of the signal was due to residual plasma, while interstitial contribution was low. However, label retention was much more pronounced in the liver than in the lung, indicating higher catabolic activity in the liver. The skin is a different case in which residual plasma only contributed very little to the measured total tissue concentration. Skin is also distinctive as the predicted maximal concentration occurred later than for the other 3 tissues.
Figure 5. A PBPK-based breakup of total tissue concentration into the contributions of the different sub-compartments. Red shows the contribution of residual plasma, blue of the interstitial space and green of the In-111 label retention. We show 4 representative examples for the different tissues: (a) Gut, which has a quite even contribution of the 3, (b) Liver which is highly degrading and therefore has a high contribution of the retained In-111 label and a quite high residual blood contribution, (c) Lung has high residual plasma, similar to liver, and fairly low interstitial contribution but less label retention and (d) Skin, where the total tissue concentration is mostly based on the interstitial contribution with only very little residual plasma and some label retained after degradation.
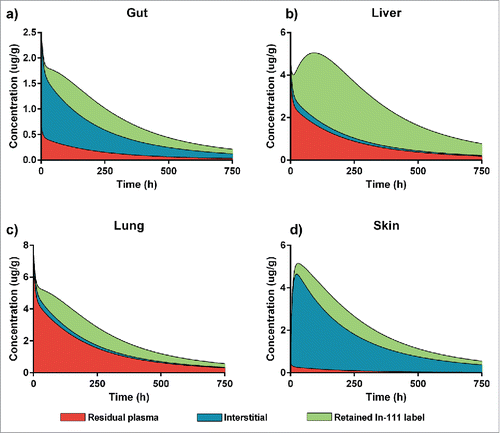
Quantitative assessment of tissue intrinsic clearances
Differences between the labeling methods allowed us to estimate the total amount of drug degraded in a given tissue. Model simulations were conducted over a long period of time (i.e., 125 days) until the entire amount of dosed antibody was completely catabolized. To assess the contribution of each tissue to the overall degradation, we multiplied the fraction of total dose degraded in each tissue by the total systemic clearance to quantitatively derive tissue-specific intrinsic clearances, presented in .
Table 2. Tissue clearances.
For FcRn WT antibody, the major clearing organ was the liver (4.75 mL/d), which accounted for ∼30% of the antibody degradation and thus was even greater in absolute terms than skin (∼25%) and muscle (19%). The rest of body (e.g., adipose, bone including bone marrow, smaller glands), which accounts for ∼26% of the body weight, was responsible for 13% of degraded antibody. The exact tissues that are responsible for these 13% of degraded antibody remain unknown. These 3 organs and the group of tissues accounted for ∼87% of the systemic clearance and thus are very important from a PK perspective. The lowest absolute clearances estimated were for the brain (0.02 mL/d) and heart (0.07 mL/d). The picture, however, changes when looking at clearances normalized by tissue weight, which indicates the specific catabolic activity of the organs. The spleen, kidney and liver (in decreasing order) displayed the highest catabolic activity for the FcRn WT antibody. This analysis also demonstrates that the high absolute clearance values in skin, and especially in the muscle and rest of body, are due to their higher tissue weights (together ∼84% of body weight), whereas their catabolic activity is less than one third of the activity in spleen.
For the FcRn− antibody, higher absolute clearance values were found in all tissues (3.5–11.2-fold). The order of the major clearing organs did not change significantly, with liver (41%) followed by skin (27%), rest of body (12%) and muscle (10%) being the main contributors to the total systemic clearance. However, the fractional contributions to the total clearance of the tissues were not the same for the FcRn− and the FcRn WT antibodies. For example, the contribution of the liver increased from 30 to 41%, whereas it decreased from 19 to 10% for muscle. With this increased clearance, the liver had the highest catabolic activity for the FcRn− variant, again followed by spleen and kidney. Absolute and weight normalized tissue clearances for both antibody variants are summarized in .
FcRn salvage capacity in tissues
We observed that the clearance increase was not the same in each tissue when comparing tissue clearances for the FcRn WT and FcRn− antibody (), indicating that the tissues do not have an equal FcRn salvage capacity. The highest clearance increase of 11.2-fold was observed in the liver, indicating that FcRn plays an important protective role in this tissue. It was followed by the heart, skin and gut. In these tissues, the FcRn protection against antibody degradation was estimated to be greater than in other tissues. For the brain, kidney, spleen, lung and muscle, less difference in tissue clearance was observed when comparing the estimated FcRn− and FcRn WT clearances.
Discussion
In this work, we quantitatively assessed the contribution of specific tissues to the systemic clearance of an untargeted monoclonal IgG antibody as FcRn WT and FcRn− variants. We modified a full PBPK model published by Ferl et al.,Citation21,25 which enabled us to distinguish between a residualizing and non-residualizing label and, for the first time, to quantitatively derive tissue-specific clearances and the salvage capacity of FcRn in 9 tissues in mice.
Our analysis demonstrates that the major tissues for antibody catabolism in mice were liver (30 and 41%), skin (∼25 and 27%), and muscle (19 and 10%), for FcRn WT and FcRn−, respectively. These tissues alone represent 74% and 78% of the total clearance. However, while they all have a high net contribution to the total clearance, this is a result of different factors. We found that, for the liver, this effect was due to a very high catabolic activity per gram tissue (see ), whereas skin and muscle have a relatively low tissue clearance per gram tissue and their large size was the reason for their high net impact on the clearance. Summing up the clearances of all investigated tissues suggested that 10% and 15% of the total clearance observed in plasma remain unexplained for FcRn WT and FcRn−, respectively. The investigated tissues accounted only for 74% of the total body weight, with tissues such as adipose tissue and bone marrow missing from the data set. Therefore, we assumed that the remaining antibody catabolism happens in a Rest of Body compartment, resulting in an average catabolic activity per gram of tissue for all tissues included in this compartment. Omitting this compartment in the model would lead to an overestimation of the clearance in other tissues because mass-balance must be accounted for in the PBPK model.
While we identified the liver, skin, and muscle as the major clearing organs given the data used for the analysis, we found that the spleen, liver, and kidney were the main organs for antibody degradation per gram of tissue. We therefore infer that these organs catabolize the most antibody molecules, making them the most susceptible to either intended or adverse effects mediated by antibodies or attached payloads.
Our determined tissue clearances are broadly consistent with published reports stating that the liver, muscle and skin are the major contributors to the systemic clearance of antibodies.Citation2,18,19 Nevertheless, compared with published results, the presented analysis has pronounced differences in the order and extent by which each tissue contributes to the total systemic clearance. For example, this is apparent when we directly compare our derived values with the tissue clearance values often used in large molecule PBPK modelsCitation26,27 that are derived by distributing the total clearance according to the fractional organ weight,Citation28 hence assuming equal catabolic activity per gram tissue for all organs. Consequently, Garg and Balthasar report that muscle (∼50%), gut (∼22%) and skin (18%) are the major clearing organs in absolute terms,Citation26 whereas our analysis reveals that the liver (∼30%), skin (∼25%) and muscle (∼19%) comprise the major clearing organs. Similar deviations were found for the other tissues (Fig. S1). In an analysis performed for an scFv-Fc compound,Citation21 tissue contributions to the total clearance of an FcRn- variant in non-tumor-bearing mice showed that the liver contributed to ∼50%, the kidney ∼8%, the spleen ∼2% and the carcass ∼40%. This is consistent with our present study in which the FcRn− construct was also mainly cleared by the liver (∼40%), with the kidney and spleen accounting for ∼4% and ∼2%, respectively.
One remaining question relates to modifications of FcRn affinity and their effect on antibody degradation in tissues. Therefore, we compared the ratio of tissue clearances of FcRn WT and FcRn- antibodies. While all individual tissue clearances increased for FcRn- antibodies, the extent of the increase differed for individual tissues (). For example, the greatest clearance increase observed was in the liver (11.4-fold), whereas the smallest was in the lung (3.5-fold). Interestingly, our analysis suggests that it might be possible to optimize FcRn affinity to increase the relative contribution of specific tissues to total clearances, and therefore, for instance, increase delivery of a payload into the liver or other tissues. Investigated clearance ratios further give an indication about the FcRn-dependent salvage mechanism in the corresponding tissue. The ratio is a function of FcRn expression, the amount of antibody presented to FcRn in the endosomal space, as well as the accessibility of FcRn for the antibodies. Hence, the ratio does not represent directly measurable FcRn expression level alone, but instead salvage capacity, i.e., a resulting net impact on antibody protection. When comparing our salvage capacity to reported FcRn tissue expression levels,Citation29,30 they agree on a high protection capacity in the liver and skin. However, we find a much lower salvage capacity for other tissues with reportedly high FcRn expression, such as the lung and spleen.
The PBPK model in this analysis was used as a tool to quantify clearances and FcRn salvaging in the different tissues for the 2 distinct FcRn variants of IgG. There are numerous other published model structures and still ongoing discussions and discrepancies around the structure, parameterization and integrated processes in published PBPK models. The model structure was chosen so that it allows us to describe the biodistribution data with the 2 labels and 2 FcRn variants simultaneously and quantify their differences in a physiologic framework. The applied model omits other complex processes, e.g., endosomal trafficking.Citation30 However, as long as a good description of the biodistribution data and the differences between the labels and FcRn variants is warranted, other model structures could theoretically be used for this analysis and should come to the same conclusions.
The FcRn affinity-dependent shift in relative contribution of tissue clearances to the systemic clearance has been well described for the 2 analyzed variants. While this approximately delimits the range in which this shift takes place for the different tissues, the model in its current state might not allow extrapolation for other FcRn variants (e.g., FcRn enhanced). This would require the analysis of more FcRn variants and integration of endogenous IgG levels for a more mechanistic description of this shift and FcRn saturation.
We suggest that the results presented here can be used to estimate the nonspecific intrinsic tissues clearances by first measuring the total plasma clearance (usually in a range of 3–16 mL/d/kg31), and second applying the fractional contributions of the different tissues derived here. Using this methodology, these values could also be used to estimate tissue clearances in other preclinical species and in humans. However, further validation is required to show that these fractional clearances are similar in different species, as is suggested to be the case for antibody distribution coefficients.Citation32
We envision the tissue clearances derived here can also benefit the development of targeted therapies for payload delivery, such as ADCs. It is well understood that target binding of antibodies and any subsequent internalization competes with unspecific cellular uptake processes. By providing a quantitative measure for the unspecific uptake of antibodies in different tissues, the exposures of tissues without target expression can be estimated and potentially compared with target-mediated uptake estimates derived from, for instance, target-mediated drug disposition profiles. Hence, the tissue clearance values derived here give a quantitative estimate of how efficient target-mediated processes need to be to direct the antibody away from tissues without target expression.
In summary, we provide a systematic quantification of tissue-specific clearances and the role of FcRn protection in the individual tissues. This work demonstrates the unique capability of a PBPK modeling approach to investigate and integrate physiologic processes in a quantitative manner. The intrinsic tissue clearances we derived can be implemented in PBPK models for therapeutic IgG antibodies as fractions of the measured systemic clearance might allow quantitative estimates of the effects of FcRn engineering and targeting on the specific tissue disposition of monoclonal antibodies.
Material and methods
Biodistribution data
The biodistribution raw data used for this analysis were kindly provided by Yip and colleagues.Citation20 In short, 2 FcRn variants (FcRn WT and FcRn-) of I-125 and In-111 labeled humanized anti-gD IgG1 antibodies were intravenously (i.v.) administered to normal CD-1 mice at a dose of 100 μg per mouse. Plasma and terminal tissue samples were taken from brain, gut (small intestine, large intestine and stomach), heart, kidney, liver, lung, muscle, skin and spleen. Available time points for the tissue PK are 6 h, 24 h, 168 h and, additionally 336 h for I-125. Three mice were measured at each timepoint. For 2 individuals, late timepoints were removed from the analysis because of obvious systematic deviation from other measured values. Both showed systematically lower concentrations in all tissues except in the liver, in which higher concentrations were measured. Overall, this biodistribution study provided 4 distinct data sets, FcRn WT/I-125, FcRn WT/In-111, FcRn−/I-125 and FcRn−/In-111.
PBPK modeling approach
The PBPK model used in this analysis is partly inspired by the structure proposed by Ferl et al (e.g., FcRn implementation)Citation25 into which they later integrated a description of the biodistribution of In-111-labeled antibody fragments.Citation21 While Ferl et al. used a distribution submodel to account for disposition of In-111-labeled catabolites, we used a retention compartment with no re-distribution of degraded In-111-labeled antibodies. In brief, each tissue in the model is represented by vascular, interstitial and endosomal spaces. The dose is administered i.v. into a distinct plasma compartment, which accounts for venous and arterial plasma. Distribution of antibodies from the plasma compartment to the vascular tissue space occurs by arterial plasma flow. From the tissue vascular space, the compound extravasates into the tissue interstitial space, flows out with the venous plasma or is taken up into the endosomal space. Extravasation from plasma is described using the 2 pore formalism.Citation33 The antibodies are removed from the interstitial space by tissue lymph flow and are returned to the plasma. After unspecific uptake into the endosomal space, low pH allows antibodies to bind to the FcRn receptor, forming an endosome. Antibodies bound to the FcRn are recycled back to the vascular space, whereas free antibodies are degraded in lysosomes.
The Ferl model was adapted in several ways to simultaneously analyze the available biodistribution data of I-125- and In-111-labeled antibodies: 1) endosomal uptake and FcRn-binding/-recycling were added to all tissues, 2) free antibody was degraded in all tissues, following endosomal uptake and a first order degradation process, 3) a label retention compartment was added to each tissue to account for signal accumulation relevant for In-111, 4) a first-order removal rate of In-111 from the cell was estimated, allowing the label to be eliminated after some time due to transport across the cell membrane or cell turnover, for instance, and 5) a rest of body compartment was added to account for tissues with no measurements (e.g., adipose, bone). Due to the rapid release from the cells and removal of I-125 from the system after IgG degradation, the effect of released free iodine label on the measured signal is assumed to be negligible. A schematic overview of the whole body and tissue model is given in . All model differential equations are provided as supplemental material.
Parameter estimation
The PBPK model was built in the Simbiology toolbox of MATLAB 2016a. All 4 antibody biodistribution data sets were simultaneously fitted to estimate unknown tissue specific parameters: convective uptake, lymph flows, FcRn salvage capacity, residual plasma volumes, endosomal uptake. Estimated systemic parameters were: marker degradation, plasma volume, FcRn recycling and degradation rate. The binding affinity to FcRn for the 2 FcRn variants was fixed to the values reported in Yip et al. to simultaneously fit FcRn WT and FcRn− data with a kon of 3.69e−4 mL/(min * nM) and 7.38e−5 mL/(min * nM) and koff of 0.222/min and 6.876/min for FcRn WT and FcRn−, respectively.Citation20 When sufficient reliable information was found, parameters were fixed to their reported physiologic values (e.g., plasma flows, organ weights, etc.).Citation20,25,34-36 A table containing all fixed physiologic parameter values and their sources can be found in the supplementary material (Table S1/S2). Parameters were estimated using fminsearchbound and lsqnonlin fitting algorithms, and in cases in which some prior knowledge was available, boundaries were used to define reasonable parameter values ranges (residual plasma fractions, plasma volume).
Assessment of tissue-specific intrinsic clearances
We used the properties of the 2 radiolabels, In-111 with a long intracellular residual time and non-residualizing I-125, and the extension of the classical PBPK model for therapeutic proteins with retention compartments to assess the intrinsic tissue clearances for each tissue. The total amount of degraded compound in the corresponding tissue was summed over the simulated time span. The simulation time chosen was long enough (125 d) to ensure that the sum of degraded compound in all tissues was equal to the injected dose. The total degraded amount in a specific tissue was derived by simulating the corresponding collecting compartment that accumulates the amount of degraded antibody over the simulated time. For each tissue, the fractional contribution to antibody degradation was derived by dividing the total degraded amount in the tissue by the initial injected dose. The systemic clearance was assessed by NCA analysis based on the plasma concentration profile (using Phoenix WinNonlin 6.4, Certara L.P.). Finally, multiplying the derived fractional degradation of each tissue with the total systemic clearance allowed the calculation of tissue intrinsic clearances for the FcRn WT and FcRn− variants. The protective capability of FcRn in each tissue was derived from the differences in tissue intrinsic clearances between the FcRn WT and FcRn− IgGs.
Disclosure of potential conflicts of interest
Ludivine Fronton is now used at Bayer AG. Findings and conclusions in this work are those of the authors and do not necessarily represent the view of Bayer AG.
Supplementary_Material.docx
Download MS Word (76.2 KB)Acknowledgments
We sincerely thank C. Andrew Boswell, Gregory Z. Ferl and Victor Yip from Genentech, Inc. for providing the experimental raw data and sharing information concerning the experimental work. Furthermore we thank Wilhelm Huisinga (University of Potsdam) for the helpful advice and inputs and Laura Gullett for useful discussions regarding fitting algorithms.
References
- Datta-Mannan A, Lu J, Witcher DR, Leung D, Tang Y, Wroblewski VJ. The interplay of non-specific binding, target-mediated clearance and FcRn interactions on the pharmacokinetics of humanized antibodies. MAbs 2015; 7(6):1084-93; PMID:26337808; https://doi.org/10.1080/19420862.2015.107519
- Wohlrab J. Pharmacokinetic characteristics of therapeutic antibodies. J Dtsch Dermatol Ges 2015; 13(6):530-4; PMID:26018364; https://doi.org/10.1111/ddg.12648
- Diao L, Meibohm B. Tools for predicting the PK/PD of therapeutic proteins. Expert Opin Drug Metab Toxicol 2015; 11(7):1115-25; PMID:25936400; https://doi.org/10.1517/17425255.2015.1041917
- Waldmann TA, Strober W. Metabolism of immunoglobulins. Prog Allergy 1969; 13:1-110; PMID:4186070
- Ward ES, Zhou J, Ghetie V, Ober RJ. Evidence to support the cellular mechanism involved in serum IgG homeostasis in humans. Int Immunol 2003; 15(2):187-95; PMID:12578848; https://doi.org/10.1093/intimm/dxg018
- Montoyo HP, Vaccaro C, Hafner M, Ober RJ, Mueller W, Ward ES. Conditional deletion of the MHC class I-related receptor FcRn reveals the sites of IgG homeostasis in mice. Proc Natl Acad Sci U S A 2009; 106(8):2788-93; PMID:19188594; https://doi.org/10.1073/pnas.0810796106
- Rath T, Baker K, Dumont JA, Peters RT, Jiang H, Qiao SW, Lencer WI, Pierce GF, Blumberg RS. Fc-fusion proteins and FcRn: Structural insights for longer-lasting and more effective therapeutics. Crit Rev Biotechnol 2015; 35(2):235-54; PMID:24156398; https://doi.org/10.3109/07388551.2013.834293
- Borvak J, Richardson J, Medesan C, Antohe F, Radu C, Simionescu M, Ghetie V, Ward ES. Functional expression of the MHC class I-related receptor, FcRn, in endothelial cells of mice. Int Immunol 1998; 10(9):1289-98; PMID:9786428; https://doi.org/10.1093/intimm/10.9.1289
- Akilesh S, Christianson GJ, Roopenian DC, Shaw AS. Neonatal FcR expression in bone marrow-derived cells functions to protect serum IgG from catabolism. J Immunol 2007; 179(7):4580-8; PMID:17878355; https://doi.org/10.4049/jimmunol.179.7.4580
- Ober RJ, Martinez C, Lai X, Zhou J, Ward ES. Exocytosis of IgG as mediated by the receptor, FcRn: An analysis at the single-molecule level. Proc Natl Acad Sci U S A 2004; 101(30):11076-81; PMID:15258288; https://doi.org/10.1073/pnas.0402970101
- Tzaban S, Massol RH, Yen E, Hamman W, Frank SR, Lapierre LA, Hansen SH, Goldenring JR, Blumberg RS, Lencer WI. The recycling and transcytotic pathways for IgG transport by FcRn are distinct and display an inherent polarity. J Cell Biol 2009; 185(4):673-84; PMID:19451275; https://doi.org/10.1083/jcb.200809122
- Gan Z, Ram S, Vaccaro C, Ober RJ, Ward ES. Analyses of the recycling receptor, FcRn, in live cells reveal novel pathways for lysosomal delivery. Traffic 2009; 10(5):600-14; PMID:19192244; https://doi.org/10.1111/j.1600-0854.2009.00887.x
- Roopenian DC, Akilesh S. FcRn: The neonatal Fc receptor comes of age. Nat Rev Immunol 2007; 7(9):715-25; PMID:17703228; https://doi.org/10.1038/nri2155
- Ober RJ, Martinez C, Vaccaro C, Zhou J, Ward ES. Visualizing the site and dynamics of IgG salvage by the MHC class I-related receptor, FcRn. J Immunol 2004; 172(4):2021-9; PMID:14764666; https://doi.org/10.4049/jimmunol.172.4.2021
- Ghetie V, Hubbard JG, Kim JK, Tsen MF, Lee Y, Ward ES. Abnormally short serum half-lives of IgG in beta 2-microglobulin-deficient mice. Eur J Immunol 1996; 26(3):690-6; PMID:8605939; https://doi.org/10.1002/eji.1830260327
- Chaudhury C, Mehnaz S, Robinson JM, Hayton WL, Pearl DK, Roopenian DC, Anderson CL. The major histocompatibility complex-related Fc receptor for IgG (FcRn) binds albumin and prolongs its lifespan. J Exp Med 2003; 197(3):315-22; PMID:12566415; https://doi.org/10.1084/jem.20021829
- Chen N, Wang W, Fauty S, Fang Y, Hamuro L, Hussain A, Prueksaritanont T. The effect of the neonatal Fc receptor on human IgG biodistribution in mice. MAbs 2014; 6(2):502-8; PMID:24492305; https://doi.org/10.4161/mabs.27765
- Henderson LA, Baynes JW, Thorpe SR. Identification of the sites of IgG catabolism in the rat. Arch Biochem Biophys 1982; 215(1):1-11; PMID:7092219; https://doi.org/10.1016/0003-9861(82)90272-7
- Wright A, Sato Y, Okada T, Chang K, Endo T, Morrison S. In vivo trafficking and catabolism of IgG1 antibodies with Fc associated carbohydrates of differing structure. Glycobiology 2000; 10(12):1347-55; PMID:11159927; https://doi.org/10.1093/glycob/10.12.1347
- Yip V, Palma E, Tesar DB, Mundo EE, Bumbaca D, Torres EK, Reyes NA, Shen BQ, Fielder PJ, Prabhu S, et al. Quantitative cumulative biodistribution of antibodies in mice: Effect of modulating binding affinity to the neonatal Fc receptor. MAbs 2014; 6(3):689-96; PMID:24572100; https://doi.org/10.4161/mabs.28254
- Ferl GZ, Kenanova V, Wu AM, DiStefano JJ, 3rd. A two-tiered physiologically based model for dually labeled single-chain Fv-Fc antibody fragments. Mol Cancer Ther 2006; 5(6):1550-8; PMID:16818514; https://doi.org/10.1158/1535-7163.MCT-06-0072
- Press OW, Shan D, Howell-Clark J, Eary J, Appelbaum FR, Matthews D, King DJ, Haines AM, Hamann P, Hinman L, et al. Comparative metabolism and retention of iodine-125, yttrium-90, and indium-111 radioimmunoconjugates by cancer cells. Cancer Res 1996; 56(9):2123-9; PMID:8616860
- Tsai SW, Li L, Williams LE, Anderson AL, Raubitschek AA, Shively JE. Metabolism and renal clearance of 111In-labeled DOTA-conjugated antibody fragments. Bioconjug Chem 2001; 12(2):264-70; PMID:11312688; https://doi.org/10.1021/bc0000987
- Rogers BE, Franano FN, Duncan JR, Edwards WB, Anderson CJ, Connett JM, Welch MJ. Identification of metabolites of 111In-diethylenetriaminepentaacetic acid-monoclonal antibodies and antibody fragments in vivo. Cancer Res 1995; 55(23 Suppl):5714s-20s; PMID:7493333
- Ferl GZ, Wu AM, DiStefano JJ, 3rd. A predictive model of therapeutic monoclonal antibody dynamics and regulation by the neonatal Fc receptor (FcRn). Ann Biomed Eng 2005; 33(11):1640-52; PMID:16341929; https://doi.org/10.1007/s10439-005-7410-3
- Garg A, Balthasar JP. Physiologically-based pharmacokinetic (PBPK) model to predict IgG tissue kinetics in wild-type and FcRn-knockout mice. J Pharmacokinet Pharmacodyn 2007; 34(5):687-709; PMID:17636457; https://doi.org/10.1007/s10928-007-9065-1
- Urva SR, Yang VC, Balthasar JP. Physiologically based pharmacokinetic model for T84.66: A monoclonal anti-CEA antibody. J Pharm Sci 2010; 99(3):1582-600; PMID:19774657; https://doi.org/10.1002/jps.21918
- Baxter LT, Zhu H, Mackensen DG, Jain RK. Physiologically based pharmacokinetic model for specific and nonspecific monoclonal antibodies and fragments in normal tissues and human tumor xenografts in nude mice. Cancer Res 1994; 54(6):1517-28; PMID:8137258
- Fan YY, Avery LB, Wang M, O'Hara DM, Leung S, Neubert H. Tissue expression profile of human neonatal Fc receptor (FcRn) in Tg32 transgenic mice. MAbs 2016; 8(5):848-53; PMID:27104806; https://doi.org/10.1080/19420862.2016.1178436
- Chen Y, Balthasar JP. Evaluation of a catenary PBPK model for predicting the in vivo disposition of mAbs engineered for high-affinity binding to FcRn. Aaps J 2012; 14(4):850-9; PMID:22956476; https://doi.org/10.1208/s12248-012-9395-9
- Deng Y, Lei M, Zhang W, Li W, Zhou E, Liu Z, Qi C. Monoclonal antibodies directed against Fpr3 protein as molecular chaperones. Hybridoma (Larchmt) 2011; 30(5):491-3; PMID:22008079; https://doi.org/10.1089/hyb.2011.0053
- Shah DK, Betts AM. Antibody biodistribution coefficients: Inferring tissue concentrations of monoclonal antibodies based on the plasma concentrations in several preclinical species and human. MAbs 2013; 5(2):297-305; PMID:23406896; https://doi.org/10.4161/mabs.23684
- Rippe B, Haraldsson B. Transport of macromolecules across microvascular walls: The two-pore theory. Physiol Rev 1994; 74(1):163-219; PMID:8295933
- Shah DK, Betts AM. Towards a platform PBPK model to characterize the plasma and tissue disposition of monoclonal antibodies in preclinical species and human. J Pharmacokinet Pharmacodyn 2012; 39(1):67-86; PMID:22143261; https://doi.org/10.1007/s10928-011-9232-2
- Davies B, Morris T. Physiological parameters in laboratory animals and humans. Pharm Res 1993; 10(7):1093-5; PMID:8378254; https://doi.org/10.1023/A:1018943613122
- Brown RP, Delp MD, Lindstedt SL, Rhomberg LR, Beliles RP. Physiological parameter values for physiologically based pharmacokinetic models. Toxicol Ind Health 1997; 13(4):407-84; PMID:9249929; https://doi.org/10.1177/074823379701300401